Enhancing Machine Translation on the Semantic Web with ChatGPT
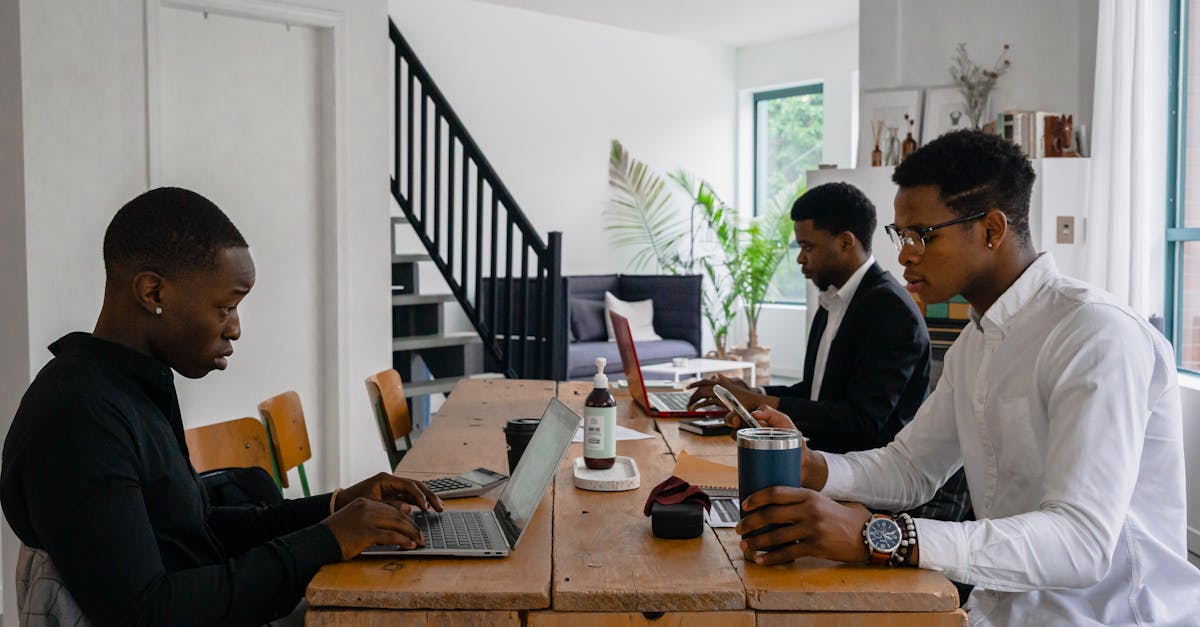
Machine translation has made significant advancements in recent years, with the advent of new technologies and techniques. One such technology that has emerged as a powerful tool in improving translation quality is the Semantic Web. By leveraging the semantic knowledge encoded in the web of data, machine translation systems are now able to better understand the underlying meaning of the source and target languages, resulting in improved translations.
The Semantic Web
The Semantic Web is an extension of the World Wide Web, designed to enable machines to understand and process the information on the web. It is built on the idea of encoding meaning and relationships between entities in a structured and machine-readable format. This is achieved through the use of resource description frameworks (RDF), ontologies, and linked data, among other technologies.
Machine Translation with Semantic Web
Machine translation systems traditionally relied on statistical models and rule-based approaches to translate sentences from one language to another. While these methods have shown some success, they often fail to capture the nuanced meaning and context of the text accurately. This is where the Semantic Web comes into play.
By incorporating semantic knowledge from the web of data, machine translation systems can go beyond word-for-word translations and understand the underlying concepts being expressed. This allows for better disambiguation of word meanings, handling of idiomatic expressions, and capturing the intended meaning of the source text.
Improved Translation Quality
The usage of the Semantic Web in machine translation has led to significant improvements in translation quality. By leveraging the vast amounts of structured data available on the web, machine translation systems can tap into a wealth of knowledge about various domains and topics, improving the accuracy and fluency of translations.
For example, by understanding the semantic relationships between entities, machine translation systems can accurately translate terms that have multiple meanings or rely on context. This avoids the common issue of generating incorrect translations due to word ambiguity.
Challenges and Future Directions
Despite its potential, integrating the Semantic Web into machine translation is not without challenges. One of the primary challenges is the availability and quality of semantic resources. Developing comprehensive ontologies and maintaining semantic data can be time-consuming and resource-intensive.
Additionally, the complex nature of natural language and the diversity of languages pose challenges in accurately representing meaning in a machine-readable format. Further research and development efforts are needed to overcome these challenges and unlock the full potential of the Semantic Web in machine translation.
Conclusion
The Semantic Web has emerged as a powerful technology in the field of machine translation. By incorporating semantic knowledge from the web of data, machine translation systems can better understand the underlying meaning of the source and target languages, resulting in improved translations. While there are challenges to overcome, the potential benefits of the Semantic Web in machine translation are significant, offering the promise of more accurate and fluent translations in the future.
Comments:
Great article! Machine translation has come a long way.
I completely agree, Mark. It's impressive how well machine translation has improved.
Indeed, the advancements in machine translation are remarkable. However, there are still some challenges to overcome.
Robert, what challenges do you think are most critical for machine translation?
Emily, one challenge is accurately capturing nuances and context, especially in languages with complex grammatical structures.
Thank you all for your comments so far! Robert, you bring up a valid point. Context is vital in achieving accurate machine translation.
I believe machine learning models like ChatGPT can address some of these contextual challenges.
Absolutely, Julia! Models like ChatGPT have shown promise in understanding and generating contextually relevant translations.
I wonder if ChatGPT can also help with translating idiomatic expressions accurately?
That's an excellent point, Anna. Idiomatic expressions can be particularly challenging for machine translation systems.
Tiffani, do you have any insights into how ChatGPT handles idiomatic expressions?
Maria, while ChatGPT has shown some improvements in this area, idiomatic expressions still pose difficulties. Further research is needed to enhance idiomatic translation.
I think incorporating multilingual knowledge bases can contribute to better semantic understanding in machine translation.
Gregory, I agree. Leveraging multilingual knowledge bases can help improve the core semantic understanding of machine translation models.
Absolutely, Robert and Gregory. Access to comprehensive multilingual knowledge can enhance the accuracy and semantic sense in machine translations.
I'm curious about the impact of using the Semantic Web in machine translation. Can it enhance the quality of translations?
Sophia, the Semantic Web can play a significant role in improving machine translation. By leveraging structured data and ontologies, it enables better understanding of context and meaning.
That sounds fascinating, Tiffani! It's exciting to see how advanced technologies are shaping the future of translation.
Indeed, Sophia! The field of machine translation holds tremendous potential, and it's only going to get better with time.
Is there any risk of bias or misinformation when using ChatGPT in machine translation?
Good question, Daniel. Like any AI system, bias and misinformation can be a concern. Continuous evaluation and improvement are crucial to mitigate those risks.
Thank you for addressing my concern, Tiffani. Rigorous monitoring and ethical guidelines will undoubtedly play a vital role.
Tiffani, what are your thoughts on the future of machine translation?
Emily, I believe machine translation will continue to advance, driven by innovations like ChatGPT and the increasing availability of multilingual data. However, human expertise will still be crucial for achieving the highest quality translations.
Tiffani, what are your thoughts on the potential applications of machine translation beyond textual content?
Daniel, machine translation can have exciting applications beyond text, such as speech translation, real-time interpretation, and even integration with augmented reality for multilingual communication.
That sounds fascinating, Tiffani! The possibilities for machine translation are truly limitless.
Indeed, Daniel. Machine translation has the potential to open doors to seamless communication across languages in various domains.
Absolutely, Robert. As technology continues to advance, machine translation will undoubtedly enable greater connectivity and collaboration.
Tiffani, what is the role of neural machine translation models like ChatGPT in preserving the linguistic diversity of languages?
Daniel, neural machine translation models like ChatGPT can contribute to preserving linguistic diversity by making translations more accessible and facilitating cross-cultural exchange. They can help in bridging language gaps and raising awareness of lesser-known languages.
That's fantastic, Tiffani! It's heartening to see how technology can contribute to language preservation and cultural appreciation.
Absolutely, Daniel. The preservation of linguistic diversity is instrumental in recognizing and valuing different cultures.
Indeed, Robert. Promoting linguistic diversity enhances cultural understanding and enables meaningful interactions across communities.
Are there any limitations to machine translation that we should be aware of?
Samuel, yes, there are limitations. Machine translation may struggle with complex language structures, cultural nuances, and accurately conveying the emotions behind a text.
Thank you for highlighting those limitations, Tiffani. It's important to be aware of the areas where machine translation may fall short.
I agree, Samuel. It's crucial to have realistic expectations and understand the current capabilities of machine translation systems.
Absolutely, Robert. Transparency about the strengths and limitations of machine translation systems is key.
Tiffani, what are some ways to assess the quality of machine translations?
Samuel, evaluating machine translation quality involves considering factors like fluency, accuracy, adequacy, and consistency. Human evaluations, automated metrics, and user feedback are commonly used methods to assess the performance of machine translation systems.
Thank you for the clarification, Tiffani. Robust evaluation measures are crucial in gauging the effectiveness of machine translations.
Indeed, Samuel. Continuous evaluation and improvement are essential for advancing the quality of machine translation systems.
Do you think machine translation will ever surpass human translators?
Alexandra, while machine translation can provide quick and convenient translations, human translators bring unique skills like cultural insights and expertise. I believe machine translation will complement rather than replace human translators.
That's a comforting thought, Tiffani. Human involvement is vital to ensure accurate and culturally appropriate translations.
Well said, Alexandra. Human translators play a significant role in maintaining high-quality translations, especially in sensitive domains like legal or medical.
Indeed, Robert. Domain-specific expertise and understanding nuances are areas where human translators excel.
I appreciate the insightful discussion here! It's fascinating to see how machine translation continues to evolve.
Absolutely, Anna! The progress we've witnessed in the field of machine translation is truly remarkable.
Thank you, Anna and Gregory. The dialogue around machine translation helps to foster a better understanding of its potential and limitations.
I'm curious about the computational resources required for training and deploying models like ChatGPT. Are they significant?
Sophia, training and deploying large language models like ChatGPT indeed require substantial computational resources. However, advancements in hardware and model optimization techniques are making it more accessible.
That's good to know, Tiffani. As accessibility improves, more organizations and individuals can leverage these powerful translation technologies.
Sophia, increased accessibility can certainly contribute to the democratization of language and cross-cultural understanding.
Precisely, Julia. Making sophisticated translation tools more accessible can bridge linguistic barriers and foster global communication.
Tiffani, what are the implications of machine translation for cross-cultural understanding?
Julia, machine translation can significantly contribute to cross-cultural understanding by enabling individuals from different linguistic backgrounds to communicate effectively. It fosters empathy, breaks down barriers, and promotes international collaboration.
That's wonderful to hear, Tiffani. Machine translation's role in promoting cross-cultural understanding is truly transformative.
Absolutely, Julia. Cross-cultural understanding is crucial in today's interconnected world, and machine translation assists in bridging those cultural gaps.
Indeed, Robert. Machine translation contributes to a more inclusive and interconnected global society.
Tiffani, how can we ensure that machine translation minimizes bias and is culturally sensitive?
Sophia, addressing bias and cultural sensitivity requires diverse training data, continuous evaluation, and active involvement of linguists and cultural experts to ensure translations are accurate and respectful.
Thank you, Tiffani. Collaboration between technologists, linguists, and communities is essential for building inclusive and unbiased machine translation systems.
Sophia, I couldn't agree more. Building inclusive and culturally respectful machine translation systems is a collective responsibility.
Absolutely, Robert. By working together, we can ensure that machine translation aligns with ethical and inclusive principles.
Tiffani, do you envision a future where machine translation becomes seamless and imperceptible to the user?
Sophia, achieving seamless and imperceptible machine translation is an ongoing goal. As models improve and technology advances, it's within the realm of possibility to provide translations that feel natural and indistinguishable from human-generated content.
That's fascinating, Tiffani! A future where machine translations are practically indistinguishable from human translations would be groundbreaking.
I agree, Sophia. Advancements in technology can lead to more fluid and seamless cross-linguistic communication.
Absolutely, Robert. Progress in machine translation holds the potential to revolutionize how we communicate across languages.
It's fantastic to envision a future where language is no longer a barrier for effective communication.
Certainly, Maria. Advancements in machine translation bring us closer to that vision of a more connected and inclusive world.
Thank you, Tiffani, for facilitating this discussion. It's been an insightful conversation.
Tiffani, what steps can be taken to improve the real-time aspect of machine translation?
Maria, improving the real-time aspect of machine translation involves optimizing computational efficiency, reducing latency, and accelerating inference. Advancements in hardware and algorithmic optimizations can contribute to faster and more responsive translations.
Thank you for the insights, Tiffani. Real-time translations can make a significant impact in various scenarios, like international conferences or online meetings.
Absolutely, Maria. Real-time translations hold immense potential in facilitating global communication and breaking down language barriers.
Are there any ethical considerations specific to machine translation that we should be mindful of?
Emily, ethical considerations in machine translation revolve around privacy, misinformation, cultural sensitivity, and bias. It's crucial to address these issues to ensure responsible and fair translations.
Thank you for raising awareness, Tiffani. Machine translation should prioritize ethical practices and minimize potential harms.
Tiffani, do you think there will be a universal language translation system in the future?
Carlos, while a universal language translation system would be ideal, language is deeply rooted in culture and constantly evolving. Achieving completely accurate translations for all languages and nuances remains a significant challenge.
I see your point, Tiffani. The complexities of languages make a universal translation system a formidable task.
Indeed, Carlos. Language is not just about words; it carries cultural significance and historical context.
That's absolutely right, Robert. The rich cultural diversity reflected in languages makes translation an intricate process.
Tiffani, how can we ensure that machine translation tools are accessible to individuals with disabilities?
Carlos, ensuring accessibility for individuals with disabilities involves considering factors like screen readers, multilingual speech recognition, and providing inclusive user interfaces. Collaborating with accessibility experts can help create more inclusive machine translation tools.
Thank you for highlighting the importance of accessibility, Tiffani. Technology should be designed with inclusivity in mind.
Well said, Carlos. Accessible machine translation tools can empower individuals with disabilities and bridge communication barriers.
Absolutely, Robert. Accessibility and inclusivity should be central in the development and deployment of machine translation systems.
Tiffani, what role can users play in improving machine translation quality?
Emily, user feedback and contributions are invaluable in enhancing machine translation quality. Reporting inaccuracies, participating in translations, and providing context-specific insights can all contribute to improving future translations.
Thank you for emphasizing the importance of user engagement, Tiffani. Together, we can collectively contribute to refining machine translation systems.
Well said, Emily. User involvement plays a significant role in bridging the gap between machine translation systems and real-world linguistic needs.
Indeed, Robert. User-centric approaches are vital for designing effective and user-friendly machine translation systems.
Thank you all for this engaging discussion! It's enlightening to explore the possibilities and challenges of machine translation.
Thank you, Anna. I appreciate everyone's valuable insights and contributions to the conversation.
Agreed, Tiffani. This discussion has provided a great platform to exchange ideas and knowledge.
Thank you all for joining this discussion! Your insights and questions have made it a thoughtful forum on the future of machine translation.
I hope this article and our conversation shed light on the advancements, challenges, and exciting possibilities in the field.
If you have any further questions or thoughts, please feel free to share them.
Once again, thank you all for your valuable participation!