Enhancing Online Shopping Fraud Detection with ChatGPT: A Breakthrough in Fraud Investigations Technology
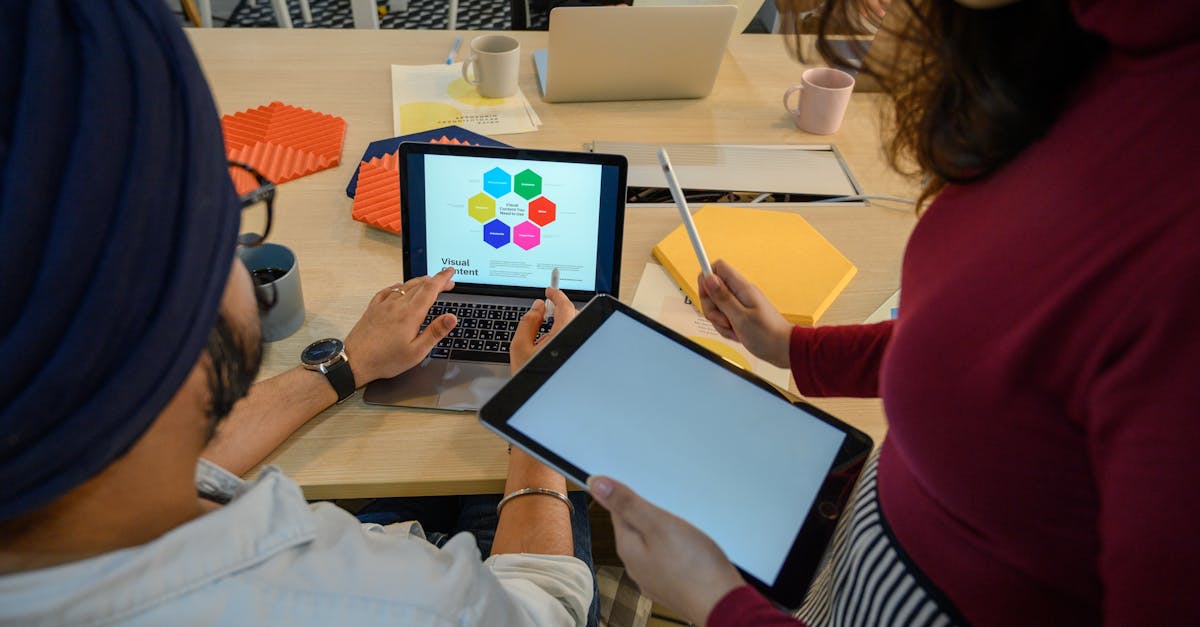
Online shopping has revolutionized the way we buy goods and services. It offers convenience, accessibility, and a wide range of options. However, with the increase in online shopping, the risk of fraud has also grown significantly. Fraud investigations play a crucial role in detecting and preventing fraudulent activities in online transactions.
One of the key technologies used in fraud investigations is the analysis of online shopping data. By utilizing advanced algorithms and machine learning models, fraud investigators can analyze large volumes of transactional data to identify patterns and anomalies that indicate suspicious activities.
Online shopping fraud detection models can analyze various data points, such as transaction timestamps, location information, purchase amounts, and customer behavior. By comparing this data against established patterns of fraudulent transactions, the models can identify potential instances of fraud.
Here are some key features and benefits of using online shopping fraud detection models:
- Automated Fraud Detection: The models can automatically analyze large amounts of data, saving time and resources for fraud investigators.
- Real-time Monitoring: The models can provide real-time alerts when suspicious transactions occur, allowing investigators to take immediate action.
- Improved Accuracy: Machine learning algorithms improve over time, increasing the accuracy of fraud detection as the model learns from new data.
- Reduced False Positives: By analyzing multiple data points, the models can distinguish between legitimate transactions and fraudulent ones, reducing false positives.
Implementing online shopping fraud detection models requires a combination of technology, expertise, and resources. Here are some key steps involved in the implementation:
- Data Collection and Integration: Gathering transactional data from various sources and integrating it into a central database.
- Data Cleaning and Preparation: Removing inconsistencies and errors from the data and preparing it for analysis.
- Model Development: Building machine learning models that can analyze the data and detect patterns of fraudulent behavior.
- Model Training and Validation: Training the models using historical data and validating their accuracy against known instances of fraud.
- Deployment and Monitoring: Implementing the models into the online shopping platform and continuously monitoring their performance.
Online shopping fraud detection is an ongoing process, as fraudsters constantly adapt their techniques to bypass security measures. Therefore, it is essential to frequently update and improve the fraud detection models to stay one step ahead of fraudulent activities.
In conclusion, technology plays a crucial role in fraud investigations, particularly in the area of online shopping fraud detection. By analyzing online shopping data using advanced algorithms and machine learning models, fraud investigators can uncover patterns and anomalies that indicate potential instances of fraud. Implementing these models can automate the detection process, improve accuracy, and reduce false positives, ultimately protecting online shoppers from fraudulent activities.
Comments:
Great article, Kanchan! The integration of ChatGPT in fraud detection seems like a game-changer.
I agree, John. This technology could really help in identifying and preventing online shopping fraud.
I'm excited to see how this technology can outsmart fraudsters and protect consumers.
It's quite impressive to see how AI is being applied to tackle such critical issues.
Kanchan, can you provide more details on how ChatGPT detects fraud?
Sure, Michael! ChatGPT uses natural language processing algorithms to analyze conversations and identify potential fraud patterns.
It's trained on vast amounts of data to understand the context and distinguish between genuine and fraudulent interactions.
By detecting suspicious behavior, it can alert fraud investigators and improve their efficiency.
This technology could be a game-changer in reducing the financial losses caused by online shopping fraud.
I wonder how effective ChatGPT is in detecting sophisticated fraud techniques.
That's a valid concern, David. While ChatGPT is highly advanced, continuous monitoring and updates are essential to address evolving fraud tactics.
I hope this technology is implemented soon. It would provide an extra layer of security and peace of mind while shopping online.
What if fraudsters find ways to manipulate or fool ChatGPT? Are there any safeguards in place?
Good question, Adam! While no system is foolproof, ChatGPT is integrated with other fraud detection mechanisms to minimize manipulation risks.
There are ongoing efforts to strengthen its defenses against adversarial attacks and improve its overall accuracy.
I wonder if implementing ChatGPT would require significant infrastructure changes for businesses.
Lucy, integrating ChatGPT doesn't necessarily require major infrastructure changes. It can be implemented as an additional module in existing fraud detection systems.
How can businesses ensure customer data privacy while using ChatGPT for fraud detection?
Great question, Alex! Privacy is a top priority. ChatGPT operates on anonymized data and doesn't store personally identifiable information.
I'm curious about the scalability of ChatGPT. Can it handle large volumes of transactions?
Absolutely, Paul! ChatGPT is designed to handle high transaction volumes efficiently, making it suitable for diverse online shopping platforms.
How long does it take for ChatGPT to flag a potential fraudulent interaction?
Oliver, the response time varies depending on several factors. However, it's designed to provide near-instant alerts to maximize fraud detection speed.
I think combining AI with human fraud investigators would be the most effective approach. What do you think, Kanchan?
Sophie, you're absolutely right! The integration of AI technologies like ChatGPT complements human investigators, enhancing their capabilities and collective fraud detection accuracy.
Kanchan, do you have any success stories or case studies showcasing the effectiveness of ChatGPT in fraud investigations?
John, there are several ongoing trials and pilots that have shown promising results. Once completed, we'll be able to share concrete success stories.
I'm excited about the potential of ChatGPT in improving the security and trustworthiness of my online shopping experience.
This article highlights the continuous development and innovation occurring in the field of fraud detection.
Thanks for the detailed explanation, Kanchan! It's fascinating to see how AI is being leveraged to combat online shopping fraud.
I hope businesses adopt this technology soon to protect their customers and maintain their reputation.
It will be interesting to see how ChatGPT adapts to new and emerging fraud techniques in the future.
Cybercriminals are always evolving, so constant updates and improvements will be crucial to stay ahead.
I appreciate the emphasis on privacy and data protection while implementing AI-based fraud detection systems.
Businesses must prioritize security measures to build trust with their customers and ensure secure online transactions.
The scalability factor will be pivotal for businesses with large customer bases and high transaction volumes.
Near-instant alerts are crucial to quickly respond to potential fraud attempts and minimize financial losses.
Combining AI with human intelligence can lead to more accurate and efficient fraud detection and prevention.
Kanchan, thank you for sharing your insights on this exciting technological development.
I'm looking forward to seeing the real-world impact of ChatGPT on reducing online shopping fraud.
This innovative integration of AI in fraud investigation is definitely a step in the right direction.
It's reassuring to see advancements like ChatGPT being made to protect consumers and ecommerce platforms.
Fraud detection technologies like ChatGPT can aid in creating a safer and more secure online shopping environment.
I hope businesses prioritize implementing advanced fraud detection systems like ChatGPT to stay ahead in the evolving threat landscape.
Effective fraud detection is crucial for maintaining customer trust and ensuring the growth of the online shopping industry.
The combination of AI and human expertise can create a formidable defense against online shopping fraudsters.
I'm curious to know if ChatGPT can adapt to different languages and cultural contexts.
Matthew, while ChatGPT is primarily trained on English data, efforts are being made to expand language support to accommodate various regions and languages.
The collaboration between technology, businesses, and consumers is essential in combating online shopping fraud.
As technology advances, fraudsters find new ways to exploit vulnerabilities, making continuous innovation in fraud detection imperative.