Enhancing Manufacturing Processes with ChatGPT: Empowering Machine Learning Integration in the Manufacturing Industry
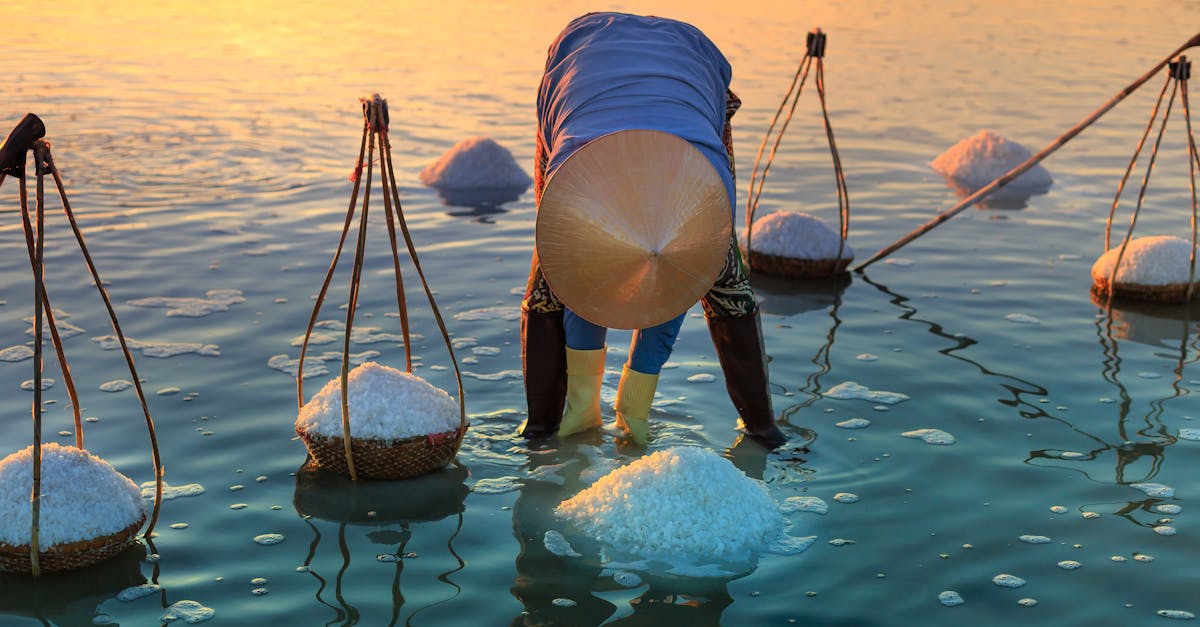
Manufacturing processes often involve complex systems, large amounts of data, and various sources of information. To optimize these processes and improve efficiency, machine learning integration has emerged as a powerful tool. By leveraging machine learning models, manufacturers can derive actionable insights from their data, leading to better decision making and improved outcomes.
What is Machine Learning Integration?
Machine learning integration refers to the process of incorporating machine learning algorithms and models into existing manufacturing systems. It involves training these models using historical manufacturing data and using them to make predictions, detect anomalies, and automate decision-making processes.
Benefits of Machine Learning Integration in Manufacturing
The integration of machine learning in manufacturing brings several benefits, including:
- Process optimization: Machine learning models can analyze manufacturing data in real-time to identify inefficiencies, bottlenecks, and areas for improvement. By optimizing processes based on these insights, manufacturers can reduce waste, improve product quality, and enhance overall productivity.
- Predictive maintenance: Machine learning algorithms can analyze sensor data from manufacturing equipment to detect patterns and anomalies. By predicting equipment failures before they occur, manufacturers can schedule maintenance activities proactively, reducing unplanned downtime and minimizing production disruptions.
- Quality control: Machine learning models can analyze large datasets to identify patterns and correlations that human operators might miss. By monitoring and analyzing data from various sensors and devices, manufacturers can detect quality issues early on, preventing defects and ensuring consistent product quality.
- Supply chain optimization: Machine learning integration can help manufacturers optimize their supply chain by predicting demand patterns, optimizing inventory levels, and reducing transportation costs. By leveraging these insights, manufacturers can streamline their operations and improve overall supply chain efficiency.
Challenges and Considerations
While machine learning integration offers significant benefits, there are also challenges and considerations to keep in mind:
- Data quality and availability: Machine learning models rely on large, high-quality datasets for training and accurate predictions. Manufacturers must ensure that they have access to relevant and reliable data before implementing machine learning integration.
- Algorithm selection: Choosing the right machine learning algorithms and models for specific manufacturing processes is crucial. With a wide range of algorithms available, manufacturers must carefully evaluate and select the ones that align with their specific goals and requirements.
- Implementation and integration: Integrating machine learning models into existing manufacturing systems can be complex. It requires collaboration between data scientists, engineers, and domain experts to ensure a seamless integration and effective utilization of the models.
- Continuous improvement: Machine learning models require periodic updating and retraining to adapt to evolving manufacturing processes and changes in data patterns. Manufacturers need to establish processes for continuous improvement and refinement of these models to maintain their effectiveness over time.
Conclusion
Machine learning integration offers a promising opportunity for manufacturers to leverage their data and improve their processes. By integrating machine learning models into manufacturing systems, manufacturers can gain valuable insights, optimize processes, reduce costs, and enhance overall productivity. However, it is essential to address the associated challenges and considerations to ensure successful implementation and long-term benefits.
Comments:
This is a great article that highlights the potential of integrating ChatGPT into manufacturing processes. I can see how it can improve efficiency and decision-making. Looking forward to seeing more advancements in this area.
I agree, Matthew! The use of machine learning in the manufacturing industry can really revolutionize how things are done. It has the potential to optimize production and reduce errors. Exciting times ahead!
I have some concerns about relying too much on machine learning in manufacturing. While it can enhance processes, we must ensure that it doesn't replace human expertise completely. Human oversight is crucial for quality control and adaptability.
Thank you all for your input! I appreciate the feedback. Robert, you raise a valid point. Machine learning should be seen as a tool to complement human expertise rather than replace it entirely. Human oversight and adaptability are indeed essential.
I find the idea of integrating ChatGPT into manufacturing fascinating. It could assist in real-time troubleshooting and provide insights into process optimization. However, we must ensure the reliability and security of the data and algorithms used.
Absolutely, Jennifer! Data security is crucial when integrating machine learning into manufacturing. Safeguarding sensitive information should be a priority to prevent any potential risks.
The integration of ChatGPT in manufacturing processes could also benefit training and skill development. It could assist in providing interactive tutorials and respond to employee queries, promoting continuous learning.
Great point, Mary! ChatGPT can indeed be utilized for employee training and support. It can facilitate on-demand access to information and help in upskilling the workforce.
While machine learning integration in manufacturing seems promising, we must also consider the potential impact on job displacement. We need to find a balance that embraces advancements while ensuring the well-being of the workforce.
Thank you, David. Job displacement is a valid concern. The goal should be to utilize machine learning to improve efficiency and create new opportunities rather than solely focusing on replacing human workers.
I appreciate your response, Lois. It's crucial to consider the ethical implications of machine learning integration as well. We must ensure that algorithms used in manufacturing are unbiased and fair to avoid any unintended consequences.
Absolutely, David. Ethics should always be at the forefront of any technological advancements. It's important to have strict guidelines and regular audits to identify and mitigate any biases in the algorithms.
Lois, could you provide any case studies where ChatGPT has been successfully implemented in manufacturing? It would be interesting to learn about specific use cases and corresponding benefits.
Emily, there have been instances where ChatGPT has been used to improve predictive maintenance in manufacturing, reducing costly downtime. I can share some case studies with you for further insights.
Lois, case studies on predictive maintenance made possible through ChatGPT would be highly beneficial. It's an area where many manufacturing industries face challenges, and insights from successful implementations can guide us.
Lois, that's a valuable case study. Predictive maintenance is critical, and integrating ChatGPT to prevent costly downtime would be a game-changer. I look forward to exploring more successful use cases.
Emily, I'll gladly share case studies on predictive maintenance with you. The successful implementation of ChatGPT in this area has proven to save significant costs and increase the overall efficiency of operations.
Lois, integrating ChatGPT successfully in predictive maintenance can be a significant advantage, especially for complex equipment. The potential for reducing maintenance costs and maximizing uptime is exciting.
Emily, definitely! The predictive maintenance aspect has shown tremendous potential in reducing costs and improving equipment reliability. It's a promising area where ChatGPT shines.
Thank you, Lois. It's reassuring to know that the ethical aspects are being considered. Incorporating unbiased algorithms can greatly contribute to a fairer and more inclusive manufacturing industry.
David, I appreciate your support for ethical considerations. By actively addressing biases and ensuring fairness, we can foster an inclusive and responsible adoption of machine learning in manufacturing.
I'm glad to see the focus on ethics, Lois. Responsible implementation of machine learning in manufacturing is essential to prevent biases, discrimination, and other unintended consequences. Regular audits can help maintain accountability.
David, I couldn't agree more. Responsible implementation and continuous monitoring are key to avoiding unintended consequences and maintaining fairness in machine learning integration.
Indeed, Lois. Regular audits can help identify any potential biases or issues and enable timely corrective measures. It's crucial to continuously evaluate and ensure the fairness and integrity of the systems.
Absolutely, David. Continuous evaluation and corrective measures ensure that the integration of machine learning in manufacturing remains fair, accurate, and unbiased.
You're right, Lois. The quality of data used is fundamental for accurate insights and effective decision-making. Garbage in, garbage out applies here, and establishing data quality protocols is crucial.
Exactly, Daniel. Ensuring high-quality data should be a priority before integrating ChatGPT. This includes data validation, preprocessing, and maintaining data integrity throughout the manufacturing process.
I'm curious about the implementation challenges when integrating ChatGPT into existing manufacturing processes. How adaptable is it? Are there any limitations we should be aware of?
Good question, Daniel! Implementation challenges can vary depending on the specific use case and infrastructure. It would be interesting to hear about any real-world examples and how they have overcome these challenges.
Thank you for addressing my question, Matthew. I agree that real-world examples would provide valuable insights. It would help us understand the impact and feasibility of integrating ChatGPT into different manufacturing sectors.
Matthew, could you share any real-world examples where the implementation of ChatGPT has faced unexpected challenges? It would be helpful in understanding the potential hurdles and mitigating them.
Daniel, unexpected challenges can include handling incomplete or inconsistent data during initial implementation or addressing context-specific nuances in different manufacturing sectors. Flexibility and adaptability are key.
Thank you for elaborating on the potential challenges, Matthew. It's crucial to consider adaptability and data quality to ensure a successful integration of ChatGPT into existing manufacturing processes.
Daniel's question is important. Understanding the adaptability of ChatGPT is crucial before implementing it. It's essential to ensure integration doesn't disrupt existing workflows but enhances them.
Daniel and Emily, you bring up valid concerns. Adaptability is a key aspect. When integrating ChatGPT, substantial planning and testing are necessary to ensure a smooth transition and minimal disruption to existing workflows.
Another challenge to address is the need for continuous monitoring and improvement of the machine learning algorithms. Regular updates and fine-tuning would be required to maximize the benefits and stay up to date with changing data patterns.
You're right, Jennifer. Developing a feedback loop and implementing a robust monitoring system for ChatGPT's algorithms would be crucial. Continuous improvement is necessary to ensure reliable and accurate insights.
I wonder how the introduction of ChatGPT in manufacturing processes would impact the overall learning curve for employees. It could require additional training to effectively utilize and interact with the technology.
Mary, you make a valid point. The introduction of ChatGPT may indeed require some additional training and adjustment. However, with intuitive interfaces and proper guidance, the learning curve can be minimized.
Lois, I agree that with proper guidance, the learning curve for employees can be minimized. User-friendly interfaces and comprehensive training programs would play a crucial role in facilitating effective integration.
Mary, I completely agree. User-friendly interfaces and comprehensive training programs are essential to ensure that employees can effectively utilize ChatGPT for learning and troubleshooting purposes.
Lois, I completely agree. Providing employees with clear instructions and ongoing support would be vital for seamless integration. It would empower them to leverage ChatGPT as a valuable tool.
Monitoring the accuracy and reliability of ChatGPT's algorithms should also involve feedback from users. Gathering user feedback and experiences can help identify areas of improvement and possible biases.
While the benefits of integrating machine learning into manufacturing are evident, we should also consider the potential risks associated with reliance on automation. Cybersecurity vulnerabilities and system failures could pose serious threats.
Robert, you bring up an important concern. Cybersecurity is indeed a crucial aspect to address when integrating machine learning technologies. Robust security measures should be implemented to safeguard against potential risks.
Lois, you're right. Cybersecurity measures should be a top priority. A breach in a manufacturing setting could lead to both financial and physical consequences. Protecting critical systems should always be a priority.
Robert, I fully agree. Cybersecurity threats can have severe consequences, and the manufacturing industry is no exception. Proper measures, like encrypted communications and network segmentation, should be implemented to mitigate risks.
Continuous monitoring also opens avenues for improvement beyond accuracy, like minimizing bias, reducing false positives/negatives, and enhancing the interpretability of ChatGPT's decision-making process.