Enhancing RNAseq Analysis with ChatGPT: Expanding Technological Applications
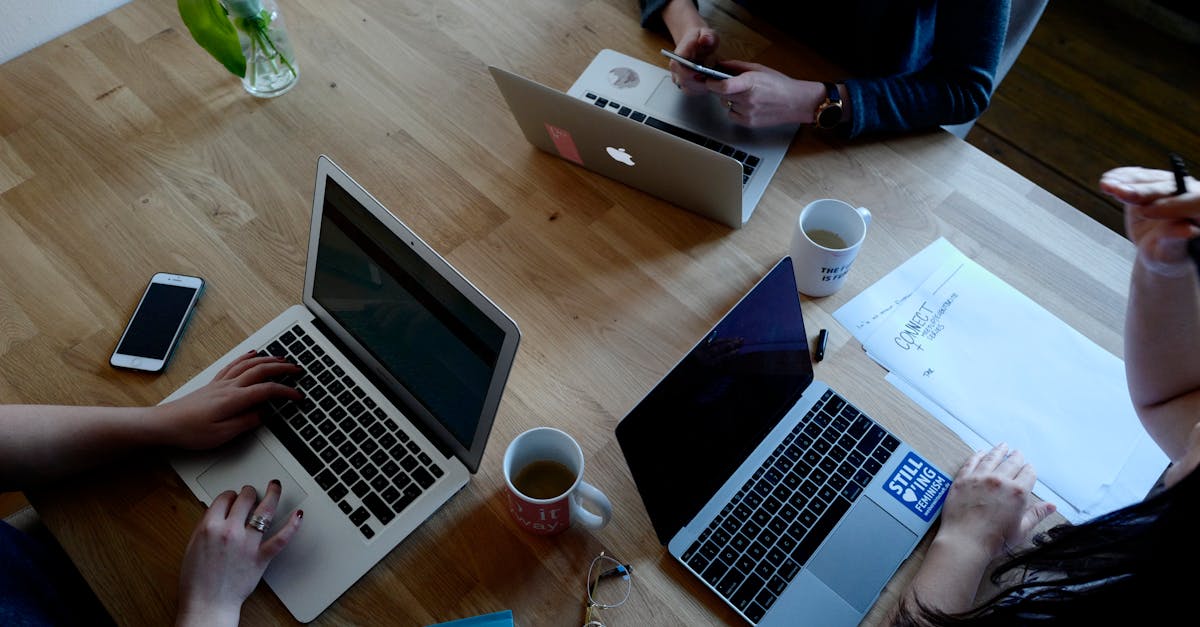
RNA-seq, or RNA sequencing, is a revolutionary technology that leverages next-generation sequencing (NGS) to reveal the presence and providence of RNA in a biological sample at a specific moment. Monitoring the continuously changing cellular transcriptome, that is, the array of all RNA molecules, has reliant applications in developmental biology, genetic disease diagnosis, and health monitoring, among other areas. Although, the value of the RNA-seq data lies greatly on the quality of the analyzed data, hence, the data screening and cleaning process comes into play.
The RNAseq Data
The RNAseq data is highly complex and diverse, including different types of RNAs, such as mRNA (messenger RNA), tRNA (transfer RNA), and rRNA (ribosomal RNA). The complexity of this data makes it intrinsically noisy. It may contain various types of inconsistencies, such as missing values, random noise, and potential outliers. These inconsistencies can bias the subsequent analysis and may lead to inaccurate conclusion. Therefore, robust data screening and cleaning strategies are crucial for maintaining the integrity of the data, and thus, the reliability of the discovered biological insights.
The Data Screening and Cleaning Challenge
Data screening or data cleaning in RNAseq technologies is a challenging task. It entails detecting and addressing anomalies and inconsistencies in the data to enhance its quality. Traditional methods for RNAseq data which involve manual screening and cleaning are labor-intensive, time-consuming, and prone to error. Even recent automated methods can lack the capability or the flexibility to handle complex inconsistencies in high-dimensional RNAseq data.
The Game Changer: ChatGPT-4
Enter ChatGPT-4: an advanced version of the ChatGPT language model developed by OpenAI. With its reinforcement learning from human feedback (RLHF) approach, ChatGPT-4 can provide innovative solutions for screening and cleaning RNAseq data. The model is a transformer neural network, adapted for both understanding and generating human-like text based on large scale data. Applied to RNAseq technologies, ChatGPT-4 is capable of detecting missing or inconsistent data and suggest solutions or automatic corrections. This can tremendously simplify the data screening and cleaning process and increases the efficiency and accuracy of RNAseq data analysis.
How ChatGPT-4 Works
The bidirectional nature of the transformer model in ChatGPT-4 allows it to ensure context-sensitive data examination. It can pick up simple patterns like missing data points or more complex inconsistencies by comparing the context in which a particular RNA datum is found against the rest of the data.
This ability to track and learn from context positions ChatGPT-4 as a powerful tool for data screening in RNAseq technologies. It can generate accurate real-time alerts when inconsistencies are found, significantly cutting down the time and effort required for the data cleaning process. Moreover, by learning from the corrections and the feedback of its users, it can improve its data cleaning algorithms, making it increasingly efficient and reliable over time.
Conclusion
RNAseq data is a gold mine of information with promising potential for understanding the genetic and molecular mechanisms of diseases and health. However, the effective use of RNAseq data requires rigorous data screening and cleaning. The advent of advanced AI technology like ChatGPT-4 is a game-changer for the field. It offers a novel and viable solution by leveraging large-scale data and context-rich predictions to detect and suggest corrections for missing or inconsistent data in RNAseq technologies. With such AI-based tools in our arsenal, science is poised to make more accurate inferences and insights from genomic data, pushing forward our understanding of complex biological systems and disease mechanisms.
Comments:
Thank you all for taking the time to read my article on enhancing RNAseq analysis with ChatGPT. I hope you found it informative and interesting. I'll be here to answer any questions or discuss any points you'd like to bring up.
Great article, Muhammad! I've been using RNAseq for my research, and ChatGPT seems like a promising addition to the analysis process. Do you have any specific examples of how ChatGPT enhances RNAseq analysis?
Thank you, Mark! ChatGPT can be particularly helpful in tasks such as quality control, differential expression analysis, and functional interpretation of RNAseq results. It can assist researchers in exploring and validating their data more efficiently.
I enjoyed the article, Muhammad! The concept of using ChatGPT to expand technological applications, especially in the field of RNAseq analysis, is fascinating. I'm curious about the potential limitations or challenges of integrating ChatGPT into existing analysis pipelines. Are there any to consider?
Samantha, it's great to hear that you found the concept fascinating! While ChatGPT is a powerful tool, like any technology, it does have some limitations. One challenge in integrating ChatGPT into analysis pipelines is the need for high-quality training data to ensure accurate and reliable results. Additionally, the interpretability of ChatGPT's decision-making process can be a potential limitation in critical or high-stakes scenarios.
I appreciate your insights, Samantha! I wonder if incorporating ChatGPT into RNAseq analysis would require specialized training for researchers who are used to traditional methods. To what extent is the learning curve?
Good question, Ellie! The learning curve depends on the familiarity of researchers with artificial intelligence and natural language processing techniques. However, OpenAI has been making efforts to provide user-friendly interfaces and resources to facilitate the integration of ChatGPT into various workflows. It may still require some initial learning, but it's meant to streamline and simplify the analysis process in the long run.
Muhammad, I'm curious about the potential for bias in ChatGPT's decision-making when applied to RNAseq analysis. How can we ensure that the tool doesn't introduce or amplify any unintentional biases in the results?
That's an important concern, Robert. OpenAI has been actively working on addressing bias in AI models, and they encourage users to provide feedback to help improve the system. However, it's crucial for researchers implementing ChatGPT to validate its outputs and critically evaluate the results to minimize potential biases that could be introduced by the tool.
Muhammad, I'm an early-career researcher in genomics, and I'm excited about the potential of ChatGPT. How accessible is it for researchers with limited resources or in developing countries?
Rachel, I understand the importance of accessibility. OpenAI has made strides to provide affordable access to ChatGPT and is actively exploring options such as lower-cost plans and business plans. They also offer free usage tiers. Their goal is to ensure that researchers, regardless of their resources, can leverage the benefits of ChatGPT in their work.
That's great to hear, Muhammad! I'm sure many researchers will appreciate the efforts to make ChatGPT accessible. Are there any specific plans to address specialized needs in the genomics and RNAseq communities?
Absolutely, Emily! OpenAI recognizes the importance of domain-specific fine-tuning and is actively exploring opportunities to address specialized needs in various fields, including genomics and RNAseq. They aim to provide models that are not only accessible but also adaptable to specific research requirements.
Muhammad, what kind of computational resources are needed to run ChatGPT effectively alongside RNAseq analysis? Will it require significant computational power or can it be run on standard setups?
Great question, Liam! While ChatGPT uses advanced machine learning models, OpenAI has made efforts to make it adaptable to a range of computational setups. It can be effectively run on standard setups, which makes it more accessible to a broader range of researchers and institutions.
Muhammad, are there any specific prerequisites or dependencies that researchers need to consider when integrating ChatGPT into their RNAseq analysis workflow?
Good point, Sophia! Researchers planning to integrate ChatGPT into their RNAseq analysis workflow should ensure they have the necessary dependencies, such as the required programming languages, libraries, and frameworks. OpenAI provides documentation and resources to guide researchers through the integration process.
Thank you for the clarification, Muhammad! That's helpful to know, and I appreciate the emphasis on providing resources to support researchers in this integration.
Muhammad, I'm curious about the potential privacy concerns when using ChatGPT for RNAseq analysis. How does OpenAI ensure the confidentiality and security of sensitive research data?
Alice, privacy and security are paramount considerations. OpenAI has strict data usage policies in place and takes the confidentiality of user data seriously. They do not use customer data sent via the API to improve their models and have implemented measures to protect sensitive research data. However, researchers should still follow best practices and exercise due diligence when sharing or transmitting data.
Thank you for addressing my concern, Muhammad! It's reassuring to know that OpenAI has measures in place to protect user data.
Muhammad, I found your article very informative! I'm wondering if ChatGPT has the potential to uncover novel insights in RNAseq data or if it mainly assists in the analysis process.
Thank you, Michael! ChatGPT primarily assists in the analysis process by providing a powerful tool for exploration, validation, and interpretation of RNAseq data. While it can certainly help uncover new insights, it's important to note that the tool's effectiveness depends on the quality and representativeness of the underlying data.
Understood, Muhammad! It sounds like ChatGPT can be a valuable addition to the RNAseq analysis toolkit, enhancing researchers' ability to extract meaningful information from their data.
Muhammad, your article highlighted the benefits of using ChatGPT in RNAseq analysis. Are there any plans to make the tool more interactive, allowing real-time conversations with the model for analysis purposes?
Thank you, Sophia! OpenAI is actively researching and developing methods to improve the interactivity and dynamic capabilities of models like ChatGPT. While I don't have specific details, it's an area of exploration to make the tool more interactive and suitable for real-time conversations as part of the analysis process.
That sounds promising, Muhammad! Real-time conversations could greatly enhance the flexibility and user experience when using ChatGPT for RNAseq analysis.
Muhammad, your article provided valuable insights into the applications of ChatGPT in RNAseq analysis. I'm curious if there are any plans to incorporate visualizations or graphical outputs in ChatGPT to aid researchers' understanding of the data.
Thank you, Sophia! While I don't have specific details, incorporating visualizations and graphical outputs in ChatGPT to aid researchers' understanding of RNAseq data is an area of interest. OpenAI is continuously exploring ways to enhance the tool's abilities and provide researchers with comprehensive insights into their data.
That's exciting, Muhammad! Visualizations can play a significant role in enhancing data interpretation and making complex RNAseq results more accessible to researchers.
Muhammad, I found your article thought-provoking! Considering the growing privacy concerns, does ChatGPT work primarily on-premises, or can it be used with cloud services as well?
Great question, Daniel! ChatGPT can be utilized with cloud services, which is beneficial for researchers who prefer cloud computing or need to leverage its scalability and flexibility. However, it's important to consider data security and privacy aspects when using cloud services, especially with sensitive or confidential research data.
Thank you for clarifying, Muhammad! It's good to know that researchers have the flexibility to choose between on-premises and cloud services for using ChatGPT in RNAseq analysis.
Muhammad, I found your article very interesting! Could ChatGPT be used to address common challenges in RNAseq data analysis, such as batch effects or data normalization?
Thank you, Daniel! ChatGPT can assist researchers in addressing common challenges in RNAseq data analysis, including batch effects and data normalization. By providing insights, suggestions, and facilitating exploratory analysis, it can be a valuable tool in understanding and mitigating these challenges during the analysis process.
That's great to know, Muhammad! ChatGPT's potential to address challenges like batch effects and data normalization can definitely make a difference in RNAseq data analysis.
Muhammad, your article provided valuable insights into the benefits of using ChatGPT for RNAseq analysis. I'm curious if there is ongoing research to optimize the interpretability of ChatGPT's decision-making process.
Thank you, Daniel! OpenAI acknowledges the importance of interpretability and is actively researching techniques to optimize the interpretability of models like ChatGPT. It's an area of focus to ensure transparency and provide researchers with a deeper understanding of the decisions made by the model during RNAseq analysis.
That's reassuring to hear, Muhammad! Enhancing the interpretability of ChatGPT's decision-making process will help build trust and facilitate more informed analyses in the field of RNAseq.
Muhammad, your article delved into the potential applications of ChatGPT in RNAseq analysis. From your perspective, what are the primary benefits and advantages of incorporating ChatGPT into the analysis workflow?
Thank you, Sophia! The primary benefits and advantages of incorporating ChatGPT into the analysis workflow include its ability to assist in exploratory analysis, generate insights, provide validation and interpretation, facilitate literature or resource discovery, and expedite aspects of the analysis process. It can enhance researchers' efficiency, expand possibilities, and open new avenues for data exploration and understanding.
That's a comprehensive overview, Muhammad! The multifaceted benefits of incorporating ChatGPT into RNAseq analysis workflows make it an exciting and promising tool for researchers.
Muhammad, I appreciate your response! Validating ChatGPT's outputs sounds critical. Are there any specific guidelines or best practices that researchers should follow in this regard?
Absolutely, Robert! Researchers should follow established scientific practices and critically evaluate ChatGPT's outputs by comparing them against other methods, consulting domain experts, or conducting empirical validation where appropriate. It's vital to take a rigorous approach to ensure the accuracy and reliability of the results obtained through ChatGPT.
Thank you for the guidance, Muhammad! I agree that a rigorous validation process is necessary to maintain the integrity of the results.
Muhammad, your article was enlightening! Do you have any recommendations for researchers who are interested in applying ChatGPT to their RNAseq analysis but are unsure how to start?
Thank you, Noah! If you're interested in applying ChatGPT to RNAseq analysis, I recommend starting by familiarizing yourself with the available resources and documentation provided by OpenAI. They offer guidelines, tutorials, and example code to help researchers get started. It's also important to connect with the community of researchers who are already using or exploring ChatGPT for RNAseq analysis, as their experiences and insights can be invaluable.
That makes sense, Muhammad! Connecting with the community and leveraging available resources seem like a good starting point for researchers looking to integrate ChatGPT into their RNAseq analysis workflows.
Muhammad, I'm curious about the potential time savings researchers can expect to gain by using ChatGPT in RNAseq analysis. Can you provide any insights on this?
Good question, Emma! While the time savings can vary depending on the specific analysis tasks and datasets, ChatGPT can help researchers streamline and automate certain aspects of the analysis process. By providing quick insights, assisting in data interpretation, and guiding researchers towards relevant literature or resources, it has the potential to save considerable time in RNAseq analysis.
Thank you for the response, Muhammad! It's exciting to think about the time-saving possibilities that ChatGPT can offer to researchers in the field of RNAseq analysis.
One more question, Muhammad! Are there any specific limitations or caveats researchers should be aware of before adopting ChatGPT for RNAseq analysis?
Certainly, Emma! Researchers should be aware that ChatGPT's performance can vary based on the input provided, and it might not always generate optimal or correct responses. It's crucial to critically evaluate the results, validate outputs against existing methods, and use ChatGPT as a tool to augment the analysis process rather than relying solely on it. Additionally, it's essential to stay updated with any newer versions or improvements in the model and follow best practices as recommended by OpenAI.
Thank you for addressing my query, Muhammad! I appreciate the emphasis on critical evaluation and responsible use of ChatGPT in RNAseq analysis.
Muhammad, your article shed light on the potential of ChatGPT in RNAseq analysis. I'm curious to know if there are any ongoing research efforts to further enhance the capabilities of ChatGPT in this regard.
Thank you, Oliver! Yes, there are continuous research efforts to enhance ChatGPT's capabilities in various domains, including RNAseq analysis. OpenAI is actively exploring techniques like domain adaptation and allows users to fine-tune the model on specific tasks or domains, potentially unlocking further potential for researchers.
That's fascinating, Muhammad! It's exciting to think about the future developments and refinements that can empower researchers even more.
Muhammad, great article! I'm curious if ChatGPT can handle large-scale RNAseq datasets with several samples, and if there are any specific performance considerations for such scenarios.
Thank you, Lucy! ChatGPT's performance with large-scale RNAseq datasets depends on factors like the available computational resources, the complexity of the analysis tasks, and the specific requirements of the analysis pipeline. While it can handle substantial datasets, researchers should consider the hardware limitations and potentially use optimization techniques like batching and parallelization to improve performance if needed.
I see, Muhammad! It's helpful to be aware of the potential performance considerations and optimization options when working with large-scale RNAseq datasets.
Muhammad, excellent article! I'm curious about the potential scalability of ChatGPT. Can it handle high-throughput RNAseq data generated in projects with a large number of samples?
Thank you, Oliver! ChatGPT can handle high-throughput RNAseq data generated in projects with a large number of samples, but scalability depends on factors like computational resources, the complexity of analysis tasks, and the specific requirements of the project. Researchers may need to optimize resource usage and employ techniques like distributed computing for large-scale analyses.
Got it, Muhammad! Managing computational resources and considering scalability will definitely be crucial when working with large-scale RNAseq projects using ChatGPT.
Muhammad, your article shed light on the promising role of ChatGPT in RNAseq analysis. What are your thoughts on the potential integration of ChatGPT with other analysis tools or pipelines to enhance research workflows?
Thank you, Oliver! The integration of ChatGPT with other analysis tools or pipelines can indeed enhance research workflows, providing researchers with a more comprehensive and interconnected analysis framework. Combining the strengths of ChatGPT with existing tools can unlock synergistic effects and enable more efficient and insightful RNAseq analyses.
That sounds promising, Muhammad! Seamless integration with existing tools can potentially amplify the benefits of ChatGPT in RNAseq analysis.
Muhammad, your article highlighted the potential benefits of integrating ChatGPT in RNAseq analysis. Are there any plans to develop specific educational resources or courses to help researchers learn and harness the power of ChatGPT?
Thank you, Emma! OpenAI is committed to supporting researchers in learning and harnessing the power of ChatGPT. Though I don't have specific details, they have been actively exploring educational resources, workshops, and courses to help researchers develop the necessary skills for integrating ChatGPT into their RNAseq analysis workflows.
That's wonderful, Muhammad! Providing educational resources and courses will undoubtedly empower researchers to make the most out of ChatGPT and enhance their RNAseq analysis capabilities.
Muhammad, I'm impressed by the potential applications of ChatGPT in RNAseq analysis. Are there any plans to make it compatible with interactive notebook environments, making the analysis process more intuitive and efficient?
Thank you, Lucas! It's an interesting suggestion. While I don't have specific information, OpenAI is actively exploring various ways to improve usability and integration with different environments. The compatibility of ChatGPT with interactive notebook environments to enhance the intuitive analysis process could be a potential consideration.
That's great to hear, Muhammad! Compatibility with interactive notebook environments could foster a more seamless and user-friendly experience for researchers using ChatGPT for RNAseq analysis.
Muhammad, I appreciate the insights you shared in your article! In your opinion, what are the most exciting future possibilities for ChatGPT in RNAseq analysis?
Thank you, Lucas! One of the most exciting possibilities for ChatGPT in RNAseq analysis is its potential to facilitate collaborative analysis platforms where researchers can interact with the model, discuss insights, and benefit from collective intelligence. This could unlock new avenues for collaboration and accelerate discoveries while improving the efficiency and effectiveness of RNAseq analyses.
That's fascinating, Muhammad! Collaborative analysis platforms powered by ChatGPT could revolutionize the way researchers approach and collaborate on RNAseq analysis.
Muhammad, your article shed light on the integration of ChatGPT in RNAseq analysis. I'm curious if there are any plans for providing pre-trained models specifically tailored for RNAseq tasks or analysis objectives.
Thank you, Emma! While I don't have specific information, OpenAI is actively researching and developing models that can be fine-tuned for specific tasks or objectives. It's highly likely that tailored pre-trained models for RNAseq analysis or similar objectives could be an area of exploration in the future.
That's exciting, Muhammad! Pre-trained models specifically tailored for RNAseq tasks could significantly enhance the capabilities and applicability of ChatGPT in this domain.
Muhammad, I assume that ChatGPT's performance could be affected by the quality and characteristics of RNAseq data. Are there any specific data preprocessing steps or considerations that researchers should keep in mind?
Absolutely, Noah! Preprocessing RNAseq data is an important step to ensure the quality and reliability of the analysis results. Researchers should consider steps such as read alignment, transcript abundance estimation, and normalization. It's crucial to follow standard best practices in RNAseq data preprocessing to obtain accurate and meaningful insights through ChatGPT or any other analysis method.
Thank you for clarifying, Muhammad! Adhering to best practices in RNAseq data preprocessing is indeed crucial for reliable analysis outcomes.
Muhammad, your article was informative! I'm wondering if there are any potential collaborations or partnerships with research institutions to further explore the applications of ChatGPT in RNAseq analysis?
Thank you, Sophie! OpenAI actively encourages collaborations and partnerships with research institutions to explore and expand the applications of ChatGPT in various domains, including RNAseq analysis. Researchers can reach out to OpenAI to discuss potential collaborations and explore novel use cases together.
That sounds exciting, Muhammad! Collaborations and partnerships can often lead to innovative breakthroughs. It's great to know that OpenAI is open to such collaborations in the field of RNAseq analysis.
Muhammad, I enjoyed your article! With the continuous improvements in AI and NLP, I'm curious if there are any plans to develop more specialized AI models specifically tailored for RNAseq analysis.
Thank you, Jacob! OpenAI has plans to explore domain-specific fine-tuning of models, which can potentially include the development of more specialized AI models specifically tailored for RNAseq analysis. The aim is to provide researchers with highly adaptable and powerful tools that can cater to their specific needs and domain requirements.
That's great to hear, Muhammad! The development of specialized AI models holds immense potential for enhancing the capabilities of RNAseq analysis.
Muhammad, I'm curious about potential performance enhancements of ChatGPT. Are there any ongoing efforts to optimize its speed and resource requirements for efficient RNAseq analysis?
Good question, Jacob! OpenAI is continuously working on optimizing model performance and resource requirements to ensure efficient usage. As more research is conducted and feedback is generated, there will likely be efforts to improve the speed and resource efficiency of ChatGPT, making it more suitable for RNAseq analysis and other computational tasks.
That's reassuring to hear, Muhammad! It's important for tools like ChatGPT to keep evolving in terms of performance to cater to the needs of researchers in the dynamic realm of RNAseq analysis.
Muhammad, your article presented a compelling case for the integration of ChatGPT in RNAseq analysis. What are your thoughts on potential ethical considerations researchers should be mindful of when using ChatGPT?
Thank you, Sophie! Ethical considerations are indeed important. Researchers should consider factors like transparency, fairness, accountability, and potential biases when using ChatGPT. It's crucial to critically analyze the outputs, ensure a diverse and representative training dataset, and apply ethical guidelines and regulations specific to their research domain.
I appreciate the emphasis on ethics, Muhammad! Responsible and ethical use of AI tools like ChatGPT is vital for maintaining the integrity and societal impact of the research conducted in the field of RNAseq analysis.
Muhammad, your article highlighted the potential of ChatGPT in RNAseq analysis. Are there any specific efforts to ensure the tool's utility for researchers with varying levels of experience in computational biology or bioinformatics?
Thank you, Sophie! OpenAI is working on making ChatGPT accessible to researchers with varying levels of experience in computational biology or bioinformatics. This includes providing user-friendly interfaces, documentation, and resources tailored to different skill levels, enabling researchers to leverage ChatGPT's utility regardless of their prior experience in these domains.
That's fantastic, Muhammad! Making ChatGPT accessible to researchers of all experience levels will foster inclusivity and drive innovation in the field of RNAseq analysis.
Muhammad, your article highlighted the potential of ChatGPT in RNAseq analysis. Are there any plans to include domain-specific literature or database integration to augment the analysis process?
Thank you, Sophie! While I don't have specific details, OpenAI recognizes the value of domain-specific literature and databases for researchers. Integration with relevant resources or databases to augment the analysis process is an area of interest and exploration for further empowering researchers using ChatGPT in RNAseq analysis.
Ellie, I've been using ChatGPT for natural language processing tasks, and I could definitely see it being valuable for RNAseq analysis. The learning curve wasn't too steep, especially if you're already familiar with AI and NLP techniques.
Thanks for sharing your experience, Alan! I haven't delved much into AI and NLP, but your insight gives me confidence that I can learn to use ChatGPT effectively in RNAseq analysis.
Great article! ChatGPT seems like a promising tool to enhance RNAseq analysis. I'm excited to see how it can expand technological applications in this field.
Thank you, Sara! I appreciate your positive feedback. Indeed, ChatGPT holds great potential to revolutionize RNAseq analysis and its applications.
I'm a bioinformatics researcher, and I'm impressed with the concept of using ChatGPT for RNAseq analysis. It could potentially help in automating repetitive tasks and improving overall efficiency.
As someone who works with RNAseq data regularly, I find the idea of incorporating ChatGPT intriguing. It could be helpful in exploring complex expression patterns and identifying novel insights.
I have some concerns about the reliability of ChatGPT in this context. How well does it handle the intricacies of RNAseq analysis and the nuances of biological data?
Valid point, Alison. While ChatGPT can provide valuable assistance, it's important to remember that it's an AI model and may have limitations. It should be used as a complementary tool rather than a replacement for domain experts.
I'm curious about the feasibility of training ChatGPT specifically for RNAseq analysis. Has any research been conducted on optimizing it for this field?
Good question, Jason. There are ongoing efforts to fine-tune ChatGPT for specialized domains like bioinformatics. Research on optimizing its performance for RNAseq analysis is underway, and initial results are promising.
The idea of using ChatGPT for data interpretation and hypothesis generation in RNAseq analysis is intriguing. It could potentially help bridge the gap between generated results and biological understanding.
Absolutely, Olivia. ChatGPT's ability to generate explanations and assist in hypothesis formation has significant value in the complex realm of RNAseq analysis.
I'm impressed with the advancements in AI technologies applied to genomics. It's exciting to see how ChatGPT can contribute to enhancing our understanding of RNAseq data.
Indeed, Nathan! AI technologies like ChatGPT have the potential to unlock hidden insights and drive breakthroughs in genomics and our knowledge of RNAseq data.
I wonder how ChatGPT compares to other tools in terms of accuracy and interpretability in the field of RNAseq analysis. Has there been a comparative study?
That's an important consideration, Sophia. There have been initial comparative studies, but more extensive research is needed to thoroughly assess the accuracy and interpretability of ChatGPT against other existing tools in RNAseq analysis.
ChatGPT sounds promising, but what about the potential biases it may have? AI models are known to inherit biases from training data, which could impact the analysis results.
You raise a valid concern, Liam. Addressing biases in AI models is crucial, particularly in sensitive fields like genomics. Efforts are being made to mitigate biases and improve the fairness of ChatGPT for better analysis outcomes.
I believe that ChatGPT could be an excellent tool for educational purposes in RNAseq analysis. It can assist students in understanding complex concepts and improve their learning experience.
Absolutely, Sarah! ChatGPT's educational potential is immense in the field of genomics. It can serve as a valuable resource to aid students and researchers in their learning journeys.
ChatGPT is undoubtedly a remarkable advancement, but we should be cautious not to overly rely on AI tools. It's essential to maintain the human element and domain expertise in RNAseq analysis.
Well said, Tom. AI tools like ChatGPT should be used as a supporting mechanism while keeping human insights and expertise at the center of RNAseq analysis.
I can see many potential applications of ChatGPT in RNAseq analysis, such as automating data preprocessing and quality control. This could save time and streamline the analysis workflow.
Indeed, Grace. Automation of time-consuming and repetitive tasks is a significant advantage of using ChatGPT for RNAseq analysis. It has the potential to enhance the overall efficiency of the workflow.
ChatGPT could be a valuable tool for researchers in small-scale labs or those with limited resources. Its accessibility and potential cost-effectiveness could democratize advanced analysis capabilities.
You make an excellent point, Lily. By providing advanced analysis capabilities, ChatGPT can level the playing field for researchers in terms of resources, enabling wider participation in genomics research.
I'm curious about the implementation details of using ChatGPT for RNAseq analysis. Is there any specific setup or training required to utilize the tool effectively?
Good question, Jack. To effectively use ChatGPT for RNAseq analysis, one needs to fine-tune the model using relevant data and provide it with context-specific instructions during training. The setup details may vary depending on the specific use case.
ChatGPT seems like a great step forward, but how user-friendly is it for researchers who may not have extensive experience with AI or coding?
Valid concern, Emily. The user-friendliness of ChatGPT for researchers without extensive AI or coding experience depends on the accessibility and intuitiveness of the interface designed around it. Simplifying the tool's usage is an ongoing focus to make it accessible to a broader user base.
I can see potential collaboration opportunities by integrating ChatGPT with existing RNAseq analysis pipelines. It could serve as an interactive assistant during analysis.
Absolutely, Maxwell! Integrating ChatGPT with existing pipelines can enable real-time interaction and assistance, improving the analytical process and helping researchers gain more insights from their RNAseq data.
ChatGPT's applications are exciting! I'm particularly interested in its role in discovering alternative splicing events and novel transcript isoforms. Could it assist in such analyses?
Certainly, Claire! ChatGPT has the potential to assist in the identification and analysis of alternative splicing events and novel transcript isoforms in RNAseq data. It can provide insights and facilitate exploration in this area of genomics.
Privacy concerns often arise when using AI models. How can the privacy of sensitive RNAseq data be ensured when utilizing ChatGPT for analysis?
A valid concern, James. Privacy protection is critical when working with sensitive genomic data. While using ChatGPT, data anonymization and proper security measures must be in place to safeguard the privacy of RNAseq data.
The ethical considerations around AI utilization are crucial. Are there any guidelines or best practices in place for responsible use of ChatGPT in genomics research?
Great question, Sophie. Ethical guidelines and best practices are being developed to ensure responsible use of AI models like ChatGPT in genomic research. Collaborative efforts among researchers and experts are instrumental in shaping these guidelines.
I'm curious about the limitations of ChatGPT in the context of RNAseq analysis. What are the areas where it may struggle or require improvement?
A valid inquiry, Emma. While ChatGPT can provide valuable assistance, it might struggle in addressing highly complex and specialized questions in RNAseq analysis. Continuous improvement and addressing the model's limitations are ongoing research endeavors.
The potential of AI like ChatGPT to facilitate large-scale data exploration in genomics is exciting. It can help researchers uncover patterns and associations that may otherwise be challenging to spot.
Absolutely, Noah! AI tools like ChatGPT can complement the efforts of researchers by assisting in large-scale data exploration and uncovering valuable patterns, leading to new discoveries in genomics.