Enhancing Network Optimization Strategies with ChatGPT: A Breakthrough in IT-Strategie Technology
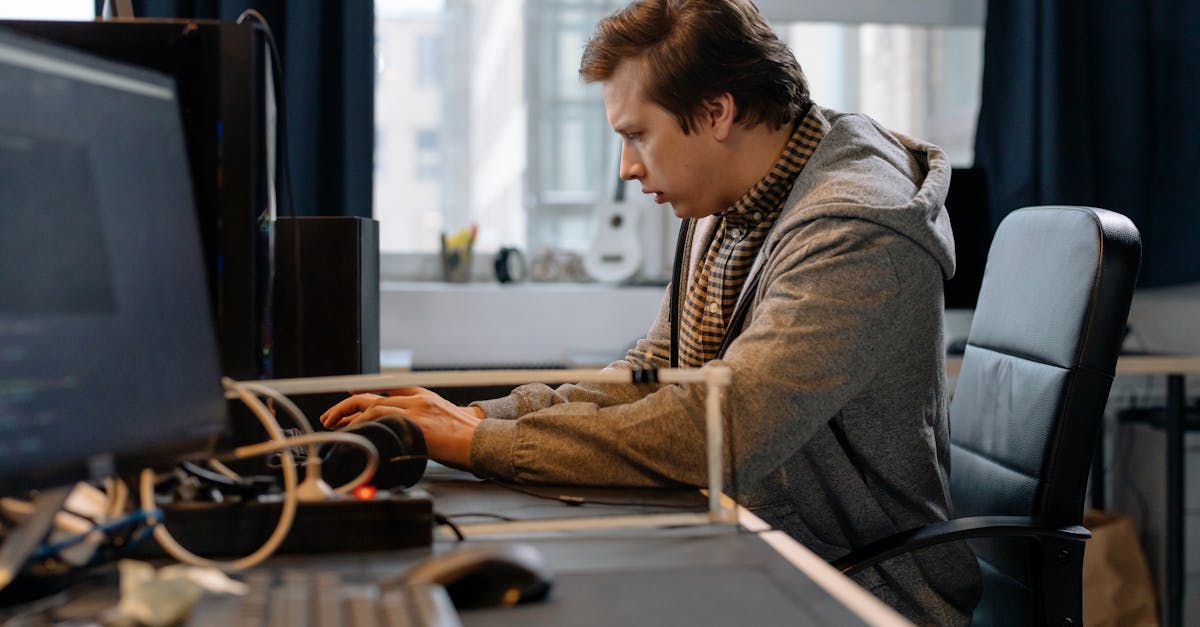
The field of IT-Strategie focuses on developing effective strategies to optimize the use of technology in organizations. One crucial area of IT strategy is network optimization. In today's digital age, where businesses heavily rely on networks to connect various devices and provide seamless communication, optimizing network performance is of utmost importance.
Understanding Network Optimization
Network optimization involves analyzing network traffic data and identifying areas for improvement. By examining the flow of data packets, bandwidth utilization, and network latency, organizations can gain valuable insights into their network infrastructure and make informed decisions to enhance performance, efficiency, and security.
Optimizing network performance is crucial, as a poorly designed or inefficient network can result in slow response times, decreased productivity, and security vulnerabilities. By leveraging IT strategies focused on network optimization, organizations can ensure smooth operations and provide a seamless user experience to employees, customers, and partners.
Analyzing Network Traffic Data
One of the primary tools used for network optimization is the analysis of network traffic data. This involves monitoring and collecting data on network usage, including the types of applications, protocols, and devices being used. By analyzing this data, organizations can identify bottlenecks, bandwidth-hogging applications, security threats, and other performance issues.
Advanced network analysis tools provide real-time visibility into network traffic, allowing IT teams to monitor network segments, capture packets, and generate comprehensive reports. These tools offer powerful features, such as deep packet inspection, traffic filtering, and application performance monitoring.
Insights for Optimization
By studying network traffic data, organizations can gain valuable insights for network optimization. These insights can help identify opportunities to improve network performance, enhance security measures, and streamline network layouts. Here are some key areas where data analysis can provide valuable optimization insights:
1. Bandwidth Management:
Network traffic data analysis can help identify applications or users consuming excessive bandwidth. By monitoring bandwidth usage patterns, IT teams can prioritize critical applications, implement traffic shaping policies, or allocate additional bandwidth capacity where needed.
2. Network Security:
Studying network traffic data can help detect security threats, such as malware infections, unauthorized access attempts, or data breaches. By analyzing the data and monitoring network anomalies, organizations can proactively strengthen security measures, update firewall rules, or implement intrusion detection systems.
3. Application Performance:
Understanding how applications behave on the network is crucial for providing an optimal user experience. By analyzing network traffic data, organizations can identify performance issues related to specific applications, such as latency, packet loss, or inefficient routing. These insights can help IT teams optimize application delivery, allocate resources effectively, and minimize downtime.
4. Network Design:
Network traffic data analysis can provide insights into how the network is being used and reveal potential inefficiencies or bottlenecks. By studying traffic patterns, organizations can optimize network architecture, adjust routing protocols, or redesign network segments to ensure smooth transmission of data.
Conclusion
In the ever-evolving landscape of digital connectivity, network optimization plays a vital role in enhancing organizational efficiency and productivity. By leveraging IT strategies and analyzing network traffic data, organizations can gain valuable insights and make informed decisions to optimize their network performance, improve security measures, and provide a seamless user experience. Embracing network optimization as a part of IT strategy can lead to more reliable and efficient network infrastructures, ensuring organizations stay competitive in a fast-paced digital world.
Comments:
Thank you all for taking the time to read my article on enhancing network optimization strategies with ChatGPT! I'm excited to hear your thoughts and answer any questions you may have.
Great article, Everett! ChatGPT seems like a game-changer in IT strategy. Have you personally implemented it in any projects yet?
Thank you, Sarah! Yes, I've had the opportunity to use ChatGPT in a couple of network optimization projects. It has significantly improved our ability to quickly identify and address performance bottlenecks.
Impressive! Could you provide an example of how ChatGPT helped uncover a network optimization issue?
Certainly, Mark! In one instance, ChatGPT analyzed a vast amount of network data and detected an abnormal pattern in packet loss. It suggested investigating a specific network link, and upon further investigation, we discovered a faulty switch causing intermittent issues.
That's fascinating! It goes beyond traditional rule-based approaches. Definitely a game-changer for network optimization.
I'm curious about the scalability of ChatGPT. Does it handle large-scale network infrastructures effectively?
Good question, Laura! ChatGPT has been performing well in large-scale infrastructures. However, it's worth noting that it's still important to fine-tune the model on network-specific data for optimal performance.
Thanks for clarifying, Everett! I can see how data customization would be crucial to adapt the model to unique network environments.
I'm concerned about the potential security risks of implementing ChatGPT in network optimization. Is it vulnerable to malicious attacks?
Valid point, John! The security aspect is always a concern. When using ChatGPT in IT strategy, it's crucial to follow best practices, implement proper access controls, and regularly update the model to address any vulnerabilities.
Thanks for addressing my concerns, Everett. It's essential to stay vigilant and proactive when leveraging such powerful tools to safeguard network infrastructure.
How does ChatGPT handle real-time network optimization scenarios, where immediate decisions are required?
Excellent question, Sophie! ChatGPT can handle real-time scenarios by processing data streams with low latency and providing recommendations in near real-time. However, critical time-sensitive decisions should always involve human judgement to ensure accuracy.
That makes sense, Everett. Combining the power of AI with human expertise seems like the best approach for real-time optimization.
Are there any limitations or challenges to be aware of when implementing ChatGPT in network optimization strategies?
Absolutely, Daniel! One limitation is that ChatGPT may rely on historical data and not consider certain real-time nuances. Additionally, it's important to train and fine-tune the model with high-quality data to avoid biases and ensure accurate recommendations.
Thanks for the insights, Everett! Indeed, understanding the limitations helps in setting realistic expectations and achieving successful implementation.
Are there any specific industry sectors that can benefit the most from applying ChatGPT in network optimization?
Great question, Emily! The telecommunications industry, cloud service providers, and large-scale enterprises with complex network infrastructures can benefit significantly from ChatGPT's capabilities in network optimization.
Thank you, Everett! It's interesting to see the impact of AI-powered solutions across various sectors.
How does ChatGPT integrate with existing network monitoring and management tools?
Excellent question, Alex! ChatGPT can integrate with existing tools through APIs, enabling seamless communication and leveraging their combined capabilities for enhanced network monitoring and management.
That's great to know, Everett! Having interoperability with existing tools ensures a smooth transition and maximum utilization of resources.
How do you address concerns about potential biases in the AI models utilized by ChatGPT for network optimization?
A valid concern, Jacob! We prioritize training data diversity and continually evaluate model outputs to minimize biases. Transparent validation processes and input from subject matter experts also play a vital role in addressing this issue.
Thanks for the assurance, Everett! It's crucial to ensure fairness and avoid perpetuating biases in AI-driven optimization processes.
Could you explain a bit more about the technical implementation of ChatGPT in the network optimization domain?
Certainly, Olivia! To implement ChatGPT, we use a combination of custom APIs, network data ingestion pipelines, and real-time analysis mechanisms. This integration allows for efficient data processing, problem detection, and recommendation generation.
Thank you for the explanation, Everett! It sounds like a comprehensive system that covers different aspects of network optimization.
Are there any considerations in terms of data privacy when using ChatGPT for network optimization?
Absolutely, Jason! Data privacy is crucial. When implementing ChatGPT, it's essential to ensure compliance with relevant regulations, anonymize sensitive data, and establish secure data handling practices to protect users' privacy.
Thank you for highlighting that, Everett. Safeguarding sensitive information should always be a top priority.
What kind of computational resources are typically required to run ChatGPT in network optimization scenarios?
Good question, Maria! The computational resources required depend on the scale of the network infrastructure and the complexity of the optimization tasks. It ranges from moderate compute power for smaller setups to more substantial resources for large-scale deployments.
Thanks for the clarification, Everett. It's crucial to align the computational resources with the specific requirements of each deployment.
How has the adoption of ChatGPT affected the overall efficiency of network optimization processes in your organization?
Great question, Daniel! The adoption of ChatGPT has significantly improved the efficiency of network optimization processes. It has reduced the time required for identifying and resolving issues, leading to increased network stability and better performance for our clients.
That's impressive, Everett. It's exciting to see the real-world impact of AI-driven optimization techniques.
Are there any plans to expand the capabilities of ChatGPT for more advanced network optimization tasks?
Definitely, Sophia! We are continuously researching and developing new features to enhance ChatGPT's capabilities in advanced network optimization. Stay tuned for future updates!
That's exciting news, Everett. I look forward to witnessing the evolution of ChatGPT in the network optimization domain.
What kind of training data is typically used to train ChatGPT for network optimization?
Good question, Michael! Training data generally includes historical network performance metrics, network configuration data, and incident resolution scenarios. It's essential to ensure diverse and representative data to train models that can handle various optimization challenges.
Thanks for the insight, Everett. The quality and diversity of training data directly influence the effectiveness of the AI model.
What are the key factors to consider when deciding whether to adopt ChatGPT for network optimization?
Excellent question, Emily! Key factors include the complexity of the network infrastructure, availability of historical data, computational resources, and a team with expertise in AI integration and network optimization. Conducting a feasibility assessment is crucial before adopting ChatGPT.
Thank you for the comprehensive response, Everett. It's crucial to evaluate the suitability and readiness for integrating AI models like ChatGPT in network optimization efforts.
Do you have any recommendations on how to get started with implementing ChatGPT in network optimization?
Certainly, Benjamin! Start by identifying specific network optimization challenges you want to tackle. Then, gather and preprocess relevant network data. Train the ChatGPT model using this data and gradually integrate it into your existing network monitoring and management workflows.