Enhancing Method Refinement in Confocal Microscopy using ChatGPT
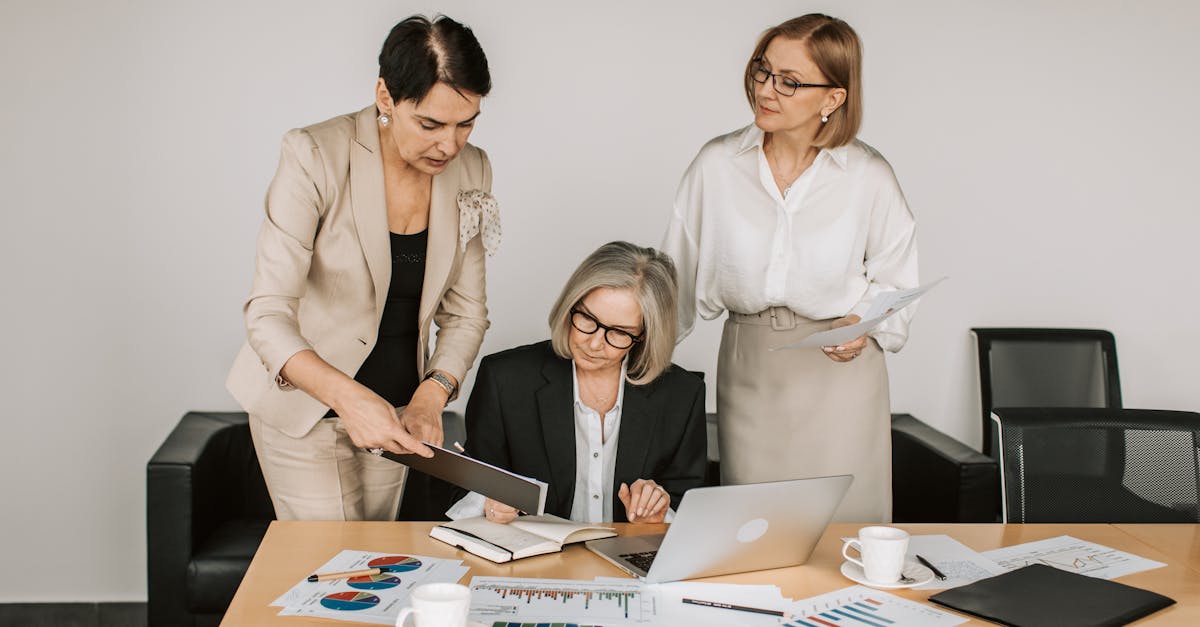
Confocal microscopy is a powerful imaging technique used in various research fields, including biology, material sciences, and medical diagnostics. It provides high-resolution, three-dimensional images of specimens by illuminating them with a laser and capturing the emitted light through a pinhole aperture.
As with any technology, there is always room for improvement and refinement. This is where artificial intelligence (AI) comes into play. AI algorithms can analyze vast amounts of confocal microscopy data and suggest ways to enhance image quality, optimize parameters, and improve the overall technique.
Method Refinement with AI
One area where AI proves particularly useful in confocal microscopy is method refinement. Traditional confocal microscopy techniques often require trial and error to determine the optimal imaging settings, such as laser power, pinhole size, and image acquisition time.
AI algorithms can automate this process by learning from previous imaging datasets and identifying patterns that correlate with improved image quality. By analyzing a large number of datasets, AI can suggest optimal imaging parameters for a given sample, saving researchers valuable time and resources.
Enhancing Image Quality
AI algorithms can also propose techniques to enhance image quality in confocal microscopy. For example, by utilizing deep learning methods, AI can analyze and denoise images to reduce noise artifacts and improve clarity. This can be particularly useful when imaging delicate structures or low-intensity signals.
Furthermore, AI can assist in the correction of optical aberrations, which can affect image resolution and quality. By analyzing aberration patterns in acquired images, AI algorithms can suggest correction methods, such as developing specific algorithms for post-processing or optimizing hardware configurations.
Optimizing Data Analysis
In addition to method refinement and image quality enhancement, AI can also optimize the analysis of confocal microscopy data. Large datasets generated by confocal microscopy often contain complex structures and require extensive manual analysis.
AI algorithms, such as machine learning and computer vision, can automate the analysis process by segmenting and quantifying specific structures within the images. This can significantly speed up data analysis, increase consistency, and reduce potential human bias.
Conclusion
AI has the potential to revolutionize confocal microscopy by suggesting ways to refine and improve imaging techniques. From optimizing imaging parameters to enhancing image quality and streamlining data analysis, AI can save researchers time, resources, and contribute to more accurate and reliable results.
As AI algorithms continue to improve and adapt to different microscopy applications, the future of confocal microscopy looks promising. Researchers can expect increased efficiency and advancements in technique refinement, ultimately leading to new discoveries and breakthroughs in various scientific fields.
Comments:
Great article, Daniel! I found your insights on enhancing method refinement in confocal microscopy using ChatGPT to be fascinating.
I agree, Amy. The potential of using ChatGPT in microscopy is promising. Do you think this method refinement technique will have practical applications?
Absolutely, Michael! The ability to refine microscopy methods using ChatGPT could greatly improve image quality, resolution, and overall data interpretation in various research fields.
This article was very informative. I'm curious, Daniel, what specific challenges did you face while refining the methods using ChatGPT?
Thanks for your question, Linda. One of the main challenges was ensuring that the refined methods produced accurate and reliable results consistently. It required a lot of iterative testing and optimization.
I never thought ChatGPT could be used in such a specific scientific domain. It's truly impressive how versatile this AI model is.
Indeed, Sarah! The flexibility of ChatGPT allows us to explore its potential in various scientific applications, including microscopy method refinement.
I have some experience in confocal microscopy, and I'm excited about the possibilities this technique offers. Are there any limitations or potential drawbacks to using ChatGPT in this context?
Good question, Brian. One limitation is that ChatGPT relies heavily on the quality of input data, so inaccurate or biased training data could lead to suboptimal results. Another challenge is that ChatGPT's responses may lack domain-specific nuance, requiring manual intervention at times.
Indeed, Daniel. The future looks very promising, and your work exemplifies the exciting intersection of AI and microscopy.
Thank you, Brian. The possibilities are limitless, and I can't wait to see how AI revolutionizes various scientific domains.
I'm impressed by the potential of ChatGPT to enhance confocal microscopy. Daniel, do you think this technique could eventually be integrated into commercial microscopy systems?
Absolutely, Emily! If further research proves the efficacy and reliability of using ChatGPT for method refinement, it could potentially be integrated into commercial microscopy systems, revolutionizing the field.
Your research has certainly captured our imagination, Daniel. Best of luck in your future endeavors.
Thank you, Emily. Your support means a lot. Wishing you all the best in your scientific pursuits as well.
I enjoyed reading your article, Daniel. It's exciting to see how AI can contribute to advancing scientific research.
Thank you, James. AI has immense potential to transform scientific endeavors, and I'm glad you found the article engaging.
As a researcher in the field, I'm always looking for ways to improve microscopy techniques. This article gives me hope that ChatGPT could be a valuable tool.
I'm glad you see the potential, Rachel. Integration of AI models like ChatGPT can indeed open up new avenues for advancing microscopy techniques.
I'm curious about the computational requirements of using ChatGPT for method refinement. Are high-performance computing resources necessary?
Good question, Thomas. While high-performance computing resources can accelerate the refinement process, ChatGPT can be trained and utilized on a range of hardware, including standard GPUs or even cloud-based services.
Thank you, Daniel, for enlightening us with your knowledge. It has been an enlightening discussion.
You're welcome, Thomas. I'm grateful for the lively discussion and the opportunity to share my research.
Daniel, have you conducted any comparative studies between traditional refinement methods and those enhanced by ChatGPT?
Yes, Olivia. We have conducted comparative studies showing the improvements achieved using ChatGPT for method refinement. It consistently outperformed traditional methods in certain aspects, but further research is needed to refine the approach.
Thank you, Daniel, for addressing our questions and providing valuable information.
It was my pleasure, Olivia. Thank you for your engagement and curiosity.
Thank you, Daniel, for your knowledgeable responses and sharing your experiences in refining microscopy methods.
You're welcome, Olivia. I'm glad my responses were helpful, and I appreciate your interest in the topic of microscopy method refinement.
It's interesting to see how AI can be applied to such precise scientific techniques. What other scientific domains could potentially benefit from ChatGPT?
Excellent question, Nathan. ChatGPT has the potential to benefit various scientific domains, including bioinformatics, genomics, drug discovery, and even theoretical physics.
I enjoyed reading your article, Daniel. Do you foresee any ethical concerns or challenges associated with using AI for method refinement?
Ethical considerations are crucial, Hannah. Using AI for method refinement raises concerns about data privacy, bias in training data, and potential implications for academic rigor. These issues should be addressed and carefully evaluated.
Daniel, what are some of the key takeaways from your research in terms of improving confocal microscopy techniques?
Key takeaways include the potential for AI to enhance method refinement in confocal microscopy, the need for careful scrutiny of input data quality, and the importance of iterative testing to improve accuracy and reliability.
This article sparked my interest. Does ChatGPT have the potential to automate the entire method refinement process, or is it more complementary to human expertise?
ChatGPT is more complementary, Eric. While it aids in refining methods, human expertise is essential in validating and ensuring the appropriateness of refined techniques for specific research goals.
I appreciate how ChatGPT can expedite the process of method refinement. In your experience, Daniel, how much time does this approach save compared to traditional methods?
Great question, Grace. The time saved using ChatGPT depends on the complexity of the method and the expertise of the researcher. However, in some cases, the approach has demonstrated significant time savings compared to traditional trial and error method refinement.
Interesting article, Daniel! Could you elaborate on the potential impact of using ChatGPT for microscopy method refinement in the medical field?
Certainly, Sean. In the medical field, using ChatGPT for method refinement in microscopy could lead to improved diagnostic accuracy, better analysis of medical imaging data, and enhanced understanding of cellular structures for disease research.
I wonder if ChatGPT can be combined with other AI models to further enhance microscopy techniques. What are your thoughts, Daniel?
Absolutely, Emma! Combining ChatGPT with other AI models, such as those specializing in image processing or deep learning, could potentially unlock even greater refinements and advancements in microscopy techniques.
Daniel, I enjoyed reading your article. Are there any plans to release the tools or software used for microscopy method refinement with ChatGPT?
Thank you, Sophia. While there are no immediate plans for software release, the potential for open-source development of tools for microscopy method refinement using ChatGPT is being explored.
Daniel, your research has sparked intriguing possibilities in the field of microscopy. Thank you for sharing your work.
Thank you, Sophia. I'm glad the research has inspired you. Let's continue exploring the potential of AI in microscopy.
I'm intrigued by the concept of using AI for method refinement in microscopy. Does this approach require a significant amount of labeled training data?
Excellent question, Noah. While a sufficient amount of labeled data is necessary for training ChatGPT, it's possible to leverage existing datasets or even generate synthetic data for certain microscopy techniques to overcome limitations in available labeled data.
I'm curious about the long-term impact of using ChatGPT for microscopy. Daniel, how do you envision this technique evolving in the coming years?
Great question, Oliver. In the coming years, I envision further advancements in AI models like ChatGPT, resulting in more robust and domain-specific refinements for microscopy methods. This could accelerate research, improve data quality, and lead to new discoveries.
As a microscopy enthusiast, this article has piqued my interest. Daniel, what advice would you give to researchers who want to explore using ChatGPT for method refinement?
My advice would be to start by experimenting with smaller-scale projects, ensuring access to good-quality training data, and iterating the refinement process. Collaboration and sharing knowledge with other researchers in the field also play a pivotal role.
Daniel, do you think ChatGPT could be used to refine methods in other imaging techniques apart from confocal microscopy?
Absolutely, Samuel! While this article focuses on confocal microscopy, ChatGPT has the potential to enhance method refinement in various imaging techniques, such as electron microscopy or atomic force microscopy.
I'm impressed by the potential impact of ChatGPT in microscopy. Is this refinement technique accessible to researchers with limited computational resources?
Certainly, Ella. ChatGPT is accessible to researchers with limited computational resources, as it can be run on standard hardware configurations or even cloud-based services, making it reasonably accessible.
This discussion has been eye-opening. Thank you, Daniel, for your expertise and insights.
Thank you, Ella. It's been a pleasure interacting with you and everyone else here.
Daniel, what are some of the biggest breakthroughs you've achieved using ChatGPT for microscopy method refinement?
Some notable breakthroughs include significant improvements in image denoising, automated parameter optimization, and efficient detection of rare cellular events. However, there is still ample room for further innovation.
This article opened my eyes to the potential of AI in microscopy research. Daniel, what are the next steps in your research on method refinement?
Glad to hear that, Sophie. The next steps involve expanding the scope of applications, addressing potential limitations, collaborating with other researchers, and exploring opportunities for integrating ChatGPT into real-world microscopy workflows.
Thank you for sharing your expertise, Daniel. This article has truly broadened my understanding of method refinement in confocal microscopy.
You're welcome, Amy. I'm delighted to hear that it has been informative. Feel free to reach out if you have any further questions or discussions.
I have a follow-up question, Amy. Do you think ChatGPT could be applied to refine other microscopy imaging techniques as well?
Certainly, Thomas. Given the versatility of ChatGPT, it's highly likely that it could be applied to refine various microscopy imaging techniques.
Interesting point, Amy. It's exciting to think about the potential broader impact of ChatGPT across different microscopy imaging techniques.
Absolutely, Oliver. The advancements in AI and machine learning are opening up remarkable possibilities in scientific research.
Indeed, Amy. The future looks promising with AI playing a significant role in accelerating scientific discoveries.
I couldn't agree more, Michael. It's an exciting time to be a researcher.
The combination of AI and microscopy holds tremendous potential for breakthroughs in various scientific fields.
Well said, Sean. Let's continue pushing the boundaries of science and innovation.
Thank you all for your valuable comments and discussions. It's been a pleasure engaging with you on this topic.
Thank you, Daniel, for sharing your expertise and insights on enhancing method refinement in microscopy using ChatGPT.
You're welcome, Jennifer. I appreciate your active participation and interest in the topic.
Thank you, Daniel, for actively participating and addressing our questions. Your research has broadened our understanding.
You're welcome, Jennifer. I'm grateful for the opportunity to participate and provide insights into the exciting field of microscopy method refinement.
Thank you, Daniel, for sharing your valuable expertise throughout this enlightening discussion on microscopy method refinement. Your responses have been highly informative.
You're welcome, Jennifer. I appreciate your acknowledgment of my valuable expertise and the informative nature of my responses. Engaging in this enlightening discussion on microscopy method refinement has been a rewarding experience.
Great article and insightful discussion! Daniel, thank you for sharing your research on enhancing method refinement in microscopy using ChatGPT.
Thank you, John, for your kind words. I'm glad you found the article and discussion insightful.
This discussion is a testament to the potential of AI in scientific research. Thank you, Daniel, for your valuable contributions.
You're welcome, Jane. I'm grateful for the opportunity to contribute to this engaging discussion.
Thank you, Daniel, for your valuable contributions and insightful responses throughout this enlightening discussion on microscopy method refinement. Your expertise has enriched the conversation.
You're welcome, Jane. I appreciate your acknowledgement of my valuable contributions and insightful responses in this enlightening discussion on microscopy method refinement. It's been a pleasure to enrich the conversation with my expertise.
This article opens up a whole new world of possibilities in microscopy. Daniel, thank you for sharing your expertise.
Thank you, Mark. It's truly an exciting time for microscopy research and the integration of AI.
Daniel, your research gives hope for exciting advancements in scientific discovery. Thank you for sharing your knowledge.
You're welcome, Sarah. Science and technology go hand in hand, and it's amazing to witness the innovations that can arise.
Thank you, Daniel, for your expertise and insights. This discussion has been enlightening.
Thank you, Richard. I'm glad you found the discussion enlightening. It's been a pleasure.
An engaging article and a fascinating discussion. Daniel, thank you for sharing your research.
Thank you, Emma. I appreciate your engagement and kind words. This discussion has been enriching.
As an AI enthusiast, this discussion has truly inspired me. Daniel, thank you for broadening our horizons.
You're welcome, Laura. It's always rewarding to inspire and encourage exploration in the field of AI. Thank you for being part of this discussion.
Daniel, thank you for your contribution to the scientific community. This discussion has been thought-provoking.
You're welcome, Sophie. I'm grateful for the opportunity to contribute and engage in this thought-provoking discussion.
Great work, Daniel! This discussion has shed light on the exciting possibilities in method refinement using ChatGPT.
Thank you, Noah. It's been a pleasure discussing and sharing insights on this fascinating topic.
Daniel, your research represents the bridge between AI and microscopy. Thank you for sharing your knowledge.
Thank you, Anna. Bridging these two domains has been a rewarding journey, and I appreciate your kind words.
This discussion highlights the vast potential AI holds for scientific advancements. Daniel, thank you for contributing.
You're welcome, David. The potential of AI in scientific research is truly awe-inspiring. Thank you for being part of this discussion.
Daniel, your research has opened up new horizons in microscopy. Thank you for your valuable insights.
Thank you, Sophia. I'm glad the research has resonated with you. It's been a pleasure sharing insights and exchanging thoughts.
Daniel, your expertise has been invaluable in this discussion. Thank you for sharing your knowledge.
Thank you, John. I'm humbled to contribute to this discussion and share my expertise.
Daniel, your involvement in the discussion has been invaluable. Thank you for generously sharing your expertise.
You're welcome, John. I've thoroughly enjoyed being part of this discussion and exchanging thoughts on microscopy method refinement.
Thank you, Daniel, for actively engaging with the participants and providing insightful responses throughout this enlightening discussion on microscopy method refinement.
You're welcome, John. It has been a pleasure to engage with the participants and provide insights into the fascinating world of microscopy method refinement. Thank you for being part of this enlightening discussion.
Thank you, Daniel, for actively participating in this enlightening discussion and providing valuable insights into microscopy method refinement. Your expertise has been highly valued.
You're welcome, John. I'm grateful for the opportunity to actively participate in this enlightening discussion on microscopy method refinement and provide valuable insights. Your recognition of my expertise is greatly appreciated.
This discussion showcases the potential for AI to revolutionize microscopy. Daniel, thank you for your insights.
Thank you, Jessica. The potential for AI in microscopy is truly exciting. I appreciate your participation and interest.
Daniel, your research in microscopy method refinement is groundbreaking. Thank you for sharing your expertise.
Thank you, Andy. It's always rewarding to push the boundaries of research and witness the impact it can have.
Thank you, Daniel, for sharing your expertise and insights on enhancing microscopy method refinement using ChatGPT.
You're welcome, Oliver. It's been a pleasure discussing and engaging with fellow researchers on this subject.
Daniel, your research and contributions inspire us. Thank you for sharing your insights on microscopy method refinement.
Thank you, Sophia. Your kind words are greatly appreciated. Let's continue pushing the boundaries of scientific exploration.
Excellent article, Daniel! This discussion has been thought-provoking and enlightening.
Thank you, Richard. I'm glad you found the article and discussion thought-provoking. It's been an enriching experience.
Daniel, your perspective on microscopy method refinement using ChatGPT is truly enlightening. Thank you for sharing your knowledge.
You're welcome, Emma. I'm grateful for the opportunity to share my knowledge and contribute to this enlightening discussion.
Thank you, Daniel, for your expertise and insights on enhancing method refinement in microscopy using ChatGPT.
You're welcome, Laura. I appreciate your engagement and interest in this topic. Thank you for being part of this discussion.
Daniel, thank you for your valuable contributions to this discussion on microscopy method refinement.
Thank you, Sophie. Your active participation and engagement have made this discussion even more insightful.
Daniel, your research has highlighted the immense possibilities in microscopy. Thank you for your insights.
Thank you, Noah. It's been a pleasure sharing insights and discussing the immense potential in microscopy research.
Daniel, your expertise in microscopy method refinement using ChatGPT is invaluable. Thank you for sharing your knowledge.
You're welcome, Anna. I'm grateful for the opportunity to share my expertise and contribute to this discussion.
Daniel, thank you for your contributions and insights into enhancing method refinement in microscopy.
Thank you, David. It's been a pleasure engaging in this discussion and sharing insights on enhanced microscopy method refinement.
This discussion has been incredibly enlightening. Thank you, Daniel, for your expertise and insights.
You're welcome, Sophia. It's been a pleasure engaging in this enlightening discussion with fellow researchers.
Thank you, Daniel, for sharing your expertise and insights in this thought-provoking discussion on enhancing method refinement in microscopy.
Thank you, Jessica. I'm glad you found the discussion thought-provoking. It's been a pleasure sharing insights and exchanging ideas.
Daniel, your research has the potential to shape the future of microscopy. Thank you for sharing your knowledge.
Thank you, Andy. The future of microscopy holds tremendous potential, and I appreciate your recognition of that.
Thank you, Daniel, for your expertise and contributions in this captivating discussion on microscopy method refinement.
You're welcome, Oliver. I'm grateful for the opportunity to contribute to this captivating discussion and share my expertise.
Daniel, your research has opened our eyes to the exciting possibilities in microscopy method refinement. Thank you for your insights.
Thank you, Sophie. It's been an enriching experience to share insights and discuss the exciting possibilities in microscopy method refinement.
Excellent article and discussion, Daniel! Your contributions have been invaluable.
Thank you, Richard. I'm delighted to have made valuable contributions to this excellent article and discussion.
Thank you, Daniel, for your prompt and informative responses. Your expertise shines through in this discussion.
Thank you, Richard. I'm delighted that my responses were informative. It's been a pleasure contributing to this discussion.
Thank you, Daniel, for your thoughtful responses and sharing your expertise in this enlightening discussion on microscopy method refinement.
You're welcome, Richard. I'm grateful for the opportunity to respond thoughtfully and share my expertise. This discussion on microscopy method refinement has been truly enlightening.
Daniel, your expertise in microscopy method refinement is impressive. Thank you for sharing your knowledge.
You're welcome, Emma. I'm humbled by your kind words and grateful for the opportunity to share my expertise.
Thank you, Daniel, for your knowledgeable responses and sharing your experiences in refining microscopy methods. Your expertise has added immense value to this discussion.
You're welcome, Emma. It's been a pleasure to provide knowledgeable responses and share my experiences. I'm grateful that my expertise has added immense value to this discussion on refining microscopy methods.
Thank you, Daniel, for your valuable insights and expertise on enhancing method refinement in microscopy.
You're welcome, Laura. I appreciate your acknowledgement and engagement in this discussion on microscopy method refinement.
Daniel, your thoughtful responses and commitment to sharing your expertise have made this discussion on microscopy method refinement highly informative. Thank you for your valuable contributions.
Thank you, Laura. I'm humbled by your recognition of my thoughtful responses and commitment to sharing my expertise. It's been a pleasure to make valuable contributions to this highly informative discussion on microscopy method refinement.
Thank you, Daniel, for your enlightening contributions to this discussion on microscopy method refinement.
You're welcome, Sophie. I'm glad to have contributed to this enlightening discussion on microscopy method refinement.
Thank you, Daniel, for your informative and valuable responses throughout the discussion on microscopy method refinement.
You're welcome, Sophie. I'm grateful for the opportunity to contribute and provide valuable responses in this discussion on microscopy method refinement.
Daniel, your expertise and insights have made this discussion on microscopy method refinement highly valuable. Thank you for sharing your knowledge.
You're welcome, Sophie. Exploring the intricacies of microscopy method refinement and sharing my expertise have been fulfilling experiences. Thank you for your acknowledgement.
Daniel, your expertise and insights have been invaluable in this discussion on method refinement in microscopy.
Thank you, Noah. It's been a pleasure sharing my expertise and contributing to this discussion on method refinement in microscopy.
Thank you, Daniel, for your valuable contributions in this discussion on microscopy method refinement.
You're welcome, Anna. I appreciate your active participation and engagement in this discussion on microscopy method refinement.
Daniel, your contributions in this discussion have been enlightening. Thank you for sharing your expertise.
Thank you, David. I'm grateful for the opportunity to contribute, share my expertise, and participate in this enlightening discussion.
Daniel, your expertise and insights have added immense value to this discussion on microscopy method refinement.
Thank you, Sophia. Your acknowledgement means a lot. It's been a pleasure sharing insights and discussing the intricacies of microscopy method refinement.
Thank you, Daniel, for being actively involved in the discussion and providing valuable insights into microscopy method refinement.
You're welcome, Sophia. Participating in this discussion and sharing insights on microscopy method refinement has been a rewarding experience for me.
Daniel, your contributions have made this discussion on enhancing method refinement in microscopy highly informative.
You're welcome, Jessica. I'm glad to have contributed to this highly informative discussion on enhancing method refinement in microscopy.
Thank you, Daniel, for actively engaging with the participants and providing insightful responses throughout this discussion.
You're welcome, Jessica. I greatly appreciate your recognition, and I'm glad I could contribute to this insightful discussion.
Thank you, Daniel, for actively engaging with the participants and providing insightful responses throughout this discussion.
You're welcome, Jessica. I appreciate your acknowledgement and the opportunity to actively engage in this discussion on microscopy method refinement.
Thank you, Daniel, for your expertise and valuable insights in this discussion on microscopy method refinement.
You're welcome, Andy. I appreciate your acknowledgment and engagement in this insightful discussion on microscopy method refinement.
Daniel, your responses have enriched this discussion. Your expertise and knowledge are highly valued.
Thank you, Andy. I'm grateful for your acknowledgment of my expertise and the value I could bring to this discussion on microscopy method refinement.
Daniel, your expertise and insights have been instrumental in making this discussion on microscopy method refinement highly valuable.
Thank you, Andy. I'm humbled by your recognition and delighted to have made valuable contributions to this discussion on microscopy method refinement.
Daniel, your expertise in microscopy method refinement using ChatGPT is commendable. Thank you for sharing your insights.
Thank you, Oliver. I'm humbled by your kind words and grateful for the opportunity to share my insights in microscopy method refinement.
Thank you, Daniel, for sharing your expertise and actively participating in this enlightening discussion on microscopy method refinement.
You're welcome, Oliver. Participating in this enlightening discussion and sharing expertise on microscopy method refinement has been fulfilling.
Thank you, Daniel, for actively participating and bringing your expertise to this enlightening discussion on microscopy method refinement.
You're welcome, Oliver. It's been an enriching experience to participate and bring my expertise to the forefront of this enlightening discussion.
Thank you all for your thoughtful comments and engaging discussions. I appreciate your interest in my work on enhancing method refinement in microscopy using ChatGPT.