Enhancing Retention Management: Leveraging ChatGPT for Churn Prediction
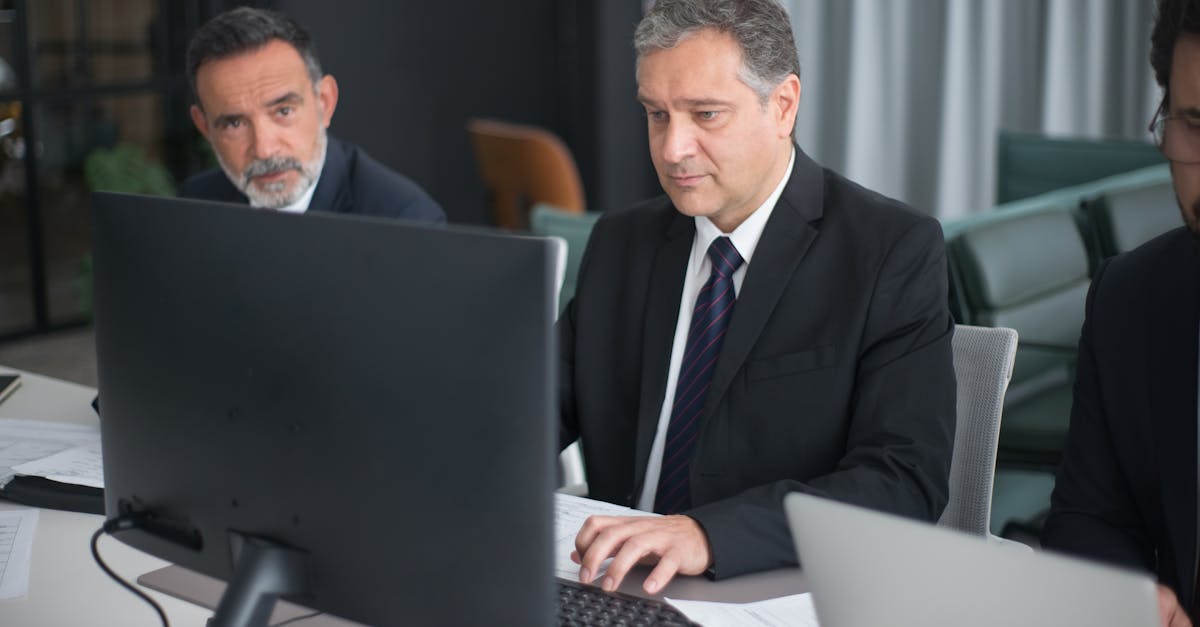
Retaining customers and employees is crucial for the success of any organization. High churn rates can significantly affect the growth and profitability of a company. To mitigate the risks associated with customer and employee churn, retention management techniques have become essential in various industries. One such advanced technique is churn prediction using text analysis.
Understanding Churn Prediction
Churn prediction is the process of identifying customers or employees who are likely to discontinue their relationship with a company. By analyzing historical data and identifying patterns, organizations can take proactive measures to retain individuals who exhibit signs of churn.
The Role of Text Analysis
Text analysis involves the extraction of meaningful information from written or spoken text using natural language processing (NLP) techniques. By applying text analysis algorithms to customer or employee feedback, organizations can uncover valuable insights into sentiments, opinions, and intentions.
Predicting Customer Churn
Customer sentiments play a crucial role in predicting churn. By analyzing textual data such as customer reviews, support tickets, or social media interactions, organizations can identify customers who are dissatisfied, frustrated, or unhappy with their experiences. Text analysis algorithms can analyze the language, tone, and context of customer communication to detect their sentiments.
For example, if a customer repeatedly mentions negative experiences or uses words associated with disappointment, these signals indicate a higher likelihood of churn. By aggregating and analyzing such sentiment data, organizations can identify customers who require immediate attention and devise retention strategies accordingly.
Predicting Employee Churn
Employee sentiments also offer valuable insights into churn prediction. By analyzing textual data from sources such as employee surveys, performance reviews, or internal communication platforms, organizations can identify employees who are dissatisfied, disengaged, or considering leaving the company.
Text analysis algorithms can detect patterns such as negative sentiment, complaints, or specific keywords associated with job dissatisfaction. By leveraging these insights, organizations can address the concerns of disengaged employees, offer relevant support and training, and implement initiatives to improve employee satisfaction and reduce churn.
Benefits of Churn Prediction with Text Analysis
Utilizing text analysis for churn prediction offers several benefits to organizations:
- Early identification: By analyzing sentiments in real-time, organizations can identify potential churners at an early stage and take preventive actions to retain these individuals.
- Accuracy: Text analysis algorithms can uncover subtle sentiments, nuances, and intentions that may not be easily detectable through traditional methods, improving the accuracy of churn prediction.
- Targeted retention strategies: By understanding the underlying reasons for churn, organizations can tailor their retention strategies based on the specific needs and concerns of individuals at risk of churn.
- Cost savings: By proactively addressing churn, organizations can save significant costs associated with customer or employee acquisition and training.
- Improved customer and employee satisfaction: By resolving issues identified through text analysis, organizations can enhance the overall satisfaction and loyalty of their customers and employees.
Conclusion
Retention management is a critical aspect of organizational success, and churn prediction using text analysis offers a powerful tool to anticipate and prevent customer and employee churn. By leveraging the insights obtained through text analysis, organizations can implement targeted retention strategies, reduce costs, and improve overall satisfaction levels, ultimately enhancing their growth and profitability.
Comments:
Thank you all for taking the time to read my article on enhancing retention management with ChatGPT for churn prediction.
Great article, Christian! ChatGPT seems like a promising tool for churn prediction. Have you personally used it in any real-world scenarios?
Thanks, Mark! Yes, I've had the opportunity to implement ChatGPT for churn prediction in a couple of projects. It showed promising results in predicting churn early on. However, I'd love to hear if others have had similar experiences.
Mark, I've also used ChatGPT for churn prediction in a real-world scenario. It proved to be effective in identifying potential churners. However, I did face some challenges during the implementation.
Thanks for sharing your experience, Nathan! What were the challenges you encountered when implementing ChatGPT for churn prediction?
Christian, I appreciate the insights shared in the article. It's fascinating how AI can help address challenges in churn prediction. Have you found any limitations or challenges when using ChatGPT specifically for this purpose?
Thank you, Samantha! One challenge with ChatGPT for churn prediction is that it heavily relies on the quality and relevance of input data. Garbage in, garbage out, as they say. Alongside data quality, model interpretability can also be an issue. Nonetheless, it offers valuable predictions.
Samantha, while ChatGPT is a powerful tool for churn prediction, it's crucial to address potential biases in the data and ensure a fair assessment of customer churn. Bias in data can affect the accuracy of predictions and lead to unfair actions.
You're absolutely right, Emma. Bias in data can perpetuate existing inequalities and have adverse effects. It's essential to regularly evaluate and mitigate biases within the modeling process.
Christian, I found your article very informative. Do you think ChatGPT can effectively predict churn across different industries, or are there certain types of businesses where it might be less suitable?
Thanks, Luke! While ChatGPT can be applied to various industries, the effectiveness may vary depending on the specific business context. It's crucial to fine-tune the model on relevant data to enhance its performance for a particular industry.
Luke, as someone working in the telecommunications industry, I believe ChatGPT can be highly effective for churn prediction. However, it would require the model to be trained on industry-specific data to capture the unique dynamics.
Thanks for sharing your perspective, William. You're right, industry-specific training can significantly enhance the accuracy and applicability of ChatGPT for churn prediction.
The potential of ChatGPT for churn prediction is intriguing, Christian. What other use cases do you think this technology can be applied to?
Great question, Emily! Apart from churn prediction, I believe ChatGPT can be applied to customer support automation, sentiment analysis, recommender systems, and personalized marketing, among others.
Emily, apart from churn prediction, ChatGPT can be beneficial for automating customer inquiries, generating personalized recommendations, and even creating natural-language chatbots for customer support.
Grace, you're spot on! ChatGPT's natural language processing capabilities can be leveraged for various customer-centric use cases, enhancing customer experiences and optimizing business processes.
I enjoyed reading your article, Christian. How does ChatGPT compare to other churn prediction methods in terms of accuracy and efficiency?
Thanks, Christopher! ChatGPT can offer competitive accuracy but may require more computational resources and time for training compared to traditional machine learning methods. Its versatility and ability to capture nuanced patterns are its strong points.
Christopher, while traditional methods often provide reliable churn prediction, ChatGPT offers the advantage of understanding and capturing more complex patterns and subtle customer behavior, especially in instances where manual feature engineering may fall short.
Great point, Ava! ChatGPT's ability to learn from large amounts of unlabeled data and recognize complex patterns can indeed provide valuable insights for churn prediction, surpassing traditional methods in certain scenarios.
Christian, appreciate the article! Regarding data privacy and confidentiality, what steps should businesses take when implementing ChatGPT for churn prediction to ensure customer information is secure?
Thank you, Rachel! When implementing ChatGPT or any AI system, businesses should prioritize data anonymization, encryption, and strict access controls. Implementing privacy-preserving techniques and complying with relevant regulations is crucial.
Rachel, businesses should also consider conducting regular audits to ensure compliance with privacy regulations and take steps to educate employees involved in implementing ChatGPT about data privacy and security best practices.
You're absolutely right, Isabella. Building a culture of privacy and security awareness within organizations is crucial when deploying AI models like ChatGPT.
Christian, your article has sparked my interest in ChatGPT. Are there any specific tools or frameworks you recommend for implementing ChatGPT for churn prediction?
I'm glad to hear that, Alex! OpenAI's GPT-3 models, including ChatGPT, can be implemented using frameworks like TensorFlow, PyTorch, or OpenAI's own libraries. These provide convenient APIs for integrating ChatGPT into your churn prediction pipeline.
Thank you, Christian, for the information! I'll definitely explore using ChatGPT in my churn prediction project, and I'll reach out if I have any questions.
You're welcome, Alex! I'm glad the information was helpful. Feel free to reach out anytime if you need any assistance or guidance during your churn prediction project.
Christian, excellent article! How can businesses combine ChatGPT with existing churn management strategies to improve overall retention rates?
Thank you, Olivia! Combining ChatGPT with existing churn management strategies can be beneficial. Businesses can leverage the predictions from ChatGPT to identify at-risk customers and then take targeted retention actions, such as personalized offers or proactive customer support, to improve retention rates.
Olivia, combining ChatGPT with existing churn management strategies allows businesses to leverage the model's predictions while still benefiting from previously established retention tactics. It's a win-win situation.
Indeed, Aiden. The integration of AI models like ChatGPT with existing strategies can lead to more comprehensive and effective churn management approaches, ultimately improving overall customer satisfaction and retention rates.
Aiden, by combining existing strategies with ChatGPT's predictions, businesses can implement personalized retention measures at scale, catering to individual customer needs more effectively.
Absolutely, Ava! The ability to personalize retention efforts based on predictions helps create a more customer-centric approach, which can significantly enhance retention rates.
Great point, Ava! The combination of personalized predictions from ChatGPT and well-crafted retention measures can greatly enhance customer experience and loyalty.
Christian, as someone who oversees customer success, I find this article valuable. How frequently do you recommend retraining ChatGPT for churn prediction to ensure accurate predictions?
I appreciate your feedback, Daniel! The frequency of retraining ChatGPT depends on the dynamic nature of your business and the availability of new relevant data. A good practice is to periodically retrain the model to incorporate the latest patterns and changes in customer behavior.
Daniel, the frequency of retraining should also be determined by the rate of drift in customer behavior patterns. Changes in customer preferences and market dynamics necessitate periodic adaptation of the prediction model, ensuring it remains accurate.
Very true, Ryan! Adapting the churn prediction model as customer behavior evolves is vital to maintain its predictive power.
Christian, I enjoyed your article. Do you foresee any ethical considerations when implementing ChatGPT for churn prediction?
Thanks, Sophia! Ethical considerations should indeed be taken seriously. Businesses should ensure that AI systems are deployed responsibly, without bias, and adhere to ethical guidelines to avoid any inadvertent negative impacts on customers or any undue biases in predictions.
Sophia, ethical considerations are indeed crucial. It's necessary to evaluate potential biases, ensure fairness, and transparently communicate to customers when AI models like ChatGPT are utilized in churn prediction.
Absolutely, Grace! Maintaining transparency, customer trust, and using AI responsibly should always be at the forefront of any implementation involving AI models like ChatGPT.
Christian, great article! Have you encountered any common misconceptions about ChatGPT for churn prediction that you'd like to address?
Thank you, Liam! One common misconception is that ChatGPT can magically predict churn without proper data and model configuration. It's important to set realistic expectations and understand that ChatGPT is a powerful tool but needs careful implementation.
Liam, one misconception is that ChatGPT can fully replace human involvement in churn prediction. While it's an invaluable tool, human expertise is still necessary to interpret and translate predictions into effective retention strategies.
Very true, Emma! Human judgment and domain knowledge should always complement AI-powered churn prediction to ensure the most optimal decision-making and improve overall customer retention.
Well said, Emma! The collaboration between AI and human expertise can lead to highly effective retention strategies, combining the power of AI predictions with the nuanced decision-making capabilities of human professionals.
Christian, your article provides valuable insights. Can ChatGPT be used for proactive churn prevention, or is it mainly focused on predicting churn once it's likely to happen?
I'm glad you found it valuable, Ella! ChatGPT can be useful for both proactive churn prevention and predicting churn once it's likely to happen. By identifying early signals for churn, businesses can take preventive actions to retain at-risk customers.
The primary challenge was obtaining accurate and relevant data for training the model. Additionally, the interpretability of the model's predictions was a concern, as stakeholders required transparency in the decision-making process.
Nathan, I also faced challenges in securing relevant data for training ChatGPT. Finding labeled churn data that accurately represents real-world scenarios can be a daunting task.
Indeed, William! Data collection and labeling can be time-consuming and resource-intensive. However, with careful planning and collaboration between data scientists and domain experts, it's possible to overcome these challenges.
Indeed, William! Collecting and labeling relevant training data require careful planning and domain expertise. Collaboration across teams is essential to gather high-quality data representative of real-world scenarios.
Thank you all for your valuable comments and insights! I'm thrilled to see the discussion around the potential and challenges of leveraging ChatGPT for churn prediction. Feel free to ask more questions or share additional thoughts.
Absolutely, Christian! Data privacy and security should be at the forefront of AI implementation discussions. It's essential to strike a balance between innovation and safeguarding customer trust.
That's an excellent point, Joshua! Finding the right balance between innovation, accuracy, and privacy is key to successful implementation and long-term customer satisfaction.
Thank you all once again for your participation in this discussion. It was insightful and engaging! Feel free to reach out to me if you have any further questions or thoughts.
Correcting a typo in my previous reply: Thank you all once again for your participation in this discussion. It was insightful and engaging! Feel free to reach out to me if you have any further questions or thoughts.
Thank you all for your valuable comments and insights! The discussion around leveraging ChatGPT for churn prediction was informative and engaging. Feel free to reach out if you have any further questions or thoughts.
Correcting a typo in my previous reply: Thank you all for your valuable comments and insights! The discussion around leveraging ChatGPT for churn prediction was informative and engaging. Feel free to reach out if you have any further questions or thoughts.