Enhancing Quality Control in FPLC Technology: Leveraging ChatGPT for Improved Efficiency and Accuracy
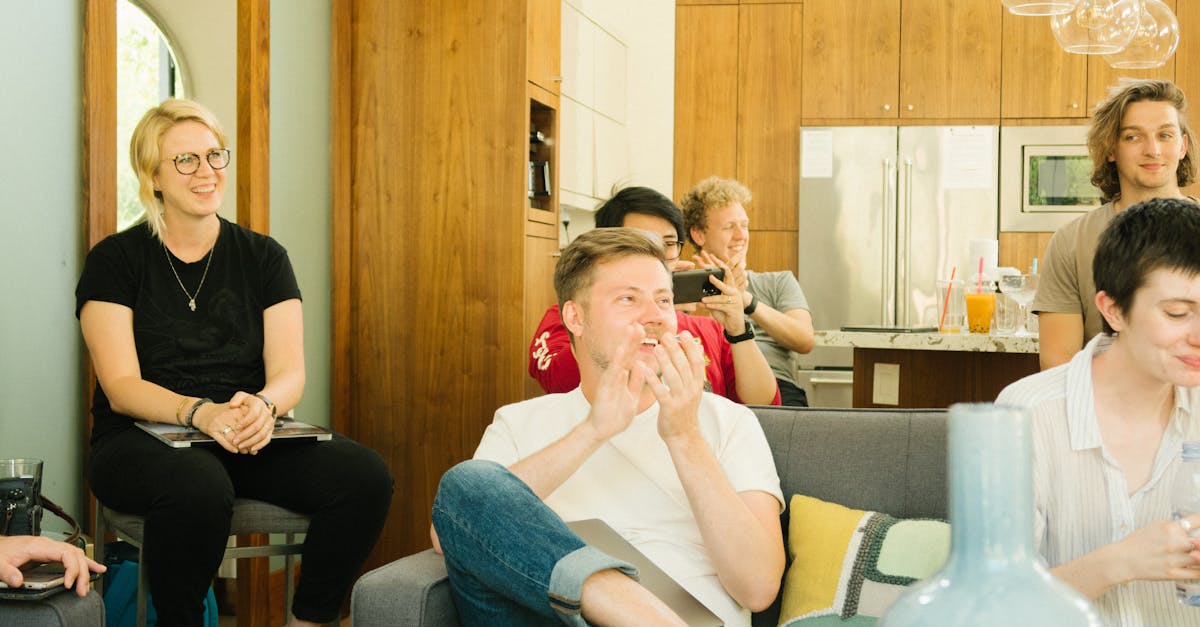
The fast protein liquid chromatography (FPLC) technology plays a crucial role in the field of quality control. It assists in ensuring the consistency and quality of processed samples, providing valuable data for quality assurance.
Introduction to FPLC
Fast protein liquid chromatography, commonly abbreviated as FPLC, is a highly efficient chromatographic technique used for protein analysis and purification. It utilizes gravity flow or low-pressure liquid chromatography combined with high-performance techniques to achieve rapid and precise separations.
The Role of FPLC in Quality Control
In the area of quality control, FPLC plays a crucial role in ensuring that processed samples meet the required standards consistently. It provides assistance in determining the consistency of protein fractions, ensuring the absence of impurities, and validating the integrity of purified samples.
Consistency Checks
FPLC facilitates the evaluation of consistency by analyzing the protein elution profiles in processed samples. By monitoring the elution patterns, any inconsistencies or variations in the protein fractions can be detected. This information enables the identification of potential deviations in the manufacturing process, ensuring consistent quality of the final product.
Impurity Detection
Through FPLC, impurities in the processed samples can be identified and quantified. The technology utilizes various methods such as size-exclusion chromatography, ion-exchange chromatography, and affinity chromatography to separate different components within the sample. By comparing the obtained chromatograms with established purity criteria, any impurities or contaminants present can be identified and quantified accurately.
Integrity Validation
FPLC plays a vital role in validating the integrity of purified protein samples. By employing methods such as molecular weight determination and assessment of peak symmetry, FPLC ensures the absence of degradation or denaturation during purification. This validation process guarantees that the purified proteins retain their native structure and biological activity, making them suitable for further research or production purposes.
Benefits and Limitations
The utilization of FPLC in quality control offers several benefits. Firstly, it enables real-time monitoring, allowing immediate detection and resolution of any inconsistencies or impurities during the production process. This ensures that only high-quality samples reach the market.
Additionally, FPLC provides accurate and reliable data, ensuring confidence in product quality. The technology allows for the analysis of multiple samples simultaneously, enhancing the efficiency of quality control processes. Moreover, FPLC can be automated, reducing human error and increasing productivity.
However, like any technology, FPLC has its limitations. It requires skilled personnel to operate and interpret the obtained results accurately. Furthermore, the cost of the equipment and consumables may be a barrier to small-scale operations. Diligent maintenance is also essential to ensure the reliability and longevity of the FPLC system.
Conclusion
FPLC technology plays a vital role in quality control, ensuring the consistency and quality of processed samples. By providing assistance in checking for consistency, detecting impurities, and validating the integrity of purified samples, FPLC enables companies to meet the required standards and deliver high-quality products to the market.
Despite its limitations, FPLC offers numerous benefits, including real-time monitoring, accurate data acquisition, and increased efficiency. As technology advances and becomes more accessible, FPLC is expected to continue its significant impact in quality control and further improve the overall quality assurance processes in various industries.
Comments:
Thank you for taking the time to read my article! I'm excited to hear your thoughts and engage in a discussion about improving quality control in FPLC technology.
Great article, Kyle! FPLC technology plays a crucial role in many industries. How do you think leveraging ChatGPT can enhance efficiency and accuracy?
Thanks, Emma! By leveraging ChatGPT, we can automate certain quality control processes and improve decision-making. ChatGPT can analyze data, detect anomalies, and provide real-time insights, saving time and reducing manual errors.
I have some concerns about relying too heavily on AI for quality control. What if the ChatGPT model misinterprets data or makes inaccurate judgments?
Valid point, Michael. While ChatGPT can bring efficiency, it's crucial to remember that it's a tool and not a replacement for human judgment. Close monitoring, a well-trained model, and regular human reviews can address potential misinterpretations or inaccuracies.
I'm curious about the implementation process. How easy is it to integrate ChatGPT into existing FPLC systems?
Good question, Sophia. Integrating ChatGPT into existing systems involves data preparation, fine-tuning the model based on specific requirements, and setting up the necessary interfaces for communication. It can vary in complexity, but there are frameworks and guides available to facilitate the process.
I'm hesitant about the potential cost of implementing ChatGPT for quality control. Can it be cost-effective for smaller businesses?
Excellent point, David. Cost is a crucial consideration. While implementing ChatGPT may require resources initially, it can lead to long-term cost savings by reducing manual labor and improving efficiency. There are also variations of the model that offer more cost-effective options.
I'm skeptical about the accuracy of AI models. How can we ensure that ChatGPT provides reliable judgments?
Valid concern, Oliver. The accuracy of ChatGPT can be improved through careful training, quality assurance steps, and continuous evaluations. By combining human expertise and AI capabilities, we can enhance reliability and ensure high-quality judgments.
What are the limitations of leveraging ChatGPT for quality control? Are there any scenarios where it may not be suitable?
Good question, Isabella. ChatGPT can be limited by the quality of training data and may struggle with unfamiliar or complex scenarios. Moreover, it may not always capture unique edge cases that human judgment can. A combination of AI and human oversight can address these limitations.
I'm interested in the potential impact of ChatGPT on workforce roles. Do you foresee any changes in job responsibilities due to its implementation?
Great question, Sophie. The implementation of ChatGPT can shift job responsibilities towards more strategic decision-making and collaboration with AI systems. Human experts can focus on reviewing, optimizing processes, and interpreting complex cases, leveraging the insights provided by ChatGPT.
Kyle, what do you think the future holds for ChatGPT and its impact on quality control in various industries?
Emma, I believe ChatGPT and similar AI technologies will continue to play a significant role in enhancing quality control across industries. As models evolve and are fine-tuned, they will become more accurate, efficient, and adaptable to different use cases. However, human oversight and expertise will remain vital.
Are there any ethical considerations when it comes to using AI like ChatGPT for quality control?
Ethical considerations are crucial, Alice. Transparency, bias detection, and fairness are important aspects to address. We must ensure clear guidelines, safeguards, and accountability in the AI implementation to avoid unintended consequences and ensure ethical decision-making.
I'm wondering if ChatGPT can be used to optimize different stages of FPLC technology. Can it go beyond quality control?
Indeed, George. ChatGPT can be leveraged beyond quality control. It can assist in data analysis, process optimization, predictive maintenance, and workflow management. By exploring its potential, we can enhance different aspects of FPLC technology.
How does ChatGPT handle uncertainty or ambiguous situations in quality control tasks?
Good question, Michael. ChatGPT can be trained on a diverse range of data to detect and handle uncertainties. By providing confidence intervals, it can indicate when certain judgments have higher degrees of uncertainty. Human experts can then review such cases for further clarification and ensure accurate decisions.
What are the key challenges in implementing ChatGPT for quality control, and how can they be overcome?
Sophia, one key challenge is obtaining high-quality training data that is representative of real-world scenarios. Additionally, addressing biases and potential errors during training is crucial. Regular model evaluations, feedback loops, and continuous improvement are essential to overcome these challenges and achieve reliable quality control.
I'm interested in the potential collaboration between ChatGPT and human experts. How can they work together effectively?
Collaboration is key, David. Human experts can provide training data, fine-tune the model, and offer continuous guidance and oversight. By combining AI capabilities with human expertise, we can take advantage of automation while ensuring the human touch in complex decision-making and handling exceptional cases.
What are the potential risks and drawbacks of implementing ChatGPT for quality control?
Great question, Oliver. Potential risks include over-reliance on AI, lack of interpretability in complex decisions, and privacy considerations with sensitive data. Drawbacks can include model biases, limitations in exceptional case handling, and the need for continuous training and updates. Addressing these risks and drawbacks should be a priority during implementation.
Kyle, do you have any examples of successful ChatGPT implementations for quality control?
Emma, there have been successful ChatGPT implementations across industries. In healthcare, it has aided in diagnosing medical conditions, while in manufacturing, it has improved defect detection. Some companies have reported significant improvements in efficiency and accuracy by integrating ChatGPT into their quality control processes.
How can we ensure the long-term reliability of AI models like ChatGPT for quality control?
Ensuring long-term reliability involves continuous monitoring, updating the model with new data, and incorporating feedback from human experts and end-users. Regular performance evaluations, addressing potential biases, and staying updated with advancements in AI research are crucial in maintaining the reliability of ChatGPT for quality control.
Could ChatGPT also assist in documenting quality control processes and generating reports?
Certainly, George! ChatGPT can assist in documenting quality control processes by extracting relevant information from data, generating reports and summaries, and providing insights on process optimizations. It can automate tasks that require analysis and documentation, saving time and ensuring accurate records.
What are the required computational resources for implementing ChatGPT for quality control?
Isabella, the required computational resources can vary depending on the scale of the implementation, the volume of data, and the complexity of the tasks. While powerful hardware like GPUs can speed up the training and inference process, there are also options to leverage cloud services and distributed computing to manage resource requirements.
Are there any privacy concerns associated with implementing ChatGPT for quality control?
Privacy is indeed a concern, Sophie. It is essential to handle data with proper security measures, ensuring compliance with relevant privacy regulations. Anonymization, permissions management, and strict access controls should be in place to protect sensitive information when utilizing ChatGPT for quality control.
How do you see the adoption of ChatGPT in quality control evolving over the next few years?
Oliver, I believe the adoption of ChatGPT in quality control will increase steadily. As the technology matures, more success stories emerge, and awareness grows, businesses will recognize the potential benefits it offers. Continued research in AI ethics, interpretability, and addressing limitations will further drive its adoption over the next few years.
What steps should companies take to implement ChatGPT for quality control while minimizing potential risks and maximizing benefits?
Emma, companies should start with small-scale pilots to evaluate the feasibility and best practices. They should establish clear guidelines, train the model on relevant data, and involve domain experts throughout the process. Regular monitoring, open channels for feedback, and continuous improvement are vital to minimize risks and maximize the benefits of implementing ChatGPT for quality control.
Looking ahead, what role do you envision human experts playing in quality control alongside AI technologies like ChatGPT?
Michael, human experts will remain crucial in quality control. They will provide domain expertise, handle complex cases, ensure ethical considerations, and review and validate AI-generated insights. Their role will evolve towards strategic decision-making, optimizing processes, and collaborating effectively with AI technologies like ChatGPT to achieve the best outcomes.
What are the potential challenges in training the ChatGPT model for quality control, and how can they be addressed?
Isabella, training the ChatGPT model can be challenging due to the need for high-quality labeled data and the risk of biases in training sets. To address these challenges, diverse and representative training data should be used, bias detection mechanisms should be implemented, and continuous evaluations of the model's performance should be conducted to mitigate potential challenges in training for quality control.
What level of technical expertise is required to implement ChatGPT for quality control?
Sophie, implementing ChatGPT for quality control requires a certain level of technical expertise. Knowledge of machine learning, natural language processing, and data preparation is essential. However, organizations can leverage external experts, collaborate with AI solution providers, or invest in upskilling their teams to bridge any expertise gaps and ensure successful implementation.
Are there any regulations or standardization efforts in place regarding the use of AI technologies like ChatGPT for quality control?
David, regulations and standardization efforts are actively advancing in response to the growing use of AI technologies. For example, in the European Union, the General Data Protection Regulation (GDPR) and the EU AI Act are vital in governing AI usage. Collaborative efforts between policymakers, industry experts, and organizations are crucial to strike the right balance and ensure responsible AI usage in quality control.