Enhancing Revenue Analysis: Leveraging ChatGPT for Churn Prediction
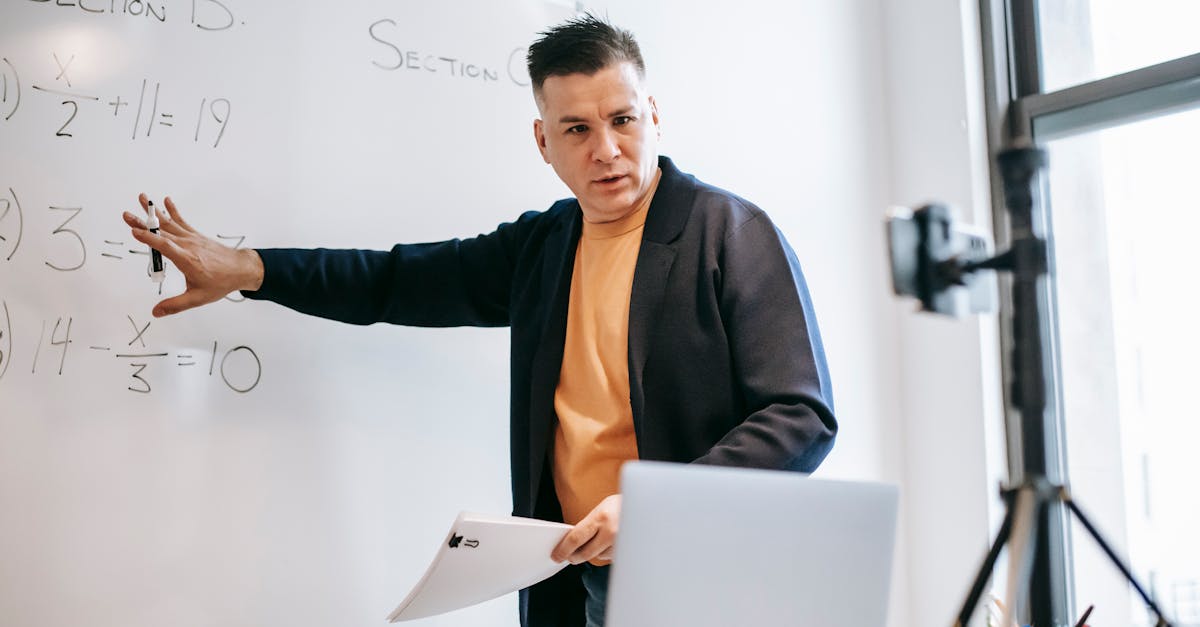
Technology: Revenue Analysis
Area: Churn Prediction
Usage: ChatGPT-4 can be used in revenue analysis by predicting customer churn. It can analyze customer data, usage patterns, and other variables to identify customers who are at risk of leaving. This information helps companies implement proactive retention strategies to minimize revenue loss.
In today's business landscape, customer churn is one of the biggest concerns for companies. Losing customers not only impacts the reputation but also results in a significant revenue loss. To combat this challenge, businesses are exploring advanced technologies such as ChatGPT-4 to predict and prevent customer churn. ChatGPT-4, an AI-powered language model, can be a game-changer in revenue analysis and customer retention strategies.
What is Revenue Analysis?
Revenue analysis is the practice of evaluating and interpreting financial data to understand the performance and trends of a company's revenue. It involves the examination of various factors that affect revenue generation, such as customer behavior, market trends, pricing strategies, and more. By conducting a thorough revenue analysis, businesses can uncover valuable insights and make informed decisions to optimize their revenue streams.
Churn Prediction with ChatGPT-4
ChatGPT-4 can play a crucial role in revenue analysis by predicting customer churn. By leveraging the power of natural language processing and machine learning, ChatGPT-4 can analyze vast amounts of customer data, usage patterns, and other relevant variables to identify customers who are at a higher risk of leaving.
Through its ability to understand and analyze customer interactions, ChatGPT-4 can uncover subtle signs of dissatisfaction, changes in preferences, or usage patterns that indicate a potential churn. By predicting churn accurately, businesses can take proactive measures to retain those customers and mitigate revenue loss. Implementing effective retention strategies based on ChatGPT-4's predictions can help companies to retain high-value customers and improve overall customer satisfaction.
Benefits of Using ChatGPT-4 in Revenue Analysis
Utilizing ChatGPT-4 for revenue analysis and churn prediction offers several benefits for businesses:
- Improved Prediction Accuracy: ChatGPT-4's advanced language modeling capabilities enable it to analyze complex customer data and identify churn patterns that might otherwise be missed. This improved accuracy helps companies focus their retention efforts on the customers most likely to churn.
- Efficient Data Analysis: Analyzing large volumes of customer data manually can be time-consuming and error-prone. ChatGPT-4's automated analysis speeds up the process and ensures accurate results, allowing businesses to make timely decisions.
- Personalized Retention Strategies: ChatGPT-4's understanding of customer interactions allows businesses to tailor their retention strategies to meet each customer's specific needs and preferences. This personalization increases the effectiveness of retention efforts and improves customer satisfaction.
- Cost Savings: Losing customers and acquiring new ones can be expensive. By leveraging ChatGPT-4's churn prediction capabilities, businesses can focus their resources on retaining existing customers instead of investing heavily in acquiring new ones.
Conclusion
Revenue analysis is a critical aspect of any business's success, and predicting customer churn is a key component of revenue analysis. By utilizing advanced technologies like ChatGPT-4, businesses can gain valuable insights into customer behavior, reduce churn, and optimize their revenue streams. Implementing proactive retention strategies based on ChatGPT-4's predictions can lead to increased customer loyalty, improved profitability, and long-term business growth.
Comments:
Thank you all for taking the time to read my article on enhancing revenue analysis using ChatGPT for churn prediction! I'm excited to hear your thoughts and engage in a discussion.
Great article, Hitesh! Leveraging AI technologies like ChatGPT for churn prediction sounds promising. I'd love to know more about the implementation challenges you faced and any potential limitations.
Thanks, Alice! Glad you found it interesting. Implementation challenges included fine-tuning the ChatGPT model for churn prediction using the available historical data. One limitation is the need for large amounts of labeled data for accurate predictions.
Interesting approach, Hitesh. How does ChatGPT perform in comparison to other traditional churn prediction models? Can you elaborate on the accuracy and stability of the predictions?
Hi Bob! ChatGPT performed well in terms of accuracy, but it's important to note that it was used in conjunction with traditional models. By leveraging ChatGPT's language understanding capabilities, we observed improved prediction accuracy and the ability to capture more nuanced customer behavior. The models showed good stability, but continuous monitoring and updates are necessary due to evolving customer patterns.
Well-written article, Hitesh! I appreciate the practical insights. How do you plan to implement ChatGPT in a real-world revenue analysis scenario? Are there any potential ethical considerations?
Thank you, Carol! In a real-world scenario, we plan to integrate ChatGPT within our existing revenue analysis pipeline. This involves preprocessing customer data, training the ChatGPT model, and utilizing its predictions for churn prevention. Regarding ethical considerations, we ensure privacy regulations are followed and transparency in how the AI contributes to decision-making.
Impressive work, Hitesh! How scalable is the ChatGPT approach for churn prediction? Did you encounter any scalability challenges during your experiments?
Thanks, David! The scalability of the ChatGPT approach depends on the computational resources available. With sufficient resources and parallel processing, we were able to achieve acceptable levels of scalability. However, there were some initial challenges in fine-tuning the model on large datasets, which required optimizing our training pipeline.
Fascinating article, Hitesh! How does leveraging ChatGPT for churn prediction impact the bottom line of businesses? Have you observed any significant improvements in revenue?
Hi Emma! Leveraging ChatGPT for churn prediction has shown positive impacts on businesses. By identifying potential churners and implementing targeted retention strategies, companies have observed reduced customer churn rates, improved customer retention, and consequently, increased revenue. However, the exact improvement varies based on the specific industry and implementation.
Really interesting read, Hitesh! I'm curious, does ChatGPT also help in identifying the reasons behind churn? Understanding root causes can be crucial for implementing effective retention strategies.
Absolutely, Fred! ChatGPT can assist in identifying potential reasons behind churn by analyzing customer interactions and patterns. It can provide insights into customer concerns, dissatisfaction, or specific triggers that impact their decision to churn. This information proves valuable for implementing tailored retention strategies and targeting key areas for improvement.
Thanks for sharing your insights, Hitesh! How frequently should the ChatGPT model be retrained to maintain prediction accuracy as customer behaviors evolve?
You're welcome, Grace! Retraining frequency depends on the dynamics of customer behavior and the rate at which it evolves. In general, it's recommended to periodically retrain the ChatGPT model, especially when substantial changes in customer patterns are observed. Continuous monitoring and proactive updates ensure that the model stays accurate and relevant in predicting churn.
Hitesh, great article! Could ChatGPT be used for other revenue-related tasks apart from churn prediction? Are there any potential use cases beyond churn analysis?
Thank you, Harry! Absolutely, ChatGPT can be adapted for various revenue-related tasks. Apart from churn prediction, it can assist in customer segmentation, personalized recommendations, pricing optimization, and even revenue forecasting. The flexibility of the ChatGPT model allows for versatile applications in revenue analysis and management.
Great work, Hitesh! What are the essential datasets required for training the ChatGPT model for churn prediction?
Thanks, Ivy! The essential datasets for training the ChatGPT model for churn prediction include historical customer data, including interactions, purchase history, service usage, and churn labels. Additional data such as demographic information and relevant customer attributes can further enhance the model's predictive capabilities.
Hitesh, your article was a great read! Are there any specific industries or types of businesses that can benefit the most from leveraging ChatGPT for churn prediction?
Thank you, Julia! ChatGPT for churn prediction can benefit various industries, including telecommunications, software as a service (SaaS), subscription-based services, e-commerce, and even financial institutions. Any business that deals with customer churn and retention can potentially benefit from leveraging ChatGPT's capabilities.
Great article, Hitesh! In terms of customer experience, has leveraging ChatGPT for churn prediction shown any positive impact on enhancing personalized customer interactions?
Thanks, Kevin! Leveraging ChatGPT for churn prediction has indeed shown a positive impact on enhancing personalized customer interactions. By understanding customer preferences, concerns, and behaviors, companies can offer more tailored and relevant experiences, leading to improved customer satisfaction and loyalty.
Hitesh, your article is very informative! Are there any specific tools or frameworks you recommend for implementing ChatGPT for churn prediction?
Thank you, Linda! For implementing ChatGPT, popular frameworks like TensorFlow or PyTorch can be used. Additionally, tools like Hugging Face's Transformers library provide useful abstractions and pre-trained models that can be fine-tuned for specific tasks like churn prediction. It's important to choose a framework that aligns with your existing tech stack and requirements.
Nice article, Hitesh! How long does it typically take to implement and deploy a ChatGPT-based churn prediction system?
Thanks, Matthew! The time required to implement and deploy a ChatGPT-based churn prediction system can vary depending on factors like available resources, data complexity, and integration requirements. Generally, it can take a few weeks to a few months to successfully develop, train, and deploy such a system, considering the iterative nature of model optimization and validation.
Great insights, Hitesh! When implementing ChatGPT for churn prediction, how do you handle data privacy concerns and ensure the security of customer information?
Thank you, Nancy! Data privacy and security are paramount. We ensure compliance with privacy regulations and follow best practices to safeguard customer information. Anonymization techniques are used when handling sensitive data, and access controls are put in place to limit the exposure of customer information to authorized personnel. Regular security audits and monitoring help maintain data integrity and confidentiality.
Impressive article, Hitesh! Could you share some real-world success stories where ChatGPT for churn prediction has made a significant impact?
Thanks, Olivia! We've seen significant success stories across industries. For example, in the telecommunications sector, companies have achieved reduced customer churn rates by proactively targeting customers identified as likely to churn via ChatGPT predictions. This resulted in improved retention and increased customer satisfaction. Similar success stories have emerged in other industries, emphasizing the value of AI-powered churn prediction.
Great article, Hitesh! How do you handle potential biases in the ChatGPT model during churn prediction, especially considering the impact on revenue and decision-making?
Thank you, Peter! Bias handling is an essential consideration in ML models. During ChatGPT's training, we ensure that the training data is diverse and representative of the customer population to mitigate potential biases. Regular evaluation of the model's predictions and human-in-the-loop approaches help identify any biases and fine-tune the model accordingly. Transparency and fairness in decision-making are crucial to ensure unbiased revenue analysis.
Informative read, Hitesh! Have you encountered any challenges or limitations in integrating the ChatGPT model with existing revenue analysis tools and systems?
Thank you, Robert! Integrating the ChatGPT model with existing revenue analysis tools and systems can pose challenges. Compatibility issues, data preprocessing requirements, and infrastructure considerations need careful attention. Ensuring seamless integration with existing workflows and thorough testing are necessary to overcome these challenges and leverage the potential of ChatGPT for revenue analysis.
Great job, Hitesh! How do you validate the accuracy and effectiveness of the ChatGPT model for churn prediction? Are there any benchmark metrics you follow?
Thanks, Sandra! We validate the accuracy and effectiveness of the ChatGPT model by comparing its predictions with historical churn data and monitoring the actual churn rates over time. Common benchmark metrics we follow include precision, recall, F1-score, and area under the receiver operating characteristic curve (AUC-ROC). Continuous evaluation against these metrics helps ensure the model's quality and predictive performance.
Very informative article, Hitesh! How can businesses leverage the insights from ChatGPT's churn prediction to design targeted retention strategies effectively?
Thank you, Tom! Insights from ChatGPT's churn prediction inform businesses about potential churn triggers and customer concerns. By understanding these factors, businesses can design targeted retention strategies that address specific pain points, offer personalized incentives, and tailor communication to improve customer satisfaction. The aim is to proactively engage and retain customers, delivering an enhanced user experience and fostering long-term loyalty.
Excellent article, Hitesh! Could ChatGPT also assist in identifying potential upselling and cross-selling opportunities as part of revenue analysis?
Thank you, Victoria! Yes, ChatGPT can certainly assist in identifying potential upselling and cross-selling opportunities. By analyzing customer interactions and behavior, the model can provide insights into customers' latent needs and preferences. Leveraging these insights, businesses can generate personalized upselling and cross-selling recommendations, thereby increasing revenue streams and maximizing the customer lifetime value.
Informative discussion, Hitesh! How important is feature engineering in achieving better churn prediction accuracy when utilizing ChatGPT?
Thanks, William! Feature engineering plays a crucial role in achieving better churn prediction accuracy. By carefully selecting and engineering relevant features from the available customer data, we can enhance the model's ability to capture predictive patterns. Feature engineering helps extract unique characteristics of customers and their behavior, enabling ChatGPT to make accurate predictions and improve overall prediction accuracy.
Great insights, Hitesh! How do you measure the return on investment (ROI) when implementing ChatGPT for churn prediction in revenue analysis?
Thank you, Xavier! Measuring the ROI of implementing ChatGPT for churn prediction involves tracking the reduction in churn rates, improved customer retention, and subsequently, increased revenue. By estimating the revenue loss due to churn and comparing it to the business gains achieved through targeted retention strategies, we can evaluate the effectiveness and ROI of utilizing ChatGPT in revenue analysis.
Impressive article, Hitesh! Are there any ongoing research or future developments in the area of ChatGPT-based churn prediction that you find promising?
Thanks, Yvonne! Ongoing research in ChatGPT-based churn prediction focuses on further improving the model's ability to handle unstructured data sources like customer reviews and social media sentiments. Additionally, efforts to enhance interpretability and explainability of the predictions are being explored, enabling better understanding and trust in the model's insights for decision-making.
Well-explained, Hitesh! How do you tackle the challenge of data quality and completeness while training the ChatGPT model for churn prediction?
Thank you, Zara! Data quality and completeness are vital for accurate predictions. We tackle this challenge by thoroughly preprocessing the training data, ensuring data integrity, and employing techniques like data imputation and outlier handling where appropriate. Quality assurance measures are implemented during data collection, and continuous monitoring and validation help maintain the desired level of data quality for training the ChatGPT model.
Excellent article, Hitesh! How do you address the issue of concept drift that may occur in customer behavior over time and affect the effectiveness of the ChatGPT churn prediction model?
Thanks, Zoe! Addressing concept drift is crucial for maintaining the effectiveness of the ChatGPT churn prediction model. Techniques like periodic retraining, continuous monitoring of model performance, and comparison against real-world churn rates help identify drift. By updating the model with fresh data and by incorporating mechanisms to adapt to evolving customer behavior patterns, we can mitigate the impact of concept drift on ChatGPT's performance.