Enhancing Root Cause Analysis in Quality Assurance: Harnessing the Power of ChatGPT '20
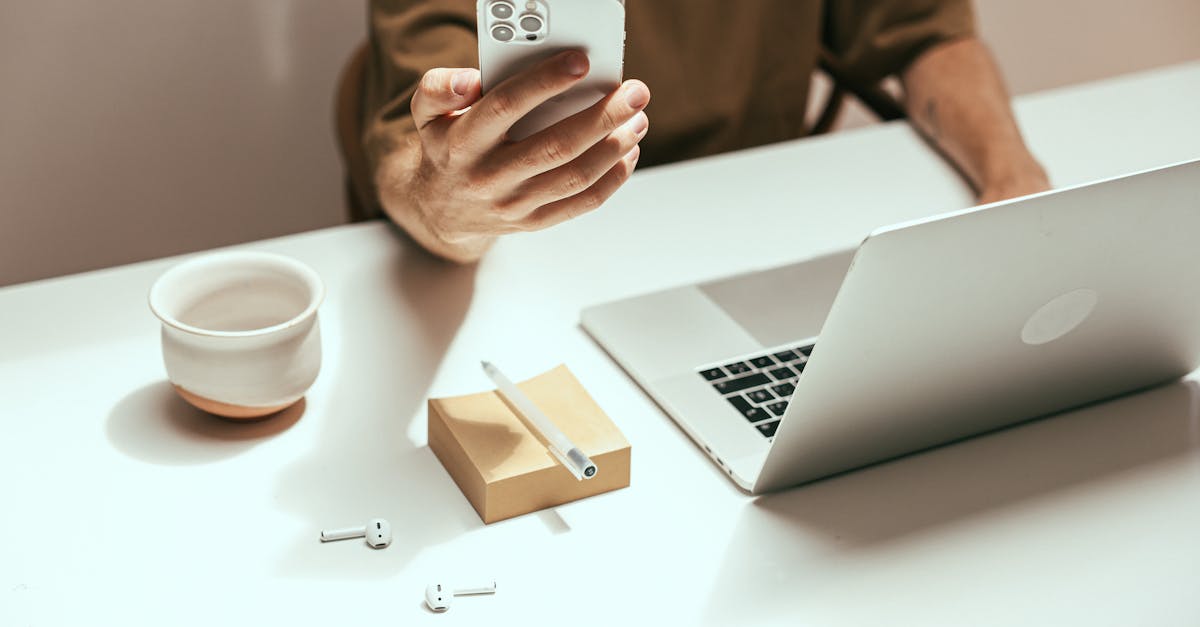
Root cause analysis is a critical process in quality assurance that aims to identify the underlying causes of defects or issues in a product or system. By understanding the root causes, organizations can take preventive measures to avoid similar issues in the future. With advancements in artificial intelligence, tools like ChatGPT-4 can now assist in this investigation process, offering recommendations and insights that can streamline the analysis.
What is Root Cause Analysis?
Root cause analysis is a systematic approach to identifying the fundamental reasons behind a problem or defect. Instead of focusing solely on the symptoms or immediate causes, it delves deeper into the factors that contribute to the issue. By addressing the root causes, organizations can implement targeted solutions that resolve the problem at its core.
The Role of ChatGPT-4 in Root Cause Analysis
ChatGPT-4, an advanced language model developed using state-of-the-art natural language processing techniques, can be a valuable tool in root cause analysis. It can assist quality assurance professionals in identifying and understanding the underlying causes of defects. Here's how ChatGPT-4 can contribute to the analysis:
- Efficient Data Processing: ChatGPT-4 can rapidly process large volumes of data, including defect reports, customer feedback, and system logs. By analyzing this data, it can help identify patterns and correlations that may indicate potential root causes.
- Contextual Understanding: The advanced AI capabilities of ChatGPT-4 enable it to comprehend complex contexts and domain-specific terminology. It can interpret defect descriptions, system behavior, and other relevant data sources to generate meaningful insights.
- Identifying Patterns and Trends: By analyzing historical data and comparing it with present situations, ChatGPT-4 can recognize recurring patterns or trends associated with specific defects. This information can guide quality assurance professionals in pinpointing potential root causes.
- Providing Recommendations: Based on its analysis, ChatGPT-4 can generate recommendations for preventing similar issues in the future. These recommendations may include process improvements, changes in system configurations, or updates to product design.
- Collaborative Problem Solving: ChatGPT-4 can act as a virtual collaborator, engaging in back-and-forth conversations to explore different hypotheses, evaluate possible causes, and refine the root cause analysis. It can provide valuable insights and challenge assumptions, fostering a more comprehensive and efficient investigation process.
Benefits and Limitations
The utilization of ChatGPT-4 in root cause analysis offers several advantages:
- Accelerated analysis: ChatGPT-4's ability to process data at an extraordinary speed enables faster detection of patterns and root causes.
- In-depth insights: The contextual understanding of ChatGPT-4 contributes to more accurate analysis and helps identify complex, interrelated causes that may go unnoticed.
- Preventive measures: The recommendations generated by ChatGPT-4 assist in implementing preventive actions to avoid similar issues in future products or systems.
- Collaborative assistance: ChatGPT-4 acts as a virtual assistant in root cause analysis, facilitating more efficient collaboration between human experts and AI.
However, it is important to acknowledge the limitations of ChatGPT-4:
- Dependency on data quality: The accuracy of ChatGPT-4's analysis heavily relies on the quality and relevance of the data provided. Insufficient or misleading data may result in inaccurate insights.
- Lack of human intuition: While ChatGPT-4 can process vast amounts of information, it lacks human intuition that might be crucial in identifying certain root causes or verifying hypotheses.
- Continuous training: Keeping ChatGPT-4 up-to-date with the latest knowledge and domain-specific information is essential for its optimal performance in root cause analysis.
Conclusion
Root cause analysis plays a vital role in quality assurance, enabling organizations to identify and address the underlying reasons behind defects. With the assistance of advanced language models like ChatGPT-4, the process of root cause analysis can become more efficient, insightful, and collaborative. By leveraging ChatGPT-4's ability to process large volumes of data, generate recommendations, and engage in conversational problem-solving, quality assurance professionals can enhance their investigative efforts and improve the overall quality of products and systems.
Comments:
Thank you all for taking the time to read my article on enhancing root cause analysis with ChatGPT '20! I'm excited to hear your thoughts and opinions.
Great article, Chris! Root cause analysis is a critical aspect of quality assurance, and leveraging ChatGPT '20 seems promising. Have you personally used it for this purpose?
Thanks, Emily! Yes, I have experimented with using ChatGPT '20 for root cause analysis, and it has shown potential in assisting with problem-solving and generating hypotheses.
ChatGPT '20 sounds intriguing, but how does it handle complex multi-dimensional problems that are common in quality assurance?
That's a good question, Daniel. ChatGPT '20 can be trained on vast amounts of data, allowing it to understand complex dimensions. It can help identify potential root causes by analyzing patterns and generating explanations based on the input it receives.
I'm wondering about the limitations of using AI like ChatGPT '20 for root cause analysis. Can it truly replace human expertise?
That's a valid concern, Sophia. ChatGPT '20 is a tool to augment human expertise rather than replace it. It can provide valuable insights, generate hypotheses, and assist in problem-solving, but human judgment and domain knowledge will always play a crucial role in the analysis process.
I'm impressed by the potential of ChatGPT '20 for root cause analysis. How do you envision it being integrated into existing quality assurance processes?
Thanks, Jessica! ChatGPT '20 can be used in conjunction with human experts during root cause analysis. It can provide alternative perspectives, propose potential causes, and help explore different angles, all of which can enrich the existing QA process and facilitate more effective problem-solving.
As a QA manager, I'm concerned about the interpretability of AI-generated insights. How do we ensure transparency and understand the reasoning behind ChatGPT '20's suggestions?
Good point, Ryan. Explainability is crucial in AI-assisted analyses. By designing the system to provide justifications for its suggestions and allowing users to probe its reasoning, we can enhance transparency and ensure that the insights generated by ChatGPT '20 can be understood and validated by QA experts.
While ChatGPT '20 seems promising, I wonder about the potential biases it might have in root cause analysis. How can we address this issue?
Addressing biases is crucial, Amanda. By carefully curating training data and incorporating diverse perspectives during the analysis process, we can mitigate biases to a certain extent. Regular monitoring and human oversight are also important to ensure fairness and prevent AI-generated insights from reinforcing existing biases.
I'm curious if ChatGPT '20 can be fine-tuned specifically for different domains within quality assurance?
Absolutely, David. Fine-tuning ChatGPT '20 with domain-specific data can improve its performance in particular areas of quality assurance. By training it on relevant datasets and refining its responses, we can make it more effective in addressing specific root cause analysis challenges within different domains.
What steps can organizations take to adopt ChatGPT '20 for root cause analysis? Is there a steep learning curve for implementation?
Implementing ChatGPT '20 involves steps like data preparation, training, integration, and continuously refining the system based on user feedback. While there might be a learning curve initially, organizations can collaborate with AI experts to streamline the adoption process and ensure successful integration into existing quality assurance practices.
I'm concerned about the scalability of using ChatGPT '20 for large-scale quality assurance processes. Can it handle the volume and speed required in such scenarios?
Scalability is indeed important, Brian. ChatGPT '20 can be optimized for performance by leveraging distributed computing and efficient infrastructure. By parallelizing computation and optimizing response times, it can handle the volume and speed requirements of large-scale quality assurance processes.
Is the ChatGPT '20 model available for organizations to use, or is it still in research and development?
The ChatGPT '20 model is currently under research and development, Lisa. However, organizations can collaborate with OpenAI to explore its potential and participate in pilot programs to test its suitability in real-world quality assurance scenarios.
Could ChatGPT '20 introduce bias if there is a lack of diversity in the data it's trained on?
Indeed, Samuel. Lack of diversity in training data can lead to biased outputs. That's why it's vital to ensure representative datasets and consider potential biases during training. By incorporating diverse perspectives and continuously monitoring the system's behavior, we can mitigate the risk of introducing bias through ChatGPT '20.
What are the potential risks associated with relying too heavily on ChatGPT '20 for root cause analysis in quality assurance?
An overreliance on ChatGPT '20 could lead to insufficient human involvement and a lack of critical thinking. It's important to use it as an aid, complementing human expertise rather than replacing it. Adequate validation of insights, following up with manual investigations, and continuously training and refining the system are crucial to mitigate potential risks.
Can ChatGPT '20 actively learn from user interactions and improve its root cause analysis capabilities over time?
Indeed, Alex. ChatGPT '20 can learn from user interactions, which enables it to improve its performance and understand the nuances of root cause analysis better. Incorporating feedback and continuous training can enhance its capabilities, making it more effective in generating valuable insights.
Considering the dynamic nature of quality assurance, how adaptable is ChatGPT '20 in keeping up with emerging trends and evolving challenges?
Adaptability is a key consideration, Olivia. ChatGPT '20 can be updated and trained on new data to keep up with emerging trends and evolving challenges in quality assurance. By incorporating continuous learning and staying updated on industry advancements, we can ensure that the system remains relevant and effective.
What are the potential ethical implications of employing AI like ChatGPT '20 in quality assurance root cause analysis?
Ethical considerations are critical, Matthew. We need to ensure privacy, prevent discrimination, and be transparent about the limitations and uncertainties of AI-generated insights. Implementing robust guidelines, ethical frameworks, and rigorous monitoring can help mitigate ethical implications while leveraging AI like ChatGPT '20 in quality assurance.
Are there any specific use cases where ChatGPT '20 has demonstrated exceptional performance in root cause analysis?
While ChatGPT '20 is still being explored, early experiments have shown promise in various root cause analysis use cases, such as software bugs & errors, manufacturing defects, and customer complaints. However, it's important to note that further research and domain-specific fine-tuning are necessary to unlock its full potential in each specific use case.
How does ChatGPT '20 handle unstructured and incomplete data during root cause analysis?
Handling unstructured and incomplete data is a challenge, Grace. ChatGPT '20 copes relatively well with unstructured text, and it can provide insights even when data is incomplete. However, it's important to ensure data completeness and use appropriate techniques to handle missing information when training the system for more accurate and reliable analysis.
What are the potential pitfalls to be aware of when implementing ChatGPT '20 for root cause analysis?
One potential pitfall is relying solely on ChatGPT '20 without human validation, which could lead to inaccurate or biased insights. Other pitfalls include overestimating its capabilities, data limitations, interoperability challenges, and potential resistance to change within the organization. Being aware of these pitfalls and addressing them proactively can lead to a more successful implementation.
What kind of computational resources are typically required to deploy ChatGPT '20 for root cause analysis?
The computational resources required for deploying ChatGPT '20 can vary based on factors like model size, training data, and usage requirements. It's typically beneficial to leverage powerful hardware infrastructure and distributed systems to ensure efficiency and scalability, especially in large-scale root cause analysis processes.
What are some alternatives to ChatGPT '20 for enhancing root cause analysis in quality assurance?
ChatGPT '20 is just one of the options, Thomas. Other alternatives include leveraging other AI models, data analytics tools, and expert systems. The choice of tool or approach depends on the specific needs, resources, and objectives of the quality assurance process in question.
How can organizations determine the ROI of implementing ChatGPT '20 for root cause analysis?
Determining the ROI involves evaluating factors like the efficiency gains, reduction in investigation time, improved accuracy in identifying root causes, and overall cost savings achieved by implementing ChatGPT '20. Conducting pilot studies, comparing results with baseline metrics, and gathering feedback from QA teams can help quantify the advantages and assess the return on investment.
How can organizations ensure the security of sensitive data when using ChatGPT '20 for root cause analysis?
Ensuring data security is paramount, Samantha. Organizations should apply strong security measures like encryption, access controls, and secure storage when handling sensitive data during root cause analysis with ChatGPT '20. Complying with relevant data protection regulations and following best practices for data privacy are essential to maintain confidentiality and prevent unauthorized access.
Does ChatGPT '20 require continuous online connectivity to operate effectively for root cause analysis?
While online connectivity is beneficial for continuous learning and accessing external resources, ChatGPT '20 can still operate effectively for root cause analysis in offline scenarios by leveraging its pre-trained knowledge. However, a dynamic environment where it can receive updates and learn from user interactions is advantageous for ensuring its performance remains up-to-date.
Are there any regulatory or legal considerations to keep in mind when deploying ChatGPT '20 for root cause analysis in certain industries?
Yes, Melissa. Certain industries, such as healthcare or finance, have specific regulations regarding data privacy, information security, and ethical AI use. It's crucial to ensure compliance with these regulations and consult legal experts to address any legal considerations that may arise when deploying ChatGPT '20 for root cause analysis in such industries.
Thank you all for your valuable questions and feedback! I appreciate your engagement in this discussion on enhancing root cause analysis with ChatGPT '20. Feel free to reach out if you have further inquiries or thoughts.