Enhancing Machine Learning Training in Photos Technology with ChatGPT
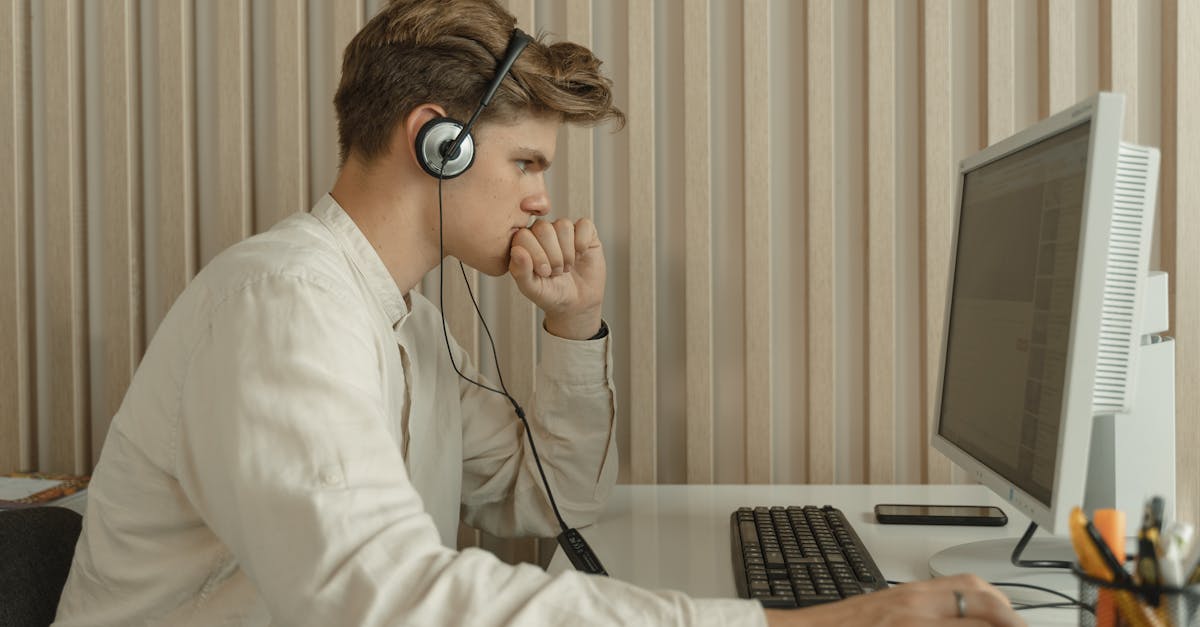
Machine learning and artificial intelligence have been transforming the field of photography, enabling computers to understand and process images in remarkable ways. From object detection and recognition to image classification and generation, training machine learning models for photo technologies has become an essential aspect of advancing this domain.
Introducing ChatGPT-4
OpenAI's ChatGPT-4, the latest iteration of their language model, has proven to be a powerful tool in various domains, including machine learning model training. With its natural language processing capabilities, ChatGPT-4 can assist in developing guidelines for training machine learning models specifically related to photo technologies.
Workflow and Best Practices
When training machine learning models for photo technologies, it is crucial to follow a systematic workflow and incorporate best practices to ensure effective and accurate results. ChatGPT-4 can provide valuable insights and guidelines in this regard. Here are some key points to consider:
- Data Collection: Acquiring a diverse and comprehensive dataset is essential. ChatGPT-4 can suggest suitable sources for datasets and can recommend methods for data augmentation to improve the robustness of the model.
- Preprocessing and Feature Extraction: Before training the model, preprocessing the images and extracting relevant features are pivotal. ChatGPT-4 can help in identifying appropriate preprocessing techniques such as resizing, normalization, and noise reduction. Moreover, it can provide insights into feature extraction methods like convolutional neural networks (CNNs) and transfer learning.
- Model Selection: Choosing the right architecture for the machine learning model is crucial for achieving optimal performance. ChatGPT-4 can suggest popular architectures like ResNet, Inception, and DenseNet, and help in understanding their pros and cons based on the specific photo technology task.
- Training and Evaluation: ChatGPT-4 can provide guidance on training the model efficiently, including setting hyperparameters, optimization techniques, and regularization methods. Additionally, it can suggest appropriate evaluation metrics to assess the model's performance accurately.
- Fine-Tuning and Transfer Learning: For improved performance on specific tasks, fine-tuning and transfer learning techniques can be employed. ChatGPT-4 can advise on how to leverage pre-trained models effectively and fine-tune them for specific photo technologies.
- Iterative Approach: Training machine learning models often requires an iterative approach. ChatGPT-4 can assist in developing a feedback loop to analyze and refine the model's performance through continuous evaluation and retraining.
Future Developments and Ethical Considerations
As photo technologies continue to evolve, it is crucial to stay updated with the latest advancements. ChatGPT-4 can aid in exploring cutting-edge techniques such as generative adversarial networks (GANs), style transfer, and image segmentation. Furthermore, it can highlight ethical considerations surrounding photo technologies, including bias detection and mitigation, privacy concerns, and fairness in algorithmic decision-making.
In conclusion, the role of machine learning in photo technologies is expanding rapidly. With ChatGPT-4's assistance, developers and researchers can harness its natural language processing capabilities to access valuable insights and guidelines for training machine learning models specifically tailored to photo technologies. By leveraging this powerful technology, advancements in photo-related applications are expected to accelerate, driving innovation in various domains.
Comments:
Thank you all for joining the discussion on my article. I'm excited to hear your thoughts on enhancing machine learning training with ChatGPT!
Great article, Edwin! The combination of machine learning training and ChatGPT sounds really promising. Can you share any examples of how this technology has been utilized so far?
Hi Edwin, thanks for the informative article. I agree with Susan, examples would be helpful to understand the potential applications better.
Certainly, Susan and Michael! One example of using ChatGPT in machine learning training for photos technology is enhancing automated image captioning systems. By applying ChatGPT during the training process, we can improve the system's ability to generate accurate and contextually relevant captions.
This is fascinating, Edwin! Has there been any research on the effectiveness of using ChatGPT in machine learning training compared to traditional methods?
Absolutely, Lisa! Several research studies have shown promising results. ChatGPT helps to introduce natural language interactions during training, allowing the model to learn and improve from a more conversational and context-rich perspective.
Interesting concept, Edwin. Are there any particular challenges or limitations when incorporating ChatGPT in machine learning training for photos technology?
Great question, Andrew! One challenge is the potential introduction of biases or incorrect information during the training process. It requires careful moderation and fine-tuning to ensure the quality and reliability of the trained models.
I see, thanks for explaining, Edwin. It's essential to address bias issues in AI systems. How do you tackle this challenge?
Absolutely, Andrew. We employ a combination of human reviewers and an iterative feedback process to continuously improve and address biases. The training data is carefully curated, and models go through multiple rounds of evaluation to ensure fairness and inclusivity.
Edwin, could you explain how ChatGPT influences the training time in machine learning for photos technology? Does it significantly increase the overall training duration?
Great question, Gregory! The training time can be increased to some extent when incorporating ChatGPT due to the conversational nature of feedback it provides. However, advancements in hardware and optimization techniques have minimized the impact on training duration, making it more manageable.
Hi Edwin! Is there any way to use ChatGPT to improve the accuracy of machine learning models in real-time scenarios?
Certainly, Alice! ChatGPT can be employed in interactive tools that allow human operators to guide ML models in real-time scenarios. This guidance helps improve accuracy and ensures the models adapt more effectively to dynamic environments.
I assume ChatGPT requires a large amount of training data. Are there any recommendations on the ideal dataset size and quality for effective machine learning training?
Good point, Jennifer! While ChatGPT benefits from a substantial amount of training data, the focus should be on quality rather than just the size. However, a larger and diverse dataset generally helps in capturing a wider range of knowledge and producing more robust ML models.
Edwin, how does ChatGPT handle user queries or interactions differently from traditional chatbot models?
Good question, Sarah! ChatGPT aims to provide more coherent and context-aware responses compared to traditional chatbot models. It can understand and maintain longer-term conversational context, resulting in more accurate and relevant replies to user queries.
Do you envision ChatGPT being used in other domains apart from photos technology?
Absolutely, Robert! While my article focused on enhancing machine learning training in photos technology, the applications of ChatGPT extend to various domains. It can be utilized in natural language processing, customer support, content generation, and much more.
Edwin, what are the prerequisites or technical skills required to implement ChatGPT in machine learning training effectively?
Good question, Daniel! Implementing ChatGPT in machine learning training requires a strong understanding of natural language processing, deep learning frameworks, and experience working with large-scale datasets. Familiarity with model fine-tuning and deployment processes are also essential.
Hi Edwin! Are there any privacy concerns associated with using ChatGPT in machine learning training?
Great question, Emily! Privacy concerns are indeed important. Careful anonymization and data protection measures are implemented during the training process to ensure users' privacy is respected. We strive to uphold the highest standards of data security and privacy.
Edwin, what are your thoughts on potential ethical implications when incorporating ChatGPT in machine learning training?
Valid point, Mark. Ethical implications are a crucial aspect in AI development. Transparency, fairness, and responsible use of AI technologies are at the core of our approach. Continuous evaluation, bias detection, and human review processes help mitigate potential ethical concerns.
I wonder if ChatGPT can handle multiple languages. Is it feasible to train models for various languages simultaneously?
Absolutely, Sophia! ChatGPT can handle multiple languages. While training models for various languages simultaneously is feasible, it's essential to consider language-specific nuances and adequately curate training data to ensure optimal performance for each language.
Hi Edwin! How does ChatGPT handle uncommon or specific terminology in different domains during machine learning training?
Good question, Nathan! ChatGPT understands and utilizes context to handle uncommon or specific terminology. However, ensuring proper exposure to domain-specific terms during training is important to enhance accuracy in those specific areas.
Edwin, what are your future plans for advancing the use of ChatGPT in machine learning training for photos technology?
Thanks for the question, Olivia! We plan to focus on further improving the interaction capabilities of ChatGPT with machine learning in the photos technology domain. Expanding its understanding of visual context and refining its conversational abilities would be key priorities.
Edwin, what is the most exciting or innovative use case you have encountered while exploring ChatGPT in machine learning training?
Great question, Sophie! One of the most exciting use cases I've encountered is the integration of ChatGPT in virtual reality experiences. It allows users to engage in realistic and interactive conversations within VR simulations, opening up new possibilities for learning and entertainment.
Thanks for sharing your insights, Edwin! I'm curious, does ChatGPT have any limitations in handling complex visual content during machine learning training?
Good question, Matthew! While ChatGPT can handle complex visual content to some extent, its primary strength lies in textual understanding and generation. To effectively incorporate complex visual content, a multimodal approach combining vision and language models might be more suitable.
Hi Edwin! What are some potential practical applications of ChatGPT in the field of photos technology?
Good question, Amy! Some practical applications include improving automated image captioning, generating image texts for visually impaired individuals, enhancing content generation in photo editing software, and optimizing search and recommendation systems for images.
Edwin, what are the next steps after machine learning training with ChatGPT for photos technology? How is the trained model deployed or utilized?
Good question, Christopher! After training, the ChatGPT model is deployed in various applications, such as image captioning systems, photo editing software, or even integrated into online platforms for interactive image-based interactions. Deployment involves mapping user input to the model and processing the generated responses.