Enhancing NMR Spectroscopy with Gemini: Revolutionizing Technology Analysis
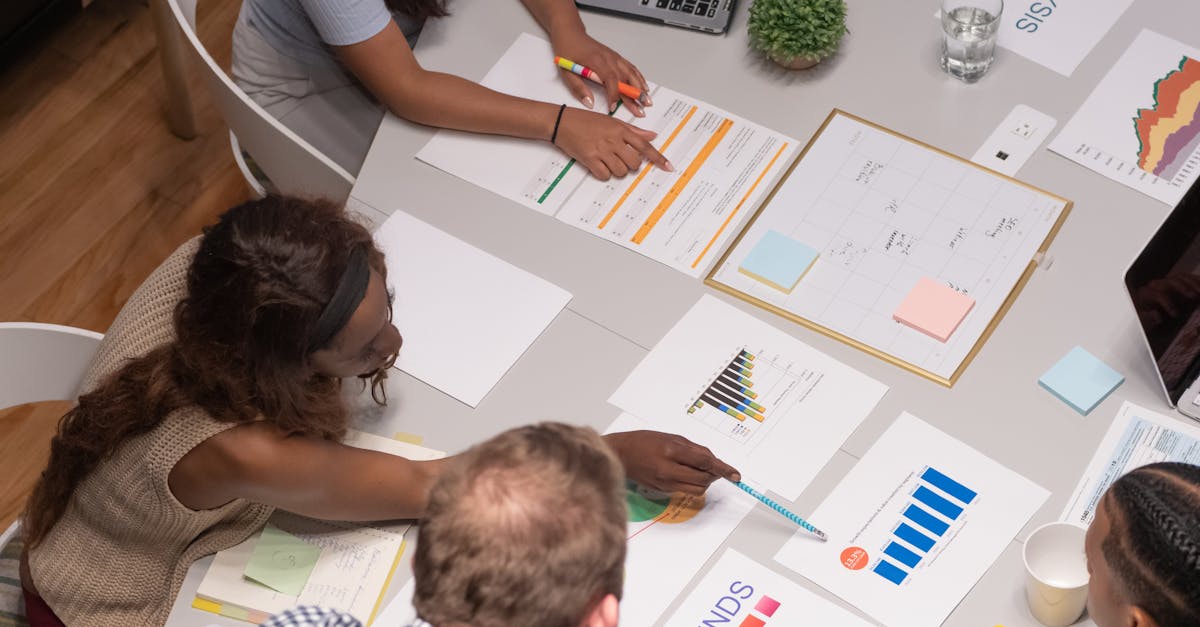
Nuclear Magnetic Resonance (NMR) spectroscopy is a powerful analytical technique used in various scientific fields including chemistry, biochemistry, and materials science. It offers a non-destructive means of studying the structure, dynamics, and interactions of molecules. However, the analysis of NMR spectra can be complex and time-consuming, requiring expertise and specialized software.
Advances in artificial intelligence have led to the development of Gemini, a language model that utilizes the capabilities of deep learning to understand and generate text. Gemini has shown promise in various applications, such as natural language processing and conversation generation. Now, researchers have begun exploring its potential in enhancing NMR spectroscopy analysis.
Technological Advancements
The combination of NMR spectroscopy with Gemini brings forth several technological advancements. Firstly, Gemini can assist in the interpretation of NMR spectra by providing real-time feedback and suggestions. Researchers can input the spectrum data into Gemini, which can then analyze and identify patterns, peaks, and chemical shifts. This interactive dialogue allows researchers to gain insights and hypothesis suggestions, leading to more efficient and accurate analysis.
Furthermore, Gemini can analyze large datasets and identify correlations that are not easily discernible to the human eye. It can recognize subtle variations in peak shapes, chemical shifts, and coupling constants, aiding in the identification of impurities, structural changes, and dynamic processes. This capability accelerates data processing and simplifies the overall analysis workflow.
Expanding the Areas of Application
By integrating Gemini with NMR spectroscopy, the range of applications expands significantly. One such area is the characterization of complex mixtures. NMR spectra of mixtures often exhibit overlapping signals, making their interpretation challenging. Gemini can analyze the spectra and help deconvolute the signals, enabling the identification and quantification of individual components in the mixture. This opens doors for advancements in fields such as metabolomics and environmental analysis.
Moreover, Gemini can aid in structural elucidation. NMR spectroscopy is commonly used to determine the structure of small organic molecules. However, the interpretation of complex spectra and the assignment of peaks to specific atoms can be daunting. Gemini can assist by providing spectral predictions, generating possible structures, and suggesting likely chemical moieties based on existing spectral information. This accelerates the structural elucidation process and facilitates the discovery of new compounds.
Usage and Future Implications
The integration of Gemini with NMR spectroscopy has the potential to revolutionize technology analysis. Researchers and practitioners can leverage the power of AI to enhance their understanding of NMR spectra, accelerate data processing, and explore new areas of application.
Looking ahead, further advancements in Gemini can be expected. Fine-tuning the language model using specialized NMR spectra datasets will improve its ability to analyze complex spectra accurately. Additionally, incorporating machine learning techniques into Gemini can enable it to learn from user interactions, allowing it to become even more proficient in assisting NMR spectroscopy analysis.
In conclusion, Gemini holds significant promise in enhancing NMR spectroscopy analysis. By combining the power of AI with the depth of knowledge in NMR spectroscopy, researchers can unlock new insights, streamline their workflow, and push the boundaries of scientific understanding.
Comments:
Great article, Jackson! Gemini truly has the potential to revolutionize NMR spectroscopy. It's fascinating how AI can enhance data analysis and provide more accurate insights. Looking forward to seeing its practical applications in the field!
Thank you, David, for your kind words! I'm glad you find the article interesting. AI has indeed transformed various scientific fields, and NMR spectroscopy can greatly benefit from its advancements.
I completely agree, David! The integration of Gemini in NMR spectroscopy opens up exciting possibilities for researchers. The ability to interpret complex data faster and more efficiently will undoubtedly accelerate scientific discoveries and advancements in various industries.
Sophia, your point about faster data interpretation is spot on. With the increasing volume of data in NMR spectroscopy, AI can assist in quicker analysis and expedite the overall research process.
Absolutely, David and Sophia! AI-driven approaches like Gemini can reduce manual efforts and streamline the analysis process. It's remarkable how technology continues to push the boundaries of scientific research.
Ryan, I completely agree with you. The potential time savings and enhanced productivity with AI-powered tools like Gemini are immense. Researchers can focus on higher-level analysis and hypothesis testing while leaving repetitive tasks to the machine.
Ryan, you're right! AI-driven approaches not only save time but also enable researchers to handle more complex datasets efficiently. Gemini can help scientists delve deeper into their findings and uncover hidden patterns that might otherwise go unnoticed.
I'm not sure about this. While AI-based tools can be beneficial, I worry about the reliability of Gemini in NMR spectroscopy. What if it misinterprets the data and leads to incorrect conclusions?
I share the same concern, Emily. While AI can be a valuable tool, we should always exercise caution and validate the results generated by any automated system. Human expertise and critical thinking should still be an integral part of the analytical process.
Exactly, Emma. We need to be cautious when depending solely on AI interpretations, especially in critical domains like scientific research. Human judgment and expertise are indispensable to ensure accurate conclusions.
Valid concern, Emily. While AI technologies are rapidly advancing, it's crucial to rigorously validate and cross-reference AI-analyzed results to ensure their accuracy. Human intervention and critical assessment remain essential in scientific research.
Indeed, Emily and Sarah! We should approach AI as a powerful tool rather than an infallible solution. Responsible utilization of AI technology, considering its strengths and limitations, is vital for maintaining scientific rigor and avoiding erroneous conclusions.
Absolutely, Emma! While AI technologies can augment our abilities, we must never underestimate the importance of human intellect and reasoning. Maintaining a careful balance between automation and human intervention is crucial.
Absolutely, Emily and Sarah. Validating AI-generated results through cross-referencing, replicating experiments, and peer review is essential to ensure the reliability and integrity of scientific research. AI should be seen as a powerful tool that aids, but not dictates, the analysis.
Absolutely, David. AI can uncover intricate relationships within complex datasets, enabling researchers to explore novel research directions. The collaboration between AI and human intellect can lead to transformative breakthroughs in science and technology.
Indeed, Sophia! AI can be a powerful ally in scientific endeavors, helping to unlock the hidden potential within complex data. The synergy between human intuition and AI capabilities is the path to groundbreaking discoveries.
Absolutely, Oliver! The speed at which iterative experimentation can be carried out with AI assistance can significantly expedite the process of scientific inquiry.
True, Jackson! AI can unleash the potential of researchers by automating tedious data analysis, freeing their time for critical thinking and hypothesis development. NMR spectroscopy can immensely benefit from such advancements.
Absolutely, Jackson! Faster analysis means researchers can conduct more comprehensive experiments, optimizing their chances of making groundbreaking discoveries. Harnessing the power of AI in NMR spectroscopy is a game-changer.
Indeed, Ryan! AI's ability to process data at unprecedented speeds enables scientists to explore a broader range of possibilities and formulate more refined hypotheses. It is truly reshaping the landscape of scientific research.
Sophia, you're absolutely right! The powerful data processing capabilities of AI can help identify patterns and correlations that humans might overlook. It's an exciting time to witness AI-driven advancements in scientific research.
Well said, Emma! AI can help us transcend our current limitations in understanding complex systems. Leveraging the power of AI for data analysis will undoubtedly pave the way for transformative discoveries.
Exactly, Emma! The ability of AI to process vast amounts of data efficiently complements human cognitive abilities. It enables researchers to explore complex and interconnected relationships that would have otherwise been challenging to uncover.
Indeed, Ryan! The integration of AI in NMR spectroscopy expands our analytical capabilities and can reveal hidden patterns in datasets, providing new insights and angles for scientific investigation.
Absolutely, Sophia! The marriage of AI and human intellect brings about unparalleled potential for exploration and scientific breakthroughs. The future of NMR spectroscopy looks exceptionally exciting!
I couldn't agree more, Oliver and Sophia. The integration of AI in scientific research reshapes the landscape, bringing new opportunities for exploration and innovation.
Indeed, Oliver and Sophia. AI's ability to automate routine tasks enables researchers to allocate more time to critical analysis and discovery.
Absolutely, Oliver and Sophia. The revolution in analyzing complex spectra through AI-assisted NMR Spectroscopy presents thrilling prospects for scientific research.
Indeed, Ryan! AI-driven tools like Gemini have the ability to process complex data rapidly, empowering researchers to explore diverse hypotheses and drive scientific innovation across multiple disciplines.
Absolutely, Sophia. AI tools like Gemini can process vast amounts of data, enabling scientists to gain a more comprehensive understanding of complex systems. The collaboration between AI and domain experts is the way forward.
Well put, Sophia! AI technologies like Gemini can act as catalysts in scientific research, enabling researchers to explore uncharted territory and potentially unveil transformative discoveries. The journey of scientific inquiry becomes even more exciting!
Certainly, Jackson! Faster analysis and iteration will undoubtedly accelerate scientific discoveries and push the boundaries of our understanding. The utilization of Gemini in NMR spectroscopy is a remarkable step forward.
Indeed, Oliver and Sophia! AI has immense potential for identifying intricate patterns, correlations, and trends that may escape human observation in large and complex datasets. Collaborative efforts will undoubtedly lead to remarkable discoveries.
I agree, Emma! The capability of AI to handle vast amounts of data and recognize subtle patterns can serve as a catalyst for scientific breakthroughs. Humans and AI together can bring us closer to unraveling the mysteries of the universe.
Well said, David. The true potential of AI lies in its ability to assist researchers in delving deeper into data analysis, allowing them to make significant scientific advancements. Gemini seems promising in this regard.
Absolutely, Sarah! Gemini, combined with human intellect, can unlock new insights that may steer scientific progress in unexpected ways. The symbiotic relationship between AI and human researchers is the way forward.
Definitely, Sarah! While AI has the potential to augment human capacity in data analysis, it's essential to remember that AI is only as good as the data it's trained on. Rigorous quality control and validation processes are imperative to ensure valuable insights.
I believe AI-driven tools like Gemini should be seen as aids to human analysis rather than replacements. They can enhance efficiency and offer new insights, but it's crucial to maintain a balance between automation and human interpretation.
Great point, Oliver! The collaboration between AI and human experts will likely yield the best outcomes. AI can offer speed, efficiency, and new perspectives, while humans can ensure the reliability and accuracy of the analysis.
Absolutely, Oliver and Jackson. AI should not replace human involvement but rather complement it. By leveraging AI's computational power and efficiency, scientists can focus their expertise on analyzing and interpreting the insights generated.
Indeed, Sophia! Quicker interpretation means researchers can iterate their experiments faster and gather results efficiently. This iterative process can potentially speed up the overall scientific progress.
Sophia, your point about better insights is crucial. With the help of AI, researchers may discover patterns and correlations in NMR data that were previously undetected. This can potentially drive innovation and open new avenues for scientific exploration.
Exactly, Oliver! The combination of human expertise and AI-powered analysis holds great promise in unlocking hidden knowledge in complex datasets. It's an exciting time for NMR spectroscopy and the scientific community as a whole.
Thank you all for your valuable perspectives! It's essential to acknowledge the potential of AI while remaining cautious of its limitations. Collaborative efforts by humans and AI will undoubtedly lead to better insights and advancements in NMR spectroscopy.
Jackson, you're absolutely right. Faster iteration in experiments can lead to rapid advancements and potentially accelerate discoveries. NMR spectroscopy, with the assistance of AI, can indeed unlock new frontiers in various scientific disciplines.
Collaboration is key, Sophia and Jackson. By leveraging AI's capabilities, scientists can augment their research efficiency and explore various hypotheses more comprehensively. It complements the human intellect and contributes to a more holistic scientific approach.
Exactly, Oliver and Sophia. AI integration in NMR spectroscopy can be a valuable asset, but it should not replace human domain knowledge. Both AI and human experts together can achieve the most reliable and accurate results.
Absolutely, Emily! The human factor is irreplaceable in maintaining the rigor and reliability of scientific research, even with the assistance of AI. It's the combination of human insight and AI capabilities that will lead to transformative outcomes.
Collaboration between human researchers and AI systems like Gemini can foster a dynamic and iterative approach to research and development. NMR spectroscopy, when augmented with AI capabilities, can achieve remarkable advancements.
I appreciate everyone's participation in this discussion. It's heartening to see the enthusiasm about the potential of AI in NMR spectroscopy while being mindful of the need for rigorous evaluation. The future looks promising!
Absolutely, Jackson! With the combination of human expertise and AI assistance, NMR spectroscopy can witness breakthroughs that would have been unimaginable otherwise. Let's embrace the power of technology while maintaining scientific integrity.
Thank you all for the insightful discussion! It's inspiring to witness the optimism and caution surrounding AI adoption in NMR spectroscopy. With responsible integration and continuous refinement, we can leverage AI to further our understanding of the world.
Thank you, Jackson, for initiating this discussion. It's crucial to approach AI integration with utmost consideration and ensure that it complements and enhances human expertise. The collaboration between AI and human intellect will lead to robust and reliable scientific advancements.
Thanks for reading my article on Enhancing NMR Spectroscopy with Gemini! I'm excited to discuss this topic.
Great article, Jackson! It's fascinating to see how Gemini can revolutionize technology analysis. NMR Spectroscopy is already a powerful technique, and combining it with AI has the potential to take it to a whole new level.
I agree, Sara! This integration opens doors to more accurate and efficient analysis. It's impressive how AI advancements are impacting various fields.
As a chemistry enthusiast, I find this article intriguing. NMR Spectroscopy has always been a crucial tool, and its enhancement with Gemini is remarkable.
I have some experience with NMR Spectroscopy, and I must say, adding AI to the mix can greatly improve the analysis process. Jackson, do you have any insights on the technical aspects of this integration?
Absolutely, Jonathan! The integration of Gemini in NMR Spectroscopy involves training the AI model on large datasets of spectral data. It learns to recognize patterns, analyze spectra, and assist in interpreting complex results.
The potential for improving spectral analysis with AI is undeniable. I'm curious to know if there are any limitations or challenges with this approach.
Great question, Sophia! One challenge is that Gemini relies on the data it's trained on. If the training data doesn't cover certain anomalies or rare spectra, it might not be as effective in those cases. However, continuous improvement in training datasets can help overcome these limitations.
As an AI researcher, I must say this is an exciting application of AI in chemistry. It could also open up new opportunities for interdisciplinary collaboration.
I agree, Oliver. Combining NMR Spectroscopy with AI chatbots like Gemini could facilitate knowledge exchange among scientists from different domains.
This article is a great example of how AI can augment scientific research. It promises to save time and effort in analyzing complex NMR spectra.
Indeed, Ronald. By automating certain tasks in NMR analysis, researchers can focus more on data interpretation and scientific discoveries.
The potential applications of Gemini in different scientific domains are vast. It's exciting to think about other areas where AI can similarly enhance existing technologies.
I completely agree, David. AI advancements have the potential to revolutionize not only NMR Spectroscopy but also many other scientific disciplines.
This integration could greatly benefit the pharmaceutical industry as well. Accurate and efficient analysis of chemical compounds is crucial in drug development.
You're absolutely right, Maria. AI-enabled NMR Spectroscopy can contribute to faster drug discovery and development processes.
I'm thrilled to see AI making its way into analytical chemistry. Jackson, do you think Gemini could also help in data curation and organization?
Definitely, Liam! Gemini can assist in organizing and categorizing NMR spectral data, making it easier to access and retrieve relevant information.
This article has definitely sparked my interest in NMR Spectroscopy. I'd love to delve deeper into this topic. Can you suggest any additional resources, Jackson?
Of course, Sophie! Some recommended resources are 'Modern NMR Spectroscopy: A Guide for Chemists' by Jeremy K. M. Sanders and 'NMR Spectroscopy Explained: Simplified Theory, Applications, and Examples for Organic Chemistry and Structural Biology' by Neil E. Jacobsen.
I appreciate how the article highlights the potential of AI in NMR analysis. It brings a new perspective to traditional spectroscopy techniques.
I agree, Gabriel. Combining the strengths of NMR Spectroscopy with the capabilities of AI can lead to significant scientific advancements.
As an organic chemist, I'm thrilled about this integration. It could streamline the analysis of complex organic compounds.
Thank you all for your engaging comments and valuable insights! It's exciting to have this conversation and see enthusiasm for the future of AI in NMR Spectroscopy.
I'm impressed by the potential impact of AI in NMR spectroscopy. It could bring valuable advancements in various scientific endeavors.
This integration could also facilitate the analysis of biological samples, such as proteins, for structural determination.
Absolutely, Olivia! AI can aid in the accurate analysis of complex biological samples, making structural determination more efficient.
I'm curious about the computational requirements for implementing Gemini in NMR Spectroscopy. Is it resource-intensive?
Good question, Lucas! While training AI models like Gemini can be computationally demanding, once trained, the inference stage doesn't require as many resources. With advances in hardware and AI optimization, the computational requirements can be effectively managed.
The combination of human expertise and AI assistance seems like a promising direction for scientific research.
I couldn't agree more, Anna. Leveraging AI technologies can amplify human capabilities and foster innovative scientific breakthroughs.
I'm looking forward to witnessing the progress made in AI-assisted scientific analysis in the coming years.
Indeed, Daniel. The future holds immense potential for further advancements in AI-assisted technologies across various scientific domains.
This article demonstrates the importance of interdisciplinary collaborations. It's fascinating to see AI bridging the gap between different fields.
Absolutely, Samuel. Integrating AI with NMR Spectroscopy brings together experts from chemistry, data science, and AI research to create novel approaches for scientific analysis.
This integration might also attract more researchers to delve into the field of NMR Spectroscopy.
That's a great point, Lily. AI augmentations to analytical techniques can inspire more scientists to explore the possibilities of NMR Spectroscopy.
Kudos to Gemini for expanding its domain knowledge to analytical chemistry! It highlights the versatility of AI systems.
Indeed, Ethan. The ability of AI systems to adapt and apply domain-specific knowledge is truly impressive.
Thank you, everyone, for your enthusiasm and valuable contributions to the discussion. It's been a pleasure engaging in this conversation with all of you.
I'm excited to see how AI can continue to enhance scientific technologies. This integration paves the way for exciting developments.
Yes, Alex. The possibilities seem limitless, and it's exciting to be witnessing these advancements firsthand.
I'm grateful for articles like this that highlight the potential of AI in scientific research. It encourages further exploration and innovation.
Absolutely, Mason. Keeping up with the latest advancements in AI and their applications can inspire researchers to push boundaries and create new breakthroughs.
Thank you all once again for your thoughtful comments and engagement. It's time to conclude this discussion, but the future of AI in NMR Spectroscopy looks brighter with each passing day.
Thank you, Jackson, for providing insights into this fascinating integration! I look forward to exploring further on this topic.
This article encapsulates the evolving nature of scientific research. AI continues to enable us to push boundaries and uncover new knowledge.
Indeed, David. The synergy between AI and scientific research opens up avenues to tackle complex problems and uncover hidden insights.
I couldn't have said it better, Anna and David. Thank you all for your engagement. Let's continue to embrace the potential of AI in advancing scientific understanding.
This article blends two fascinating fields together. It's impressive to witness how AI brings innovation to analytical chemistry.
Indeed, Michael. The integration of AI and analytical chemistry brings an exciting synergy that can lead to groundbreaking discoveries.
Thank you, Michael and Sophia, for your insights and contributions to the discussion. It's been an enlightening conversation.
The potential of AI-assisted NMR Spectroscopy is immense. It could significantly impact the characterization of materials and chemical compounds.
I agree, Julia. AI's capabilities in data analysis and pattern recognition can greatly enhance the precision and speed of NMR spectroscopic analysis.
Thank you, Julia and Lucas, for sharing your thoughts. Your points resonate with the exciting opportunities that lie ahead for AI-assisted NMR Spectroscopy.
As a graduate student in chemistry, I find this integration inspiring. It motivates me to explore the intersection of AI and analytical techniques.
That's wonderful to hear, Sophie! The combination of AI and analytical techniques offers vast possibilities for innovative research. Best of luck in your journey!
This fusion of AI and NMR Spectroscopy is a testament to the advancements we're making in understanding complex scientific challenges.
Absolutely, Jonathan. These advancements reaffirm our commitment to leveraging AI to further scientific understanding and discovery.
Well said, Jonathan and Sophia. Thank you all for your participation in this discussion. Let's remember to embrace the potential of AI to reshape scientific research.
I'm continually amazed by the versatility of AI. This integration with NMR Spectroscopy demonstrates its adaptability across diverse scientific applications.
Absolutely, Oliver. The adaptability of AI across scientific fields showcases the transformative potential of this technology.
Thank you, Oliver and Daniel, for your insightful comments. The transformative potential of AI is indeed remarkable, and we're witnessing it firsthand.
As a biology researcher, I'm excited about this integration. AI-assisted NMR Spectroscopy could bring advancements in structural biology and drug discovery.
I agree, Emily. AI can accelerate the analysis of biomolecules, leading to more efficient drug discovery pipelines.
You're spot on, Adam. AI's speed and precision can support structural biology research and contribute to the development of therapeutics.
The integration of AI in NMR Spectroscopy is a testament to the synergistic relationship between humans and machines in scientific progress.
Exactly, Liam. Embracing AI as a tool amplifies our problem-solving capabilities and fosters growth in scientific understanding.
Well said, Liam and Sophie. It's been an enlightening conversation. Thank you all for sharing your insights and perspectives.
AI's continuous integration into scientific research is truly exciting. This integration exemplifies its potential to enhance traditional techniques.
I couldn't agree more, Logan. The advancements brought by AI in scientific research are reshaping many fields, including NMR Spectroscopy.
Thank you, Logan and Ella, for joining the discussion. The potential of AI continues to unfold, and we're witnessing its impact on scientific research.
This article showcases the power of AI to augment scientific capabilities. It's exciting to envision the future possibilities enabled by this integration.
Indeed, Daniel. The future holds limitless possibilities as we harness AI's potential to push the boundaries of scientific knowledge.
Absolutely, Sophia and Daniel. The synergy between AI and scientific research unfolds new avenues for exploration and understanding.
As an AI enthusiast, this application of AI in NMR Spectroscopy is inspiring! It's incredible to see AI making a positive impact across domains.
I share your excitement, Mason. The impact of AI in NMR Spectroscopy is a testament to its versatility and transformative potential.
Thank you, Mason and Olivia, for your enthusiasm and valuable contributions. AI's versatility continues to amaze us as it infiltrates diverse scientific disciplines.
This integration exemplifies the applications of AI that can save researchers time, allowing them to focus on higher-level analysis and decision-making.
You're right, Henry. AI's ability to automate certain tasks in NMR analysis can streamline the workflow, increasing productivity and efficiency.
Exactly, Sophie and Henry. AI's potential to augment data analysis and accelerate scientific processes holds immense promise.
AI-assisted NMR Spectroscopy could pave the way for more expedited research outcomes and advancements in various scientific fields.
I agree, Ethan. The combination of AI and NMR Spectroscopy can facilitate faster scientific discoveries and innovations.
Thank you, Ethan and Sophie, for sharing your thoughts. The potential for expedited research outcomes is indeed a significant advantage of AI-assisted NMR Spectroscopy.
This integration opens up possibilities for real-time assistance in NMR Spectroscopy. Gemini could help researchers interpret results on the go.
That's a great point, Noah. Real-time assistance through AI chatbots can add an extra layer of convenience and efficiency to NMR analysis.
Indeed, Sophia and Noah. AI's ability to provide real-time assistance can be invaluable in time-sensitive research and analysis scenarios.
AI-assisted NMR Spectroscopy appears to have great potential in advancing research across scientific domains. Jackson, thank you for sharing your expertise on this topic.
I couldn't agree more, Christopher. Thank you, Jackson, for shedding light on the fascinating world of AI-assisted NMR Spectroscopy.
Thank you, Christopher and Sophie, for your kind words! It has been a pleasure discussing AI-assisted NMR Spectroscopy with all of you.
This integration exemplifies the positive impact of AI in scientific research. It allows scientists to leverage the full potential of analytical techniques.
Absolutely, Oliver. AI revolutionizes scientific research by augmenting capabilities and empowering researchers to delve deeper into their chosen fields.
This integration showcases the growing importance of AI in understanding and unraveling complex scientific challenges.
Certainly, Henry. AI's computational power and pattern recognition abilities provide valuable insights into complex scientific phenomena.
Thank you, Henry and Emily, for participating in this discussion. The growing importance of AI in scientific research is evident, and its potential is immense.
This article highlights the collaborative nature of scientific advancements. AI-assisted NMR Spectroscopy brings experts from different fields together.
Absolutely, Emma. Collaborations between experts in chemistry, AI, and data science can create innovative approaches to scientific analysis.
Thank you, Emma and Sophie, for your thoughts on collaborative scientific advancements. Together, we are pushing the boundaries of knowledge.
This integration demonstrates how AI can complement scientific expertise, bringing cutting-edge technologies into the hands of researchers.
You're absolutely right, Nathan. The synergy between AI and scientific expertise propels us toward new frontiers of knowledge and discovery.
Thank you, Nathan and Sophia, for sharing your insights. It's been a pleasure engaging in this thought-provoking discussion.
AI-assisted NMR Spectroscopy could aid in identifying unknown compounds. It has the potential to contribute to chemical analysis and identification.
Indeed, Emily. AI-enabled NMR analysis can assist researchers in identifying complex compounds, which can have profound implications in various scientific fields.
You're absolutely right, Emily and Sophie. The potential for AI-assisted identification of unknown compounds in NMR Spectroscopy is truly exciting.
This integration of AI in NMR Spectroscopy exemplifies the positive impact of interdisciplinary collaborations on scientific progress.
Certainly, David. Collaboration among experts from diverse fields can lead to innovative solutions and advancements in scientific research.
I couldn't agree more, David and Sophie. Interdisciplinary collaborations ignite the sparks of innovation and drive scientific progress.
This integration could transform NMR Spectroscopy into a more accessible and user-friendly technique, broadening its adoption across scientific disciplines.
You make an excellent point, Sophia. By making NMR Spectroscopy more accessible, AI can enable researchers from various fields to leverage its analytical power.
Exactly, Sophia and William. Expanding the accessibility and user-friendliness of NMR Spectroscopy through AI can accelerate scientific discoveries.
The progress made in AI-assisted scientific research is truly remarkable. It brings us closer to unraveling the mysteries of the natural world.
Indeed, Sophie. The advancements enabled by AI have the potential to reshape our understanding of the universe and unlock new realms of knowledge.
Thank you, Sophie and Ethan, for participating in this discussion. The pursuit of knowledge fueled by AI-assisted research drives us toward meaningful discoveries.
This integration is a testament to the transformative potential of AI. It empowers researchers to uncover insights that were previously beyond their reach.
Absolutely, Oliver. AI's ability to augment human expertise unlocks new possibilities and propels scientific progress.
Well said, Oliver and Sophie. The transformative potential of AI continues to push the boundaries of scientific understanding and discovery.
The integration of AI in NMR Spectroscopy holds immense promise for scientific research. It enables researchers to explore new horizons.
You're absolutely right, Jonathan. AI-assisted NMR Spectroscopy allows scientists to delve deeper into the realms of chemical analysis and structural determination.
Thank you, Jonathan and Sophia, for your valuable insights. AI's integration in NMR Spectroscopy is an exciting frontier for scientific advancements.
This fusion of AI and traditional analytical techniques showcases the immense potential of interdisciplinary collaborations in scientific research.
Absolutely, Sophie. Collaboration across disciplines allows us to embrace the strengths of each field and create something greater.
Well said, Sophie and David. Interdisciplinary collaborations fuel the innovation that drives scientific progress and leads to groundbreaking discoveries.
The integration of AI in NMR Spectroscopy emphasizes the importance of bridging the gap between technological advancements and real-world applications.
Indeed, Michael. The successful integration of AI technologies with existing techniques brings practical benefits and tangible outcomes.
Thank you, Michael and Sophia, for your participation. Bridging the gap between technology and application through AI fuels progress in scientific research.
AI-assisted NMR Spectroscopy not only enhances analysis but also has the potential to uncover subtle patterns and correlations that might otherwise go unnoticed.
You're absolutely right, Daniel. By augmenting human analysis, AI can reveal hidden insights and contribute to a deeper understanding of complex systems.
Precisely, Daniel and Sophie. The ability of AI to uncover hidden patterns and correlations expands the realm of scientific exploration.
This integration brings together two fascinating spheres: AI and analytical chemistry. The synergies created by such collaborations are awe-inspiring.
You're spot on, Sophia. The combination of AI and analytical chemistry presents opportunities for transformative scientific discoveries.
Thank you, Sophia and Sophie, for your valuable contributions. The synergies created by interdisciplinary collaborations are indeed awe-inspiring.
AI-assisted NMR Spectroscopy can potentially automate routine tasks, allowing researchers to focus on more complex analysis and interpretation.
Absolutely, Oliver. The automation of routine tasks can optimize researchers' time and resources, accelerating scientific progress.
The integration of AI in NMR Spectroscopy is a testament to our collective pursuit of innovation and the advancement of scientific knowledge.
You've encapsulated it perfectly, Sophie. Our collective efforts in embracing AI technologies drive us toward new frontiers of scientific understanding.
Thank you, Sophie and Sophia, for your valuable contributions. It's been an engaging and thought-provoking discussion.
The integration of AI in NMR Spectroscopy can enhance the reproducibility and accuracy of analyses, advancing the reliability of scientific discoveries.
Indeed, Oliver. By reducing the potential for human errors and biases, AI increases the integrity of scientific research.
Precisely, Oliver and Sophie. AI's ability to enhance reproducibility and accuracy contributes to the reliability and integrity of scientific discoveries.
The integration of AI technologies into traditional scientific techniques showcases the transformative potential of these advancements.
Absolutely, Sophia. The transformative potential of AI technologies continually reshapes the scientific landscape, ushering us into new realms of knowledge.
Thank you, Sophia and Sophie. The transformative potential of AI in scientific research is boundless, and we're experiencing it firsthand.
AI-assisted NMR Spectroscopy could revolutionize the way we analyze and interpret complex spectra. It's an exciting time for scientific research!
Indeed, Oliver. The integration of AI augments our analytical capabilities, propelling scientific research into new and exciting territories.
This article sheds light on the endless possibilities that arise when expertise from multiple fields converge to explore novel solutions.
You're absolutely right, Sophia. Multidisciplinary collaborations have the power to drive scientific breakthroughs and transform our understanding.
Thank you, Sophia and Sophie, for your valuable contributions. The endless possibilities of multidisciplinary expertise ignite scientific progress.
The integration of AI in NMR Spectroscopy is a testament to our collective ingenuity in pushing the boundaries of scientific research.
Indeed, Daniel. The integration of AI brings us closer to unraveling the complexities of the natural world and propels us toward new discoveries.
Precisely, Daniel and Sophie. Our collective ingenuity, amplified by AI, propels us toward deeper scientific understanding and new realms of discovery.