Enhancing Polymer Characterization: Leveraging ChatGPT in Flammability Testing
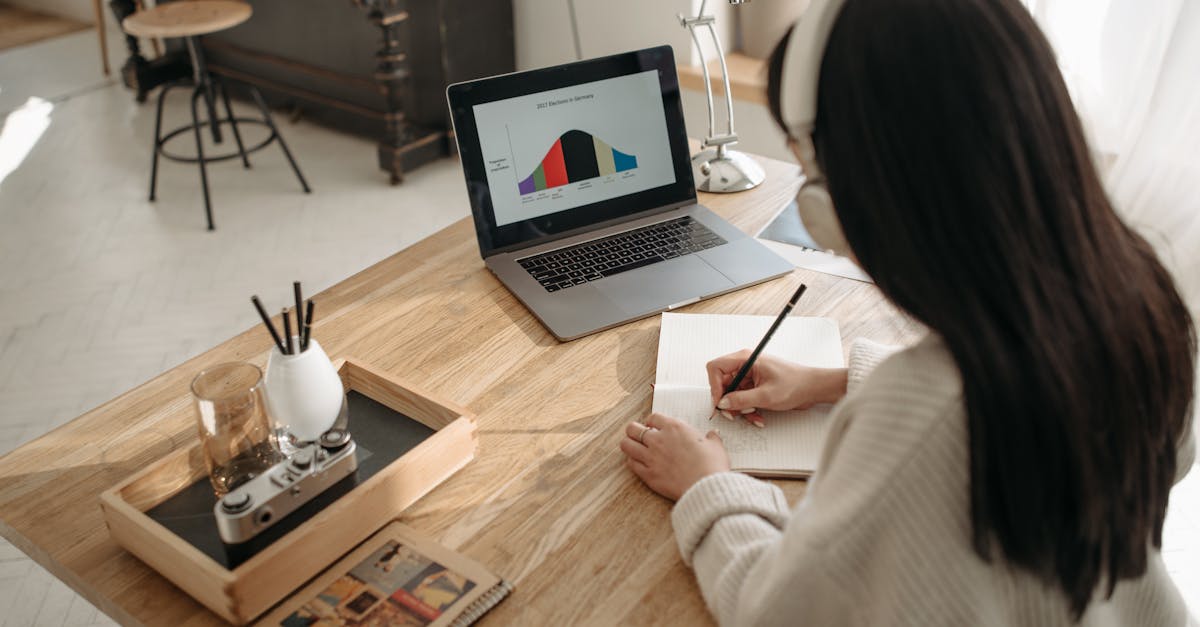
Flammability testing is a critical aspect of ensuring the safety and reliability of polymer-based materials. With the advancement of technology, the field of polymer characterization has seen significant developments in accurately predicting and interpreting the flammability characteristics of different polymers.
One of the emerging technologies in this field is the use of Chatgpt-4, an advanced AI model, to assist in the prediction and interpretation of flammability test results. Leveraging its natural language processing capabilities, Chatgpt-4 can analyze the test data and provide valuable insights into the flammability behavior of polymers.
Flammability testing involves subjecting polymers to specific conditions, such as exposure to a flame or controlled ignition in a laboratory setting. These tests aim to evaluate the ignition, flame spread, and self-extinguishing properties of different polymer materials.
By using Chatgpt-4, researchers and engineers can input the test parameters, including the polymer type, sample dimensions, heat source characteristics, and environmental conditions. The AI model then analyzes this data and predicts the flammability characteristics of the polymer material under investigation.
Moreover, Chatgpt-4 can assist in the interpretation of flammability test results. It can provide detailed explanations and insights into why certain polymers exhibit specific flammability behaviors. This information is crucial for researchers and engineers to understand the underlying mechanisms and develop strategies for improving the fire resistance of polymer materials.
The usage of Chatgpt-4 in polymer characterization offers several benefits. Firstly, it reduces the need for extensive and time-consuming experimental testing. Researchers can use the AI model to quickly assess the flammability characteristics of different polymers, saving both time and resources.
Secondly, Chatgpt-4 can help researchers identify potential risks associated with the use of specific polymer materials in different applications. It enables them to make informed decisions regarding the suitability of polymers in specific environments or industries, such as construction, automotive, or electronics.
Furthermore, the AI model can aid in the development of fire safety regulations and standards for polymer-based materials. By analyzing a vast amount of flammability test data, Chatgpt-4 can contribute to the establishment of guidelines that ensure the safe use of polymers in various industries.
In conclusion, the integration of Chatgpt-4 in polymer characterization brings significant advancements in predicting and interpreting the flammability characteristics of different polymers. By leveraging AI technology, researchers and engineers can efficiently analyze and understand the behavior of polymer materials under fire conditions. This paves the way for safer and more reliable applications of polymers in various industries, ultimately enhancing fire safety standards.
Comments:
Thank you all for taking the time to read and comment on my article about leveraging ChatGPT in flammability testing. I'm excited to hear your thoughts and answer any questions you may have!
Great article, Jesse! I found it really insightful. Leveraging AI in polymer characterization can undoubtedly improve efficiency and accuracy. Are there any limitations or challenges in implementing ChatGPT in this field?
Thank you, Sara! While ChatGPT can be a powerful tool, there are indeed limitations. One challenge is ensuring the training data covers a wide range of polymers, as the model's effectiveness can vary across different types. Additionally, certain aspects like plasticizers, fillers, or additives may impact accuracy. Evaluation of the model's performance on various polymer types becomes crucial.
Hey Sara, I completely agree with you. Implementing AI in polymer characterization has tremendous potential. However, we must ensure that the trained models receive continuous updates to keep up with advancements in materials science. Otherwise, the knowledge might become outdated. What are your thoughts on this?
Liam, you make an excellent point. Continuous updates to the models are crucial to keep up with evolving materials science knowledge. Collaboration between AI researchers, polymer scientists, and experts from related fields can ensure that the models incorporate the latest advancements and improve their accuracy and usefulness. It's a dynamic field, and staying at the forefront of research is vital in maintaining the value of AI in polymer characterization.
Liam, I completely agree. Continuous updates to the trained models are vital in ensuring their relevance and accuracy. Collaboration between researchers, scientists, and industry experts can help identify the latest trends, discoveries, and advancements. This way, AI can continually adapt to the evolving field of materials science and ensure that the predictions remain reliable and valuable.
Sara, I'm glad you share the same view! Collaboration and knowledge exchange between different fields are essential for the success of AI models in polymer characterization. By establishing strong connections, we can marry the expertise of materials scientists, polymer experts, and AI researchers to push the boundaries of what can be achieved. It's exciting to see how these synergies can shape the future of flammability testing.
Sara, you hit the nail on the head! Collaboration is key to ensure AI models don't become outdated. Regular feedback from field experts and researchers can help identify necessary updates, account for new discoveries, and address any limitations. By fostering an environment of continuous improvement and knowledge exchange, we can maximize the value and practicality of AI in polymer characterization.
Hi Jesse, thanks for sharing your expertise. I have a question regarding the transferability of the trained model. Can the ChatGPT model trained on one type of polymer be effectively used for assessing the flammability of different polymers?
Hi Steven! The transferability of the trained model is an excellent point. While it's possible to use a model trained on one type of polymer for related types, it may not perform as well. Optimizing the model for specific polymer properties can yield better results. However, fine-tuning and retraining on different polymers can be time-consuming and computationally intensive.
Hi Steven, that's a great question! While some properties of polymers may be transferable across different types, flammability can vary significantly. It's important to consider the unique chemical composition and structural characteristics of each polymer. Training the model on a diverse range of polymer types would improve its generalization capabilities, but there might still be limitations when applied to completely different materials.
Kristen, thank you for your response. I agree that the chemical composition and structure of each polymer play a significant role in flammability. It's clear that the model's effectiveness will vary for different materials. Considering the limitations, it's important to validate the specific model's performance on the target polymer before relying solely on AI predictions. Incorporating domain knowledge alongside AI can help achieve more reliable results.
Steven, you're welcome! Validating the model's performance on the target polymer is critical to assess its reliability. While there may be shared characteristics across different polymers, the behavior during combustion can vary significantly. By comparing AI predictions with experimental results and consulting domain experts, we can gauge the model's accuracy and make informed decisions accordingly.
Kristen, I appreciate your response. Validating the model's performance on the target polymer becomes crucial, especially for safety-critical applications. Relying solely on AI predictions without considering domain knowledge can lead to undesirable outcomes. By combining the strengths of AI and human expertise, we can achieve more reliable and accurate flammability assessments in various polymer scenarios.
Jesse, your article was a great read! I'm curious to know if ChatGPT can purely rely on text-based inputs or if visual data can also be incorporated to enhance the flammability testing process?
Thanks, Emily! ChatGPT primarily relies on text-based inputs. However, incorporating visual data, such as images or videos, into the flammability testing process can provide valuable context. It could enable the model to learn from visual cues, helping it understand polymer behavior during combustion better. This integration presents an exciting avenue for future research.
Hey Emily, glad you enjoyed the article! While ChatGPT primarily relies on text data, incorporating visual data could greatly enhance the accuracy and reliability of flammability testing. Images or videos could provide additional information about the polymer's behavior during combustion, complementing the textual description. Hybrid models combining text and visual data might deliver better performance. What do you think?
Oliver, I completely agree with you. The combination of text and visual data can provide a more comprehensive understanding of the flammability behavior. Hybrid models can integrate both modalities to capture and leverage the intrinsic knowledge present in both forms of data. This would pave the way for a more robust and accurate testing process. It's an exciting direction and could lead to significant improvements in polymer characterization.
Emily, absolutely! Incorporating visual data can provide valuable additional insights. By capturing the visual aspects of polymer combustion, we can enhance the model's ability to detect patterns and understand complex correlations. Hybrid models that leverage both visual and text data hold great promise in improving flammability testing accuracy and broadening our understanding of polymer behaviors.
Oliver, I completely agree with you. Combining different modalities of data, such as visual and textual, can give us a more comprehensive understanding of the flammability behavior of polymers. We can capture the nuances that may not be entirely described by text alone. Hybrid models hold great potential in improving the accuracy and reliability of flammability testing.
Sophia, I'm glad you share the same perspective. Combining visual data with text reinforces the overall understanding of polymer flammability. It's an exciting development that can enhance the accuracy of predictions and potentially uncover new correlations that may be elusive when relying solely on textual inputs. It opens doors for novel applications and advancements in the field.
Oliver, I completely agree! By incorporating visual and textual data, we can create a more holistic testing approach. Visual cues can provide supplementary information that might be challenging to convey through text alone. Hybrid models considering both modalities can facilitate more accurate predictions and a deeper understanding of the complexities involved in flammability testing.
Jesse, really interesting article! I'm wondering if there are any risks associated with relying heavily on AI-based models for flammability testing. Are there any potential safety concerns or limitations we should consider?
Thanks, Carlos! While AI-based models can significantly enhance flammability testing, there are certain risks. One important consideration is the model's generalization capabilities. It's crucial to test and validate the model's performance against real-world scenarios and ensure it can handle variations in polymer composition and conditions. Furthermore, human oversight should always be in place to prevent any potential safety concerns.
Carlos, I believe it's essential to approach AI-based models for flammability testing with caution. One possible risk is the potential for biases in the training data, leading to inaccurate results or safety hazards. Ensuring diverse and representative training datasets can help mitigate this concern. It's also critical to have human experts involved in the development and validation stages to prevent any unforeseen risks.
Anne, thank you for sharing your insights. Biases in training data can indeed introduce risks and compromise the reliability of AI models. Diverse and representative datasets are crucial, and efforts should be made to detect and mitigate any biases during the training process. Human involvement, especially from experts in the field, can aid in reducing safety concerns and ensuring the models are reliable tools rather than potential hazards.
Anne, I completely agree with you. The involvement of experts from the industry and academia in the training process is crucial. Their insights and domain expertise can ensure that the AI models are reliable and realistic. Collaborative efforts allow us to harness the power of AI while mitigating potential risks, thereby bringing us closer to harnessing the true potential of AI in polymer characterization.
Anne, I completely agree. Combining the expertise of industry professionals, academics, and AI researchers fosters a more holistic approach to tackling the challenges associated with flammability testing. Through collaboration, we can harness the strengths of each field and build optimized, reliable AI models that align with the objectives and safety requirements of the industry.
Carlos, I completely agree with you! A multidisciplinary approach expands the perspective and capabilities, ensuring the AI models align with the industry requirements and safety standards. Collaboration allows us to combine theoretical knowledge, practical expertise, and cutting-edge AI techniques to address the challenges posed by flammability testing. It's an exciting time to witness the positive impacts of collaboration in this field.
Anne, collaboration enables us to tackle challenges collectively and pool diversified expertise toward a common goal. By integrating AI models into the industry while considering the insights provided by experts, we can ensure safer and more efficient flammability testing. It's an exciting journey to witness the transformative potential and overcome challenges by embracing collaboration.
Carlos, I couldn't agree more. Joint efforts allow us to blend the advancements in AI with practical domain knowledge, ensuring that the resulting solutions meet the requirements of the industry. Collaborative approaches pave the way for reliable, efficient, and safe flammability testing, ultimately benefiting various sectors dependent on polymer characterization. It's a fascinating journey that lies ahead!
Anne, you've summarized it beautifully! The collaboration between AI and human experts holds tremendous potential. By integrating the best of both worlds, we can develop safe and reliable AI models that can aid in accurate flammability assessments. The combined strength of AI techniques and human expertise is a powerful force for progress in polymer characterization.
Hi Jesse! Loved your article. In terms of deploying ChatGPT for flammability testing, what would be the ideal size of the training dataset? Are there any minimum requirements for achieving good results?
Hi Hannah! The ideal training dataset size can vary depending on several factors, including the complexity of the problem and the desired performance. In general, larger datasets tend to yield better results. However, having a minimum amount of training data is essential to avoid overfitting and improving the model's ability to generalize. The specific requirements may depend on the specifics of the polymer and the testing objectives.
Hi Jesse, great article! Considering the importance of training data, how do you ensure the training data adequately represents the variations in flammability properties within a specific polymer type?
Nina, that's a great question! When ensuring the training data adequately represents variations in flammability properties within a specific polymer type, it's essential to collect samples from a wide range of sources and ensure diversity in the dataset. This includes different manufacturers, processing techniques, environmental conditions, and other relevant factors. It's crucial to capture the full spectrum of variations inherent to the polymer type under consideration.
Jesse, thank you for your response! Incorporating diversity in the training data seems like a robust approach to capture variations. It's important to ensure that the AI model learns to generalize well within a specific polymer type. Appreciate the insight!
Nina, I completely agree with Jesse. Diversity in the training data not only helps the model capture variations but also ensures it can handle real-world scenarios effectively. Incorporating diverse samples can expose the model to a wide range of factors and variations, allowing it to make more accurate predictions in practice. By covering the full spectrum of possibilities, we enhance the overall reliability of the model's predictions.
Jesse, great article! I was wondering how the incorporation of AI in flammability testing affects the overall timeline for conducting tests and obtaining results. Does it significantly reduce the time required or introduce any bottlenecks?
Kieran, I'm glad you asked! The incorporation of AI in flammability testing can potentially reduce the time required to obtain results. By leveraging AI models, predictions can be made based on available data without the need for lengthy physical testing in all cases. However, it's important to note that AI models still require comprehensive training and validation, which can take time initially. So, while it can speed up the process in the long run, it may introduce some bottlenecks initially.
Nina, you're welcome! Ensuring variations are adequately represented in the training data is crucial for models to generalize effectively. Conveying the diversity of a polymer type helps the model learn and make accurate predictions across a broader range of scenarios. By capturing the essence of variations, we equip the model to provide reliable insights and predictions for a given polymer.
Nina, thank you for the clarification. It's great to see that AI can potentially reduce the overall testing time. Though initial investment in time for training and validation is required, the long-term benefits of faster predictions and testing outweigh the initial bottlenecks. AI has the potential to revolutionize the field of flammability testing and lead to significant time and cost savings.
Kieran, I'm glad it clarified the timeline aspect for you! Indeed, the initial investment of time for training and validation is necessary to ensure the AI model's accuracy and reliability. Once the model is established and fine-tuned, it can provide significant time savings in later stages. The potential time reduction and improved efficiency are indeed valuable benefits, making AI a promising tool for flammability testing.
Nina, I appreciate your response. The time-saving potential of AI is impressive, and it's great to see that the initial investment offers long-term benefits. Advancements like these fuel the progress in materials science and bring us a step closer to more efficient and reliable processes. The future looks promising for flammability testing!
Nina, you're welcome! Capturing variations within a specific polymer type is crucial for accurate predictions. By including a diverse range of samples and scenarios in the training data, the model can learn to navigate the complexities and provide reliable insights. I'm glad you found the information helpful!
Hi Hannah! The size of the training dataset is indeed an important consideration. To achieve good results with ChatGPT, a sufficient amount of data is required to capture the variability and nuances in flammability testing. While there is no fixed minimum requirement, having a few thousand labeled instances would generally be a good starting point. A larger dataset can further enhance the model's performance, but it should strike a balance considering resource constraints.
David, thank you for your response! Having a few thousand labeled instances as a starting point makes sense. It's important to find a balance between quality and quantity in the training dataset. Resource constraints are definitely a factor to consider, and ensuring that the data covers a diverse range of scenarios can help the model generalize better. Thanks for clarifying!
Hannah, you're welcome! Striking the right balance between data quantity and quality is indeed crucial. It's also worth noting that iterative training and fine-tuning can further improve the model's performance. As the dataset evolves, incorporating feedback, new data, and addressing limitations can refine the model's ability to accurately predict flammability outcomes. It's an ongoing process of incrementally enhancing the model's effectiveness.
Combining visual and textual data truly gives us the best of both worlds. Textual information can provide detailed descriptions of properties and behaviors, while visual data allows us to observe and analyze the physical aspects. By leveraging both modalities, we can create a more comprehensive and robust understanding of polymer flammability, which can lead to better predictions and overall safety.
Combining the strengths of AI models and human expertise is essential for accurate assessments. Human experts bring domain knowledge and real-world experience to the table, enabling them to identify subtle indicators and nuances that AI models may overlook. The collaboration between AI and human experts can enhance the overall reliability and safety of flammability testing in polymer characterization.