Enhancing Quality Control in Deepwater Technology Using ChatGPT
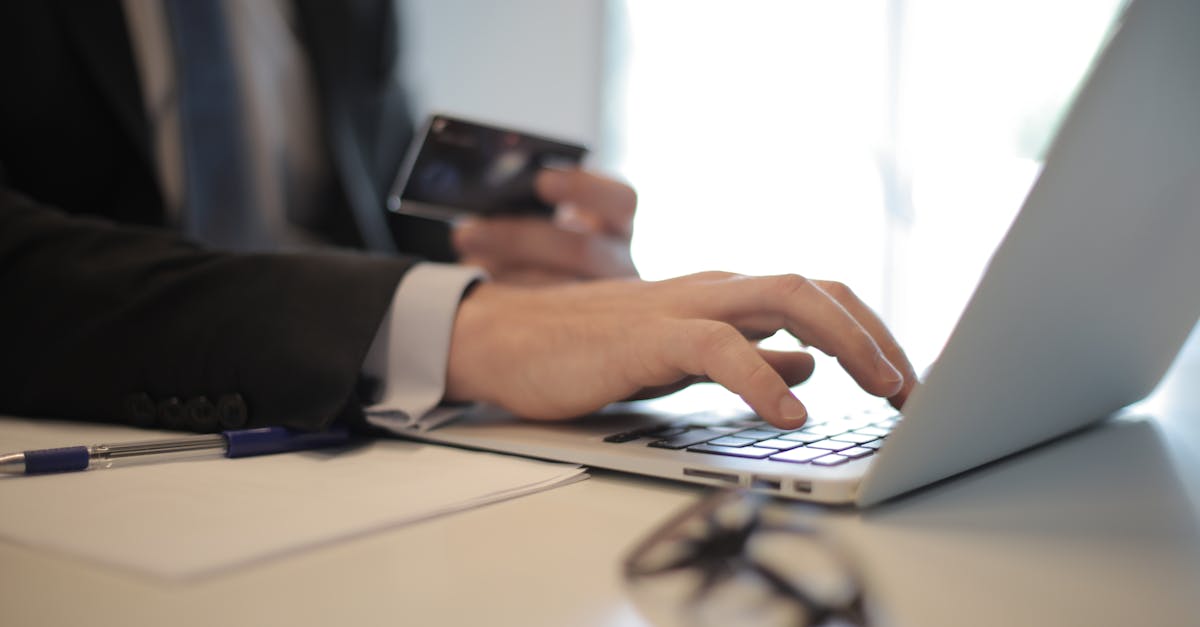
The advancements in technology have revolutionized various industries, including the quality control sector. Deepwater technology, with its sophisticated capabilities, has emerged as a valuable tool in maintaining accuracy and efficiency in quality control processes. In this article, we explore how Deepwater technology can support quality control and ensure seamless operations.
Overview of Deepwater Technology
Deepwater technology refers to the use of advanced algorithms and machine learning techniques to analyze and interpret vast amounts of data collected in deepwater environments. These environments are often challenging to access and can pose risks to human operators.
Deepwater technology leverages powerful computational models to understand, manage, and optimize operations. It is widely used in industries such as oil and gas, renewable energy, and marine research.
Application in Quality Control
One of the crucial aspects of quality control is monitoring and reviewing accuracy in recorded data. Deepwater technology, combined with artificial intelligence, can analyze data collected during quality control processes and identify anomalies or inconsistencies. This allows for early detection of errors and prompt corrective actions.
Additionally, Deepwater technology can analyze overall operations and identify areas for improvement. By recognizing patterns and trends in data, it enables quality control professionals to optimize processes, minimize wastage, and reduce costs.
Benefits of Deepwater Technology in Quality Control
There are several benefits associated with the usage of Deepwater technology in quality control:
- Increased Accuracy: Deepwater technology can detect even subtle errors or anomalies in recorded data, ensuring high levels of accuracy in quality control processes.
- Efficient Monitoring: With its ability to process large amounts of data quickly, Deepwater technology enables real-time monitoring of quality control operations.
- Early Error Detection: By identifying errors early on, Deepwater technology allows for timely corrective actions, preventing potential issues from escalating.
- Process Optimization: Analyzing data patterns and trends helps optimize quality control processes, leading to enhanced operational efficiency.
- Cost Reduction: By minimizing errors and optimizing processes, Deepwater technology helps reduce costs associated with quality control activities.
Conclusion
Deepwater technology has a significant impact on quality control processes, supporting accuracy in recorded data and overall operations. Its ability to analyze vast amounts of data and identify anomalies ensures that quality control remains thorough and efficient. With the continued advancements in Deepwater technology, we can expect further improvements in quality control practices, benefiting industries across the board.
Comments:
Thank you all for joining this discussion on enhancing quality control in deepwater technology using ChatGPT. I'm excited to hear your thoughts!
This is an interesting topic, Lois. Deepwater technology has been widely used, but it's crucial to ensure quality control for safety and environmental reasons.
Absolutely, Michael. Quality control measures are critical to mitigate risks and prevent accidents in deepwater technology applications.
I agree with both of you. The advancements in AI, like ChatGPT, could potentially enhance quality control processes, but it's important to ensure its reliability and accuracy in such critical applications.
That's a valid point, Jonathan. We need to thoroughly validate the outputs generated by ChatGPT and consider its limitations before relying on it for quality control in deepwater technology.
I completely agree, Emily. It's essential to establish a robust validation framework and perform extensive testing of ChatGPT's outputs to ensure its effectiveness and identify any potential weaknesses.
Lois, trusting AI-based quality control systems with critical decision-making in deepwater technology necessitates the establishment of rigorous validation processes and continuous monitoring to ensure their reliability and accuracy.
Quality control in deepwater technology is undoubtedly critical. Besides AI-based solutions, what other methods or approaches can we use to enhance quality control in this field?
Great question, David. In addition to AI, incorporating IoT sensors and real-time monitoring systems can provide comprehensive data for quality control analysis in deepwater technology.
Indeed, Sarah. Leveraging IoT and real-time monitoring can allow us to collect valuable data on various parameters, helping us identify any anomalies or deviations from expected behavior.
Lois, in the pilot projects integrating ChatGPT, were there any notable improvements in quality control processes compared to traditional methods?
Sarah, do you think implementing ChatGPT with real-time monitoring systems would require significant changes in existing infrastructure for deepwater technology projects?
I believe a multidisciplinary approach is necessary for effective quality control in deepwater technology. Combining the expertise of engineers, data scientists, and domain specialists is crucial in identifying potential risks and designing suitable controls.
Absolutely, Alex. Deepwater technology projects involve complex systems, so it's essential to have a diverse team of experts to ensure quality control from different perspectives.
While AI can be beneficial, we must consider potential biases in the training data that could affect the quality control outcomes. Ethical considerations in AI development should not be overlooked.
I appreciate your insight, Michael. Bias in AI algorithms is an important concern that needs to be addressed. A transparent and inclusive approach to training data selection and evaluation is crucial.
Lois, could you shed some light on the specific applications of ChatGPT in deepwater technology's quality control?
Michael, ChatGPT can assist in quality control by analyzing large datasets collected from sensors, processing real-time data, and detecting potential abnormalities or risks by comparing them with predefined patterns. It can also support decision-making processes for quality control measures.
Lois, have there been any practical implementations of ChatGPT for quality control in the deepwater technology industry?
Alex, there have been pilot projects where ChatGPT was used to enhance quality control processes in deepwater technology. These projects focused on analyzing real-time data and identifying deviations from expected patterns to trigger appropriate responses or preventive actions.
Lois, would it be advisable to establish a clear escalation process when the outputs from ChatGPT contradict human experts' opinions in quality control for deepwater technology?
Lois, what are the major challenges in implementing ChatGPT for quality control in deepwater technology, and how can we overcome them?
Michael, some challenges include data availability and quality, bias in training data, and potential limitations of AI models such as ChatGPT. Overcoming these challenges requires careful selection and evaluation of training data, addressing biases, and continuous refinement of AI models through rigorous testing and feedback loops.
Lois, what measures can be taken to ensure the transparency of ChatGPT's decision-making process in quality control of deepwater technology?
Rachel, to ensure transparency, it's important to document the decision-making process of ChatGPT, including the input data, model configuration, and any pre- or post-processing steps. External audits and validation by domain experts can also provide valuable insights.
Lois, how can we handle cases where the outputs from ChatGPT contradict the opinions or recommendations of human experts in the field of deepwater technology quality control?
Jonathan, while AI can assist, maintaining open communication and fostering collaboration between AI systems and human experts is crucial in handling contradicting outputs to make informed decisions in quality control.
Lois, do you believe that AI-based quality control systems like ChatGPT will eventually replace or significantly reduce the need for human involvement in deepwater technology?
That's an important question, Rachel. While AI can greatly enhance quality control processes, human expertise, judgment, and decision-making will continue to play a crucial role in the deepwater technology industry.
Rachel, I believe AI will augment human involvement rather than fully replace it. The collaborative interaction between AI systems and human experts can lead to safer and more reliable quality control in deepwater technology.
Lois, based on the pilot projects you mentioned, what are the potential cost savings or operational benefits associated with using ChatGPT for quality control in deepwater technology?
Lois, were there any specific metrics used to evaluate the effectiveness of ChatGPT in the pilot projects for deepwater technology's quality control?
Michael, the evaluation metrics used in those pilot projects varied, but they generally focused on accuracy, precision, recall, and comparing ChatGPT's performance against established quality control benchmarks.
Lois, considering the importance of safety and reliability in deepwater technology, what steps can be taken to ensure continuous improvement and refinement of AI models like ChatGPT for quality control purposes?
Michael, continuous feedback loops involving domain experts, ongoing validation, and periodic model retraining based on new data can contribute to the continuous improvement and refinement of AI models in quality control for deepwater technology. Regular audits can also identify areas for enhancement.
I think it's also worth mentioning the role of regulations in ensuring quality control in deepwater technology. Strict adherence to safety standards and protocols is necessary to prevent accidents and environmental damage.
Rachel, you're absolutely right. In-depth understanding and compliance with applicable regulations are vital to ensure quality control in deepwater technology and prevent potential disasters.
Emily, how can we effectively validate the outputs generated by ChatGPT for quality control purposes in deepwater technology?
Jonathan, to validate the outputs generated by ChatGPT, one approach could involve comparing its analysis with historical data and feedback from human domain experts to ensure consistency and accuracy.
Emily, what potential limitations or challenges do you think we might face when validating ChatGPT's outputs using historical data and human expert feedback?
Absolutely, Rachel. Regulatory frameworks play a vital role in establishing a baseline for quality control requirements in deepwater technology projects.
Additionally, conducting thorough risk assessments and incorporating preventive and corrective measures throughout the project lifecycle are key aspects of quality control in deepwater technology.
Very true, Emily. It's crucial to identify potential risks and implement robust risk management strategies to ensure high-quality standards in deepwater technology.
I'm glad to see such a comprehensive discussion on various factors contributing to quality control in deepwater technology. This exchange of thoughts is invaluable.
Indeed, Lois. The collaboration of professionals in these discussions helps us gain new insights and approaches to improve the quality control of deepwater technology.
Sarah, do you think combining ChatGPT's capabilities with real-time monitoring systems could lead to more accurate and timely identification of potential quality issues in deepwater technology?
Jonathan, combining ChatGPT with real-time monitoring systems can enable continuous data analysis along with advanced AI-powered algorithms. This integration has the potential to improve the accuracy and speed of quality control in deepwater technology.
Absolutely, Sarah. It's essential to have open dialogues like this to collectively enhance the quality control processes in deepwater technology.
Additionally, the evaluation process considered other factors such as the time taken for decision-making, false positive and false negative rates, and the ability to detect known anomalies.
In the pilot projects, cost savings were achieved through improved efficiency in detecting potential issues and initiating preventive measures promptly. The operational benefits included enhanced safety, reduced downtime, and optimized resource allocation, ultimately leading to cost-effectiveness in deepwater technology projects.