Enhancing Process Safety: Leveraging ChatGPT for Predictive Maintenance in Industrial Technology
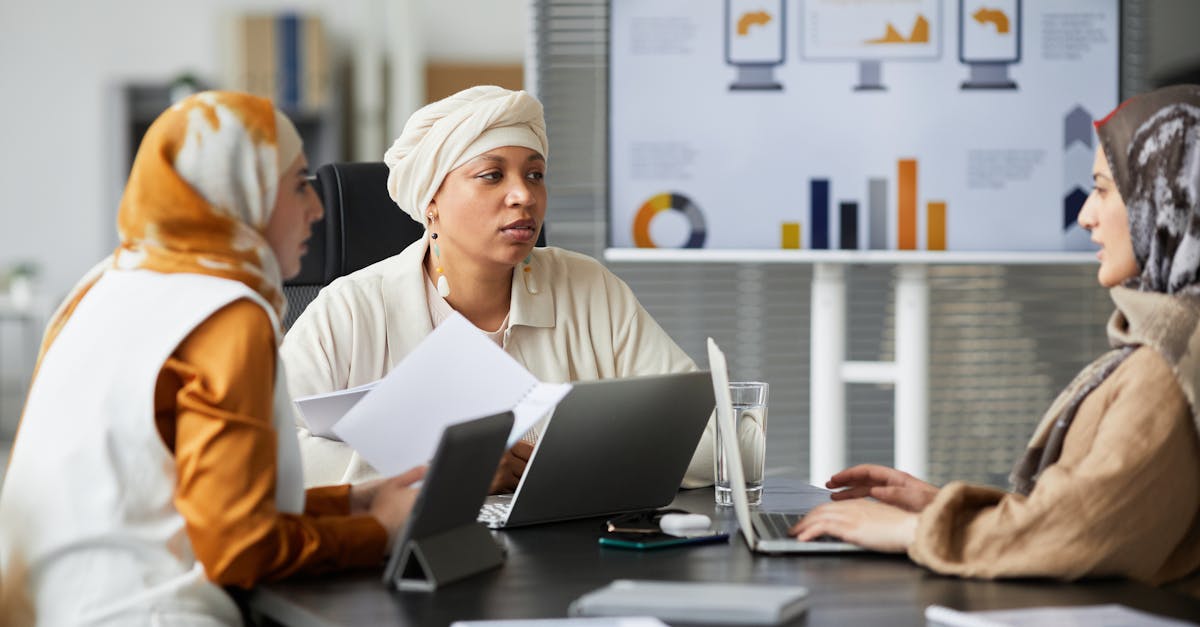
Process safety is a crucial aspect of industrial operations, as the prevention of accidents and equipment breakdowns is of utmost importance for both safety and efficiency. Traditional maintenance practices often rely on scheduled inspections or reactive repairs, which can lead to costly downtime and potential hazards if unexpected equipment failures occur. However, with the advancements in technology, particularly in machine learning, predictive maintenance has emerged as a valuable tool to identify and prevent such breakdowns before they happen.
What is Predictive Maintenance?
Predictive maintenance is an approach that leverages data analysis and machine learning algorithms to predict equipment failures or breakdowns. By continuously monitoring and analyzing data from various sources such as sensors, historical records, and real-time operating conditions, machine learning models can identify patterns and anomalies that indicate the potential for equipment failure. This proactive approach allows maintenance teams to take corrective actions before a breakdown occurs, minimizing the impact on safety and productivity.
Application of Machine Learning in Predictive Maintenance
Machine learning algorithms play a vital role in predicting equipment breakdowns based on historical data and real-time monitoring. These algorithms can identify complex patterns and correlations that may not be apparent through traditional analysis methods. Through the use of machine learning, process safety teams can gain insights into equipment health, identify early warning signs, and make more informed decisions about maintenance interventions.
Data Collection and Analysis
The predictive maintenance process begins with the collection of relevant data. This data includes historical maintenance records, sensor data, operating conditions, and other parameters that can impact equipment performance. The collected data is then analyzed using machine learning algorithms to find patterns indicating degradation or failure. These algorithms learn from historical data to make predictions about future equipment behavior.
Fault Detection and Classification
Machine learning models can be trained to detect and classify faults based on patterns identified in the data. By comparing real-time sensor readings with historical data, the models can identify anomalies and classify them into different fault categories. This helps maintenance teams prioritize their efforts and allocate resources effectively.
Remaining Useful Life Prediction
Predictive maintenance also enables the estimation of the remaining useful life (RUL) of equipment. By analyzing sensor data and performance indicators, machine learning models can predict how much time an asset has before an impending failure. This allows maintenance teams to plan interventions ahead of time, reducing downtime and improving process safety.
Benefits of Predictive Maintenance in Process Safety
The adoption of predictive maintenance using machine learning brings several benefits to process safety and efficiency:
- Improved Safety: By predicting equipment breakdowns, potential safety hazards can be addressed proactively, preventing accidents and ensuring the well-being of personnel.
- Reduced Downtime: Predictive maintenance helps avoid unexpected equipment failures, minimizing downtime and maximizing productivity.
- Cost Savings: By identifying equipment issues early, maintenance interventions can be planned and scheduled efficiently, reducing repair costs and preventing larger-scale breakdowns.
- Enhanced Equipment Performance: Continuous monitoring and analysis of equipment data allow for better understanding of asset health and performance, leading to optimized operations and improved overall efficiency.
Conclusion
Machine learning has revolutionized the way process safety teams approach maintenance practices. Predictive maintenance, enabled by advanced data analysis and machine learning algorithms, offers significant advantages in predicting equipment breakdowns before they happen. By harnessing the power of machine learning, organizations can improve safety, reduce downtime, and optimize the efficiency of their industrial operations.
Comments:
Thank you all for reading my blog post on 'Enhancing Process Safety: Leveraging ChatGPT for Predictive Maintenance in Industrial Technology'. I'm excited to hear your thoughts and engage in a discussion!
Great article, Ghadeer! I found the concept of using ChatGPT for predictive maintenance fascinating. It has the potential to revolutionize the industrial technology sector.
I agree, Sarah. ChatGPT's ability to analyze and predict maintenance needs can greatly improve efficiency and prevent potential accidents in industries.
I agree, Andrew. It's amazing how artificial intelligence can be applied to enhance safety measures and prevent accidents in hazardous industrial environments.
Absolutely! The predictive capabilities of ChatGPT can save companies a lot of time and resources by identifying maintenance requirements before they become critical issues.
While ChatGPT seems promising, have there been any real-world applications of this technology in the industrial sector? I'd love to learn about some practical case studies.
That's a great question, Alex. ChatGPT has indeed been applied in various industrial settings. One notable example is a major oil refinery that utilized ChatGPT for maintenance predictions, resulting in a significant reduction in unplanned downtime.
I'm curious about the training process for ChatGPT. How is it trained to accurately predict maintenance needs in such complex industrial environments?
Excellent question, Robert. ChatGPT is trained on large datasets that include historical maintenance records, sensor readings, and other relevant data from industrial environments. It learns patterns and correlations to make accurate predictions.
Thanks for explaining, Ghadeer. It's impressive how ChatGPT can make sense of complex industrial data to generate meaningful insights.
I can see the benefits of using ChatGPT for predictive maintenance, but what are the potential challenges and limitations associated with this approach?
That's an important aspect to consider, Michelle. One challenge is the quality of data. The accuracy of predictions heavily relies on the quality of historical data available. Additionally, maintenance requirements can sometimes be influenced by unpredictable factors, making accurate predictions challenging in those cases.
Ghadeer, do you think ChatGPT can completely replace human expertise in the field of industrial maintenance?
Interesting question, Jeffrey. While ChatGPT can aid in predictive maintenance, it shouldn't replace human expertise. Humans bring critical insights and decision-making capabilities that complement the predictions made by ChatGPT. It should be seen as a valuable tool to enhance human performance.
I agree, Ghadeer. Human expertise remains critical, especially in complex scenarios where judgment and decision-making based on various factors are required.
I wonder if ChatGPT can adapt to different industrial sectors and their unique maintenance requirements. Can it be trained specifically for particular industries?
Good question, Nathan. ChatGPT can indeed be fine-tuned and trained specifically for different industrial sectors. By incorporating domain-specific data and adjusting the training process, it can adapt to the unique maintenance requirements of industries.
I'm concerned about the security aspect. What measures are in place to ensure that sensitive industrial data used for training ChatGPT is protected?
Valid concern, Linda. Protecting sensitive industrial data is crucial. Access controls, encryption, and anonymization techniques are employed to safeguard the data used for training ChatGPT. Furthermore, data handling complies with relevant industry standards and regulations.
Appreciate the clarification, Ghadeer. Maintaining data security is vital, especially when dealing with sensitive information in industrial environments.
I'd like to know more about the cost-effectiveness of implementing ChatGPT for predictive maintenance. Can smaller companies afford such technology?
Good point, Thomas. Initially, implementing ChatGPT for predictive maintenance might require some investment in deployment and data preparation. However, in the long run, the potential cost savings resulting from reduced downtime and improved efficiency can outweigh the initial costs.
Absolutely! Proactively addressing maintenance needs can avoid catastrophic failures and ensure the safety of workers and the environment.
You're right, Emily. Identifying maintenance requirements beforehand can save companies from costly repairs and mitigate potential risks.
Absolutely, Emily. Proactive measures in maintenance are crucial to ensure the long-term sustainability of industries.
Well said, Sarah. It's about avoiding potential disasters and establishing a culture of continuous improvement.
Agreed, Emily. Effective maintenance planning can create safer work environments and reduce the environmental impact of industrial operations.
Definitely, Alex. Drawing insights from complex data can empower decision-makers to take proactive actions and optimize industrial processes.
I also wonder if ChatGPT can handle dynamic changes in industrial processes or if it requires retraining whenever changes occur.
Good question, Michelle. ChatGPT can adapt to some degree of dynamic changes in industrial processes without requiring retraining. However, significant changes may necessitate retraining to ensure accurate predictions in the updated environment.
Thanks for the clarification, Ghadeer. Flexibility in accommodating dynamic changes is crucial in highly agile industries.
Exactly, Michelle. AI technologies should be seen as enablers, complementing human expertise, rather than replacements.
That's impressive! Having industry-specific models built on ChatGPT makes it more valuable for industrial users.
I see the potential benefits in the long term. Companies of all sizes should consider the economic advantages and prioritize the safety of their operations.
ChatGPT's predictive maintenance capabilities can significantly improve overall equipment effectiveness (OEE) in factories.
Indeed, Michael. Improved maintenance predictions can reduce downtime, increase equipment availability, and enhance overall productivity in manufacturing facilities.