Enhancing Predictive Analytics for Fraud Investigations: Harnessing ChatGPT Technology
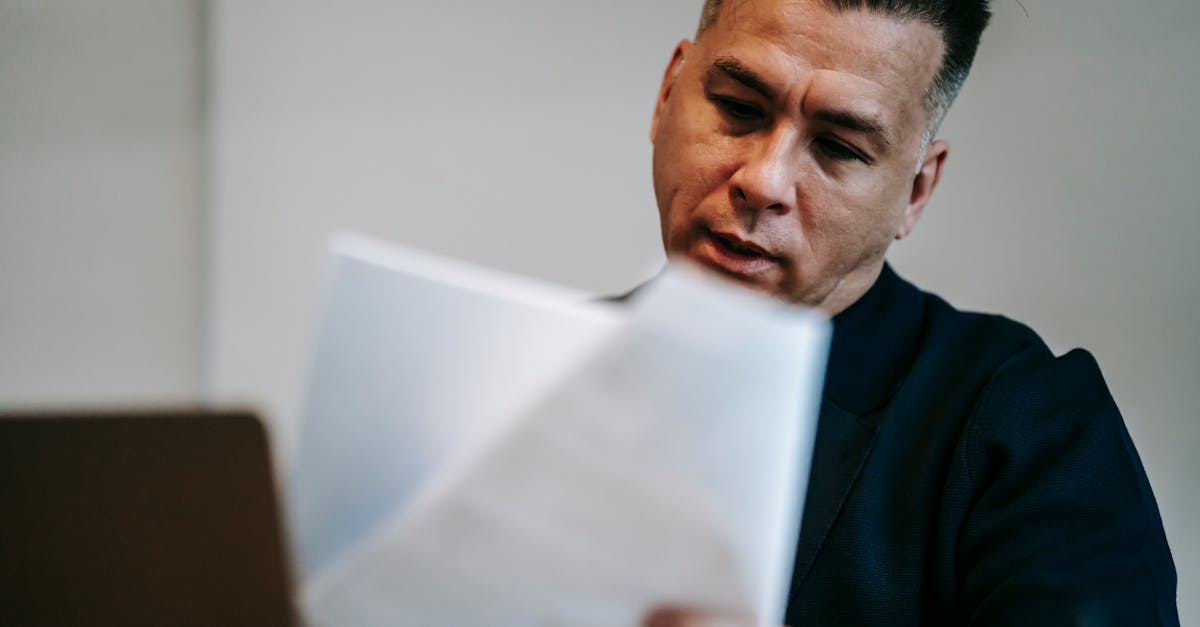
Introduction
Fraud is a pervasive threat in today's digital landscape, affecting businesses and individuals alike. Criminals continuously evolve their tactics to exploit vulnerabilities and loopholes in systems, creating a pressing need for effective fraud investigation techniques. This is where predictive analytics comes into play.
Understanding Predictive Analytics
Predictive analytics leverages advanced statistical techniques and machine learning algorithms to analyze current and historical data and predict future outcomes. By extracting knowledge and patterns from vast datasets, it enables organizations to make data-driven decisions, identify potential threats, and prevent fraudulent activities.
The Role of Predictive Analytics in Fraud Investigations
Fraud investigations heavily rely on analyzing data to uncover patterns that indicate fraudulent activities. Traditional methods involved manual examination of transaction records, but they were time-consuming and often inefficient. Predictive analytics, on the other hand, offers a more proactive approach by automating the process and significantly increasing accuracy.
Using predictive analytics, investigators can analyze vast amounts of structured and unstructured data, including financial records, customer information, social media feeds, and more. By looking for specific patterns, correlations, and anomalies within this data, they can identify potential fraudulent individuals or activities early on.
The Benefits of Predictive Analytics in Fraud Investigations
1. Early Detection: Predictive analytics enables the identification of potential fraud in its early stages, allowing investigators to take preventive measures and mitigate risks before significant losses occur.
2. Improved Efficiency: By automating the analysis process, predictive analytics saves time and resources, enabling investigators to focus on critical tasks and streamline their operations.
3. Real-time Monitoring: Predictive models can be implemented to continuously analyze incoming data and detect suspicious activities in real-time, ensuring immediate action can be taken.
4. Enhanced Accuracy: Predictive analytics considers historical data, patterns, and various parameters to identify fraudulent activities accurately, reducing false positives and false negatives.
Challenges and Considerations
While predictive analytics offers immense potential for fraud investigations, it is not without challenges. One of the main considerations is the quality and reliability of the data being analyzed. Inaccurate or incomplete data can lead to skewed predictions and potentially miss suspicious activities.
Additionally, data privacy and protection are critical concerns. Organizations must ensure that data used for predictive analytics is handled securely and adheres to legal and ethical guidelines.
Conclusion
Predictive analytics plays a crucial role in fraud investigations by empowering organizations to proactively identify and prevent fraudulent activities. By leveraging current and historical data, predictive models can effectively analyze patterns and anomalies to detect potential threats. However, it is essential for organizations to address challenges such as data reliability and privacy to fully harness the benefits of this technology.
As fraudsters continue to refine their tactics, the use of predictive analytics will undoubtedly become increasingly vital in staying one step ahead. By adopting this powerful technology, businesses and individuals can safeguard their assets and protect themselves from the ever-growing threat of fraud.
Comments:
Thank you all for joining this discussion on predictive analytics for fraud investigations!
Great article, Kanchan! Predictive analytics has indeed revolutionized fraud investigations. It enables us to identify patterns and anomalies in large datasets efficiently.
I agree, Sara. The use of AI models like ChatGPT can help uncover hidden connections and predict fraudulent activities before they occur. It's a valuable tool for fraud prevention.
I'm impressed with the advancements in predictive analytics! It saves so much time and resources compared to traditional, manual methods of fraud detection.
Absolutely, Maria! It's incredible how predictive analytics can detect fraud patterns in real-time and provide immediate alerts for investigation. It's a game-changer.
I have a question for Kanchan. How does ChatGPT technology handle data privacy concerns in fraud investigations?
Good question, Emily. ChatGPT adheres to strict data privacy protocols. It doesn't store personal information and ensures anonymity. Data security is a top priority.
That's reassuring, Kanchan. In fraud investigations, confidentiality and privacy of sensitive information are crucial. It's good to know that ChatGPT prioritizes data security.
Thanks for clarifying, Kanchan. The importance of maintaining data privacy cannot be emphasized enough, especially in sensitive cases like fraud detection.
I enjoyed reading the article, Kanchan. The capability of predictive analytics to uncover previously undetectable fraud patterns is remarkable. It has truly enhanced fraud investigations.
Thank you, Samuel. Predictive analytics has come a long way, and I'm glad it's making a positive impact on fraud investigation techniques.
As technology advances, fraudsters find new ways to commit fraud. How can predictive analytics keep up with their evolving tactics?
Great point, Sophia! Fraudsters constantly adapt, but predictive analytics evolves too. It learns from new patterns and utilizes machine learning algorithms to detect emerging fraud techniques.
I think it would be helpful if predictive analytics could have a feedback loop with investigators. Human expertise combined with AI models can enhance fraud detection.
Absolutely, Oliver! Feedback from investigators is invaluable for training and fine-tuning predictive analytics models. Collaboration between humans and AI is the way forward.
Agreed, Kanchan! Collaborative efforts can leverage the strengths of both humans and AI, enabling effective fraud prevention and detection.
I'm curious about the scalability of using ChatGPT for fraud investigations. Can it handle large volumes of data effectively?
Good question, Robert. ChatGPT technology is designed to scale horizontally, allowing efficient processing of large datasets. It can handle the increasing demands of fraud investigation operations.
That's impressive, Kanchan! As data volumes grow, it's crucial to have scalable solutions for fraud detection. It ensures timely and effective analysis.
I've seen predictive analytics being used in some industries. How transferrable are these techniques to fraud investigations in different domains?
Good question, Daniel. The techniques and concepts of predictive analytics are applicable across various domains. It's adaptable, and with proper customization, it can be effective in fraud investigations regardless of the industry.
That's reassuring to know, Kanchan. The ability to apply predictive analytics in different industries is a testament to its versatility and value.
Thanks for the clarification, Kanchan. It's good to know that predictive analytics can be adapted to different industries, making it a valuable tool for fraud detection across domains.
I'm glad to hear that predictive analytics continues to evolve. It shows promise in staying one step ahead of fraudsters. Great article, Kanchan!
Thank you all for your engaging comments and questions. I appreciate your interest and insights!
Predictive analytics can be a real game-changer in fraud investigations. It enables proactive measures rather than reactive responses.
I'm curious about the limitations of ChatGPT in fraud investigations. What are the challenges it might encounter?
Good question, Ella. ChatGPT may face challenges when data is incomplete or inaccurate, as it relies on the quality and integrity of the information it processes. Additionally, the model's predictions may not always be 100% accurate, requiring human validation and expertise.
That makes sense, Kanchan. The importance of data quality and the need for human intervention in validating model predictions are vital considerations in fraud investigations.
I appreciate the emphasis on collaboration between AI and human expertise in fraud investigations. It combines the power of technology with critical thinking for better outcomes.
Indeed, Sophie. Humans and AI complement each other's strengths, resulting in more robust fraud prevention and detection strategies.
Predictive analytics is a powerful tool, but we must ensure ethical use and accountability. Human oversight and responsible deployment are imperative.
You're absolutely right, Jennifer. Ethical considerations and responsible deployment are essential to maintain trust and fairness while leveraging predictive analytics in fraud investigations.
I've seen predictive analytics bring positive changes in the fraud detection landscape. It can help streamline operations and allocate resources effectively.
Certainly, George. The efficiency and accuracy of predictive analytics allow organizations to focus resources on high-priority fraud cases, increasing the overall effectiveness of fraud investigations.
Predictive analytics needs continuous updates and maintenance to adapt to evolving fraud techniques. Staying ahead of fraudsters is an ongoing challenge.
You're right, Clark. Regular updates and ongoing maintenance of predictive analytics models are necessary to address emerging fraud patterns. Dynamic adaptation is key.
I'm glad to see that there is recognition of the need for constant evolution. Continuous improvement is crucial in the fight against fraud.
Predictive analytics has transformed fraud investigations into a proactive process. It allows organizations to act before the damage is done.
Indeed, Olivia. By leveraging predictive analytics, organizations can minimize losses, protect their stakeholders, and mitigate risks effectively.
Kanchan, can you elaborate on how predictive analytics enhances efficiency in fraudulent transaction detection?
Of course, Laura. Predictive analytics algorithms can quickly analyze transactional data, flagging potentially fraudulent activities in real-time. This enables prompt action and reduces the time spent on manual investigation of false positives.
That's true, Kanchan. The ability to analyze transactions rapidly helps identify suspicious patterns early, minimizing monetary losses from fraudulent activities.
As fraudsters become more sophisticated, the need for advanced analytical tools like ChatGPT grows. It's important to stay one step ahead.
Absolutely, Emma. The development of advanced tools like ChatGPT equips fraud investigators with the necessary capabilities to combat increasingly sophisticated fraud techniques.
Predictive analytics can significantly reduce investigation time, allowing investigators to focus on high-risk cases and prevent financial losses.
Indeed, Lucas. By automating certain aspects of fraud investigation, predictive analytics frees up resources and enables investigators to prioritize cases effectively.
Kanchan, can you share any success stories where predictive analytics made a substantial impact on fraud investigations?
Certainly, Sophia. In a recent case, predictive analytics algorithms flagged a series of seemingly unrelated transactions, leading to the early detection of a money laundering operation. This resulted in swift action and significant financial recovery.
That's impressive, Kanchan! These success stories demonstrate the huge potential predictive analytics holds in combating fraud and protecting companies from financial harm.