Enhancing Quality Control in Timber Technology: Harnessing the Power of ChatGPT
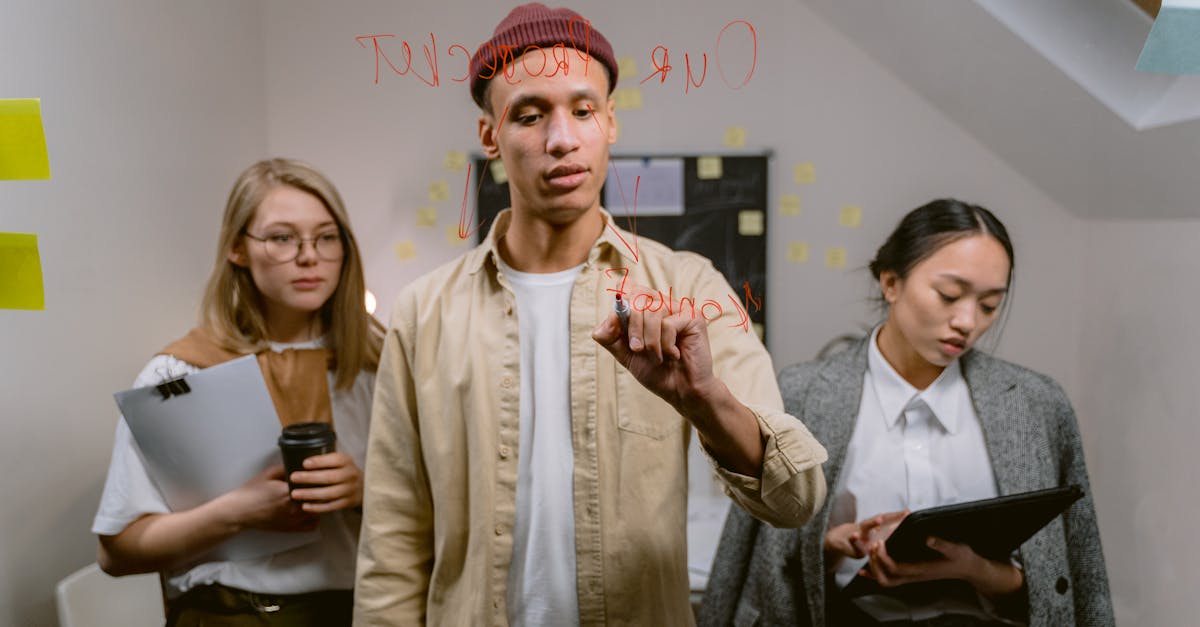
Timber is a widely-used material in various industries, including construction, furniture manufacturing, and paper production. However, ensuring the quality of timber can be challenging, as defects can significantly impact the final product's structural integrity and aesthetic appeal. With advancements in technology, particularly the development of GPT-4 (Generative Pre-trained Transformer 4), timber quality control has taken a significant leap forward.
The Role of GPT-4 in Timber Quality Control
GPT-4 is an advanced language model powered by artificial intelligence and machine learning algorithms. Its ability to analyze vast amounts of data and generate insightful predictions makes it a valuable tool for timber quality control. By utilizing GPT-4, manufacturers can identify potential defects in timber and take proactive measures to enhance the overall quality of their products.
Traditional methods of timber quality control often rely on manual inspection, which is time-consuming and prone to human error. GPT-4 offers an automated and efficient alternative to this process. By feeding the AI model with existing data on timber defects, it can learn to identify patterns and characteristics associated with various types of defects.
Once trained, GPT-4 can quickly analyze new timber samples and predict potential defects. By flagging potentially defective pieces early in the production process, manufacturers can take corrective actions, such as reprocessing or discarding them, minimizing waste and maintaining high-quality standards.
The Impact on Overall Product Quality
Predicting timber defects through GPT-4's analysis allows manufacturers to improve the overall quality of their products. By identifying potential defects early on, manufacturers can reduce the risk of producing faulty or subpar items. This, in turn, leads to increased customer satisfaction, as consumers receive products that meet their expectations in terms of both functionality and appearance.
Besides enhancing customer satisfaction, GPT-4's usage in timber quality control also helps minimize financial losses for manufacturers. Defective timber can lead to costly rework or even product recalls, negatively impacting a company's bottom line. By leveraging GPT-4 to identify and mitigate defects, manufacturers can save both time and money in the production process.
Challenges and Future Considerations
While GPT-4 presents promising opportunities for improving timber quality control, several challenges need to be addressed. The accuracy of defect predictions heavily relies on the quality and diversity of the training data provided to the AI model. To ensure a robust analysis, manufacturers must invest in collecting and curating extensive datasets that adequately represent different types of timber defects.
Furthermore, the integration of GPT-4 within existing timber quality control processes requires careful planning and consideration. Manufacturers may need to adapt their workflows and train their staff to effectively utilize GPT-4's outputs in decision-making. Additionally, ensuring data privacy and security throughout the AI implementation process is crucial.
Looking ahead, future advancements in AI technology, including improved versions of GPT, hold the potential to enhance the accuracy and efficiency of timber defect analysis. As these models continue to evolve, manufacturers can expect even more advanced capabilities to support their quality control efforts.
Conclusion
GPT-4 has revolutionized timber quality control by providing an automated and efficient means of analyzing and predicting defects. By leveraging the power of artificial intelligence and machine learning, manufacturers can enhance the overall quality of their products, reduce waste, and increase customer satisfaction. While challenges remain, the continued development of AI technology promises even greater progress in timber quality control in the future.
Comments:
Thank you for the informative article! It's great to see how ChatGPT can be utilized in enhancing quality control in timber technology.
I never thought AI could be applied to timber technology. This is fascinating!
@Sarah Thompson, indeed! AI has the potential to revolutionize various industries, including timber technology.
I agree, Sarah! AI has so many applications we never even considered before.
As a timber industry professional, I'm intrigued by the concept. How exactly does ChatGPT improve quality control?
@Mark Johnson, ChatGPT can help in quality control by analyzing large amounts of data, identifying patterns and potential defects, and offering real-time suggestions for improvements.
I'm impressed by the potential of ChatGPT, but what about its accuracy in identifying defects? Is it reliable enough?
@Tina Roberts, that's a valid concern. While ChatGPT is powerful, it still relies on the accuracy of the data it's trained on and might have limitations. However, it can be a valuable tool when combined with human expertise.
Thanks for addressing my concern, Arnie! The collaboration between AI and humans definitely seems like the way forward.
I have reservations about relying too heavily on AI in quality control. Human judgement and intuition can't be replaced entirely.
@Paul Anderson, you're absolutely right. AI is not meant to replace human expertise, but rather to augment it. The combination of AI and human judgement can lead to more efficient and effective quality control processes.
I completely agree, Paul! Human judgement and intuition play a crucial role in quality control.
Absolutely, Brenda! The collaboration between humans and AI systems can lead to more accurate and reliable quality control.
Exactly, Paul! Human expertise and intuition should always play a role in quality control to ensure reliable outcomes.
This technology sounds promising, but what are some potential challenges in implementing ChatGPT for quality control in timber technology?
@Emily Hill, some challenges could include acquiring high-quality training data, ensuring the AI model is properly fine-tuned for the timber industry, and addressing any biases that may arise from the training data.
Wouldn't using AI for quality control require a significant investment in infrastructure and training for employees?
@Simon Young, implementing AI for quality control would involve initial investments in infrastructure and specialized training. However, the long-term benefits can outweigh the costs, leading to improved efficiency and cost savings.
I agree, Simon. Implementing AI for quality control would require a thoughtful approach for successful integration.
Indeed, Michelle! A careful and well-planned integration of AI into quality control processes is crucial for success.
Michelle, you're absolutely right. The successful implementation of AI in quality control requires a thorough understanding of the industry and its specific needs.
I'm concerned about potential job losses due to AI taking over quality control tasks. How can we ensure that employees are not replaced but rather benefit from these advancements?
@Nancy Lewis, that's a valid concern. Companies should focus on reskilling and upskilling employees to work alongside AI systems, creating new job roles that leverage human expertise in conjunction with AI capabilities.
Nancy, I think it's important to focus on how AI can augment human abilities rather than replace humans entirely.
Completely agree, Nancy. Companies should invest in training and upskilling employees to adapt to new roles alongside AI systems.
Nancy, as long as employees are equipped with the necessary skills to work alongside AI, these advancements can lead to increased job satisfaction and growth opportunities.
Are there any real-world examples of companies successfully using ChatGPT for quality control in timber technology?
@Alan Smith, there are a few companies exploring the use of AI, including ChatGPT, in quality control for timber technology. However, it's still an emerging field, and more case studies and practical implementations are needed.
I can see the potential benefits, but how accessible is ChatGPT for small and medium-sized businesses in the timber industry?
@Diana Martin, currently, accessing and implementing ChatGPT can require technical expertise and resources, which might be a barrier for smaller businesses. However, as AI technology advances, we can expect more user-friendly and accessible solutions to emerge.
That's a valid concern, Diana. Accessibility to AI tools should be a priority to ensure the technology's benefits reach businesses of all sizes.
Do you think ChatGPT can be applied to other sectors beyond timber technology?
@Liam Davies, absolutely! ChatGPT's versatility allows it to be useful in various sectors, from manufacturing to healthcare and customer service. The technology has broad potential applications.
While AI can bring many benefits, we must ensure that ethical considerations are prioritized. How can we address potential biases and prevent any unintended consequences in quality control?
@Sophia Adams, you raise a crucial point. To address biases and unintended consequences, it's essential to have diverse and representative training data, rigorous testing, and ongoing monitoring. Ethical guidelines should be developed and followed.
Are there any regulatory frameworks in place to govern the implementation of AI in quality control?
@Benjamin Turner, currently, there might not be specific regulatory frameworks in place for AI in quality control. However, as the technology progresses and becomes more widely adopted, regulatory bodies are likely to develop guidelines to ensure responsible and safe use.
I'm curious about the potential cost savings that ChatGPT can bring to quality control in timber technology. Has there been any research on this?
@Olivia Green, cost savings can arise from increased efficiency and reduced errors in quality control. While specific research on ChatGPT's cost-saving potential in timber technology may be limited, studies on AI applications in other industries have shown promising results.
What implications does the use of AI in quality control have on the sustainability and environmental impact of the timber industry?
@Leo Richardson, AI can contribute to sustainability efforts by helping optimize processes, reducing waste, and improving resource utilization. It has the potential to positively impact the sustainability and environmental footprint of the timber industry.
Great question, Leo! AI-driven optimization can have positive environmental implications, such as minimizing waste and resource utilization.
I agree, Amy! AI-driven optimization can bring tangible benefits to both operational efficiency and environmental sustainability.
Absolutely, Amy! AI has the potential to revolutionize processes, enabling businesses to reduce waste and minimize their ecological footprint.
I can see AI benefiting quality control in timber technology, but what about product innovation? Can ChatGPT contribute to that as well?
@Melissa Brooks, AI, including ChatGPT, can aid in product innovation by analyzing market trends, customer feedback, and design possibilities. It can help generate creative ideas, optimize product features, and streamline the innovation process.
Melissa, AI can assist in innovation by generating new ideas and insights, ultimately leading to more advanced and market-oriented products.
Well said, Melissa! ChatGPT can aid in the ideation and design phases, leading to more innovative and customer-centric products.
Definitely, Melissa! ChatGPT's ability to analyze data and generate insights can greatly enhance product innovation in the timber industry.
I'm concerned about potential privacy issues when implementing AI for quality control. How can we ensure that customer data and sensitive information are protected?
@Matthew Lee, privacy and data protection are crucial considerations. Implementing security measures, anonymizing data, and adhering to relevant data protection regulations are essential to ensure customer data and sensitive information are adequately protected.
Thank you, Arnie, for moderating this insightful discussion. Let's continue pushing the boundaries of AI in quality control.
Absolutely, Matthew! It's been a pleasure discussing the potential of AI in quality control with all of you.
Thank you, Emma! Your contributions have been valuable. Let's strive for responsible and effective implementation of AI in quality control.
Absolutely, Thomas! Responsible implementation of AI is crucial to ensure it brings tangible benefits to quality control in an ethical and sustainable manner.
Thank you, Emma! It was a pleasure discussing AI in quality control. Let's continue exploring the potential together.
Completely agree, Sophie! This discussion has been thought-provoking, and I'm excited to witness the progress of AI in quality control.
Thank you, Aiden, for your insightful contributions. Let's stay optimistic as we navigate the future of AI in quality control.
Absolutely, Elizabeth! It was a pleasure discussing the potential of AI in quality control. Let's embrace its benefits responsibly.
I appreciate the benefits AI can bring, but how do we maintain a balance between automation and the human touch in quality control?
@Isabella Ward, finding the right balance is key. Human judgement, creativity, and intuition are valuable aspects that shouldn't be overlooked. Ensuring human oversight and collaboration with AI systems can help strike the right balance in quality control processes.
Timber technology is evolving rapidly. How future-proof is the use of ChatGPT for quality control?
@Max Turner, AI technologies like ChatGPT are constantly evolving, and their adaptability allows them to stay relevant. By regularly updating the model, incorporating new data, and refining the training process, the use of ChatGPT for quality control can remain future-proof.
Would ChatGPT be able to understand and respond to domain-specific terms and jargon used in the timber industry?
@Peter Hall, with the right training data and fine-tuning, ChatGPT can be taught domain-specific terms and jargon used in the timber industry. It can become familiar with industry-specific language, enabling more accurate and relevant responses.
How can companies ensure the privacy and security of their confidential timber technology data when utilizing AI for quality control?
@Grace Peterson, companies can protect their confidential data by implementing robust security measures, data access controls, encryption, and ensuring compliance with data protection regulations. Partnering with trusted AI providers that prioritize privacy can also be advantageous.
What kind of training would technicians and employees need to effectively work with ChatGPT in quality control processes?
@Oliver Smith, technicians and employees would benefit from training that familiarizes them with AI concepts, ChatGPT's capabilities, and its specific implementation in quality control. Technical skills related to data analysis and interpretation would also be valuable.
Thanks, Arnie! This discussion has been enlightening. Excited to follow the developments of ChatGPT in the timber industry.
How can companies ensure transparency and accountability in the decision-making processes of the AI system during quality control in timber technology?
@Sophie White, ensuring transparency and accountability can be achieved by thoroughly documenting the decision-making algorithms, regularly auditing and validating the AI system's performance, and involving human experts in the oversight of the quality control process.
I agree, Sophie. Transparency and accountability are vital to ensure the responsible use of AI in decision-making processes.
Absolutely, Rachel! Well-defined and transparent decision-making processes are necessary to ensure AI systems are accountable.
What are the scalability considerations when implementing ChatGPT for quality control in larger timber technology operations?
@Richard Stewart, scalability considerations include ensuring that the AI infrastructure can handle large volumes of data efficiently, optimizing computational resources, and fine-tuning models to maintain performance as the scale of operations grows.
I'm excited about the potential of ChatGPT in the timber industry. How soon do you think it will become a mainstream tool for quality control?
@Katie Rogers, the adoption of AI, including ChatGPT, in quality control is already underway in various industries. While it may take some time for widespread adoption in the timber industry, the rapid advancement of technology suggests it could become mainstream relatively soon.
Thank you all for the engaging discussion! Your questions and insights have been valuable. If you have any more thoughts or questions, feel free to continue the conversation.
Thank you, Arnie! It's been a thought-provoking discussion. Excited to see how ChatGPT progresses in timber technology.
Agreed, Lily! Thank you, Arnie, for sharing your insights. Looking forward to further developments in this space.
Thank you, Joshua! It was great discussing the potential of ChatGPT in quality control. Looking forward to future advancements.
Likewise, Joshua! Thank you, Arnie, for sharing your knowledge and insights on this exciting topic.
Thanks, Arnie! It was an engaging discussion. Excited to see what the future holds for ChatGPT in quality control.
Indeed, Edward! The potential of ChatGPT to enhance quality control processes is promising. Thanks, Arnie, for your valuable input.
Sarah, it's amazing how AI is finding its way into diverse areas like timber technology. Exciting times ahead!
Thanks, Arnie, for conducting this insightful discussion. Looking forward to further advancements in the application of AI in quality control.
Absolutely, Daniel! It was a pleasure engaging in this conversation. Cheers to the future of AI in quality control!
Thank you, Olivia, for your valuable contributions throughout this discussion. Let's embrace the potential of AI in quality control.
Agreed, Ethan! This discussion has been enlightening, and it's clear that AI can bring immense benefits to quality control processes.
Arnie, do you think there should be specific guidelines or standards in place to ensure the ethical and responsible use of AI in quality control?
@Daisy Ward, that's an important consideration. Establishing guidelines or standards can help ensure a consistent and responsible approach to AI in quality control. Collaborative efforts involving industry experts, practitioners, and regulatory bodies should be encouraged.
Thanks for facilitating this discussion, Arnie. It's been eye-opening to explore the ethical considerations in AI-driven quality control.
Thank you, Ethan, for engaging in this conversation and emphasizing the importance of ethical guidelines. Let's strive for ethical AI practices in quality control.
Absolutely, Grace! Ethical AI practices are crucial to ensure that quality control processes are both effective and transparent.
Thank you, Ethan, for facilitating this insightful discussion. It's important to keep ethics at the forefront when integrating AI into quality control.
Well said, Mila! Ethical considerations should guide the adoption and implementation of AI in quality control.
Absolutely, Anthony! Integrating ethics into AI-driven quality control is crucial to ensure responsible and beneficial outcomes.
Thank you, Alexandra, for your contributions to this discussion. Let's continue advocating the ethical use of AI in quality control.
I agree, Harper. Ethical practices are necessary to harness the full potential of AI in quality control while ensuring human well-being.
I agree, Daisy. Having clear guidelines can help address ethical concerns and prevent potential misuse of AI systems in quality control.
Absolutely, Elijah! Ethical guidelines can provide a framework for companies to navigate the ethical challenges associated with AI in quality control.
Ethical guidelines are pivotal in shaping the responsible and beneficial use of AI in quality control. Thank you for addressing this, Daisy.
Indeed, Aiden. Establishing ethical guidelines helps ensure the alignment of AI systems with the ethical standards and societal expectations of quality control processes.
Finding the right balance between automation and the human touch is essential. Both have unique strengths that, when combined, can result in superior quality control.
Scalability is a significant consideration. AI systems should be designed to handle increasing data volumes and larger operations without sacrificing performance.
Completely agree, Jason. Scalability is crucial for the successful implementation of AI in quality control.
Maintaining performance as operations scale up is an important aspect to consider when utilizing AI systems like ChatGPT.
I agree, Sophie. AI systems should be adaptable and capable of handling larger volumes of data as operations expand.
I'm also excited about the potential of ChatGPT in quality control! It could be a game-changer for the timber industry.
I believe that with continuous advancements in AI technology, mainstream adoption of ChatGPT for quality control in the timber industry is not too far off.
The benefits of ChatGPT in quality control are promising. It would be interesting to see more practical implementations and success stories.
AI has the potential to revolutionize multiple industries. It's exciting to witness its progress!
AI-driven optimization can contribute significantly to reducing the environmental footprint in industries like timber technology.
Innovation and AI go hand in hand. ChatGPT can bring valuable insights and enhance the overall product development process.
Transparency is critical to build trust in AI systems. Companies should strive for openness and clear communication regarding their quality control procedures.
With the ongoing advancements in AI technology, mainstream adoption of ChatGPT in quality control might be nearer than we think.
Indeed, Isla! The potential benefits of ChatGPT in quality control make it an interesting tool for the timber industry.
I'm excited to see how ChatGPT evolves and becomes more accessible for quality control purposes in various industries.
It was a pleasure discussing AI and quality control with all of you! Let's stay curious and continue exploring the possibilities.
Indeed, Sophia! This discussion has been enlightening, and it's great to see such enthusiasm for AI and quality control.
Thank you all for the enlightening conversation! Excited to see AI evolve and positively impact quality control in various industries.
Likewise, Mason! It's been a pleasure discussing AI with you all. Let's keep pushing the boundaries of what's possible.
Thank you, Austin, for your valuable contributions. It's been a great discussion, highlighting the promising role of AI in quality control.
Thanks, Liam! It was a pleasure exchanging ideas with you. Let's continue exploring the potential of AI in quality control.
Guidelines would ensure a more standardized and ethical approach, fostering trust in AI systems used in quality control.
I couldn't agree more, Logan! Well-defined guidelines would contribute to the responsible and transparent use of AI in quality control.
Thank you, Abigail, for highlighting the importance of guidelines. Let's work towards a future where AI in quality control is used ethically and responsibly.
Precisely, Mia! It's crucial to establish guidelines that foster ethical practices and ensure AI brings positive outcomes in quality control.
I wholeheartedly agree, Jake. Ethical guidelines provide a necessary framework to harness the potential of AI in quality control without compromising ethical standards.
Thank you, Abigail, for emphasizing the importance of ethical guidelines. Let's strive for an AI-powered quality control process that is fair, transparent, and ethically sound.
I hope that ChatGPT becomes more accessible in the timber industry soon. It could really benefit quality control processes.
@Liam Hill, I share your hope! As AI technology progresses, we can expect greater accessibility and user-friendly tools for quality control in the timber industry.
Finding the right balance between automation and the human touch is key to maximizing the benefits of AI in quality control.
Exactly, Sophie! The integration of AI should complement and enhance human expertise, ensuring the best possible outcomes.
Well said, Joseph! A human-AI collaboration can result in improved quality control without undermining the significance of human intuition and decision-making.
AI's potential to revolutionize industries is truly remarkable. It will be exciting to witness the advancements driven by AI in quality control.
Absolutely, Liam. AI in quality control holds significant promise, and it's progressing rapidly across various sectors.
I completely agree, Sophie. AI-driven quality control has the potential to redefine standards and create more efficient and reliable processes.
Well said, Samuel! The transformative impact of AI in quality control is already evident, and it will only continue to grow.
Having clear guidelines would also contribute to building public trust and minimizing any concerns related to the adoption of AI in quality control.
Absolutely, Lily! Clear ethical guidelines would provide a framework for responsible AI usage, promoting trust and transparency in quality control processes.
Well said, Charlotte! Ethical guidelines are essential for AI to be applied in quality control in a manner that respects moral values and safeguards human interests.
Thank you all for the insightful discussion! Ethical guidelines are critical to ensure AI-driven quality control benefits everyone involved.