Enhancing Reliability Engineering in Technology Through the Use of ChatGPT
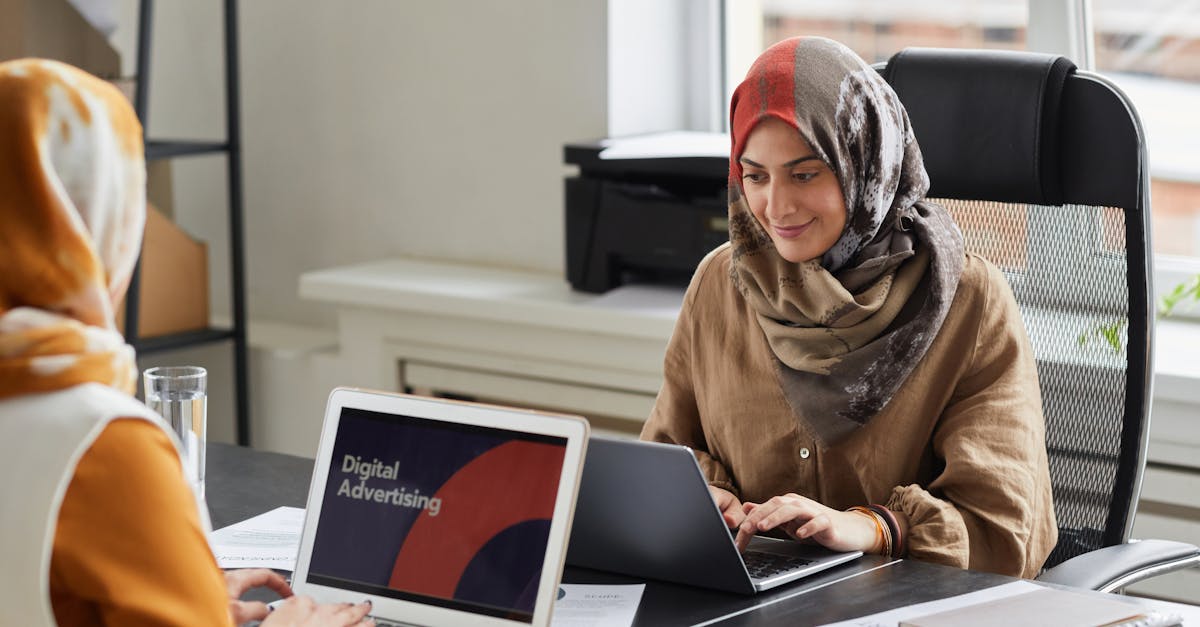
Reliability engineering has become an integral part of manufacturing and production industries. It is a field of engineering that emphasizes the capability of systems to perform without failure over a certain period. In the present times, the scope of this discipline has broadened to include predictive maintenance in its ambit. This practice involves the prediction of future failures of machinery to prevent them from occurring. The advances in technology have paved a way for predicting these failures. One such technological innovation that holds huge potential is the utilization Chatgpt-4 as a tool for predictive maintenance.
Reliability Engineering: A Brief Overview
Reliability engineering is based on the principle of ensuring that systems and components meet their performance requirements. This is a field that prioritizes the dependable operation of systems. An unreliable piece of machinery or equipment can result in operational inefficiencies, unanticipated expenses, and, at worst, catastrophic system failures.
One of the main components of reliability engineering is predictive maintenance. As an approach, predictive maintenance allows companies to anticipate errors and breakdowns, correcting them before they escalate. It leverages continuous monitoring data and advanced analytics to forecast when equipment parts might fail. The ability to predetermine when a failure might likely occur helps in optimized planning for maintenance tasks — without disrupting the normal operation of systems.
Chatgpt-4: A Technological Revolution
The modern predictive maintenance landscape is rapidly evolving, driven by breakthroughs in technology. Among these technologies, one that stands out is the Chatgpt-4. It is an advanced machine learning model developed by OpenAI that endows computers with the ability to understand and generate human-like text.
At first glance, it's not immediately apparent how a computer program designed to generate text like a human could be of use to reliability engineers. However, the key idea here lies in the ability of Chatgpt-4 to interpret and generate human-like text. This aspect can be further utilized to analyze maintenance data and predict machinery failures before they even happen.
How Chatgpt-4 can Predict Machinery Failures
The potential of Chatgpt-4 for predictive maintenance can be unleashed by 'training' it on maintenance data. This data might contain a record of machine faults, precise steps taken to rectify those faults, and details of when and why the machine failed. Once trained on this data, Chatgpt-4 would be able to recognize patterns or anomalies that might indicate a future failure.
The system could be set up to monitor a machine's operational data in real-time. This would include data such as temperature, pressure, and other key parameters related to general operations. When the model has been properly trained, it can swiftly process and evaluate this data to identify concerning trends or anomalies. If abnormal patterns are detected, the model can warn operators about potential failures. Thus, allowing teams to rectify the issue before it escalates into a full-blown machine breakdown.
Conclusion
To conclude, the incorporation of Chatgpt-4 in the field of predictive maintenance holds immense potential. This AI-powered model could revolutionize the way industries manage their machinery, It can maximize efficiency, reduce costs, and importantly prevent catastrophic failures. As its potential gets fully explored, the merger of reliability engineering and AI will undoubtedly usher in a new era of industrial efficiency and productivity.
Comments:
Thank you all for taking the time to read my article on enhancing reliability engineering using ChatGPT. I'm excited to hear your thoughts and engage in a discussion.
Great article, Vicki! ChatGPT indeed seems like a promising technology that could greatly enhance reliability engineering. It can help automate repetitive tasks, provide real-time analysis, and suggest improvements. I'm curious to know if there are any potential limitations to its application. Thoughts?
Hi Michael, I agree with you on the potential benefits of ChatGPT. One limitation I can think of is that it heavily relies on the input data quality. If the data used to train the model is flawed or biased, it could negatively impact the reliability of its suggestions. Proper data sanitization would be crucial. What do others think?
Michael, to add to Jennifer's point, another limitation is the potential for the model to generate false positives or negatives. While ChatGPT is impressive, it's not infallible. Human validation and thorough testing would be essential to ensure its reliability. Do you agree?
Jennifer and Mark, thank you for sharing your insights. I completely agree with your points regarding data quality and validation. It's crucial to rely on human judgment and oversight to avoid potential pitfalls. ChatGPT should support human decision-making, not replace it.
I found this article to be quite interesting. ChatGPT can certainly streamline the reliability engineering process and boost efficiency. However, I wonder if there could be any ethical concerns when using AI in such critical domains. Thoughts?
Indeed, Sarah, ethical concerns are a valid point to consider. Bias in the training data, inadvertent discrimination or lack of transparency in the decision-making process are some potential risks. It's crucial to ensure that AI systems like ChatGPT are designed with fairness and ethics in mind. Vigilant monitoring and bias-checking are crucial.
Sarah and Jennifer, you both raise important ethical considerations. Transparency should be a fundamental aspect of using AI technologies like ChatGPT. Organizations need to be accountable, actively addressing biases and ensuring fairness and inclusivity. Frequent audits and diverse human oversight can help minimize the risks associated with AI-driven decision-making.
Vicki, your article offers valuable insights into integrating ChatGPT into reliability engineering. One aspect I wonder about is the potential cybersecurity risks of relying on an AI-powered system. How can we ensure data security and protect against malicious attacks?
Daniel, that's a valid concern. Cybersecurity should be a priority when implementing AI systems. Proper encryption, access controls, and continuous monitoring are essential. Additionally, regular security audits and prompt vulnerability patching should be part of the overall strategy. Do you agree?
Absolutely, Sarah. Cybersecurity is a critical aspect when leveraging AI technologies. Organizations should follow industry-best practices, conduct regular security assessments, and foster a culture of security awareness among employees. Protecting sensitive data is of utmost importance to maintain reliability and trust in the system.
Vicki, I enjoyed reading your article. The potential of ChatGPT in reliability engineering is immense. However, I wonder if incorporating such AI systems can lead to a loss of human expertise. How do we strike the right balance between automation and human intervention?
Emily, you bring up a crucial point. While AI can automate tasks and provide valuable insights, it should always complement human expertise, not replace it entirely. Humans possess contextual understanding and intuition that machines lack. Striking the right balance between automation and human intervention is vital for optimal results.
I completely agree with Michael. AI should be treated as a tool that empowers human experts, fostering collaboration and amplifying their capabilities. Expert knowledge combined with AI-driven analysis can lead to more accurate and efficient decision-making in reliability engineering.
Thank you, Emily, Michael, and Jennifer, for your thoughtful comments. Striking the right balance between automation and human expertise is indeed key. AI should augment and support human professionals, enabling them to focus on higher-level tasks and complex problem-solving while benefitting from AI-driven insights.
Vicki, your article shed light on an exciting application of AI in reliability engineering. I'm curious to know if you have any recommendations on implementing ChatGPT in small-scale businesses with limited budgets. Is it economically feasible?
Thomas, while Vicki can provide more insight, I believe implementing ChatGPT in small-scale businesses would depend on various factors. It might be challenging due to budget constraints, but open-source alternatives or cloud-based solutions with flexible pricing could be potential options. Finding the right balance between cost and benefit is crucial.
Thomas and Mark, you've touched upon an important point. Affordability and budget considerations can be a challenge. However, as AI technologies evolve, we're seeing more accessible options emerge, including cloud-based services with flexible pricing models. Small-scale businesses should carefully assess their needs, weigh the benefits, and explore cost-effective solutions.
Vicki, your article provides valuable insights into the potential benefits of ChatGPT in reliability engineering. I'm interested to know if there are any known real-world applications in the industry already. Any success stories so far?
Megan, there are indeed real-world applications of AI in reliability engineering. Some industries, like manufacturing and power generation, have started implementing AI-driven systems to enhance predictive maintenance, optimize operations, and improve reliability. While specific success stories may vary, the potential for significant improvements is there.
Sarah, thank you for your response. Indeed, various industries are beginning to embrace AI technologies to bolster reliability engineering efforts. These applications are still evolving, but there have been notable success stories, such as reduced downtime, improved asset management, and cost savings. It's an exciting time for reliability engineering!
Vicki, your article was informative. I appreciate the insights you shared. As the technology advances, do you think AI will completely redefine reliability engineering as we know it, or will it be more of an incremental evolution?
John, thank you for your question. While AI has the potential to revolutionize reliability engineering, I believe it will be more of an incremental evolution. AI will augment the capabilities of reliability engineers, helping them make better decisions and optimize processes. It will enhance and elevate the field, but human expertise will remain crucial.
Vicki, your article was well-written and compelling. I'm curious to know if ChatGPT can be used to analyze unstructured data sources, such as free-form text from customer feedback, to improve reliability engineering. Any thoughts on this?
Sophia, absolutely! ChatGPT's capability to analyze and process unstructured data, like customer feedback, can significantly benefit reliability engineering. By extracting valuable insights from these sources, engineers can identify recurring issues, track performance trends, and take proactive measures, ultimately enhancing the reliability of their systems.
Vicki, I found your article to be quite interesting. However, I wonder if there are any regulatory challenges when implementing AI technologies like ChatGPT in reliability engineering. Are there any compliance considerations to keep in mind?
Jonathan, regulatory challenges are indeed a consideration. Depending on the industry and location, there might be specific compliance requirements regarding data privacy, security, and ethical use of AI. Adhering to relevant regulations and maintaining transparency can help organizations navigate these challenges effectively.
Jennifer, you've raised an important aspect. Compliance with regulations should always be a priority when implementing AI technologies. Organizations must conduct a thorough assessment of applicable laws, industry standards, and ethical frameworks. Adherence to these guidelines ensures the responsible and trustworthy use of AI in reliability engineering.
Vicki, excellent article! I'm curious to know if there are any ongoing research efforts to further enhance ChatGPT specifically for reliability engineering. Any future advancements we can expect?
David, research and development efforts in the field of AI and reliability engineering are ongoing. Ongoing research aims to improve the accuracy, adaptability, and scalability of AI models for engineering tasks. We can expect future advancements that offer increased domain-specific knowledge and more robust decision-support capabilities.
Sarah, thank you for adding to the discussion. You're absolutely right. The research community is actively working on refining AI models for reliability engineering. Future advancements will likely focus on addressing domain-specific challenges, incorporating advanced analytics, and leveraging emerging technologies like machine learning and natural language processing.
Vicki, your article was insightful. I'm interested to know if there are any cost-effectiveness studies available that demonstrate the economic benefits of implementing ChatGPT in reliability engineering. Any references you can share?
Olivia, while I don't have specific studies at hand, there is growing evidence suggesting the cost-effectiveness of implementing AI technologies in reliability engineering. Reduced maintenance costs, increased operational efficiency, and minimized downtime are factors that contribute to potential economic benefits. Research papers and case studies exploring this area can provide further insights.
Vicki, your article hit on some key points. To take a step back, I'm curious to know what inspired you to explore and write about the intersection of reliability engineering and ChatGPT?
Daniel, great question. As an engineer, I've always been fascinated by the potential of AI-driven solutions in improving reliability and efficiency. The advancements in language models, like ChatGPT, prompted me to explore their application in reliability engineering. I believe it's an exciting area that can drive significant progress in the field.
Vicki, thank you for sharing your insights. Are there any other AI technologies or models, apart from ChatGPT, that hold promise in enhancing reliability engineering?
Sophia, alongside ChatGPT, there are several other AI technologies that can support reliability engineering. Machine learning algorithms, neural networks, and deep learning models have shown promise in various reliability-related tasks, such as anomaly detection, predictive maintenance, and failure analysis.
Jennifer, you're absolutely right. Other AI technologies like machine learning and deep learning have demonstrated their efficacy in reliability engineering. These models can leverage large datasets to extract patterns, identify potential failure points, and enable proactive maintenance strategies. The combination of various AI tools can unleash their full potential in enhancing reliability.
Vicki, your article highlighted the impact of ChatGPT in reliability engineering. I'd like to know if there are any specific industry sectors where ChatGPT has shown exceptional benefits so far?
Oliver, while Vicki can provide more insights, I've come across successful integration of ChatGPT in sectors like energy, aerospace, and manufacturing. These industries benefit from real-time analysis, predictive maintenance, and reliable decision-support systems. However, it's worth exploring potential applications in other sectors as well.
Mark, you're absolutely right. Sectors such as energy, aerospace, and manufacturing have embraced ChatGPT to reap the benefits of enhanced reliability engineering. However, as the technology continues to evolve, its applications can extend to various other industries, where reliability and efficiency are paramount.
Vicki, your article provided a comprehensive understanding of using ChatGPT in reliability engineering. However, can you shed light on the potential challenges of deploying and integrating such AI systems in existing engineering workflows?
Laura, integrating AI systems like ChatGPT in existing engineering workflows can present challenges. Engineering processes might need to be reconfigured to accommodate the technology, and initial training of the models can be time-consuming. Additionally, change management, user adoption, and providing necessary training to engineers are crucial for successful integration.
Laura, to add to Vicki's response, ensuring a smooth integration requires bridging the gap between AI technologies and domain knowledge. Collaboration between data scientists, reliability engineers, and domain experts is vital to gain valuable insights from AI models and effectively use them to optimize reliability engineering workflows.
Vicki, your article was an informative read. I'm curious to know about any ongoing challenges or areas of improvement for ChatGPT in the context of reliability engineering. Any thoughts on this?
Oliver, while ChatGPT has shown immense potential, there are still challenges to address. One area of improvement is ensuring ChatGPT's contextual understanding and domain specificity. Customization to reliability engineering requirements and fine-tuning can enhance its accuracy and relevance. Ongoing research and development efforts aim to overcome challenges and make the technology even more effective.
Vicki, your article was insightful. As we explore AI applications in reliability engineering, what considerations should organizations keep in mind while selecting an AI technology like ChatGPT?
George, organizations should consider a few key factors when selecting AI technologies. These include the model's reliability, accuracy, ease of integration, scalability, vendor support, and data privacy. Evaluating these aspects ensures that the chosen AI technology aligns with the organization's specific needs and reliability engineering objectives.
Jennifer, you've covered the important considerations well. Reliability, accuracy, and scalability are vital when selecting an AI technology like ChatGPT. Additionally, organizations should ensure the technology aligns with their existing infrastructure, data management practices, and supports their long-term reliability engineering goals.
Vicki, your article was insightful. Are there any industry standards or best practices emerging for the use of AI technologies in reliability engineering?
Megan, industry standards and best practices for AI in reliability engineering are still evolving. However, organizations can refer to established frameworks like the ISO 55000 series and the Industrial Internet Consortium's Industrial AI Safety Framework as foundational guidance. Collaboration between industry experts, researchers, and policymakers is driving the development of more specific best practices.
Sarah, thank you for your response. Indeed, the development of industry-wide best practices is an ongoing process. By actively participating in relevant forums, organizations can contribute to the creation of standards that promote the responsible and effective use of AI technologies in reliability engineering.
Vicki, your article was enlightening. Are there any known limitations when it comes to deploying ChatGPT in real-time and dynamic reliability engineering scenarios?
Emily, great question. One limitation is the potential latency in generating responses. Real-time scenarios might require prompt and near-instantaneous suggestions, which can be challenging for ChatGPT. Balancing response time with accuracy remains a focus area for further improvements in deploying the technology effectively in dynamic reliability engineering scenarios.
Expanding on Vicki's point, ensuring that ChatGPT responds accurately and adapts to dynamic scenarios is crucial. Real-time data updates, addressing unexpected events, and continuously evaluating the system's performance are essential for its successful deployment in dynamic reliability engineering contexts.
Vicki, thank you for sharing your insights. How can organizations effectively manage the potential risks and challenges associated with deploying AI technologies like ChatGPT in reliability engineering?
Thomas, effective risk management relies on a proactive approach. Organizations should establish protocols for continuous monitoring, analyzing system performance, and addressing potential biases or errors. Transparency, accountability, and regular audits are key to managing risks and ensuring responsible use of AI technologies in reliability engineering.
Mark, you've highlighted essential risk management practices. Organizations should also foster a culture of learning from mistakes, encouraging engineers to report issues, and providing channels for feedback and improvement. Collaboration between reliability engineers, data scientists, and stakeholders enables robust risk management and long-term success.
Vicki, your article was thought-provoking. As AI technologies like ChatGPT continue to evolve, do you think there will be more standardized platforms specifically tailored for reliability engineering in the future?
David, as AI technologies mature and become more prevalent in reliability engineering, we can expect platforms specifically tailored for the field to emerge. These platforms will likely offer reliability-specific features, integrations with industry-standard tools, and domain-specific customization options. Standardization can enhance collaboration and promote best practices within the reliability engineering community.
Vicki, your article sparked an interesting conversation. What are your thoughts on using AI technologies like ChatGPT for proactive reliability engineering rather than reactive approaches?
George, proactive reliability engineering is indeed a valuable approach. AI technologies like ChatGPT can play a crucial role in enabling proactive strategies by predicting potential failures, suggesting preventive measures, and analyzing trends. By leveraging AI for proactive reliability engineering, organizations can minimize downtime, reduce costs, and increase overall system reliability.
Vicki, great article! I'm curious to know if you have any recommendations on how organizations can effectively educate and train reliability engineers to work alongside ChatGPT?
Olivia, training reliability engineers to work alongside ChatGPT requires a multi-faceted approach. Organizations should provide comprehensive training on AI technologies, including their strengths, limitations, and ethical considerations. Collaborative sessions, workshops, and real-world use cases can help engineers understand how to effectively integrate ChatGPT within their workflow, leveraging its capabilities and validating its suggestions.
Jennifer, your recommendations are spot-on. Organizations should also encourage an environment of continuous learning and adaptation. Regular knowledge-sharing sessions, forums for exchanging experiences, and engaging engineers in ongoing improvements foster a culture where ChatGPT becomes an integral part of reliability engineering practices.
Vicki, your article opened up exciting possibilities for reliability engineering. Can ChatGPT be enhanced to handle visual data analysis along with text-based insights?
Paul, expanding ChatGPT's capabilities to handle visual data analysis is an exciting avenue for research and improvement. While ChatGPT primarily focuses on text-based insights, advancements in computer vision and multimodal learning could enable future versions of ChatGPT to integrate visual data and provide more comprehensive analyses for reliability engineering.
Vicki, your article was engaging. Are there any notable risks or challenges associated with relying too heavily on AI technologies like ChatGPT in reliability engineering?
Sophia, relying too heavily on AI technologies can introduce risks. Over-reliance might reduce human expertise, limit critical thinking, and create blind spots. It's crucial to strike a balance and use AI as a tool that augments human judgment and decision-making, leveraging the proficiency of engineers alongside the benefits of ChatGPT to achieve optimal results.
Vicki, your article provided valuable insights. In your opinion, what would be the key success factors for organizations adopting AI technologies like ChatGPT in reliability engineering?
Laura, key success factors include strong leadership support, collaboration between reliability engineers and data scientists, continuous learning and adaptation, and an organizational culture that embraces innovation and change. A holistic approach addressing technical, cultural, and management aspects ensures successful adoption and integration of AI technologies in reliability engineering.
Sarah, you've summed up the key success factors well. Open communication channels, clear goals, and effective change management are also vital. Organizations should foster an environment where engineers feel empowered and encouraged to contribute their expertise while effectively leveraging AI technologies like ChatGPT.
Vicki, your article was informative. I'm curious to know if there are any data management challenges organizations should consider when adopting AI technologies like ChatGPT.
David, adopting AI technologies like ChatGPT brings data management challenges. Organizations should ensure data quality, reliability, and availability for training and validating the model. Additionally, proper data governance, compliance with data privacy laws, and implementing effective data backup and recovery strategies are crucial for successful AI deployment in reliability engineering.
Vicki, your article provided valuable insights. Could you elaborate on how ChatGPT can assist in the creation of reliability engineering documentation and knowledge databases?
George, ChatGPT can be invaluable in creating reliable engineering documentation and knowledge databases. It can assist in generating technical reports, extracting insights from existing documentation, and offering real-time suggestions for improving documentation quality. By leveraging ChatGPT, engineers can streamline the process of capturing and disseminating knowledge effectively.
Jennifer, absolutely! ChatGPT's natural language processing capabilities make it an ideal tool for assisting in the creation of reliable engineering documentation. It can help automate workflows, aid in standardization, and contribute to the development of comprehensive knowledge databases, facilitating knowledge sharing and continuous improvement within reliability engineering teams.
Vicki, your article was fascinating. Are there any current limitations in hardware or infrastructure that organizations need to consider before implementing ChatGPT in reliability engineering?
Emily, hardware and infrastructure requirements are indeed factors to consider. ChatGPT's models can be resource-intensive and require significant computational power for training and deployment. Organizations should assess their existing infrastructure, computing capabilities, and scalability requirements to ensure that they can effectively support ChatGPT's hardware needs for seamless implementation in reliability engineering.
Vicki, your article shed light on an important topic. In your opinion, what are the key drivers behind the adoption of AI technologies like ChatGPT in reliability engineering?
Megan, several key drivers contribute to the adoption of AI technologies in reliability engineering. These include the need for enhanced operational efficiency, improved maintenance practices, increased asset reliability, and the growing availability of big data. The potential to reduce costs, optimize workflows, and gain a competitive edge also drives organizations to explore and adopt AI-driven solutions.
Sarah, you've captured the key drivers well. Reliability engineering is a critical aspect for organizations, and AI technologies like ChatGPT offer the potential to address existing challenges effectively, optimize resource allocation, and enable data-driven decision-making, ultimately leading to improved reliability and overall business performance.
Vicki, your article was enlightening. Are there any privacy concerns associated with implementing ChatGPT, considering it processes and analyzes sensitive engineering data?
John, privacy concerns are indeed important when implementing AI technologies. Organizations must prioritize data privacy and establish appropriate access controls and encryption during data handling and processing. Additionally, anonymizing sensitive data, implementing secure communication protocols, and adhering to relevant privacy regulations are crucial to protect sensitive engineering data when using ChatGPT.
Vicki, great article! What are your thoughts on the potential bias in AI models like ChatGPT and its impact on reliability engineering outcomes?
Sophia, potential bias in AI models is a significant concern. Biased training data or biased development can introduce unintended discrimination and impact reliability engineering outcomes. Organizations must subject AI models like ChatGPT to rigorous testing, validation, and continuous monitoring to identify and mitigate any biases that may arise. Transparency and diverse inputs during model development also help reduce bias.
Vicki, what kind of expertise would be required to effectively utilize ChatGPT in reliability engineering?
Sophia, a strong understanding of reliability engineering principles and data analysis would be beneficial. It's essential to possess the expertise to validate ChatGPT's recommendations and ensure they align with industry standards.
Vicki, regarding industry standards, do you think there is a need for ChatGPT-specific benchmarks or certifications in the field of reliability engineering?
Emma, it's an interesting point. With the growing adoption of AI in various industries, having benchmarks and certifications to ensure the reliability and accuracy of AI systems would be beneficial in reliability engineering as well.
Vicki, I completely agree. Standardized benchmarks and certifications would create a common framework for evaluating and comparing different AI systems used in reliability engineering.
Vicki, your article was thought-provoking. How can reliability engineers ensure that AI technologies like ChatGPT remain robust and reliable over time?
Laura, ensuring the robustness and reliability of AI technologies like ChatGPT requires constant monitoring and evaluation. Reliability engineers should engage in ongoing model validation, test its performance against real-world scenarios, and diligently address any identified issues. Frequent retraining with updated data, regular model performance assessments, and collaboration with data scientists are key to maintaining reliability and effectiveness over time.
Jennifer, you've provided excellent suggestions. Reliability engineers should also establish mechanisms for user feedback and incorporate user experiences to drive continuous improvements in ChatGPT's performance and reliability. This iterative approach fosters a feedback loop for learning and adaptation, making AI technologies more robust and aligned with reliability engineering needs.
Vicki, your article highlighted the potential of AI in reliability engineering effectively. What are the key factors organizations should consider while evaluating the suitability of ChatGPT for their specific reliability engineering needs?
Oliver, to evaluate ChatGPT's suitability, organizations should consider factors like their engineering domain, specific reliability challenges, available data sources, and desired outcomes. Training and deployment costs, AI model interpretability, and integration requirements should also be evaluated to ensure ChatGPT aligns with the organization's specific reliability engineering needs.
Mark, you've summarized the key evaluative factors well. Organizations must thoroughly assess their requirements, consider potential benefits and limitations, and conduct pilots or proof-of-concept experiments to validate ChatGPT's efficacy before full-scale integration. This holistic evaluation ensures that ChatGPT is suitably aligned with the organization's reliability engineering needs.
Vicki, your article was a great read. How do you foresee the future of reliability engineering being shaped by AI technologies like ChatGPT?
Thomas, AI technologies like ChatGPT hold immense potential to shape the future of reliability engineering. They will enable engineers to make data-driven decisions, optimize maintenance strategies, and enhance operational efficiency. While human expertise will remain invaluable, ChatGPT and similar AI tools will augment the capabilities of reliability engineers, leading to improved reliability, reduced costs, and increased customer satisfaction.
Vicki, your article provided significant insights. How can organizations ensure the responsible and ethical use of ChatGPT and other AI technologies in reliability engineering?
Daniel, ensuring responsible and ethical use of ChatGPT and AI technologies in reliability engineering requires a holistic approach. Organizations should establish clear guidelines and policies, conduct regular ethical reviews, and implement mechanisms for transparency and bias mitigation. Regular training and awareness programs, combined with ethical oversight, help foster a culture of responsible AI use within organizations.
Sarah, you've captured the core aspects of responsible AI use well. It's crucial to actively involve reliability engineers, data scientists, and other stakeholders in discussions around ethics, transparency, and accountability. By aligning AI technologies like ChatGPT with established ethical frameworks and industry best practices, organizations can ensure responsible deployment and maximize the positive impact on reliability engineering.
Vicki, your article was insightful. Do you think AI technologies like ChatGPT will become indispensable in reliability engineering, or will they remain complementary tools?
Olivia, I believe AI technologies like ChatGPT will become indispensable in reliability engineering. They will augment engineers' capabilities, enabling them to analyze vast amounts of data, gain actionable insights, and optimize decision-making processes. ChatGPT will become an essential tool, enhancing reliability and efficiency. However, it will always work alongside human expertise, making reliability engineering a collaborative field.
Vicki, thank you for your enlightening article and engaging in this discussion. It's fascinating to consider the potential impact of AI technologies like ChatGPT in reliability engineering. I look forward to witnessing the further advancements and real-world implementations in the future!
Michael, I'm glad you found the article valuable, and I appreciate your participation in the discussion. The potential of AI technologies like ChatGPT in reliability engineering is indeed thrilling. I share your excitement and look forward to witnessing how organizations harness the power of AI to drive revolutionary enhancements in reliability engineering. Thank you all for the fantastic discussion!
Thank you all for engaging with my article! I'm excited to hear your thoughts on using ChatGPT to enhance reliability engineering in technology.
Great article, Vicki! ChatGPT can definitely be a game-changer for reliability engineering. The ability to quickly analyze and troubleshoot potential issues using natural language processing is incredible.
I couldn't agree more, Mark! Traditional methods of reliability engineering often involve manual analysis and can be time-consuming. ChatGPT's automation capabilities could significantly improve efficiency.
Emily, you mentioned efficiency. How much time do you think ChatGPT can potentially save in the reliability engineering process?
Adam, it's hard to put an exact number on it. However, I believe ChatGPT can speed up repetitive tasks like log analysis and provide real-time recommendations, potentially reducing hours or even days of manual work.
That sounds promising, Emily. It would be interesting to see some real-world case studies where ChatGPT has been implemented in reliability engineering.
Adam, I've come across a case study where ChatGPT reduced the time spent on log analysis by approximately 60%. It's an impressive improvement!
Emily, that's impressive! It clearly shows the tangible benefits ChatGPT can bring to the table. Thanks for sharing the case study.
Emily, thanks for the insights. The potential time savings and real-time recommendations offered by ChatGPT in reliability engineering are truly exciting!
While the concept is interesting, I wonder about the potential limitations of relying too heavily on an AI system like ChatGPT. How reliable can it be when dealing with complex technology systems?
Valid concern, Alex. While ChatGPT can provide valuable insights, it should be used as a tool alongside human expertise. Reliability engineering will still require a human touch to ensure accuracy.
Alex, while there might be limitations, it's important to remember that AI systems continue to improve with time and new developments. ChatGPT might be a stepping stone towards even more advanced technologies.
Exactly, Alex. We must acknowledge the current limitations while also understanding the potential and continuous advancements in AI technology like ChatGPT.
Julia, I agree. AI systems have come a long way, and we should embrace their potential while being cautious about their limitations in critical domains like reliability engineering.
Alex, embracing AI while exercising caution is definitely the way to go. We need to strike a balance to leverage the technology's benefits without compromising safety and reliability.
Alex, it's important for reliability engineers to understand the underlying workings of ChatGPT and its limitations to make informed decisions and maintain accountability.
Sophia, absolutely. Reliability engineers need to stay up-to-date with the advancements in AI and continually learn to effectively utilize these tools.
Alex, continuous learning and adaptation to ever-evolving AI technologies are vital for reliability engineering professionals to enhance their skills and remain valuable in the industry.
Sophia, as AI systems become more prevalent in reliability engineering, it would also be essential to incorporate related ethical considerations and guidelines into the profession.
Sophia, continuous learning is crucial, not just for reliability engineers but also for AI systems like ChatGPT. Regular updates and improvements will help keep it aligned with evolving industry needs.
I've had experience working with ChatGPT, and it has been quite useful in identifying patterns and anomalies in system logs. However, it is important to validate its suggestions before taking action.
I can see ChatGPT being beneficial for preventive maintenance in technology. It could assist in predicting potential failures, helping companies take proactive measures and avoid costly downtime.
Absolutely, Sarah! Predictive maintenance is a key area where ChatGPT can shine. With its ability to analyze data and detect patterns, it can provide valuable insights for avoiding unplanned outages.
Vicki, do you have any suggestions for integrating ChatGPT into existing reliability engineering processes? Any best practices?
Good question, Liam! It's crucial to start with a small-scale proof of concept, identify specific use cases, and gradually expand implementation. Additionally, continuous monitoring and evaluation will help ensure the system's accuracy and effectiveness.
Vicki, continuous monitoring and evaluation make sense. It ensures that any issues or inaccuracies in ChatGPT's recommendations can be identified and rectified promptly.
Vicki, by involving stakeholders and relevant experts in the evaluation process, it would be easier to make informed decisions about the effectiveness and potential risks of ChatGPT in reliability engineering.
Liam, you're right. A collaborative approach involving all stakeholders would help in addressing concerns, managing expectations, and ensuring successful integration of ChatGPT into existing reliability engineering processes.
Emma, collaborative approaches that involve diverse perspectives can contribute to the responsible and effective utilization of AI in reliability engineering.
Olivia, collaboration fosters innovation and allows for the development of reliable and safe AI systems that can truly benefit the reliability engineering field.
Olivia, involving a diverse range of professionals will help ensure that AI systems like ChatGPT in reliability engineering serve the needs of different perspectives and avoid biases.
Emma, involving stakeholders from different departments and considering their requirements will help ensure that ChatGPT in reliability engineering aligns with broader organizational goals.
Vicki, continuous monitoring and evaluation can also help identify any bias or potential ethical concerns that may arise with ChatGPT's recommendations. Ethical considerations are critical.
Sarah, what challenges do you foresee in implementing ChatGPT for preventive maintenance?
Oliver, one challenge would be collecting and structuring enough relevant data for ChatGPT's training. It requires historical maintenance records, failure data, etc. Also, getting buy-in from the engineering team might take some effort.
Sarah, how scalable do you think the implementation of ChatGPT for preventive maintenance can be?
Oliver, scalability depends on factors like data availability, computational resources, and the complexity of the systems being monitored. It would require careful planning and infrastructure to ensure seamless scalability.
Sarah, on the positive side, once the initial effort of data collection and system integration is done, using ChatGPT for preventive maintenance could result in significant cost savings and increased system uptime.
James, you're absolutely right. The initial investment in implementing ChatGPT for preventive maintenance can lead to long-term savings and enhance the overall reliability of technology systems.
Sarah, in addition to cost savings, preventive maintenance can have a positive impact on customer satisfaction by minimizing disruptions and ensuring smooth operation of technology systems.
James, exactly. Keeping technology systems running smoothly and minimizing any negative impact on end-users is crucial for building trust and loyalty.
Sarah, scalability is indeed an important aspect. Technology systems vary in size and complexity, and ChatGPT's implementation should be adaptable to different scales for effective preventive maintenance.
Oliver, scalability can lead to wider adoption of ChatGPT in preventive maintenance, driving industry-wide improvements in technology reliability and performance.
ChatGPT could also be useful in knowledge sharing among reliability engineers. The system could store and retrieve past solutions, making it easier to learn from previous experiences.
Michael, with ChatGPT's knowledge storage abilities, reliability engineers can focus more on complex issues and problem-solving rather than spending time searching for past solutions.