Enhancing Report Generation in Confocal Microscopy: Leveraging ChatGPT for Unparalleled Accuracy and Efficiency
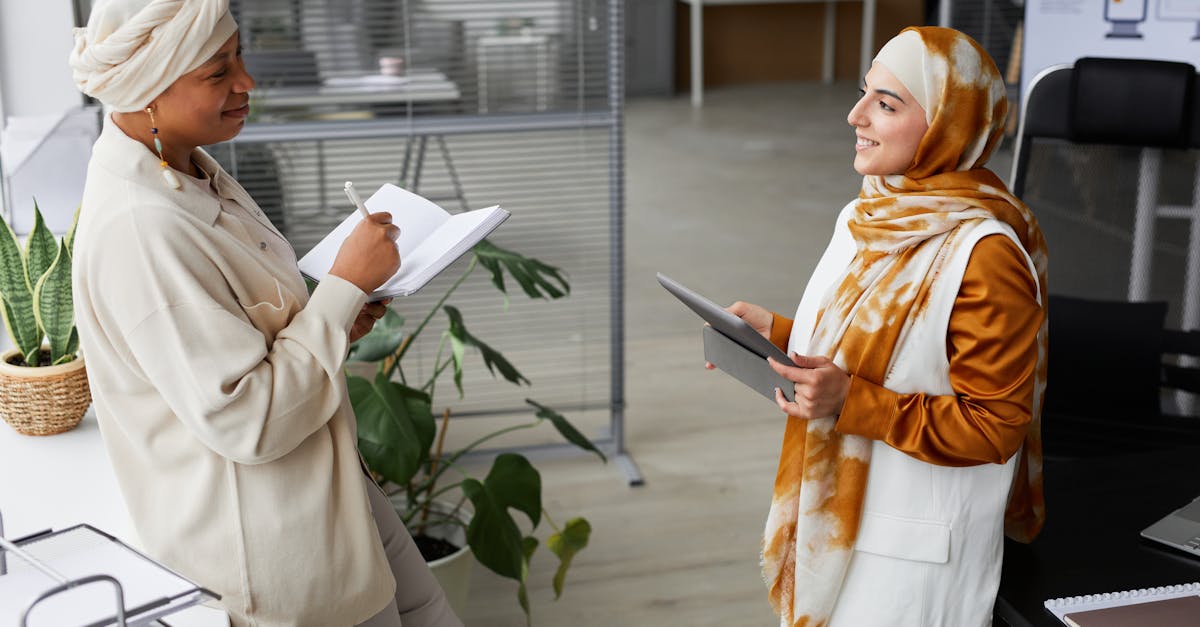
Confocal microscopy is a powerful imaging technique used in various scientific fields to visualize and analyze three-dimensional structures of samples. Its ability to provide high-resolution images has revolutionized the way researchers observe and study biological specimens, leading to numerous insights and breakthroughs.
While confocal microscopy has traditionally required manual analysis and interpretation of acquired images, recent advancements in artificial intelligence (AI) have enabled the automatic generation of reports based on experimental observations. This technological integration has significantly enhanced the efficiency and accuracy of data interpretation, allowing researchers to focus more on data analysis and scientific discovery.
How does it work?
Confocal microscopy captures images by illuminating samples with a laser beam and detecting the emitted fluorescent light. The generated images consist of multiple slices or planes that can be reconstructed into a three-dimensional representation of the sample. These images contain valuable information about the structure, morphology, and localization of specific molecules within the sample.
Artificial intelligence algorithms can be trained to recognize and analyze specific structures or patterns within confocal microscopy images. This involves training the AI model on a large dataset of annotated images, where experts have manually identified and labeled the features of interest. The AI model learns to identify these features and can subsequently generate reports summarizing the observed structures and their characteristics.
Advantages of AI-generated reports
The use of AI in report generation for confocal microscopy offers several advantages:
- Improved efficiency: AI algorithms can rapidly analyze large volumes of confocal microscopy images and generate reports in a matter of seconds. This eliminates the need for manual analysis, saving researchers significant time and effort.
- Consistency and accuracy: AI algorithms can provide consistent and objective analysis, reducing the potential for human error and subjectivity in report generation. This ensures reliable and reproducible results for further analysis and comparison.
- Enhanced data interpretation: AI-generated reports can provide detailed information on the observed structures, such as their size, shape, and distribution. This facilitates better data interpretation and aids in identifying significant findings that might have otherwise been overlooked.
- Facilitates collaboration: AI-generated reports can be easily shared and accessed by multiple researchers, promoting collaboration and enabling the exchange of knowledge and insights. This enhances scientific progress and fosters interdisciplinary research.
Future prospects
The integration of artificial intelligence in confocal microscopy report generation is continuously evolving. Ongoing research aims to further improve the accuracy and capabilities of AI algorithms in analyzing and interpreting confocal microscopy images. This includes advancements in image segmentation, pattern recognition, and machine learning techniques.
Furthermore, the development of AI models capable of learning from unlabeled data sets opens up exciting possibilities for unsupervised analysis of confocal microscopy images. These models can automatically identify and classify structures of interest, even without prior annotation, potentially uncovering novel findings or unexpected relationships.
As AI technology continues to advance, the generation of automatic reports for confocal microscopy will become more sophisticated, enabling researchers to extract deeper insights from complex imaging data. This will undoubtedly revolutionize the field of microscopy and accelerate scientific discoveries in various disciplines.
Comments:
Thank you all for taking the time to read my article on enhancing report generation in confocal microscopy with ChatGPT!
Great article, Daniel! I found it very informative and well-researched. ChatGPT seems like a promising tool for improving accuracy and efficiency in report generation.
I agree, Jonathan! The potential of leveraging chat-based models like ChatGPT for scientific purposes is exciting. I can see it being a game-changer in the field of microscopy.
Absolutely, Laura! The combination of automated report generation and the accuracy offered by ChatGPT can save researchers a significant amount of time and effort.
Indeed, Laura. The level of accuracy and efficiency provided by ChatGPT can streamline research and accelerate discoveries in the field of microscopy.
Absolutely, Michael! The time saved through automated report generation can be better utilized in analyzing results and conducting further experiments.
You're right, Sophia. Researchers can focus more on the interpretation and implications of their findings rather than spending excessive time on writing detailed reports.
That's true, Jonathan! Researchers can focus on the bigger picture and explore new avenues of research rather than getting bogged down in routine report writing.
Absolutely, Sophia! ChatGPT can be a valuable asset in empowering researchers to focus on their core work while still generating high-quality reports efficiently.
David, I'm glad to see that ChatGPT's potential for enhancing report generation in microscopy is being recognized. It can be a significant step forward in our workflows.
Indeed, Sarah! The integration of cutting-edge AI technologies like ChatGPT can have a profound impact on various scientific workflows, including microscopy and report generation.
Thank you, Daniel! I'll definitely reach out if I need any assistance on implementing ChatGPT in my microscopy reports.
As a confocal microscopy user, this article caught my attention. It's fascinating how AI technologies can enhance our workflow. I'm curious to know how ChatGPT handles complex microscopy data.
That's a great question, Sarah! ChatGPT can handle complex microscopy data by training on a large amount of labeled examples. It learns patterns and correlations, allowing it to generate accurate reports based on the input data.
Nice work, Daniel! It's impressive how ChatGPT can improve the accuracy of report generation. This could be a useful tool for both researchers and clinicians in the field of microscopy.
Thanks, Daniel! It's reassuring to know that ChatGPT can handle complex data. I'll definitely explore its potential in improving my microscopy reports.
You're welcome, Sarah! Feel free to reach out if you have any more questions regarding ChatGPT and its application in microscopy.
I appreciate your offer, Daniel. I'll keep that in mind and surely reach out if I need any assistance with ChatGPT implementation.
Sarah, as someone experienced in microscopy, I believe ChatGPT can be a valuable tool in generating precise and accurate reports. It could potentially reduce the chances of overlooking important details.
I completely agree, Alexandra. Having a tool like ChatGPT that is trained to pay attention to details can greatly enhance the accuracy of generated reports.
Sarah, using ChatGPT for report generation can enhance the quality and precision of outputs, leading to more reliable and reproducible research in confocal microscopy.
You're welcome, Sarah! Feel free to reach out anytime. I'm here to assist you in leveraging ChatGPT for your microscopy reports.
I can definitely see the advantages of using ChatGPT for report generation in confocal microscopy. It could potentially reduce human error and provide more consistency in the generated reports.
The potential to leverage AI in microscopy is exciting, but I wonder if there are any limitations to be aware of when using ChatGPT?
That's an important consideration, Emily. While ChatGPT has shown impressive capabilities, it may sometimes generate outputs that seem plausible but are incorrect. It's crucial to validate the generated reports thoroughly to ensure accuracy.
Thank you for highlighting the need for validation, Daniel. The automated aspect of report generation is indeed beneficial, but it shouldn't replace thorough inspection by domain experts.
Exactly, Emily! Human expertise and validation are crucial to ensuring the accuracy and reliability of any AI-generated reports, including those produced with ChatGPT.
Absolutely, Daniel! Human expertise adds a crucial layer of judgment and intuition, especially when dealing with unique or challenging cases that may not have been encountered during training.
That's a crucial point, Daniel. AI tools like ChatGPT should always be seen as an augmentation to human expertise, not a complete replacement.
Exactly, Emily! Collaborating with researchers and domain experts can help fine-tune AI models like ChatGPT to handle even the most challenging cases with higher accuracy.
Well said, Emily! The synergy between AI and human expertise is key to achieving the best possible outcomes in scientific research and report generation.
Absolutely, Laura and Jonathan! The collaboration between researchers, AI experts, and developers is crucial for harnessing the true power of AI in scientific domains.
I wonder how ChatGPT compares to other AI models in terms of accuracy. Has it achieved state-of-the-art results for report generation?
Good question, Rachel! While ChatGPT has shown impressive accuracy, it's important to note that it's still a challenging task. It's constantly improving, but achieving state-of-the-art results requires ongoing research and development.
Thanks for the insight, Daniel! It's understandable that achieving state-of-the-art results requires continuous research and development in this field.
I can see how using ChatGPT can save time and effort, but how does it handle rare or unique cases where there might not be enough training data?
Excellent question, Oliver. While rare or unique cases may pose a challenge initially, ChatGPT can still provide reasonable outputs based on the patterns it has learned from the available data. Continued training and exposure to additional cases can further improve its handling of such scenarios.
Thanks for addressing my concern, Daniel! It's good to know that ChatGPT can adapt even in cases where there might be a lack of training data for specific scenarios.
Oliver, while rare cases may present a challenge initially, one possible approach is to iteratively improve the model's performance by incorporating additional training data or fine-tuning the model based on domain experts' feedback.
I appreciate your response, Daniel. It is reassuring to know that ChatGPT has room for adaptation and improvement.
Validation by experts plays an essential role in ensuring the accuracy of generated reports. It's reassuring to see that ChatGPT acknowledges this need.
Indeed, Rachel! While AI models like ChatGPT can automate and improve the generation process, human experts' guidance and validation ensure the highest level of quality.
Absolutely, Emily! The collective efforts of AI and human expertise can unlock the true potential of scientific advancements while maintaining reliable and accurate reports.
I couldn't agree more, Rachel and Emily! The collaboration between AI and human experts is crucial for the successful integration of technology in scientific research.
Exactly, Emily! AI tools are meant to assist and augment our work, ultimately leading to higher quality outcomes.
Collaboration is the key to success when it comes to AI-assisted report generation. Researchers and AI experts can learn from each other and achieve remarkable results.
Well said, Laura! The synergy between the expertise of researchers and the capabilities of AI models like ChatGPT holds tremendous potential for advancing scientific research.
The collective knowledge and expertise of different domains coming together can unlock new possibilities and significantly improve various scientific endeavors.
Absolutely, Jonathan! The interdisciplinary collaboration between different fields can lead to breakthroughs that otherwise might not have been possible.
Well said, Jonathan and Laura! Synergistic collaborations are at the core of scientific advancements, and they can drive transformative outcomes.