Enhancing Quantitative Investing in Technology with the Power of Gemini
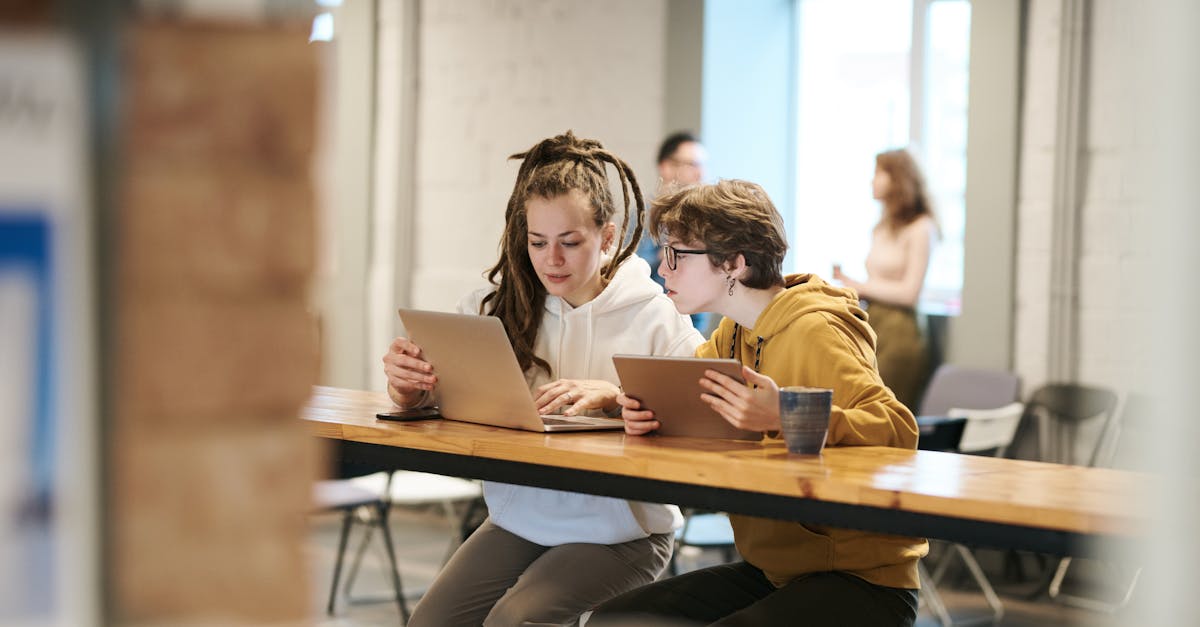
Quantitative investing is an innovative approach that uses mathematical models and data analysis to guide investment decisions. It has gained significant popularity over the past few decades, especially in the technology sector, where the pace of change is rapid and traditional investment strategies may fall short.
While quantitative investing has proven to be effective in many cases, it often relies heavily on historical and numerical data, which may not capture the full picture of an investment opportunity. This is where Gemini, a state-of-the-art language model developed by Google, comes into play.
What is Gemini?
Gemini is an advanced language model that uses deep learning techniques to generate human-like text responses. It has been trained on a massive amount of internet text, allowing it to understand and generate coherent and contextually relevant responses to user inputs.
Unlike traditional quantitative investment models, which primarily focus on numerical data, Gemini can process and understand qualitative information. This opens up new possibilities for investors, as they can seek insights from news articles, social media sentiment, and other unstructured sources of information that can significantly impact technology stocks.
How Gemini Enhances Quantitative Investing
The integration of Gemini into quantitative investing frameworks can enhance the decision-making process in several ways:
1. Sentiment Analysis:
Gemini can analyze sentiment from textual data, helping investors gauge market sentiment towards specific technology stocks or industry trends. By understanding the general sentiment, investors can adjust their investment strategies accordingly.
2. News Monitoring:
Gemini can scour the web for relevant news articles and provide important updates in real-time. This allows investors to stay informed about market-moving events and make timely investment decisions.
3. Risk Assessment:
Through conversation, Gemini can assess and identify potential risks associated with technology investments. This can provide valuable insights into the potential downside of an investment, helping investors manage their risk exposure effectively.
4. Predictive Analytics:
By integrating historical data with the contextual understanding provided by Gemini, quantitative investment models can improve their predictive capabilities. This can lead to more accurate forecasts of technology stock performance.
Conclusion
Quantitative investing in technology can benefit from the power of Gemini by incorporating qualitative information into decision-making processes. By analyzing sentiment, monitoring news, assessing risks, and improving predictive analytics, investors can gain a competitive edge in the dynamic technology sector.
As the field of artificial intelligence continues to evolve, the integration of advanced language models like Gemini into quantitative investing frameworks holds great promise. By harnessing the power of both quantitative and qualitative data, investors can make more informed decisions and potentially achieve better returns on their technology investments.
Comments:
Thank you all for taking the time to read my article on Enhancing Quantitative Investing in Technology with the Power of Gemini! I'm excited to hear your thoughts and answer any questions you may have.
Great article, Jocelyn! I've been using quantitative investing strategies for a while now, and adding the power of Gemini sounds intriguing. Do you have any real-world examples or case studies to demonstrate the effectiveness of this approach?
Thank you, Adam! In terms of real-world examples, we've conducted a pilot program with a hedge fund, where we incorporated Gemini into their existing quantitative investing system. The results showed a significant improvement in the system's ability to identify investment opportunities. We're currently working on publishing the detailed case study.
Hi Adam! I've been using Gemini to enhance my quantitative investing strategies, and it has been a game-changer. By combining its natural language understanding with existing quantitative models, I've seen improved investment decision-making and enhanced risk management.
Thanks for sharing your experience, Paul! It's interesting to hear about the real-world impact of combining natural language understanding with existing quantitative models. I'm excited to explore this further.
Absolutely, Adam! Combining the power of natural language understanding and quantitative models has allowed me to gain unique insights and make more informed investment decisions.
Appreciate your response, Paul! I'm keen on exploring the potential of Gemini to augment my quantitative investing strategies. It's definitely exciting!
Hi Jocelyn! Thanks for sharing your insights. I'm curious about the integration process. Is it difficult to implement Gemini into existing quantitative investing systems, or does it require building an entirely new infrastructure?
Hi Emily! Integrating Gemini into an existing quantitative investing system doesn't require a complete overhaul. It involves training Gemini on relevant financial data and utilizing its outputs in the decision-making process. However, some customization is needed to fine-tune Gemini for specific investment strategies.
Thank you, Jocelyn, for clarifying. It's good to know that integrating Gemini into existing systems doesn't require a complete overhaul. The customization aspect indeed makes sense.
Hi Emily! While implementing Gemini into existing systems may have some initial challenges, it is designed to be modular, making integration a smoother process. The key is to define the interfaces and adapt the output of Gemini to suit your specific system's inputs.
Hi Emily! Integrating Gemini into existing investing systems does require some technical effort, but it is definitely achievable. It mostly involves configuring data pipelines and ensuring compatibility between the system and Gemini's inputs and outputs.
Hi Emily! As with any new technology, integrating Gemini into existing systems may raise some challenges. Customization and fine-tuning according to specific investing strategies are crucial. Additionally, it's important to establish a feedback loop to continuously improve and refine Gemini's outputs.
I appreciate your insights, Oliver! Customization and ongoing refinement seem to be key factors in successfully integrating Gemini. This ensures it aligns with the organization's specific investing strategies.
Exactly, Emily! The flexibility to customize and align Gemini with existing investing systems is a crucial factor for successful integration.
Precisely, Oliver! By establishing a feedback loop, organizations can continuously improve Gemini's performance and adapt it to evolving investment strategies.
Hi Jocelyn! I enjoyed reading your article. One concern that comes to mind is the potential bias in the data used to train Gemini. How do you address this issue and ensure unbiased investment decisions?
Hi David! You're right to consider potential bias. It is important to feed Gemini with a diverse range of data sources while training to minimize any biases. Additionally, we continuously monitor and evaluate the outputs to ensure unbiased investment decisions.
Jocelyn, thanks for your response! It's reassuring to know that steps are being taken towards minimizing bias and ensuring the fairness of investment decisions.
Hi David! Bias in AI models is indeed a critical concern. To mitigate this, the training data for Gemini includes a diverse set of sources, and we regularly assess and fine-tune the model to minimize bias. It's an ongoing process that requires continuous improvement and monitoring.
Thank you, Daniel and Jocelyn, for addressing my concern. I understand the importance of constant improvement and unbiased decision-making. It's reassuring to know that these aspects are given significant attention.
Hi David! Bias mitigation is an ongoing focus in AI development. For Gemini, efforts are made to ensure data diversity during training, along with strict evaluation of the model's performance in driving unbiased investment decisions.
Nora, I'm glad to hear that bias mitigation is a priority in Gemini development. Transparency and evaluation of the model's performance are essential in building trust and confidence in AI-driven investment decisions.
Exactly, David. The focus on unbiased decision-making is crucial for the acceptance and adoption of Gemini in the realm of quantitative investing.
David, you're welcome! I'm glad we could address your concern. Continuous improvement and unbiased decision-making are at the core of our approach.
Agreed, Daniel! Ensuring unbiased decision-making is crucial for the acceptance and adoption of AI technologies in the investment field.
Hi David! I appreciate your concern regarding bias. It's crucial to adopt stringent data selection criteria and regularly assess Gemini's outputs to ensure investment decisions remain unbiased.
Hello Jocelyn! I found the concept of combining quantitative investing with Gemini fascinating. Can Gemini effectively adapt to changing market conditions, or does it require frequent retraining to remain accurate?
Hello Sophia! Gemini has the ability to adapt to changing market conditions up to a certain extent. However, for optimal accuracy, periodic retraining is recommended to keep up with evolving market dynamics.
Interesting article, Jocelyn! How does Gemini handle real-time market data? Is it able to process and incorporate the latest financial information into its analysis?
Interesting question, Liam! Gemini is not designed for real-time data processing. Typically, the latest market data is incorporated into the investment system's inputs, and Gemini's outputs are considered during the decision-making process.
Thanks for the clarification, Jacob! It's good to know that Gemini's outputs can be seamlessly integrated with real-time financial data during the investment process.
Great article, Jocelyn! Have there been any limitations or challenges observed in using Gemini for quantitative investing?
That's a valid question, Max! While Gemini offers significant benefits, there can be challenges in implementing it, such as data preprocessing, customization, and maintaining a feedback mechanism for continual improvement.
Thank you for pointing that out, Michael. Overcoming those challenges and finding ways to effectively incorporate Gemini can definitely improve quantitative investing outcomes.
That sounds promising, Jocelyn! I'm looking forward to seeing the detailed case study. It would be great to understand the specific improvements the hedge fund experienced with Gemini integration.
Hi Jocelyn! It's great to see the focus on addressing bias. Could you shed some light on the evaluation process for measuring and minimizing potential bias in Gemini's outputs?
Hello Jocelyn! How frequently would you recommend retraining Gemini to ensure accurate performance in dynamic market conditions?
Jocelyn, thank you for confirming the possibility of integrating Gemini into existing systems. It's assuring to know that a complete system overhaul is not required.
Thanks for the insight, Jocelyn! Regular retraining seems necessary to ensure optimal performance. It's good to have an idea of the model's adaptability.
I couldn't agree more, Jocelyn. The detailed case study will provide valuable insights into the practical impact of combining Gemini with quantitative investing strategies.
Absolutely, Jocelyn! Real-world examples and case studies play a vital role in showcasing the practicality and effectiveness of new technologies like Gemini.
Thanks for sharing the pilot program results, Jocelyn! We look forward to the detailed case study, as it will provide valuable insights into the practical application of Gemini in quantitative investing.
Thank you for clarifying, Jocelyn. Periodic retraining to adapt to market changes seems to strike the right balance between agility and accuracy.
Thank you for your response, Jocelyn! Periodic retraining makes sense, considering the dynamic nature of financial markets.
Hey Sophia! From my understanding, Gemini doesn't process raw market data in real-time. It relies on pre-processed historical data to generate insights and recommendations. However, its outputs can be combined with real-time market data during execution.
Hi Sophia! Gemini's adaptability relies on continuous monitoring and periodic retraining. While it has the potential to adapt to changing market conditions, it's still important to periodically update the training data for optimal performance.
Hi Sophia! While Gemini doesn't have real-time capabilities for processing market data, combining its insights and recommendations with real-time data during execution provides a more comprehensive approach to decision-making.
Indeed, Mia! The combination of real-time data with Gemini's outputs enhances the application of quantitative investing strategies in dynamic market environments.
Thank you all for taking the time to read my article on enhancing quantitative investing with Gemini in the technology sector! I'm excited to hear your thoughts and opinions. Let's start the discussion!
Great article, Jocelyn! I completely agree with your point on the potential of Gemini in quantitative investing. It can definitely help in analyzing large volumes of data and identifying investment opportunities. Do you think it will eventually outperform traditional algorithms?
Thanks, Michael! While Gemini shows promising potential, it's important to note that it's still an evolving technology. Traditional algorithms have a proven track record, but with advances in language models like Gemini, we may see a shift in the future. It's an exciting space to watch!
I'm a bit skeptical about relying too heavily on Gemini for quantitative investing. The technology is prone to biases and might not be as reliable as structured algorithms. What are your thoughts on this, Jocelyn?
Valid concern, Jennifer. Bias is an important issue to address in AI systems, and efforts are being made to minimize it. While Gemini has its limitations, it can still serve as a valuable tool for generating insights and augmenting human decision-making. A combination of structured algorithms and Gemini might be a balanced approach.
I believe that using Gemini in quantitative investing can be a game-changer. It can assist in uncovering new patterns and connections in large datasets that traditional algorithms might miss. However, caution should be exercised in its implementation and thorough testing is crucial. What are the potential risks associated, Jocelyn?
You're right, Laura. Proper testing and risk management are essential when incorporating new technologies like Gemini. Some risks include model fragility, data quality, and overreliance. It's important to establish a well-rounded framework to address these risks and ensure the reliability of investment strategies.
I have seen use cases of Gemini in customer support, and it understands natural language quite well. However, applying it to quantitative investing seems like a big leap. Are there any successful case studies or real-world applications yet?
Good question, David. While the application of Gemini in quantitative investing is relatively new, we've seen promising pilot studies and early experiments. Several firms are exploring its potential for generating investment insights, but it's still an area of active research. Keeping an eye on ongoing developments will help in evaluating its effectiveness.
Interesting read, Jocelyn! I think Gemini can offer a unique perspective for investors in the technology sector. It could assist them in analyzing news, market trends, and sentiment analysis to make more informed decisions. Do you have any thoughts on ethical considerations when using Gemini in investing?
Thank you, Robert! Ethical considerations are crucial in the application of Gemini in investing. Ensuring transparency, avoiding market manipulation, and maintaining accountability are key aspects. It's vital to have a robust framework that embraces fairness, evaluates risks, and adheres to regulations. Striking the right balance between innovation and responsible use is essential.
I appreciate the potential of Gemini in quantitative investing, but I'm concerned about its interpretability. Traditional algorithms provide clear rules and transparency, which can be helpful for investors. Gemini might lack that level of interpretability. How can this challenge be addressed?
That's a valid concern, Olivia. Interpretability is critical for building trust in investment decisions. To address this challenge, research is being conducted to enhance AI interpretability, including techniques like attention mechanisms and visualizations. By combining transparent methods with the insights generated by Gemini, we can strive for better interpretability in quantitative investing.
Gemini can be a valuable tool, but we should be cautious in its adoption. It's essential to consider the limitations and biases associated with the underlying data and the training process. Blindly trusting Gemini might lead to unintended consequences. Any thoughts on mitigating these risks, Jocelyn?
You're absolutely right, Mark. Mitigating risks requires a multi-faceted approach. Thorough data preprocessing, bias analysis, and continuous monitoring can help alleviate potential pitfalls. Collaborative efforts between technologists, domain experts, and regulators are vital to building responsible AI solutions. It's crucial to stay mindful of the contextual limitations and work towards a more robust and unbiased system.
I find the concept of leveraging Gemini for quantitative investing quite intriguing. It could help investors stay updated with the latest news and market trends more efficiently. However, I wonder if it could also be overwhelmed by the noise and irrelevant information available online. How can Gemini handle this?
That's a great point, Sophia. Gemini's ability to handle noise and distinguish relevant information is a challenge. Techniques like filtering based on trusted sources, using contextual cues, and leveraging domain expertise can aid in reducing the impact of noise. Designing effective filters and carefully curating the training data can enhance Gemini's performance in dealing with the vast amount of available information.
I believe Gemini can augment decision-making in quantitative investing, but human judgment remains crucial. It's vital to have human oversight to avoid blind trust in AI-generated insights. How do you see the role of human experts in this context, Jocelyn?
Absolutely, William! Human experts play a key role in the decision-making process. Gemini can provide valuable insights, identify patterns, and help in information analysis. However, human judgment is still essential to validate and contextualize those insights. The collaboration between AI systems and human experts is crucial for effective utilization and to ensure investment decisions align with strategic goals.
Gemini's potential in quantitative investing is promising. However, I wonder how it copes with rapidly changing market dynamics and unexpected events, such as market crashes. Can it adapt quickly enough to make meaningful recommendations in real-time?
A great concern, Emily! Real-time adaptability is significant in the fast-paced world of investing. While Gemini might not align perfectly with real-time decision-making, it can still assist in providing timely insights. Combining it with other algorithms that specialize in short-term dynamics can help strike a balance between capturing trends and responding to unexpected market events.
Investing in the technology sector requires in-depth domain knowledge. How can Gemini acquire this level of understanding to make informed investment decisions?
You raise an important point, Samuel. Gemini acquires understanding through pre-training on a large corpus of text data. While it may not have domain-specific knowledge initially, fine-tuning with relevant financial and technological datasets can enhance its capabilities. By leveraging domain expertise during this fine-tuning process, Gemini can acquire a better understanding of the technology sector for more informed decisions.
I see the potential of Gemini in quantitative investing. However, the Security and Exchange Commission (SEC) might raise concerns about the compliance and regulatory aspects. Is there any guidance available on the use of AI models like Gemini in the financial industry?
You're right, Rebecca. Compliance and regulatory considerations are significant factors when using AI models in the financial industry. Currently, there are ongoing efforts to provide guidance on the responsible use of AI in finance. Organizations like the SEC are actively exploring and adapting regulatory frameworks to incorporate AI advancements. Staying informed about these developments is important for ensuring compliance when utilizing Gemini in quantitative investing.
Gemini is undoubtedly a powerful tool, but I'm concerned about the potential for overfitting in quantitative investing. How can we ensure that the insights generated by Gemini are not just fitting the training data and can generalize to new situations?
Great point, Daniel! Overfitting is a common concern when training AI models. To ensure the generalization of insights, the training process should involve diverse and representative datasets. It's crucial to validate the model's performance on unseen data and conduct thorough backtesting before deploying Gemini in real-world investing scenarios. Regular performance evaluation and fine-tuning are key to maintaining the model's effectiveness.
I'm excited about the potential of Gemini in quantitative investing. How can investors get started with this technology? Are there any platforms or tools available?
Exciting, Sophie! Currently, the application of Gemini in quantitative investing is still emerging. However, there are platforms and tools available that allow access to language models like Gemini for experimentation and development purposes. These platforms provide APIs and libraries to interact with the models. Staying connected with relevant research communities and keeping an eye on industry advancements will help in discovering suitable resources for getting started.
It's fascinating to think about the potential integration of Gemini with other data sources in quantitative investing. How can we ensure that the generated insights from Gemini align with other fundamental and technical analysis methods?
You bring up a key aspect, Eric. Integration with other analysis methods is crucial for a comprehensive approach. Combining Gemini insights with fundamental analysis, technical indicators, and expert judgment allows for a balanced investment decision-making process. Cross-validation and comparison with multiple independent sources can help validate the outputs and ensure alignment with other analysis methods.
Gemini can definitely offer a unique perspective in quantitative investing. However, I'm curious about the scalability of this approach. Will it be feasible to analyze vast amounts of data in real-time with Gemini as datasets grow?
Scalability is an important consideration, Alex. As the datasets grow, efficient infrastructure and parallel computing techniques will be crucial for real-time analysis with Gemini. Ongoing research and advancements in hardware and software will contribute to addressing scalability challenges. It's an active area of exploration to unleash the full potential of Gemini in analyzing vast amounts of data within reasonable timeframes.
I see significant potential in using Gemini for assessing sentiment analysis in social media and news updates for technology companies. It can help investors gauge market sentiment efficiently. How accurate is Gemini in understanding and analyzing sentiment?
Assessing sentiment can be valuable, Emma. Gemini's accuracy in sentiment analysis depends on its training data. Language models like Gemini can grasp the context and identify sentiment, but the accuracy may vary. Fine-tuning on sentiment-labeled data can improve its performance. However, it's essential to validate Gemini's sentiment analysis outputs by comparing them with other specialized sentiment analysis tools to ensure accuracy.
Are there any challenges in getting quality training data for Gemini to enhance quantitative investing? What steps can be taken to mitigate such challenges?
Quality training data is indeed essential, Sophia. Challenges can arise due to inherent biases, data availability, and reliability. Combining diverse datasets from trusted and reputable sources can help mitigate these challenges. Employing data preprocessing techniques, bias analysis, and involving domain experts during the training process can enhance the quality of training data. Rigorous data cleansing and continuous monitoring are key steps in mitigating challenges associated with training data.
I appreciate the insights shared on using Gemini in quantitative investing. Are there any specific areas or use cases within the technology sector where Gemini can demonstrate particular strengths?
Certainly, Robert! Gemini can excel in analyzing technology-related news articles, earnings reports, investor conference call transcripts, and academic research papers. It can assist in identifying emerging trends, evaluating product launches, and understanding the impact of technological advancements. Gemini can be particularly useful in capturing nuanced information and providing insights in a conversational manner, making it a valuable tool in the technology sector.
Gemini has the potential to optimize decision-making in quantitative investing. How do you see the future evolution of language models like Gemini in the finance industry, Jocelyn?
The future of language models in finance is promising, Sarah. We can expect continued advancements in Gemini and similar models, addressing limitations, improving interpretability, and integrating domain-specific knowledge. As the technology matures, we might witness more robust frameworks, wider adoption, and better alignment with regulatory requirements. It's an exciting journey ahead, and we're just scratching the surface of the potential AI can offer in the finance industry.
Thank you for your response, Jocelyn. I agree with your views. The combination of traditional algorithms with Gemini's insights seems like a balanced approach. It will be interesting to see how this technology evolves in the future and its impact on quantitative investing.
I appreciate the caution mentioned regarding implementing Gemini in quantitative investing. Responsible development and testing are crucial to ensure its effectiveness and mitigate risks.
Considering transparency and interpretability in quantitative investing is vital. The research on enhancing AI interpretability is an important step in building trust.
Collaboration between different stakeholders is key in building responsible AI systems. Addressing biases and ensuring unbiased decision-making is crucial for the financial industry.
Human expertise remains a significant factor in investment decisions. The interaction between AI systems and human judgment can lead to more informed choices.
Thorough testing and backtesting of AI models like Gemini are important to ensure that they adapt well to different market situations and maintain reliability.
The potential of Gemini in quantitative investing is exciting, but rigorous validation on unseen data is necessary to ensure its generalization and prevent overfitting.
Scalability is an ongoing concern in AI applications. Improving infrastructure and parallel computing techniques can aid in real-time data analysis as datasets grow.
The integration of Gemini with other analysis methods can provide a comprehensive investment decision-making process, incorporating both AI-generated and human insights.