Enhancing Product Backlog Management in Application Lifecycle Management with ChatGPT
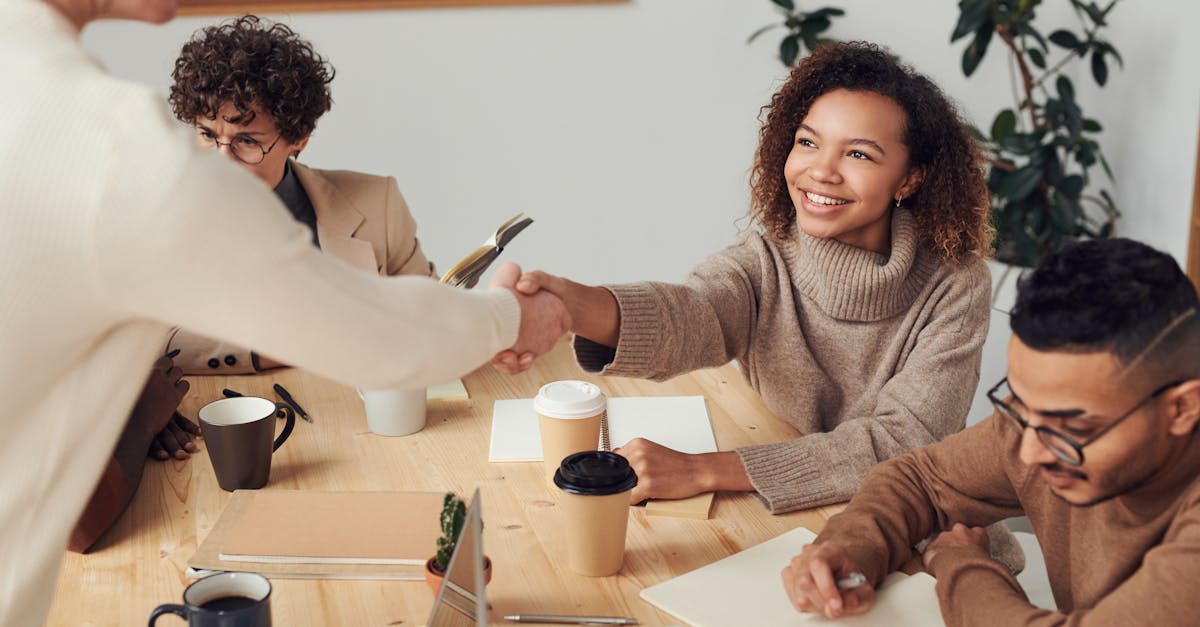
Application Lifecycle Management (ALM) is a set of practices, processes, and tools used to manage the entire lifecycle of a software application. It includes requirements management, release management, configuration management, and more. One important aspect of ALM is product backlog management, which involves organizing and prioritizing the features and enhancements that need to be developed.
A product backlog is a list of all the desired features, enhancements, and bug fixes for a software application. It serves as the single source of truth for what needs to be done in the development process. To effectively manage the product backlog, ALM tools and practices can be utilized.
Benefits of ALM in Product Backlog Management
ALM provides several benefits for product backlog management:
- Organizing and prioritizing: ALM tools allow teams to easily organize and prioritize items in the product backlog. Features can be categorized, and their priority can be adjusted based on business value, customer feedback, or other criteria.
- Generating user stories: ALM tools often provide templates and guidelines for creating user stories. User stories capture the requirements from the perspectives of end users and enable teams to better understand the needs and expectations of those using the software.
- Planning iterations/sprints: ALM tools help teams plan iterations or sprints by allowing them to assign user stories from the product backlog to specific development cycles. This ensures that the development effort is organized and time-boxed, leading to better productivity and focus.
- Tracking progress: ALM tools provide visualization features to track the progress of user stories and overall project development. Teams can easily see how much work has been completed, what is pending, and any bottlenecks that need to be addressed.
- Collaboration: ALM tools facilitate collaboration among team members, stakeholders, and other stakeholders involved in product backlog management. They provide a centralized platform where everyone can access and contribute to the backlog, ensuring efficient communication and alignment.
Conclusion
Application Lifecycle Management is a valuable practice in product backlog management. It provides tools and practices that enable teams to effectively organize, prioritize, and track the development efforts. Utilizing ALM ensures a streamlined and efficient development process, leading to successful software applications that meet the needs and expectations of end users.
Comments:
Thank you all for your interest in my article on enhancing product backlog management with ChatGPT. I'm excited to discuss it further!
Great article, Jim! ChatGPT seems like a powerful tool for improving collaboration and communication in application lifecycle management.
I agree, Emily. By enabling real-time conversations and reducing the need for constant meetings, ChatGPT can increase productivity and efficiency.
I have some concerns about relying too heavily on AI-based tools. They can't fully understand the complexities of product backlog management and may miss important nuances.
That's a valid point, Maria. While ChatGPT can assist in certain aspects, it should never replace human judgment and expertise in product backlog management.
I agree with Jim. AI should be seen as a helpful aid, not a complete replacement for human decision-making and understanding.
Has anyone implemented ChatGPT in their ALM processes? I'm curious to hear about real-world experiences.
I have tried integrating ChatGPT into our ALM workflow, and it has been quite beneficial. It saves time and enhances collaboration, especially for remote teams.
Glad to hear that, Sarah. It's always encouraging to see positive experiences with implementing new tools like ChatGPT. How did your team adapt to using it?
Initially, there was some resistance, but after understanding the benefits and providing proper training, everyone embraced it. Now, it's an integral part of our ALM process.
I see the value in using ChatGPT, but what about data privacy and security concerns? Has that been addressed?
Data privacy and security are crucial aspects, David. When implementing ChatGPT, it's important to ensure that appropriate measures are taken to protect sensitive information.
Jim, could you explain more about the measures for protecting sensitive information when using ChatGPT?
Certainly, David. Implementing appropriate access controls, encryption, and secure connection protocols are critical to protect sensitive data when using ChatGPT.
Thank you for the clarification, Jim. Implementing ChatGPT securely is indeed crucial in today's data-driven landscape.
You're absolutely right, David. Security considerations should always be at the forefront when adopting AI-based tools.
It would be interesting to hear more about the integration process. How easy or complex was it to incorporate ChatGPT into existing ALM tools?
In my experience, integration wasn't too difficult. However, it required close collaboration with our development team and some custom development work to achieve a seamless integration.
Agreed, Emily. Integrating ChatGPT into ALM tools may vary depending on the existing infrastructure and the level of customization desired.
Indeed, the ease of integration can vary. It's important to assess your specific needs, consult with AI experts, and plan accordingly for a successful integration.
I have concerns about potential biases in AI models like ChatGPT. How can we ensure fair and unbiased recommendations?
Addressing biases is crucial, Sarah. It involves training ChatGPT on diverse datasets and regularly reviewing its output to ensure fairness and avoid undue influence.
Jim, I enjoyed reading your article. ChatGPT indeed seems like a promising tool for backlog management. Have you considered any potential drawbacks?
Thank you, Timothy. While ChatGPT has its strengths, potential drawbacks could include over-reliance on AI, difficulty in handling context-specific knowledge, and challenges in maintaining model accuracy over time.
Jim, would you recommend any best practices for reviewing ChatGPT's output to identify biases?
Sarah, could you share some specific use cases where ChatGPT has been particularly beneficial in your ALM workflow?
Sure, Mark. ChatGPT has helped in tasks like requirements elicitation, clarifying user stories, and prioritizing backlog items. It has reduced communication gaps and improved efficiency.
I think AI companions like ChatGPT can become increasingly proficient with more training data and continuous feedback loops.
Emily, could you elaborate on the custom development work required to integrate ChatGPT seamlessly?
Sure, Marcia. We needed to develop custom APIs and backend infrastructure to connect our existing ALM tools with ChatGPT's capabilities. It involved collaboration between developers and AI experts.
Thanks, Emily. It seems like integrating ChatGPT requires a collaborative effort from different teams to ensure a smooth adoption.
Indeed, Alice. Collaboration and close coordination are essential to address any technical and process-related challenges during the integration process.
Training and providing feedback loops to ChatGPT has definitely helped it better understand our specific needs and improve over time.
We've been considering implementing ChatGPT in our ALM processes, but what are the associated costs?
Cost considerations depend on factors like the extent of integration, customization requirements, and the chosen implementation approach. It's essential to evaluate those aspects.
It's helpful to have a diverse review team that includes individuals from different backgrounds. They can conduct regular audits, evaluate output samples, and provide feedback to address any biases effectively.
To minimize biases, should we consider training ChatGPT on industry-specific datasets?
Yes, Daniel. Training ChatGPT on industry-specific datasets can help reduce biases and improve its understanding of context and nuances specific to the application lifecycle management domain.
Jim, is it advisable to use external AI ethics experts to review and validate ChatGPT's output for biases?
Certainly, Daniel. Involving external AI ethics experts can provide an unbiased perspective and help organizations ensure fair and ethical AI practices.
What steps can we take to ensure data privacy while using ChatGPT?
To ensure data privacy, it's crucial to comply with relevant data protection regulations, implement necessary security measures, and regularly review the data handling practices related to ChatGPT.
I believe AI companions can become great aids, but they should always augment human intelligence rather than replace it.
Exactly, Alex. AI companions like ChatGPT are most powerful when they collaborate with human experts, leveraging the strengths of both humans and AI.
Could you provide a rough estimation of the costs involved in implementing ChatGPT?
Adam, the costs can vary significantly based on factors like the scope of integration, the licensing model, and any additional development efforts. It's recommended to consult with AI vendors or experts for more accurate estimations.
Training on industry-specific datasets seems like a practical approach to avoid potential biases in ChatGPT's responses.
Indeed, Linda. Tailoring the training datasets to the application lifecycle management domain can significantly improve ChatGPT's performance and minimize biases.
Collaborating with AI companions like ChatGPT can speed up decision-making and foster innovation in ALM by providing different perspectives.
Absolutely, Alex. Diverse perspectives, aided by AI, can lead to more well-rounded decisions and drive continuous improvement in ALM practices.