Enhancing Protocol Development in Flow Cytometry: Harnessing the Power of ChatGPT
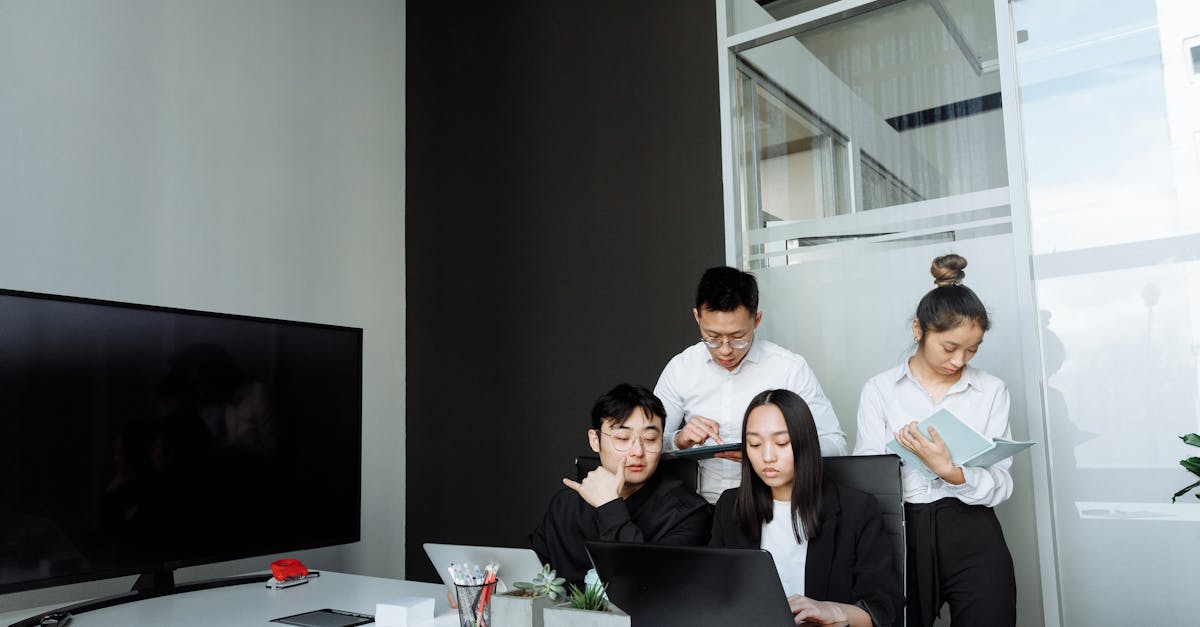
Flow cytometry is a powerful technique used to analyze and quantify the characteristics of individual cells or particles in a heterogeneous population. It is widely used in various research fields, such as immunology, cancer biology, and stem cell research, to understand cellular functions and identify specific cell types.
Developing a robust and optimized protocol is crucial for obtaining accurate and reliable data from flow cytometry experiments. Traditionally, protocol development involved extensive trial and error, which often required significant time and resources. However, with the advancement in artificial intelligence (AI) technology, the process can now be more streamlined and efficient.
Using AI models, researchers can train the system to analyze large datasets of flow cytometry experiments, learn the patterns, and identify the optimal combinations of parameters for specific experimental needs. This technology offers valuable insights and recommendations to improve the efficiency and accuracy of flow cytometry protocols.
One of the key advantages of AI-driven protocol development is the ability to reduce experimental variability. By analyzing multiple parameters simultaneously, AI models can identify the optimal conditions for staining, sample preparation, and instrument settings. This minimizes experimental variability between samples and enhances the overall reproducibility of the results.
Another benefit of using AI in protocol development is the accelerated optimization process. AI models can quickly analyze a vast amount of data and generate suggestions for optimizing experimental conditions. Researchers can then validate these suggestions in the lab, leading to more efficient protocol development and saving time and resources.
Additionally, AI models can assist in troubleshooting flow cytometry experiments. When encountering unexpected results or technical issues, researchers can input the experimental data into the AI system to obtain potential explanations and suggestions for improvement. This can help researchers identify and rectify experimental errors promptly.
It is important to note that AI is not meant to replace the expertise and experience of the researchers. Instead, it serves as a valuable tool in the protocol development process, providing data-driven insights and suggestions. Researchers can still utilize their knowledge and judgment to make informed decisions based on the AI recommendations.
In conclusion, AI-driven protocol development is revolutionizing flow cytometry experiments by providing valuable insights, recommendations, and troubleshooting assistance. By leveraging AI models, researchers can optimize experimental conditions, reduce variability, and accelerate the protocol development process. This technology holds immense potential to enhance the efficiency, accuracy, and reproducibility of flow cytometry experiments in various research areas.
This article provides an overview of how AI can assist in developing and optimizing protocols for flow cytometry experiments. Embracing AI in flow cytometry protocol development can unlock new possibilities and enable researchers to explore deeper into the cellular world.
Comments:
Thank you all for taking the time to read my article on enhancing protocol development in flow cytometry. I'm excited to hear your thoughts and insights!
Great article, Sameer! I really enjoyed reading about the potential of harnessing ChatGPT for protocol development in flow cytometry. It's impressive how AI can assist in optimizing experimental procedures.
I agree, Michael! The ability to utilize AI technology for protocol development could significantly streamline research processes in flow cytometry and improve efficiency.
Absolutely, Emily! As someone who works in a flow cytometry lab, I can see the benefits of incorporating AI in protocol optimization. It could save us time and resources.
Thank you, Michael and Emily! Indeed, AI has the potential to revolutionize how we approach protocol development. It can help researchers refine variables and reduce errors.
While I can appreciate the potential benefits, I have concerns about relying too heavily on AI for protocol development. Human expertise is critical, and AI cannot replace it entirely.
You make a valid point, Richard. AI should be seen as a tool to augment human expertise, rather than replace it. Collaborative efforts between AI and researchers can lead to better outcomes.
I completely agree with Richard. AI can provide valuable suggestions, but researchers should always exercise critical thinking and consider the limitations of AI algorithms.
Absolutely, Connie. Critical thinking remains essential in research. AI can help with data analysis and optimization, but human judgment is irreplaceable.
I'm curious to know if ChatGPT considers different cell types and their specific requirements while suggesting protocol optimizations. Flow cytometry protocols can vary depending on the cells involved.
That's an important consideration, Sarah. ChatGPT has been trained on a wide range of protocols, including various cell types. However, researchers should still be cautious and validate AI-generated suggestions for their specific use cases.
I have concerns about the availability and affordability of AI-powered tools like ChatGPT for smaller research labs or individual researchers. Will it be accessible to everyone?
Valid concern, David. While there may be costs associated with AI tools initially, there is a push to make them more accessible and affordable. Open-source solutions and collaborations can help bridge the gap.
That's good to know, Sameer. Accessibility is crucial to ensure that the benefits of AI in flow cytometry protocol development reach researchers from diverse backgrounds and institutions.
I wonder if integrating AI in flow cytometry protocols might increase standardization across labs. It could potentially reduce variability and allow for better comparisons between studies.
You bring up an excellent point, William. Utilizing AI for protocol optimization can aid in achieving better standardization and reproducibility, which are vital for advancing scientific research.
I believe ChatGPT could also facilitate collaboration and knowledge sharing among researchers. It could help create a community-driven approach where insights are shared for continuous improvement.
Absolutely, Michael! AI-powered tools like ChatGPT have the potential to foster collaboration and facilitate the exchange of best practices, ultimately benefiting the entire research community.
One concern I have is the interpretability of AI-generated protocols. How can we trust the suggestions made by AI if we can't fully understand how it arrived at those recommendations?
Interpretability is indeed crucial, Emily. Efforts are being made to develop explainable AI models that provide insights into the decision-making process. Transparent and interpretable AI is vital for building trust among researchers.
I'm excited about the potential of AI in flow cytometry protocol development, but I also worry about the learning curve. Will researchers need extensive AI training to utilize these tools effectively?
It's a valid concern, Olivia. While familiarity with AI concepts can be beneficial, efforts are being made to develop user-friendly interfaces that minimize the learning curve. AI tools should be accessible and intuitive for researchers.
I'm glad to see advancements in flow cytometry protocol development. It has the potential to accelerate discoveries and increase research output. Exciting times ahead!
Indeed, Connie! The future of flow cytometry protocol development looks promising with the integration of AI. It's an exciting field to be a part of!
I can see ChatGPT becoming an invaluable tool for researchers working in flow cytometry. The potential to optimize protocols quickly and accurately is incredible!
Although I have concerns, I can't deny the potential benefits of AI in flow cytometry protocol development. Sameer, thank you for shedding light on this fascinating topic!
You're welcome, David! It's important to discuss the potential benefits as well as the concerns surrounding AI in flow cytometry. Thank you for engaging in the conversation!
I'm excited to see how AI continues to shape the field of flow cytometry protocol development. It has the potential to revolutionize the way we conduct experiments.
Absolutely, Michael! The progress we are witnessing is just the beginning. AI-powered protocol development has the potential to unlock new possibilities and further our understanding of biological systems.
I can't wait to see AI-powered tools like ChatGPT become more accessible to researchers. It could truly transform the way we approach flow cytometry protocols.
I share your enthusiasm, Olivia! Making AI tools accessible and empowering researchers to leverage their potential is a priority. Exciting times lie ahead for flow cytometry!
I appreciate how the article acknowledged the need for collaboration between AI and researchers. It highlights the importance of human expertise throughout the process.
Absolutely, Sarah! Flow cytometry protocol development should be a collaborative effort, with AI providing insights and researchers contributing their expertise. Together, we can achieve better outcomes.
Standardization and reproducibility are essential in scientific research. If AI tools can assist in achieving these goals, it's definitely a step in the right direction.
I couldn't agree more, William! Standardization and reproducibility are crucial for advancing scientific knowledge. AI can play a significant role in improving these aspects in flow cytometry.
I appreciate how Sameer addressed the potential concerns surrounding AI-powered protocol development. It's essential to have open discussions and consider all aspects.
Thank you, Emily! Open discussions help us address concerns, understand different perspectives, and drive the responsible integration of AI in flow cytometry protocol development.
The future of flow cytometry looks promising with advancements like ChatGPT. Kudos to the researchers and developers working towards enhancing protocol development!
Absolutely, Connie! The contributions from the research and development communities are driving flow cytometry forward. Exciting times are ahead, indeed!
Thank you, Sameer, for sharing your insights on the potential of ChatGPT in flow cytometry protocol development. It was an enlightening read!