Enhancing Pharmacovigilance in Medicinal Chemistry: Leveraging ChatGPT for Advanced Safety Monitoring
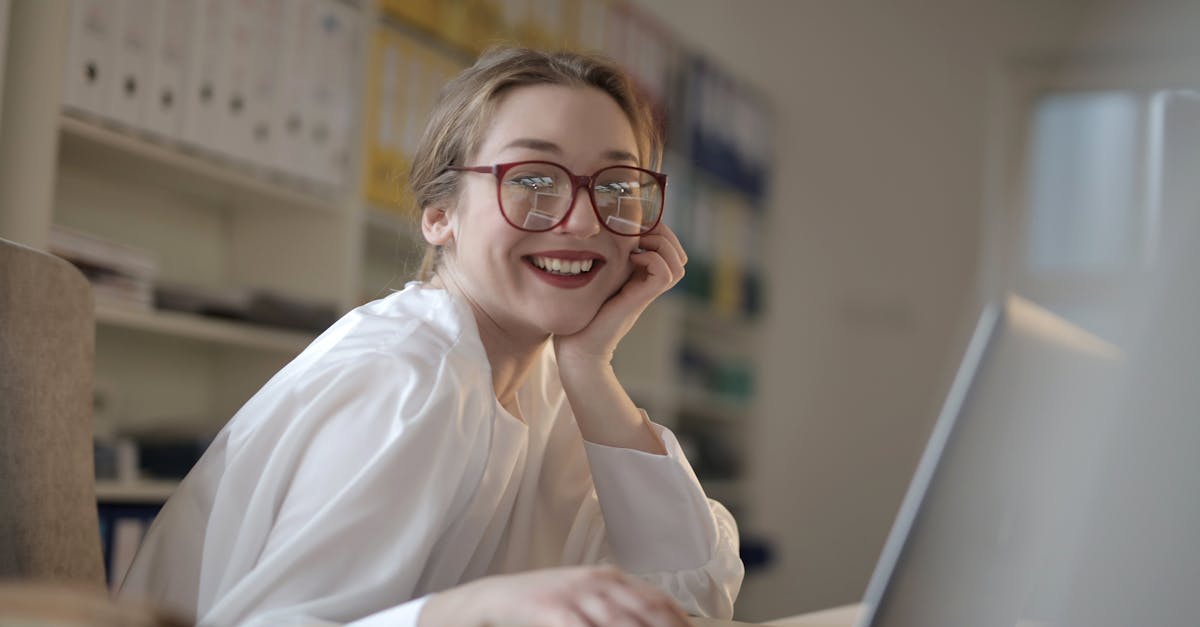
Medicinal chemistry, a multidisciplinary science, plays a crucial role in the development of safe and effective pharmaceutical products. Among its various applications, medicinal chemistry provides valuable insights into pharmacovigilance, a field focused on monitoring and assessing adverse effects of drugs after they have been released to the market.
Pharmacovigilance involves the collection, analysis, and interpretation of data related to the safety and efficacy of pharmaceutical products. It aims to identify, evaluate, and minimize potential risks associated with drug treatments, ensuring the overall well-being of patients.
The Role of Medicinal Chemistry in Pharmacovigilance
Medicinal chemistry utilizes its expertise in drug design, synthesis, and analysis to contribute significantly to pharmacovigilance. By employing various analytical techniques, medicinal chemists play a pivotal role in the detection and characterization of drug-related adverse effects.
Through data analysis, medicinal chemists can identify patterns and trends in reported adverse events or unexpected reactions. They utilize their understanding of the chemical structure and properties of drugs to determine potential causes of adverse effects. This knowledge enables them to provide useful information for regulatory agencies, healthcare professionals, and patients.
Furthermore, medicinal chemists collaborate with other experts in the field, such as toxicologists and pharmacologists, to comprehensively evaluate the safety profiles of pharmaceutical products. By combining their knowledge and skills, these professionals contribute to the identification of early signs of potential adverse effects.
Pharmacovigilance Tools and Techniques
Medicinal chemistry employs a range of tools and techniques to support pharmacovigilance processes:
- Data Mining: Medicinal chemists use computational methods to extract useful information from large databases containing reports of adverse events. By analyzing this data, they can identify hidden associations and potential risk factors.
- Chemoinformatics: This field involves the application of computational methods to analyze chemical data. Medicinal chemists utilize chemoinformatics tools to identify structural alerts or substructures associated with specific adverse effects, helping in the early detection and prediction of potential risks.
- Disease Pathway Analysis: Medicinal chemists analyze the biological pathways affected by drugs to understand their mechanisms of action and potential side effects. This analysis aids in the identification and prediction of adverse effects and supports the development of safer drug alternatives.
- Pharmacokinetic Studies: Medicinal chemists study the absorption, distribution, metabolism, and excretion of drugs in the body to evaluate their safety and predict potential adverse effects. This information assists in optimizing drug dosages and minimizing risks associated with drug interactions.
Benefits of Medicinal Chemistry in Pharmacovigilance
The integration of medicinal chemistry in pharmacovigilance processes offers several benefits:
- Early Detection: By analyzing adverse event data, medicinal chemists can identify potential risks associated with pharmaceutical products at an early stage, allowing for prompt action to minimize harm.
- Improved Safety Profiles: Through the use of predictive tools and understanding of chemical structures, medicinal chemists contribute to the design of safer drugs, reducing the likelihood of adverse effects.
- Enhanced Regulatory Compliance: Medicinal chemistry supports regulatory agencies in evaluating the safety of drugs during clinical trials and post-market surveillance, ensuring compliance with safety standards and regulations.
- Better Patient Outcomes: The incorporation of medicinal chemistry insights in pharmacovigilance processes ultimately leads to improved patient safety, satisfaction, and overall health outcomes.
Conclusion
Medicinal chemistry plays a vital role in the field of pharmacovigilance by leveraging its knowledge and expertise to improve drug safety. Through data analysis, collaborative efforts, and the utilization of various tools and techniques, medicinal chemists contribute to the early detection and characterization of potential adverse effects in pharmaceutical products.
These contributions are instrumental in ensuring the overall well-being of patients and maintaining public trust in the pharmaceutical industry. By embracing the synergy between medicinal chemistry and pharmacovigilance, we can continue to enhance drug safety and improve patient care.
Comments:
Thank you all for reading my blog post on enhancing pharmacovigilance in medicinal chemistry! I'm excited to discuss this topic with you.
Great article, Paul! Pharmacovigilance is crucial for ensuring drug safety. I'm curious, how do you think ChatGPT can contribute to advanced safety monitoring?
Thanks for your question, Maria! ChatGPT can assist in various ways, such as automatic extraction of adverse drug reactions from medical literature, analyzing patient-reported data for potential safety signals, and even predicting the likelihood of adverse events based on chemical structures.
I find the idea of leveraging AI for pharmacovigilance intriguing. However, how can we ensure the accuracy and reliability of ChatGPT's outputs when it comes to drug safety monitoring?
Thomas, that's an important concern. ChatGPT's outputs can be prone to biases and erroneous information. It's crucial to have rigorous verification and validation processes in place to ensure the reliability of its outputs, especially in critical domains like drug safety monitoring.
I completely agree, Lisa. Validation is key for AI applications in pharmacovigilance. The outputs from ChatGPT can act as decision support or alert systems, but human experts should always review and confirm the findings before taking any actions.
Hi Paul! I enjoyed reading your article. Considering the vast amount of data in pharmacovigilance, how can ChatGPT handle the challenge of scalability for large-scale safety monitoring?
Thanks, Sara! ChatGPT can benefit from scalable computing infrastructure and distributed processing to handle large-scale pharmacovigilance data. By efficiently parallelizing computations, it can analyze and monitor safety data from diverse sources, helping in the identification and assessment of potential risks.
This article highlights an exciting use case for AI in pharmacovigilance. Do you have any insights into how ChatGPT can improve signal detection compared to traditional methods?
Great question, Mark. One advantage of ChatGPT is its ability to process unstructured data, such as medical literature, social media posts, and patient forums, where valuable insights can often be hidden. By leveraging language understanding and pattern recognition, ChatGPT can potentially identify safety signals that might be missed by traditional methods, thus improving the overall signal detection process.
While AI can enhance pharmacovigilance, there are also concerns about data privacy and protection. How can we ensure patient data confidentiality when utilizing ChatGPT for safety monitoring?
Emily, that's an important aspect. To ensure patient data confidentiality, anonymization techniques and robust data security measures need to be implemented when using ChatGPT. Additionally, compliance with privacy regulations and obtaining informed consent from patients whose data is used are crucial for maintaining data protection.
Paul, thanks for shedding light on this topic. I'm curious, can ChatGPT also assist in assessing the safety of drug-drug interactions?
Absolutely, Frank! ChatGPT can be utilized to assess potential drug-drug interactions by analyzing relevant scientific literature, clinical trial data, and adverse event reports, providing valuable insights for better understanding and managing the safety profile of combination therapies.
It's fascinating to see the potential of AI in pharmacovigilance. Paul, what are some of the challenges or limitations of leveraging ChatGPT for advanced safety monitoring?
Indeed, Rachel, there are challenges to consider. ChatGPT's performance heavily relies on the quality, relevance, and accuracy of the data it's trained on. Domain-specific knowledge and contextual understanding can sometimes be limited. Continuous improvement with feedback from domain experts and ongoing research is necessary to address these challenges.
This article presents a promising approach, Paul. How do you see the collaboration between AI and human experts evolving in the context of pharmacovigilance?
Thanks, Daniel! Collaboration is key. While AI tools like ChatGPT can automate tasks and aid in decision-making, human experts play a crucial role in validating outputs, interpreting results, and applying their extensive domain knowledge. The collaboration between AI and human experts is likely to evolve towards a symbiotic relationship, enhancing pharmacovigilance capabilities.
Paul, do you think there are regulatory challenges when implementing AI systems like ChatGPT for safety monitoring, and how can those be addressed?
That's a great question, Linda. The regulatory landscape should indeed be considered. Regulatory agencies will need to establish guidelines and frameworks for evaluating and approving AI-based systems in pharmacovigilance. Transparent reporting of methods, validation processes, and adherence to regulatory standards can help address the regulatory challenges.
I'd like to know more about the training process of ChatGPT for pharmacovigilance. Could you provide some insights, Paul?
Certainly, Robert! Training ChatGPT for pharmacovigilance involves a large-scale dataset consisting of medical literature, drug labels, adverse event reports, and other relevant sources. The model learns patterns, relationships, and associations from this data to generate meaningful outputs. It's an iterative process involving fine-tuning and continuous evaluation to ensure performance and accuracy.
AI in pharmacovigilance seems promising, but it may also introduce ethical considerations. How can we address the potential biases and ethical implications associated with ChatGPT's use in safety monitoring?
Sophia, you're right. Bias and ethics are important factors. Addressing biases requires diverse training data and carefully designing evaluation metrics. Ethical implications can be addressed through transparency in AI systems, explainability of results, and continual evaluation based on ethical frameworks, ensuring fairness, accountability, and minimizing unintended consequences.
Well said, Sarah. The development and deployment of AI systems in pharmacovigilance should be done responsibly, considering the potential impact on patient safety and societal well-being. Identifying and addressing biases and ethical implications is vital.
The integration of AI in pharmacovigilance holds promise, but do you think there will be any resistance or skepticism towards relying on AI for decision-making in this field?
That's a valid concern, Jason. Skepticism and resistance towards AI-assisted decision-making can be expected initially. Building trust through rigorous validation, transparency, and involving human experts in the decision-making process can help overcome resistance and gradually establish confidence in AI's capabilities.
It's great to see AI advancements contributing to pharmacovigilance. Paul, how do you think AI can assist in real-time safety monitoring and prompt signal detection?
Excellent question, Carlos! AI can process large volumes of safety data in real-time, allowing for the identification of potential safety signals promptly. By continuously monitoring and analyzing data from diverse sources, including post-marketing surveillance, social media, and patient reports, AI systems like ChatGPT can enhance real-time safety monitoring by detecting emerging risks and trends.
This is an exciting development in pharmacovigilance, Paul. Are there any ongoing research efforts or future directions you're particularly excited about in this domain?
Absolutely, Olivia! Ongoing research aims to improve AI models' interpretability and explainability, ensuring transparency in decision-making processes. Additionally, combining multiple AI techniques like natural language processing, knowledge graphs, and machine learning can enhance the accuracy and reliability of safety monitoring systems. These directions hold great potential.
I appreciate your insights, Paul! How can pharmaceutical companies effectively incorporate AI-based pharmacovigilance tools into their existing systems and workflows?
Thank you, Julia! Integration of AI tools requires a thoughtful approach. Pharmaceutical companies can start by conducting pilot studies to evaluate the feasibility and benefits of AI-based pharmacovigilance tools. Collaborating with AI experts, domain specialists, and ensuring seamless integration into existing systems and workflows can help realize the full potential of AI in improving drug safety monitoring.
Paul, what are some potential limitations of using AI tools like ChatGPT for pharmacovigilance, considering the dynamic and ever-evolving nature of drug safety monitoring?
You raise an important point, Anthony. The evolving nature of drug safety monitoring requires continuous updates and adaptation of AI tools. Limitations may arise due to emerging drug classes, insufficient training data, or changes in safety regulations. Regular model retraining, proactive data collection, and staying up to date with the ever-changing landscape are crucial to mitigate these limitations.
I can see how AI can revolutionize pharmacovigilance. How do you envision the future of AI-driven safety monitoring in the pharmaceutical industry, Paul?
Great question, Grace! AI-driven safety monitoring holds immense potential. In the future, we can expect more sophisticated AI models with improved accuracy and efficiency, seamless integration with existing safety monitoring systems, and increased reliance on real-time data analysis for timely risk identification and mitigation. The collaboration between AI and human experts will remain crucial, leading to safer and more effective medications.
Paul, your article provides valuable insights. Can you elaborate on the role of explainability and interpretability in AI-driven pharmacovigilance?
Certainly, Ethan! Explainability and interpretability are crucial, especially in critical domains like pharmacovigilance. It's essential to be able to understand and interpret the decisions made by AI systems. Explainable AI techniques, such as generating explanations for model outputs, can provide insights into the reasoning behind recommendations, aiding human experts in decision-making and ensuring accountability.
Thanks for sharing your expertise, Paul. What steps do you think should be taken to ensure the responsible and ethical deployment of AI systems in pharmacovigilance?
You're welcome, Sarah. Responsible deployment of AI systems in pharmacovigilance requires a multi-faceted approach. This includes adhering to ethical guidelines, obtaining informed consent from patients, ensuring data privacy, continuous model evaluation, involving human experts throughout the process, and fostering collaborations between regulators, industry, and researchers to establish best practices and regulatory frameworks.
Paul, do you foresee any challenges related to the acceptance and adoption of AI-driven safety monitoring tools among healthcare professionals?
Certainly, David. Widespread acceptance and adoption of AI-driven safety monitoring tools may face challenges initially, such as concerns about reliability, inadequate training on AI systems, or lack of familiarity with the technology. Engaging healthcare professionals in the development process, providing training opportunities, and demonstrating the benefits of AI in practical scenarios can help overcome these challenges and facilitate broader acceptance.
The use of AI in pharmacovigilance holds great potential. How can AI systems like ChatGPT keep up with the rapid growth of medical literature and evolving scientific knowledge?
Good point, Amy. The rapid growth of medical literature is a challenge. AI systems like ChatGPT can leverage natural language processing capabilities to process and analyze vast amounts of literature efficiently. Continuous training, incorporating the latest research findings, and leveraging the expertise of human curators can help keep up with the evolving scientific knowledge and ensure the accuracy and relevance of the outputs.
Hi Paul! AI can assist in various aspects of pharmacovigilance. Are there any specific AI techniques or algorithms that prove particularly beneficial for safety monitoring?
Hi Alan! Indeed, various AI techniques contribute to safety monitoring. Natural language processing techniques help in extracting information from unstructured text, while machine learning algorithms aid in analyzing large datasets and identifying patterns. Additionally, knowledge graphs can help represent complex relationships between drugs, adverse events, and patient characteristics. The combination of these techniques creates a powerful toolbox for pharmacovigilance.
This article provides valuable insights into the future of pharmacovigilance. How do you envision the role of AI in ensuring proactive drug safety rather than just reactive monitoring?
Glad you found it valuable, Mary! AI can play a transformative role in shifting pharmacovigilance towards proactive drug safety. By analyzing emerging safety signals in real-time, integrating patient data, and leveraging predictive models, AI can identify potential risks early on, leading to proactive measures such as targeted post-marketing studies, label updates, or even drug withdrawals if necessary. The goal is to prevent harm and improve patient safety proactively.
Thank you all for your engaging comments and questions! I truly appreciate the discussion around AI-driven safety monitoring in pharmacovigilance. Let's continue our efforts to ensure the safe and effective use of medications.
I completely agree, Paul. Explainability and addressing biases are crucial to ensure the ethical and fair deployment of AI systems in pharmacovigilance.
Transparency and ongoing evaluation can help in maintaining ethical AI practices in the field of pharmacovigilance.
The trustworthiness of AI systems in pharmacovigilance heavily depends on addressing biases, ensuring fairness, and ethical decision-making.
Establishing trust in AI-assisted decision-making will be crucial to gaining acceptance and adoption in pharmacovigilance.
Human experts' involvement in decision-making can help alleviate skepticism and build confidence in AI's contribution to pharmacovigilance.
Efficient real-time safety monitoring can revolutionize pharmacovigilance, aiding timely risk detection and mitigation.
Improving interpretability and combining various AI techniques hold promise for advancing safety monitoring in pharmacovigilance.
The collaboration between AI and human expertise will be a key driver in the evolution of safety monitoring capabilities in pharmacovigilance.
Keeping AI models up to date with the rapid changes in drug safety regulations and emerging drug classes is crucial for their effectiveness.
The future of AI-driven safety monitoring in pharmacovigilance looks promising, with improved models, real-time analysis, and increased collaboration between AI and human experts.
Seamless integration of AI-driven safety monitoring systems into existing workflows will be vital for the widespread adoption and effectiveness of pharmacovigilance tools.
Absolutely, Grace! Integrating AI-driven safety monitoring seamlessly into existing workflows will maximize the benefits and improve patient outcomes.
Addressing healthcare professionals' concerns, providing training opportunities, and demonstrating the benefits of AI can help foster acceptance and adoption in pharmacovigilance.
Efficient natural language processing and continuous model updates are key to keeping AI systems up to date with the growing medical literature for accurate safety monitoring.
The combination of natural language processing, machine learning, and knowledge graphs unleashes the full potential of AI in pharmacovigilance.
Indeed, Alan! The combination of AI techniques can unlock powerful insights to enhance safety monitoring capabilities in pharmacovigilance.
AI's role in proactive drug safety will revolutionize pharmacovigilance by preventing harm and ensuring patient safety.
Precisely, Mary! The proactive role of AI in identifying and mitigating risks early on holds immense potential for improving patient safety in pharmacovigilance.
Thank you all for the insightful discussion and exploration of AI in pharmacovigilance. It has been a pleasure engaging with each one of you!
Signing off now, but feel free to continue the conversation. Take care, everyone!