Enhancing Quality Control in Flow Cytometry with ChatGPT
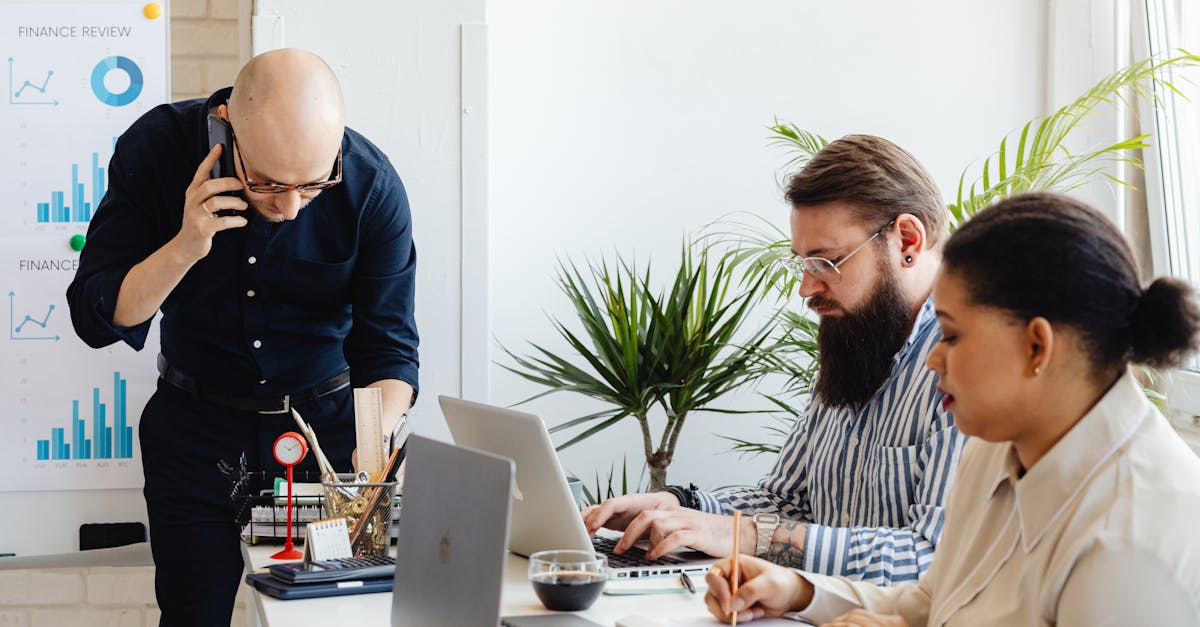
Introduction to Flow Cytometry
Flow cytometry is a technology used in biotechnology and medical research to analyze and sort cells. It allows scientists to measure and characterize multiple properties of individual cells quickly and efficiently. By using fluorescently labeled antibodies, flow cytometry can sort and separate cells based on size, shape, and protein expression levels. This technology has revolutionized various fields, including immunology, hematology, and microbiology.
Quality Control in Flow Cytometry
Quality control plays a crucial role in ensuring the accuracy and reliability of flow cytometry results. Any variations or inconsistencies in the analysis process can lead to inaccurate data, affecting research outcomes and conclusions. To address this challenge, a real-time tracking and inspection model has been developed to monitor the flow cytometry procedures and ensure quality standards are met.
Real-time Tracking and Inspection Model
The real-time tracking and inspection model utilizes computer vision and machine learning algorithms to continuously monitor and assess the flow cytometry process. By analyzing the images obtained during the analysis, the model can detect potential issues and deviations from the standard protocols. It can provide real-time feedback to the operator, enabling corrections to be made immediately.
Key Features and Benefits
The real-time tracking and inspection model offers several key features and benefits:
- Quality Assurance: By monitoring the flow cytometry process in real-time, the model ensures that all steps, including sample preparation, staining, and analysis, are performed correctly and consistently. This reduces the risk of human error and enhances the overall quality of the results.
- Automatic Error Detection: The model can automatically detect errors such as improper gating, instrument misalignment, or sample contamination. It provides immediate feedback to the operator, allowing timely corrective actions to be taken.
- Time and Cost Efficiency: With real-time tracking and inspection, potential issues can be detected early, avoiding costly reagents, sample wastage, and repeat experiments. The model also reduces the time required for manual inspection and troubleshooting.
- Standardization: By enforcing standard protocols and procedures, the model ensures consistency and comparability across different flow cytometry experiments. This is particularly beneficial in multi-center studies or when comparing data from different research groups.
- Data Integrity: The real-time tracking and inspection model enhances data integrity by minimizing the impact of human errors or deviations from the established protocols. This is especially important for studies involving large datasets or complex analyses.
Conclusion
The real-time tracking and inspection model for flow cytometry have significantly improved the quality control process in this field. By utilizing computer vision and machine learning technologies, it provides real-time monitoring, error detection, and feedback to operators, resulting in more reliable and accurate flow cytometry data. Incorporating this model in flow cytometry procedures ensures adherence to quality standards and enhances the overall efficiency of the analysis process.
Comments:
Thank you all for reading my article on enhancing quality control in flow cytometry with ChatGPT! I'm excited to hear your thoughts and answer any questions you may have.
Great article, Sameer! Flow cytometry is such an important technique in biomedical research, and using ChatGPT to enhance quality control seems like a fantastic idea.
I've been working with flow cytometry for a few years now, and I'm intrigued by the idea of using ChatGPT to improve quality control. Sameer, can you elaborate on the specific aspects of flow cytometry where ChatGPT can be helpful?
Sure, Mark! ChatGPT can assist in various quality control aspects, such as data preprocessing, identification of anomalies, gating strategy optimization, and automated reporting. Its natural language processing capabilities make it versatile for analyzing and interpreting flow cytometry data.
As a researcher who frequently deals with flow cytometry data, I'm excited about the potential of ChatGPT. Sameer, have you tested this approach in a real research setting?
Yes, Natalie! We conducted a pilot study in collaboration with an immunology lab, where we incorporated ChatGPT into their flow cytometry workflow. The results were promising, showing improved accuracy and efficiency in quality control processes.
Thank you, Sameer, for sharing your expertise and patiently answering our questions. Your work on ChatGPT in flow cytometry is groundbreaking.
This sounds fascinating, Sameer! What are the potential limitations or challenges of using ChatGPT for quality control in flow cytometry?
Good question, Lisa! ChatGPT heavily relies on the training data it receives, so it's important to ensure the quality and diversity of the data it learns from. Additionally, the interpretability of its decisions can be a challenge, especially when handling complex flow cytometry datasets.
I can see how ChatGPT can be useful for beginners in flow cytometry, but what about experienced users? Is it still beneficial for them?
Absolutely, Alex! Even experienced users can benefit from ChatGPT's assistance. It can help streamline their workflow, provide alternative analysis approaches, and reduce human error in quality control processes.
I have a concern regarding data privacy. Sameer, how does ChatGPT handle sensitive flow cytometry data?
Good point, Rita! Data privacy is crucial. ChatGPT does not store any user data or personally identifiable information. It operates in a secure and confidential manner, ensuring the privacy of flow cytometry data.
Do you think ChatGPT can completely replace manual quality control efforts in flow cytometry, Sameer?
Not completely, James. ChatGPT is designed as a tool to assist in quality control, augmenting human expertise rather than replacing it. It can save time and improve efficiency, but manual verification and judgment remain important.
What are the computing requirements for using ChatGPT in flow cytometry, Sameer?
Great question, Pauline! ChatGPT can be run on a standard computer, although more complex flow cytometry datasets may benefit from more powerful hardware to handle the computation efficiently.
Thank you, Sameer! Knowing the computing requirements helps in planning the resources needed when integrating ChatGPT into flow cytometry workflows.
I'm curious about the implementation process of integrating ChatGPT into a flow cytometry workflow. Sameer, can you provide some insights on that?
Certainly, Jennifer! Integrating ChatGPT involves training the model on flow cytometry data, creating a user-friendly interface for interactions, and integrating its outputs into the existing workflow. It's a collaborative process involving scientists, developers, and domain experts.
Are there any plans to develop a commercial product based on this idea, Sameer?
Yes, Emma! Based on the positive results of our pilot study, we are exploring the development of a commercial product to make ChatGPT more widely accessible to the flow cytometry community.
I agree, Sameer! AI technologies like ChatGPT have the power to transform scientific research by enabling efficient and accurate analyses.
Thanks for sharing the insights, Sameer! Collaborative integration of ChatGPT into the workflow sounds like a challenge worth undertaking for flow cytometry labs.
I can see how ChatGPT can be a valuable tool in flow cytometry, but are there any other potential applications for this technology in similar domains?
Absolutely, Susan! ChatGPT's capabilities extend beyond flow cytometry. It can be applied to other data-intensive domains like genomics, proteomics, and even clinical diagnostics, where data analysis and quality control are critical.
This article has piqued my interest! Sameer, what are some open challenges in the field of flow cytometry that ChatGPT can potentially address?
Glad to hear that, Daniel! ChatGPT can potentially address challenges such as improving reproducibility and standardization in flow cytometry assays, assisting in rare event detection, and aiding in the identification of data artifacts or technical issues.
It's fascinating how AI can be leveraged in research techniques like flow cytometry. Sameer, do you have any plans for further research in this area?
Definitely, Rachel! There's much more to explore. We plan to investigate advanced techniques to enhance ChatGPT's understanding of flow cytometry data, as well as collaborate with more research labs to validate its performance across diverse applications.
I've thoroughly enjoyed the article, Sameer! Do you have any recommended resources for someone who wants to learn more about flow cytometry and its applications?
Thank you, Jake! There are some excellent resources available, such as 'Introduction to Flow Cytometry' by Shapiro, 'Practical Flow Cytometry' by Howard Shapiro, and the website of the International Society for Advancement of Cytometry (ISAC). These can provide a solid foundation for understanding flow cytometry.
I wonder if ChatGPT can be used in real-time analysis during flow cytometry experiments. Sameer, do you have any insights on that?
That's an interesting possibility, Sophia! While real-time analysis is not the primary focus at the moment, with further advancements, it may be feasible to integrate ChatGPT into the flow cytometry workflow to provide immediate quality control feedback as experiments progress.
Sameer, I'm impressed by the potential of ChatGPT in flow cytometry. Are there any plans to make the underlying code or model open-source?
Thank you, Emily! We are actively considering releasing certain components of the code or models to the community. Open-sourcing can foster collaboration, enable improvements, and address concerns related to transparency and reproducibility.
I'm glad to see the potential of ChatGPT in flow cytometry, Sameer! AI's role in streamlining scientific research processes is only going to grow in the future.
What kind of computational resources are required to train ChatGPT on flow cytometry data, Sameer?
Training ChatGPT on flow cytometry data typically requires a high-performance computing environment with sufficient GPU resources. The exact specifications depend on the size and complexity of the dataset, but it can be computationally intensive.
I'm curious about the potential impact of ChatGPT on the field of flow cytometry. Sameer, how do you foresee this technology evolving in the future?
Great question, Linda! In the future, I believe ChatGPT and similar AI technologies will become integral parts of flow cytometry workflows. They will contribute to improved data analysis, quality control, and decision-making processes, ultimately enhancing research outcomes.
I couldn't agree more, Sameer! The integration of AI technologies into flow cytometry workflows will undoubtedly enhance research outcomes.
This article has given me a new perspective on applying AI to flow cytometry. Sameer, what are some of the specific challenges you faced while developing ChatGPT for this domain?
I'm glad you found it insightful, Oliver! The challenges included obtaining sufficient labeled data for training, handling the multidimensionality and complexity of flow cytometry data, and designing an interactive interface that aligns well with the needs of flow cytometry experts.
Are there any limitations on data size or the number of parameters that ChatGPT can handle effectively in flow cytometry analysis, Sameer?
Indeed, Ethan! The effectiveness of ChatGPT in flow cytometry analysis can be influenced by the size of the dataset and the number of parameters, as more extensive datasets and complex parameter combinations may require additional fine-tuning or computational resources.
Thanks for clarifying, Sameer! Considering the data size and parameter complexities is crucial when applying AI solutions like ChatGPT.
I'm excited about the potential of ChatGPT in flow cytometry! Sameer, do you think AI will play a more significant role in streamlining scientific research processes in the future?
Absolutely, Michelle! AI has the potential to revolutionize scientific research by automating repetitive tasks, accelerating data analysis, and providing new insights. It will empower researchers and enable breakthroughs across various domains.
This article presents an exciting application of AI in flow cytometry. Sameer, what are some key factors to consider when implementing ChatGPT in a research lab setting?
Great question, Nathan! Implementing ChatGPT in a research lab requires careful consideration of factors such as user training, integration with existing tools, validation against domain-specific standards, and addressing potential challenges related to data privacy and reproducibility.
The potential of ChatGPT in flow cytometry is intriguing, Sameer. Do you have any suggestions on how to convince researchers to adopt this technology?
Thank you, Caroline! To encourage researchers to adopt ChatGPT, it's essential to demonstrate its usability, positive impact on research outcomes, and provide case studies or pilot study results showing its effectiveness in real-world flow cytometry applications.
As a Flow Cytometry lab manager, I'm excited about the potential benefits of ChatGPT. Sameer, what is the approximate timeline for the commercial product release?
Great to hear your excitement, Julian! While I can't provide an exact timeline at the moment, we are actively working towards a commercial release and expect it to be available within the next year.
That's fantastic, Sameer! I'll be eagerly awaiting the release of the commercial product. Flow cytometry labs can greatly benefit from ChatGPT.
Looking forward to it, Sameer! ChatGPT has the potential to be a game-changer in flow cytometry quality control.
Thank you, Sameer! I'll eagerly await the commercial release. ChatGPT has the potential to simplify and improve flow cytometry QC.
Thank you, Sameer! I'll eagerly await the commercial release. ChatGPT has the potential to simplify and improve flow cytometry QC.
Looking forward to the commercial release, Sameer! ChatGPT can truly revolutionize flow cytometry QC.
I'm excited about the potential benefits of ChatGPT in flow cytometry QC, Sameer! Looking forward to the commercial release.
This article raises some interesting possibilities! Sameer, what are the ethical considerations when using AI like ChatGPT in flow cytometry?
Ethical considerations are indeed important, Melissa! It's crucial to ensure data privacy, address biases in AI models, maintain transparency, and mitigate any potential negative impact on human decision-making or professional expertise.
Thank you all for the engaging discussion and thought-provoking questions! Your feedback and insights are immensely valuable in shaping the future of ChatGPT in flow cytometry.
Excellent article, Sameer! I'm excited to see how ChatGPT can revolutionize quality control in flow cytometry.
I completely agree, Sameer! ChatGPT can offer experienced users valuable insights and suggest alternative analysis approaches we might not have considered.
Thanks for the clarification, Sameer! It's great to know that ChatGPT's hardware requirements are manageable for most labs.
Exactly, Paul! ChatGPT's accessible computing requirements make it a feasible solution for many flow cytometry labs.
Thanks for the information, Pauline! It's good to know that ChatGPT's hardware requirements are manageable for most research labs.
As an experienced flow cytometry user, I can definitely see the benefits of incorporating ChatGPT into my workflow. It could save a lot of time and improve accuracy.
I'm looking forward to the further development of ChatGPT in flow cytometry. It has the potential to greatly enhance data analysis and decision-making.
Real-time analysis with ChatGPT during flow cytometry experiments sounds exciting! It could provide immediate feedback and help troubleshoot any issues.
Implementing ChatGPT in a research lab seems to require a multidisciplinary approach. Collaboration between scientists and developers is essential for a successful integration.
The impact of AI on scientific research is undeniable. It's exciting to see how ChatGPT and similar technologies will shape the future of flow cytometry.
Case studies and real-world examples of ChatGPT's benefits in flow cytometry will definitely help convince researchers to adopt this technology.
It's good to know that ChatGPT can be used on standard computer hardware. This makes it more accessible for labs with limited computational resources.
Real-time quality control feedback during experiments would be a game-changer. It could save a lot of time and prevent costly mistakes.
Considering factors like user training, validation, and addressing challenges is essential for successful implementation of ChatGPT in a research lab.
Demonstrating ChatGPT's usability and positive impact through case studies would definitely help researchers embrace this technology.
Collaboration between different stakeholders is crucial for integrating ChatGPT smoothly into flow cytometry workflows.
Ensuring data privacy and addressing biases are indeed important considerations when using AI in domains like flow cytometry.
Training ChatGPT on flow cytometry data sounds computationally demanding. Having access to powerful GPU resources will likely be crucial.
Considering factors like user training, integration, and validation is essential when implementing ChatGPT in a research lab.
Real-world case studies and results will play a crucial role in convincing researchers about the benefits of integrating ChatGPT into their flow cytometry workflow.
Addressing ethically relevant aspects, such as data privacy, transparency, and maintaining human expertise, is crucial when integrating AI like ChatGPT into flow cytometry.
Open-sourcing certain components or models of ChatGPT could accelerate advancements, foster collaboration, and address concerns related to transparency and reproducibility.
Addressing ethical aspects and ensuring unbiased and transparent decision-making are crucial when using AI like ChatGPT in flow cytometry.
Thank you all for the engaging discussion and your enthusiasm! I'm truly excited to witness the positive impact of ChatGPT on flow cytometry quality control and beyond.
Thank you, everyone, for your engaging comments and insightful questions! Your enthusiasm for ChatGPT in flow cytometry is truly inspiring.
It has been a pleasure discussing ChatGPT in flow cytometry with all of you. Your feedback and discussions have been immensely valuable. Thank you, and happy researching!