Enhancing Recommendation Systems: Leveraging ChatGPT for Pesquisa Technology
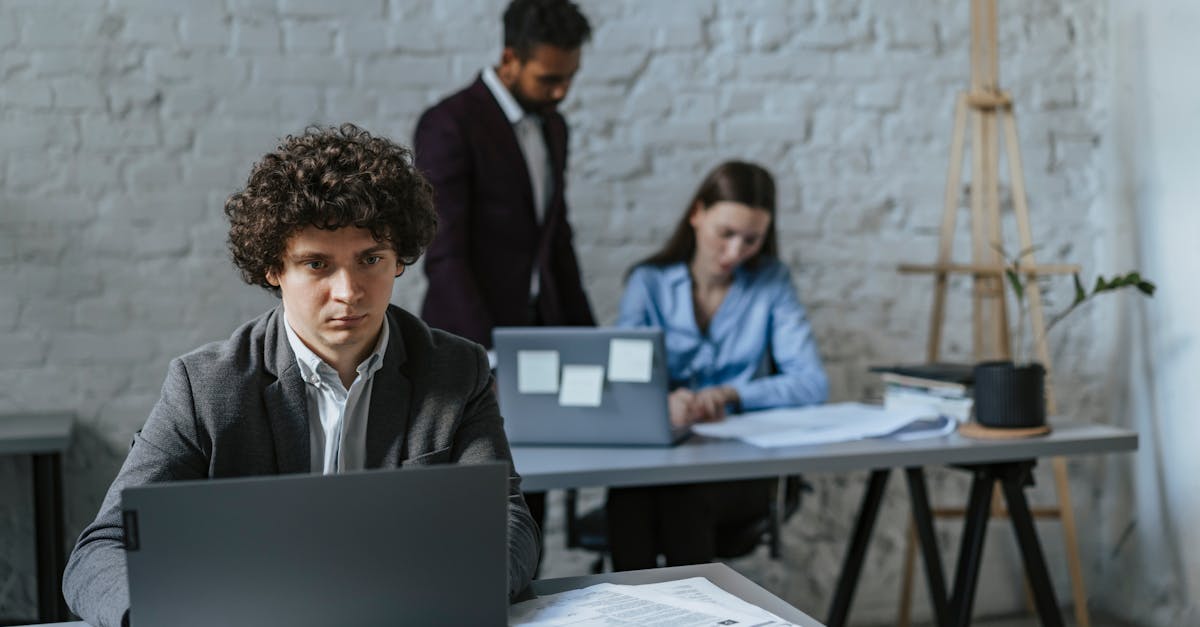
Recommendation systems have become an increasingly prevalent feature of digital platforms. They utilize vast amounts of data to provide users with tailored content based on their past behaviors, preferences, and interactions. The purpose of recommendation systems is to personalize the user's experience and increase user engagement on platforms such as social media, e-commerce sites, and streaming services. Recently, the technology 'Pesquisa' has been making waves for its potential usage in enhancing these systems.
What is Pesquisa Technology?
Pesquisa, a technology developed with the objective to analyze, process, and understand data, is increasingly being utilized across various digital platforms. Its ability to interpret complex user behaviors and preferences with high precision makes it an ideal candidate to help refine recommendation systems. Pesquisa technology uses a sophisticated algorithm and learning models to understand the user's behavior based on the generated content. It then uses this understanding to make predictions and yield recommendations that are personalized to the user's taste.
The Role of User-generated Content in Recommendation Systems
User-generated content plays a significant role in building a powerful recommendation system. This data comes directly from the users in the form of reviews, ratings, comments, shares, likes, or any other interaction. By analyzing this content, it's possible to discern user preferences and interests. However, the management and understanding of such a massive amount of unstructured data can be a challenging task. Here is where Pesquisa technology comes in.
Pesquisa Technology in Action
As a method of refining recommendation systems, Pesquisa begins by collecting user-generated content. This high-speed technology can capture vast amounts of user data in real-time, allowing for a constant flow of information. Next, Pesquisa processes and analyzes these data points. Thanks to advanced algorithms, Pesquisa can highlight patterns, trends, and correlations within the user data. By understanding these, Pesquisa can refine the recommendation system of a digital platform.
After analyzing the user-generated content, Pesquisa's next step is to interpret this data. Using AI and machine learning models, Pesquisa can understand complex user behavior patterns and preferences. With this deeper insight, it can predict what a user may be interested in viewing or purchasing next, tailoring recommendations accordingly.
Benefits of Utilizing Pesquisa Technology in Recommendation Systems
There are many benefits of harnessing the power of Pesquisa in recommendation systems. Firstly, it allows for highly personalized user experiences. By interpreting user-generated content, Pesquisa can understand an individual's distinct tastes and preferences. It can make accurate predictions, resulting in more relevant and appealing recommendations.
Secondly, Pesquisa's adaptability is highly beneficial. The technology can learn and adapt to changing user behaviors over time, allowing it to adjust its predictions and recommendations as required. This constant evolution ensures that the system stays relevant and useful to individual users, even as their interests and preferences change.
Lastly, Pesquisa makes recommendation systems more efficient. With this technology, platforms can process vast amounts of data quickly and accurately, and produce high-quality recommendations. This efficiency can lead to increased user engagement and satisfaction, ultimately boosting platform performance.
Conclusion
In the era of data-driven decision-making, Pesquisa technology has shown exponential potential in refining recommendation systems. By harnessing user-generated content in a more efficient and sophisticated way, Pesquisa can help digital platforms to provide more personalized, engaging, and satisfying user experiences. As Pesquisa technology continues to evolve, the impact it can make on recommendation systems and in the broader digital landscape is bound to become even more substantial.
Comments:
Thank you for taking the time to read my blog article on enhancing recommendation systems using ChatGPT for Pesquisa Technology. I would love to hear your thoughts and feedback.
Great article, Claudio! I found it very informative and well-explained. It's fascinating to see how AI technology like ChatGPT can be leveraged to improve recommendation systems.
I completely agree, Anna! The integration of ChatGPT into recommendation systems has the potential to enhance personalization and provide more accurate suggestions.
I'm curious about the scalability of using ChatGPT in recommendation systems. Claudio, what challenges did you encounter in terms of scalability?
Good question, Sophia. One of the main challenges was the computational resources required to run ChatGPT effectively. Scaling up the model can pose difficulties in terms of cost and performance. However, optimization techniques and efficient hardware utilization can address these challenges.
I have a concern regarding the potential biases in the recommendation system. How can we ensure that ChatGPT doesn't inadvertently promote biased content or stereotypes?
Valid concern, Chris. Bias mitigation is crucial to ensure fairness. While ChatGPT alone may not completely eliminate biases, it is important to curate diverse and representative training data and implement bias-aware fine-tuning techniques. Regular monitoring and auditing of system outputs can help address any biases that may arise.
I really enjoyed your article, Claudio! One question that comes to mind is how well ChatGPT adapts to different domains. Have you tested its performance across various recommendation scenarios?
Thanks, Lisa! ChatGPT has shown promising adaptability across different domains. It can be fine-tuned on specific recommendation datasets to improve its relevance and effectiveness within those domains. Extensive evaluation and experimentation help assess its performance in varied recommendation scenarios.
The collaboration between recommendation systems and ChatGPT sounds intriguing. Claudio, what are the specific benefits of incorporating a conversational AI model like ChatGPT into recommendation systems?
Good question, David. By incorporating ChatGPT, recommendation systems can offer more interactive and personalized experiences. Users can engage in conversations to provide feedback, refine preferences, and receive tailored recommendations in real-time. This conversational aspect enhances user engagement and satisfaction.
I appreciate the insights, Claudio! However, I'm curious about the potential privacy implications of using ChatGPT in recommendation systems. Could you shed some light on how user data is handled and protected?
Absolutely, Emily! Privacy is of utmost importance. When using ChatGPT in recommendation systems, it's essential to implement robust privacy measures. User data should be anonymized, encrypted, and stored securely. Adhering to privacy regulations and obtaining user consent is vital to protect their sensitive information.
The applications of ChatGPT in recommendation systems seem promising. What is the typical deployment process like for incorporating ChatGPT into an existing recommendation system?
Great question, Oliver. The deployment process involves several steps. First, ChatGPT needs to be fine-tuned on relevant recommendation data. Once trained, it can be integrated into the recommendation system's backend infrastructure. Extensive testing and monitoring are essential before rolling it out to users.
Claudio, do you think incorporating ChatGPT into recommendation systems could potentially replace traditional collaborative filtering algorithms?
That's an interesting point, Sophie. While ChatGPT offers a more personalized and interactive approach, it may not entirely replace collaborative filtering algorithms. Instead, these methods can be combined to leverage the strengths of both and improve overall recommendations.
I wonder how user acceptance and trust play a role when adopting ChatGPT in recommendation systems. Claudio, have you come across any user studies or surveys on this aspect?
Good question, Adam. User acceptance and trust are indeed crucial factors. While I haven't conducted specific user studies, existing research indicates that users appreciate the conversational nature of ChatGPT and find it valuable in improving recommendation experiences. Building transparency and explaining the system's limitations can further enhance user trust.
I can see how ChatGPT can add a human touch to recommendation systems. Claudio, are there any ethical considerations that should be taken into account when deploying ChatGPT in such systems?
Absolutely, Isabella. Ethical considerations are paramount. It's important to ensure the responsible use of ChatGPT and adhere to ethical guidelines. Transparency, fairness, and accountability should be embedded in the system design, and regular audits should be carried out to prevent unintended consequences.
Great job on the article, Claudio! Since ChatGPT relies on pretrained models, how do you ensure that it stays up-to-date with the latest information and trends?
Thank you, Laura! To keep ChatGPT up-to-date, periodic model updates and retraining with recent data are necessary. It's crucial to continually monitor the performance and evaluate if any model updates are required to align with the latest information and trends in recommendation scenarios.
Interesting read, Claudio! Considering the limitations of ChatGPT, how do you handle cases when it might generate irrelevant recommendations or fail to understand user inputs properly?
Good question, Rachel. Handling cases of irrelevant recommendations or misunderstandings is challenging. Techniques like user feedback loops, active learning, and incorporating fallback mechanisms can help improve system performance in such cases. Iterative refinement and continuous evaluation play a crucial role in addressing these limitations.
I enjoyed your article, Claudio! It got me thinking about the potential risks of deploying recommendation systems powered by ChatGPT. Are there any measures to mitigate these risks?
Thank you, Daniel! Mitigating risks in recommendation systems powered by ChatGPT involves measures like regular auditing, bias detection and reduction, and incorporating user feedback channels. Close monitoring and staying informed about emerging risks and vulnerabilities also help in actively addressing potential issues.
Claudio, I wanted to know if ChatGPT can handle multi-modal recommendations, involving not only textual data but also images or other media types.
Good question, Emily. While ChatGPT primarily focuses on text-based interactions, it can still be part of a recommendation system that includes multi-modal content. By leveraging additional AI models for image or media analysis, ChatGPT can provide tailored recommendations that consider multi-modal inputs.
I'm impressed by the potential of ChatGPT in recommendation systems. Claudio, can you share some examples of real-world applications where ChatGPT has been successfully integrated?
Certainly, Tom! ChatGPT has been successfully integrated into e-commerce platforms, news aggregators, and music streaming services. It enables more interactive and personalized recommendation experiences, leading to improved user engagement and satisfaction.
Claudio, I'm curious about the computational requirements and latency involved when deploying ChatGPT in real-time recommendation systems.
Good question, Hannah. Deploying ChatGPT in real-time recommendation systems can be resource-intensive. The computational requirements depend on the model's size, hardware infrastructure, and the number of simultaneous requests. Reducing latency requires efficient hardware utilization, optimization techniques, and considerations like request batching and caching.
I enjoyed your article, Claudio! With the constant advances in AI, do you foresee any future developments or potential improvements in recommendation systems with the help of technologies like ChatGPT?
Thank you, Evan! In the future, we can expect further advancements in recommendation systems with the help of technologies like ChatGPT. Improved language models, multi-modal capabilities, and better contextual understanding have the potential to enhance recommendation accuracy, personalization, and user satisfaction.
Claudio, I'm curious about the fine-tuning process for ChatGPT in the context of recommendation systems. How do you go about selecting the most appropriate training data for the fine-tuning phase?
Good question, Sophia. Selecting appropriate training data for fine-tuning involves considering various factors. Typically, a combination of existing recommendation datasets, user feedback, and specific domain-related data can be used. Ensuring diverse, representative, and high-quality training data is crucial for improving the model's relevance and performance for the recommendation domain.
Claudio, are there any limitations or challenges in using ChatGPT for recommendation systems that you think need further research or development?
Absolutely, Oliver. Some areas that require further research and development include addressing bias, improving interpretability, enhancing user control and customization, and handling long-term user preferences. Ongoing research in these areas will help overcome current limitations and advance the field of recommendation systems powered by ChatGPT.
Great article, Claudio! I can see the potential benefits of incorporating ChatGPT into recommendation systems. It can add a conversational element and enhance user engagement. Thanks for sharing your expertise.
Thank you, Jessica! I appreciate your kind words and support. It's a pleasure to share insights and contribute to the advancement of recommendation systems.
I found your article very interesting, Claudio! How do you see the future collaboration between AI models like ChatGPT and recommendation systems shaping the way we discover and consume various forms of content?
Thank you, Alex! The collaboration between AI models like ChatGPT and recommendation systems has the potential to revolutionize content discovery and consumption. It can enable more personalized, adaptive, and satisfying experiences across multiple domains, including e-commerce, media, and information retrieval.
Claudio, what are the key factors to consider when deciding whether to incorporate ChatGPT into an existing recommendation system?
Good question, Sarah. Several factors come into play when making this decision. Some key considerations include the specific requirements and characteristics of the recommendation system, available computational resources, potential benefits in terms of personalization and user engagement, and the feasibility of integrating ChatGPT within the existing infrastructure.
Claudio, could you provide some examples of how ChatGPT has been evaluated for recommendation tasks?
Certainly, Jack! ChatGPT has been evaluated using various metrics, including accuracy of recommendations, user satisfaction surveys, relevance assessments, and A/B testing. These evaluation methods offer insights into the performance and effectiveness of ChatGPT in recommendation scenarios.
Claudio, I appreciate your article! Besides recommendation systems, can ChatGPT be applied to other areas in AI or computer science?
Absolutely, Sophie! ChatGPT can be applied to a wide range of areas beyond recommendation systems. It has potential applications in natural language understanding, dialogue systems, customer support, content generation, and more. The versatility of ChatGPT makes it a valuable tool across various AI and computer science domains.
Thank you all for your thoughtful comments and engaging discussion! I appreciate your valuable insights and feedback on the topic of enhancing recommendation systems using ChatGPT. If you have any more questions or ideas, feel free to share.