Enhancing Network Quality Assurance: Leveraging ChatGPT for Network Design Technology
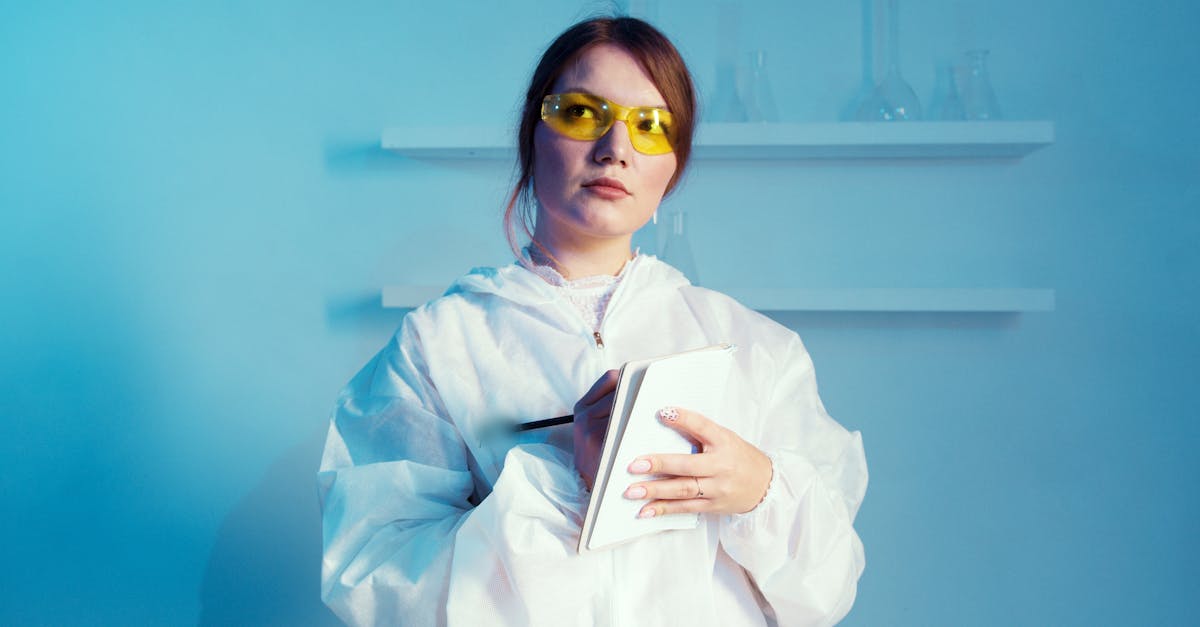
Network design plays a crucial role in ensuring efficient and reliable communication among devices in various industries and organizations. As technology advances, it becomes increasingly important to implement quality assurance methodologies to guarantee the optimal performance and stability of network designs. With the introduction of ChatGPT-4, an advanced chatbot AI, suggestions for quality assurance methodologies have become more accessible and reliable.
Testing Frameworks
One of the primary aspects of network quality assurance is testing. Testing frameworks help evaluate the reliability, scalability, and security of network designs. ChatGPT-4 can suggest different testing methodologies, including:
- Functional Testing: This assesses the network's ability to perform specific tasks according to its intended functionalities.
- Performance Testing: It involves measuring the network's speed, throughput, and response time under various workload conditions.
- Security Testing: This checks the network's vulnerability to potential security breaches and ensures proper implementation of security measures.
- Compatibility Testing: It verifies the network's interoperability with different devices, operating systems, or software.
Benchmarking Techniques
Benchmarking is another crucial aspect of network quality assurance. It involves comparing network designs against industry standards or best practices. ChatGPT-4 can suggest benchmarking techniques to assess the performance and efficiency of network designs. Some common benchmarking techniques include:
- Network Latency Benchmarking: This measures the time delay in data transmission, ensuring it meets predefined expectations.
- Bandwidth Utilization Benchmarking: It tracks the network's bandwidth usage to identify potential bottlenecks and optimize data transmission.
- Reliability Benchmarking: This assesses how consistently the network delivers data without disruptions or failures.
- Scalability Benchmarking: It evaluates the network's ability to handle increasing data loads and growing user demands.
Performance Monitoring Strategies
Continuous monitoring of network performance is essential to identify any potential issues and maintain optimal performance. ChatGPT-4 can suggest effective performance monitoring strategies, including:
- Real-Time Monitoring: This tracks the network's performance metrics in real-time, allowing immediate detection and resolution of any abnormalities.
- Alert Systems: It sets up automated alerts to notify network administrators and engineers about critical performance deviations or failures.
- Log Analysis: This involves analyzing log files to identify patterns, trends, or anomalies that may affect the network's performance.
- Capacity Planning: It helps predict future network utilization and growth, ensuring appropriate resources are allocated to maintain performance.
In conclusion, network design quality assurance methodologies are essential for ensuring reliable and efficient communication among devices. With the assistance of ChatGPT-4, network designers and administrators can rely on suggested testing frameworks, benchmarking techniques, and performance monitoring strategies to optimize their network designs. By implementing these methodologies, organizations can minimize downtime, enhance network performance, and provide seamless connectivity to their users.
Comments:
Thank you all for your comments! I'm glad you found the article interesting. If you have any questions or would like me to elaborate on any specific aspect, feel free to ask.
Great article, Robyn! I found the chatbot aspect fascinating. Do you think it could be integrated with existing network monitoring tools to enhance their capabilities?
I agree, Michael. The idea of leveraging ChatGPT for network design technology is quite innovative. Robyn, could you elaborate on how the chatbot performs network quality assurance?
Michael and Natalie, thank you for your questions. ChatGPT can indeed be integrated with existing network monitoring tools. It can analyze network data, identify potential issues, and provide real-time insights to enhance network quality assurance. The chatbot interacts with network administrators, troubleshoots problems, and offers suggestions based on historical network data and best practices.
Robyn, I'm curious about the limitations of using a chatbot for network design. Are there any potential risks or challenges in relying on AI-powered technology for such critical tasks?
That's a great question, Lisa. While chatbots like ChatGPT can be beneficial, there are indeed challenges to consider. AI-powered chatbots heavily rely on the quality and quantity of training data. If the data is biased or incomplete, the chatbot's suggestions may not always be optimal. Additionally, there might be instances where the chatbot fails to understand complex network issues or misinterprets the context. Therefore, it's crucial to use chatbots as a tool and consider human expertise along with their recommendations.
I wonder how well the chatbot can adapt to specific network environments. Networks can vary greatly, and administrators might have specific requirements. Robyn, have you explored the customization aspects of ChatGPT for network design?
You raise a valid point, Samuel. Customization is important, as different network environments have unique characteristics. ChatGPT can be fine-tuned and trained on specific network data to better understand and provide context-aware suggestions. By incorporating customization, the chatbot can adapt to various network configurations and cater to specific requirements.
I'm curious about the potential impact of ChatGPT on the workload of network administrators. Could it significantly reduce the time and effort spent in network design and troubleshooting?
Amy, great question! ChatGPT can undoubtedly assist network administrators in reducing their workload. By automating certain tasks, it can provide faster responses, offer suggestions, and even perform preliminary troubleshooting. This allows administrators to focus their expertise on more complex issues rather than spending excessive time on routine tasks.
Robyn, do you see any potential ethical concerns with using AI-powered chatbots for network design? For example, could reliance on AI technology potentially lead to job loss or reduced demand for network administrators?
Brian, you bring up an important consideration. While there is always a concern about job displacement when AI technologies are introduced, in the case of chatbots like ChatGPT, their primary goal should be to augment human capabilities rather than replace administrators. Instead of reducing demand, these technologies can help administrators focus on higher-level tasks and complex problem-solving. It's essential to view AI as a tool that complements human expertise rather than a substitute.
I'm intrigued by the potential applications of this technology beyond network design. Can ChatGPT be used in other IT domains?
Absolutely, Emily! While the focus of this article is on network design, the underlying technology of ChatGPT can be applied to various IT domains. From system administration to cybersecurity, chatbots can assist in tasks like diagnostics, threat analysis, and configuration management. The versatility of the approach enables its applicability in numerous areas of IT.
Robyn, I'm interested to know if there are any alternatives to ChatGPT for network design. Are there other AI-based solutions available in the market?
Good question, Oliver. While ChatGPT is one of the prominent AI-based chatbot solutions, there are other alternatives available. Natural language processing (NLP) frameworks like Rasa or Dialogflow can be used to build customized chatbots. However, these alternatives might require more development efforts compared to leveraging pre-trained language models like ChatGPT.
Robyn, I'm curious about the scalability of using AI-powered chatbots. Can ChatGPT handle large-scale network environments?
Jessica, scalability is an essential aspect to consider. While ChatGPT can handle conversations with users effectively, scaling it to accommodate large-scale network environments might require additional optimization and infrastructure considerations. It's crucial to align the chatbot's performance with the network's requirements and allocate appropriate resources accordingly.
Robyn, I appreciate the insights you provided in the article. It's evident that AI-driven chatbots have the potential to revolutionize network design. I'm excited to see how this technology progresses in the coming years!
Thank you, George! I share your excitement. The continuous advancements in AI technologies, combined with human expertise, can contribute to significant improvements in network design and quality assurance.
Robyn, could you elaborate on the challenges of training an AI chatbot like ChatGPT for network design? Was there a need for extensive domain-specific training data?
Jake, excellent question. Training AI chatbots like ChatGPT for network design does require extensive and specific training data. The data needs to cover various network scenarios, configurations, and possible issues. Gathering sufficient domain-specific data and ensuring its quality are crucial steps to train an effective chatbot. Without appropriate data, the chatbot might struggle to provide accurate suggestions or responses.
I'm curious about the user experience aspect. Do network administrators generally find it easy to interact with chatbots like ChatGPT, or do they face any challenges in obtaining the desired assistance?
Sophia, user experience is key when it comes to chatbots. While AI chatbots have come a long way, there can still be challenges in ensuring a seamless interaction. The chatbot's responses should be informative, clear, and easily understandable. Additionally, administrators may face challenges in correctly framing their queries or accurately describing network issues. Continuous user feedback and improvements in the chatbot's natural language understanding are crucial to enhance the user experience.
Robyn, you mentioned leveraging historical network data for network quality assurance. Are there any specific approaches or techniques used by ChatGPT to draw insights from historical data?
Matthew, ChatGPT can leverage techniques like recurrent neural networks (RNNs) to process historical network data. By analyzing past network behavior and performance, the chatbot can identify patterns, detect anomalies, and provide recommendations based on those insights. However, it's important to note that the effectiveness of these approaches depends on the availability and quality of historical data.
I'm intrigued by the potential collaboration between chatbots and human experts. Robyn, in your experience, how important is it to have a seamless interaction between AI chatbots and human administrators?
Ella, seamless interaction between AI chatbots and human administrators is vital for effective collaboration. AI chatbots should assist administrators by providing context-aware suggestions and insights, but they should also be designed to seek clarification when needed. Open channels of communication between AI and human experts ensure that the chatbot's recommendations are aligned with domain-specific expertise and can help in knowledge transfer too.
Robyn, thank you for shedding light on the potential benefits and challenges of using AI chatbots for network design. Are there any notable success stories or real-world implementations of this technology?
Liam, there have been successful implementations of AI chatbots for network design. Various organizations have embraced chatbot technologies to enhance network quality assurance, optimize troubleshooting processes, and improve overall network performance. While specific real-world implementations may vary, these examples demonstrate the practicality and potential impact of AI chatbots in the field of network design.
Robyn, what is the current state of the technology? Is ChatGPT ready for deployment in production network environments, or are there further improvements and research needed?
Aiden, while ChatGPT and similar AI chatbot technologies have made significant progress, there is still ongoing research and improvements happening in the field. The deployment readiness of ChatGPT depends on factors like the specific network requirements, the availability of quality training data, and the desired level of trust in the AI system. Further enhancements in general language understanding, domain-specific training, and addressing potential biases will be beneficial for wider production deployment.
Robyn, how does the integration of a chatbot like ChatGPT affect the overall network security posture? Are there any potential security risks associated with AI-powered chatbots?
Isabella, integration of chatbots like ChatGPT should be done with security considerations in mind. While the chatbot itself can contribute positively to network security by identifying vulnerabilities and providing real-time insights, there are potential risks to address. These include ensuring secure data transmission and access controls for the chatbot, guarding against potential malicious inputs, and constantly monitoring and updating the system to mitigate evolving security threats.
Robyn, are there any known limitations of using AI chatbots for network design that we should be aware of?
Daniel, while AI chatbots like ChatGPT offer valuable assistance, there are limitations to consider. Chatbots heavily rely on the quality and diversity of training data, so biases or data limitations may impact their recommendations. Additionally, they might struggle to understand highly complex or nuanced network issues, and there could be cases where their suggestions may need validation from human experts. It's important to view chatbots as a valuable tool alongside human expertise rather than solely relying on them.
Robyn, how can network administrators gain trust in the recommendations provided by ChatGPT? Are there mechanisms to validate or verify the chatbot's suggestions?
Victoria, trust is crucial when relying on ChatGPT's recommendations. One way to enhance trust is by having a feedback loop where administrators can rate the usefulness and accuracy of the chatbot's suggestions. Additionally, maintaining human oversight and validation of critical decisions can ensure the chatbot's suggestions align with an administrator's expertise. Transparency regarding the system's limitations and ongoing improvements further contributes to building trust in its recommendations.
Robyn, what potential impact could AI chatbots have on the overall speed and efficiency of network troubleshooting?
Ethan, AI chatbots like ChatGPT can substantially impact the speed and efficiency of network troubleshooting. By automating certain tasks, chatbots can provide faster responses, perform preliminary diagnostics, and offer suggested solutions. This enables network administrators to tackle issues more swiftly and efficiently, freeing up time for them to focus on more intricate problems and strategic network planning.
Robyn, do you believe AI chatbots will become an indispensable tool in network design in the near future? Or do you see their usage remaining more supplementary?
Claire, AI chatbots like ChatGPT can become increasingly valuable tools in network design, but I believe their usage will predominantly remain supplementary. The combination of AI-powered chatbots and human expertise can provide superior network design outcomes. While chatbots can automate routine tasks, enhance troubleshooting, and offer recommendations, they should be viewed as augmenting human capabilities and supporting administrators rather than fully replacing their expertise.
Robyn, are there any challenges specific to adopting chatbot technologies in enterprise-level network environments?
Leo, adopting chatbot technologies in enterprise-level network environments can present unique challenges. Ensuring compatibility with existing network infrastructure, managing access controls and security, and meeting scalability requirements are some of the key considerations. Additionally, aligning the chatbot's performance with the organization's network design goals and expectations requires careful planning and coordination.
Robyn, what do you think are the key factors that will drive broader acceptance and adoption of AI chatbots in network design?
Riley, several key factors can drive broader acceptance and adoption of AI chatbots in network design. Demonstrating clear value and tangible benefits, addressing security and privacy concerns, providing transparent explanations of the chatbot's recommendations, and fostering trust through iterative improvements are important. Furthermore, promoting collaboration between AI and human experts, leveraging user feedback, and showcasing successful real-world implementations can contribute to broader acceptance and adoption of AI chatbot technologies.
Robyn, how can organizations ensure the ethical use of AI chatbots in network design to avoid potential biases or unintended consequences?
Luna, ethical use of AI chatbots is crucial. Organizations should invest in diverse and representative training data to mitigate inherent biases. Transparency in the chatbot's decision-making processes, clear disclosure of limitations, and continuously monitoring for potential biases are essential. It's also important to establish guidelines for human oversight, intervention, and accountability. By addressing these aspects, organizations can minimize potential biases and unintended consequences while ensuring fair and ethical use of AI chatbots in network design.
Robyn, how do you envision the future evolution of AI chatbots for network design? Do you expect them to become more autonomous and capable of handling even complex network issues?
Blake, the future evolution of AI chatbots for network design is promising. With advancements in natural language understanding, domain-specific training, and more robust models, chatbots can become more autonomous and handle increasingly complex network issues. However, it's important to remember the value of human expertise and the need for chatbots to collaborate seamlessly with network administrators. Striking the right balance between automation and human interaction will drive their future development and capabilities.