Enhancing Quality Control in Manufacturing Operations: Leveraging ChatGPT Technology
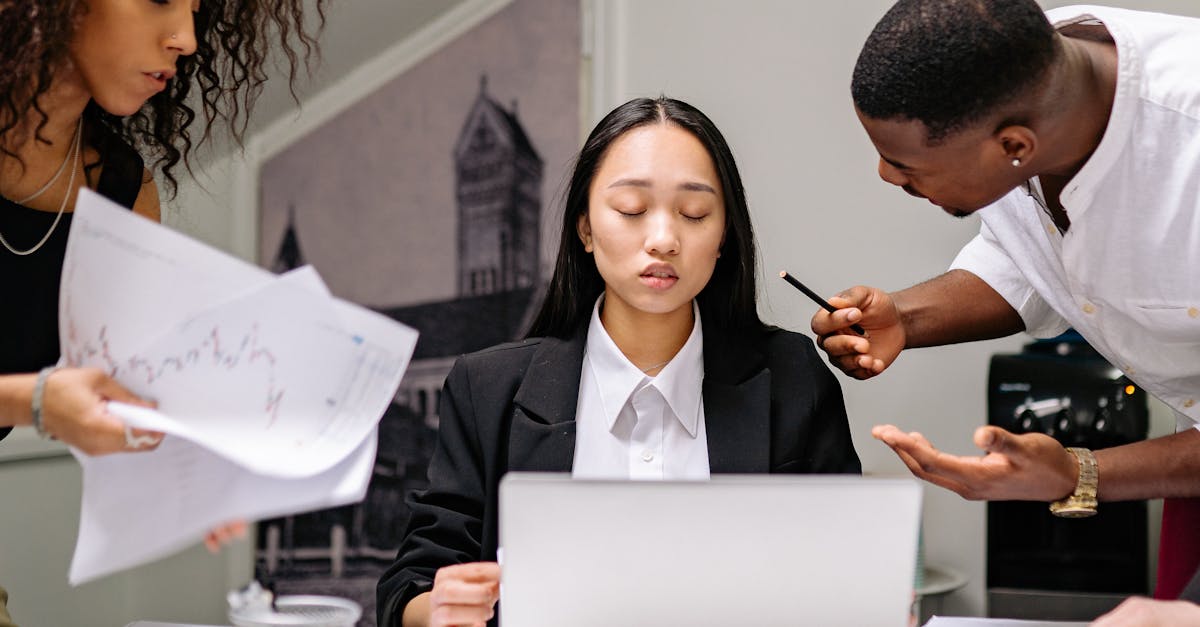
The field of manufacturing operations has greatly benefited from advancements in technology. One such technology that has revolutionized quality control procedures is data analysis. By utilizing advanced algorithms and statistical analysis, manufacturers can now analyze product quality data to predict potential quality issues faster and more accurately than ever before.
Quality control is an essential aspect of manufacturing operations. It ensures that the end products manufactured meet the required specifications and adhere to the defined standards. By identifying and resolving quality issues early in the production process, manufacturers can minimize waste, reduce customer complaints, and improve overall profitability.
Traditionally, quality control in manufacturing relied on manual inspections and random sampling techniques. While these methods are still useful, they have their limitations. Human error, subjectivity, and the inability to analyze vast amounts of data in a timely manner are common challenges faced by manufacturers.
With the advent of technology, manufacturers have access to sophisticated software tools that can automate the quality control process and provide real-time analysis of product quality data. These tools can collect data from various sources, such as sensors, testing equipment, and production systems, and consolidate it into a centralized database.
Once the data is collected, advanced algorithms and statistical models can be applied to identify patterns, trends, and anomalies in the data. By analyzing historical data and comparing it with real-time data, manufacturers can predict potential quality issues and take preemptive actions to prevent them from occurring.
Machine learning techniques can be employed to build predictive models that can learn from past quality issues and accurately predict future ones. By continuously feeding new data into these models, manufacturers can refine and improve their predictive capabilities over time.
Manufacturers can also leverage data analysis to uncover root causes of quality issues. By analyzing the correlations and dependencies between various process parameters and quality metrics, manufacturers can identify the factors that significantly impact product quality. This knowledge can then be used to optimize production processes, reduce defects, and enhance overall product quality.
In addition to predictive analysis, data analysis can also provide manufacturers with real-time monitoring capabilities. By setting up automated alerts and notifications based on predefined quality thresholds, manufacturers can take immediate corrective actions when deviations from the desired quality levels occur.
Furthermore, data analysis can facilitate data-driven decision making in quality control. By providing manufacturers with actionable insights and visualizations, manufacturers can make informed decisions based on objective data rather than subjective opinions.
- Improved quality control procedures
- Early detection of potential quality issues
- Reduction in customer complaints
- Optimization of production processes
- Enhanced product quality
- Real-time monitoring and alerts
- Data-driven decision making
In conclusion, the use of data analysis technology in the area of quality control in manufacturing operations offers significant advantages. It enables manufacturers to analyze product quality data to predict potential quality issues faster and more accurately. By leveraging advanced algorithms, statistical analysis, and machine learning techniques, manufacturers can minimize waste, reduce defects, optimize production processes, and enhance overall product quality. With data-driven decision making and real-time monitoring capabilities, manufacturers can stay on top of quality control and ensure customer satisfaction.
Comments:
Thank you all for joining the discussion on my blog article titled 'Enhancing Quality Control in Manufacturing Operations: Leveraging ChatGPT Technology'. I'm excited to engage in this conversation and hear your thoughts!
As an engineer in the manufacturing industry, I find the concept of leveraging chatbot technology for quality control intriguing. It has the potential to streamline processes and improve efficiency. However, I'm concerned about the accuracy and reliability of the AI system. Has anyone here experienced using chatbot technology in a manufacturing setting?
Hi Adam, I've actually implemented a chatbot system for quality control in my company. Initially, there were some challenges with accuracy, but with continuous fine-tuning and training, it has become quite reliable. We still need human intervention for complex issues, but overall, the chatbot has improved our operations.
Adam, I share your concerns regarding the accuracy of AI systems in manufacturing. While they can be helpful, I believe it's essential to have human oversight and expertise. The chatbot technology could be a valuable assistant, but ultimately, decisions should be made by professionals who can evaluate all factors.
I'm intrigued by the idea of using chatbots for quality control, but I have reservations about the level of customization and flexibility they offer. How adaptable are these chatbot systems to different manufacturing environments?
Sarah, chatbot systems can be customized to fit specific manufacturing environments. You can train them with data specific to your processes and quality control requirements. Of course, there might still be limits to their adaptability, but with proper configuration, they can be quite flexible and effective.
While chatbot technology seems promising, I wonder if it could potentially disrupt the existing workforce, leading to job losses. What are your thoughts on this aspect of implementing chatbot systems in manufacturing operations?
Frank, I understand your concern. However, instead of eliminating jobs, chatbots can complement human workers. They can assist in repetitive and time-consuming tasks, freeing up employees to focus on more complex and critical aspects. It's all about finding the right balance between automation and human expertise.
As a quality control manager, I believe that leveraging chatbot technology could potentially lead to faster identification of defects and more efficient resolution. It could greatly impact the overall quality management process. I'd love to hear from professionals who have successfully integrated chatbots into their daily operations.
Linda, in my experience, chatbots have significantly improved our defect tracking and resolution process. They enable real-time analysis and identification of potential issues, allowing us to take proactive measures. The key is to integrate the chatbot seamlessly into existing quality control workflows.
I agree with you, Linda. We've implemented chatbots for quality control in our manufacturing operations, and it has been a game-changer. It not only enhances efficiency but also helps us identify patterns and trends that would be challenging to spot manually. It's definitely worth exploring!
Although the use of chatbot technology in quality control is intriguing, I wonder about the potential security risks. Manufacturing operations often involve sensitive data and intellectual property. How can we ensure the protection of such information when utilizing chatbots?
Mark, security is indeed a critical aspect to consider. When implementing chatbot technology, it's vital to have rigorous access controls, encryption mechanisms, and regular vulnerability assessments. Additionally, ensuring that data is stored on secure servers and applying necessary security protocols can help mitigate risks.
Mark, you raise a valid concern. It's crucial to partner with reputable chatbot providers who have robust security measures in place. Conducting thorough due diligence and implementing proactive security practices will help safeguard sensitive manufacturing data.
I'm interested in learning about the potential cost savings when leveraging chatbot technology for quality control. Can anyone share their experiences regarding the financial benefits of implementing chatbots in manufacturing operations?
Mary, while the cost savings may vary depending on the scale and complexity of your operations, chatbots can contribute to overall efficiency gains. By automating certain quality control tasks, reducing errors, and improving defect resolution time, they can help optimize costs in the long run.
Mary, in my experience, chatbots have proven to be cost-effective. They reduce the need for manual intervention in routine tasks, saving both time and resources. However, it's important to consider the implementation and maintenance costs when evaluating the financial benefits.
I'm curious about the learning curve associated with adopting chatbot technology. How long does it typically take for employees to adapt and fully utilize the system effectively?
Brian, the learning curve for employees can vary depending on the complexity of the chatbot system and the level of training provided. However, with a user-friendly interface, clear instructions, and accessible support, employees can quickly adapt within a few weeks of regular usage.
I appreciate the potential of chatbot technology for quality control, but I also believe it's crucial to maintain a human touch. The expertise and problem-solving ability that humans bring to the table cannot be replaced entirely by AI. Finding the right balance is key.
Claire, you're absolutely right. AI should be seen as an assistant rather than a replacement. By leveraging chatbot technology, we can enhance human decision-making and problem-solving capabilities, leading to improved quality control outcomes.
I'm curious to know more about the scalability of chatbot systems in manufacturing. Can they handle large volumes of data and complex quality control processes effectively?
Oliver, chatbot systems can certainly handle large volumes of data and complex processes. However, it's essential to design and train them accordingly. By distributing data processing and utilizing scalable infrastructure, chatbots can effectively handle the demands of manufacturing quality control.
One potential concern I have is the language and communication capabilities of chatbots. Do they have the flexibility to understand and adapt to different terminologies and communication styles used in manufacturing operations?
Grace, language and communication capabilities are indeed crucial for chatbots in manufacturing. Ideally, the chatbot system should be trained and configured to understand the manufacturing terminologies used within your organization. Customization allows for better communication and more accurate responses.
Are there any potential ethical concerns to consider when leveraging chatbot technology in manufacturing quality control?
Benjamin, the use of chatbot technology does raise some ethical considerations. Some potential concerns include data privacy, bias in decision-making algorithms, and the impact on workers' rights. It's important to address these issues proactively by implementing appropriate safeguards and ethical guidelines.
In addition to its potential benefits, I'm curious about the limitations of chatbot technology in the context of manufacturing. What are some of the challenges that companies may face when implementing chatbots?
Alicia, some challenges include the need for continuous training and improvement of the chatbot system, ensuring data accuracy and integrity, maintaining user trust, and managing integration with existing systems. Overcoming these challenges requires proper planning, monitoring, and ongoing optimization.
How does leveraging chatbot technology impact the overall customer experience and satisfaction in manufacturing operations?
Paul, when implemented effectively, chatbot technology can enhance the customer experience in manufacturing. By providing real-time support, quick responses to queries, and efficient issue resolution, it contributes to overall customer satisfaction. However, human support should also be available for complex customer needs.
I'm wondering if there have been any studies or research conducted to quantify the impact of chatbot technology on quality control in manufacturing?
Mike, there have been studies and research efforts in this area. Several case studies and industry reports have shown positive impacts on quality control processes, such as improved defect identification rates, faster issue resolution, and enhanced efficiency. These findings support the value of leveraging chatbot technology.
It's fascinating to see the evolving role of AI in quality control. However, it's important not to overlook the importance of continuous improvement and human expertise. AI is a tool that should complement and empower human decision-making, rather than replace it.
Claire, I couldn't agree more. The goal is to leverage AI to augment human capabilities and enable more effective decision-making and problem-solving. By combining the strengths of both humans and AI, we can drive significant improvements in quality control processes.
I'm concerned that chatbots may lack context understanding and may not be able to handle the complexity of certain manufacturing processes. How can we ensure that chatbots effectively fulfill their role in quality control?
Thomas, ensuring that chatbots effectively fulfill their role requires careful training and continuous fine-tuning. By incorporating contextual information, providing access to relevant data sources, and enabling clear escalation paths for complex issues, we can enhance their ability to handle the unique challenges of manufacturing processes.
Considering the potential benefits and challenges, what would be the recommended approach for organizations looking to implement chatbots in their manufacturing quality control?
Anna, a recommended approach would involve conducting a thorough assessment of quality control processes, identifying areas where chatbots can add value, and setting clear objectives. Partnering with experienced chatbot providers, investing in training and support, and ensuring continuous improvement will contribute to successful implementation.
Thank you all for your valuable insights and contributions to the discussion. It's evident that chatbot technology has the potential to significantly enhance quality control in manufacturing operations. While challenges exist, proper planning, training, and collaboration can ensure successful implementation. Let's keep embracing technology for continuous improvement!