Enhancing Root Cause Analysis in Technology with ChatGPT: A Game-Changing Approach
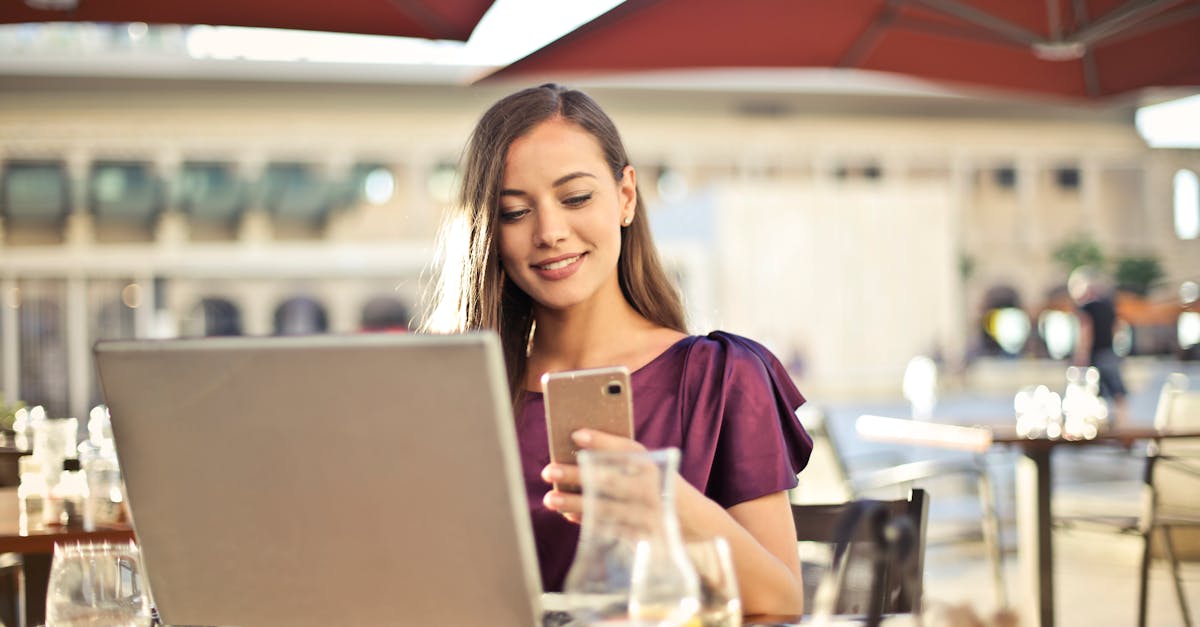
Root Cause Analysis (RCA) is a valuable technique used in IT Operations to identify the underlying reasons behind system failures or operational issues. It plays a crucial role in minimizing downtime, enhancing system performance, and improving overall reliability.
Introduction to Root Cause Analysis
RCA involves a systematic approach to problem-solving by investigating the events and conditions leading up to a specific issue. By analyzing logs, system metrics, and user reports, IT professionals can determine the root cause and implement appropriate corrective actions.
How RCA Assists in Troubleshooting
ChatGPT-4, powered by OpenAI's advanced language model, can assist in the RCA process by leveraging its natural language processing capabilities and knowledge of IT systems. It can analyze complex data sets, uncover patterns, and generate insights to aid IT professionals in troubleshooting.
Analyzing Logs and System Metrics
Logs and system metrics provide valuable information about the behavior and performance of IT systems. ChatGPT-4 can process these data sources, identify anomalies, and highlight potential areas of concern. By analyzing log files, it can trace the sequence of events leading up to the failure, helping IT professionals understand the exact point of failure and its causes.
Understanding User Reports
User reports often contain valuable insights into system issues, capturing user experiences, errors encountered, and the context of the problem. Through natural language understanding, ChatGPT-4 can analyze user reports to identify common issues, patterns, or possible user error that could be contributing factors to the problem. This helps in prioritizing and addressing the most critical issues quickly.
Generating Insights and Recommendations
Based on the information gathered from logs, system metrics, and user reports, ChatGPT-4 can generate insights and recommendations to help IT professionals pinpoint the root cause of an issue. It can propose possible solutions, best practices, or areas that require further investigation. Such suggestions assist IT teams in making informed decisions and taking appropriate actions to prevent future occurrences.
The Benefits of RCA in IT Operations
Implementing RCA in IT operations offers several benefits:
- Minimizing downtime: By identifying and resolving root causes promptly, RCA helps minimize the impact of system failures and reduces downtime.
- Enhancing system performance: RCA enables IT teams to identify bottlenecks, inefficiencies, or misconfigurations that hinder system performance and take proper corrective actions.
- Improving reliability: Continuously performing RCA helps organizations learn from incidents and implement preventive measures to improve system reliability.
- Increasing customer satisfaction: Quick resolution of issues and improved system reliability contribute to enhanced customer satisfaction.
Conclusion
Root Cause Analysis, with the assistance of advanced language models like ChatGPT-4, empowers IT professionals to troubleshoot and identify the underlying causes of system failures or operational issues. By leveraging AI-driven analytics, IT teams can proactively resolve problems, reduce downtime, and enhance system performance, leading to improved productivity and customer satisfaction.
Comments:
Thank you all for your interest in my article on enhancing root cause analysis with ChatGPT! I'm excited to discuss this game-changing approach with you. Feel free to ask any questions or share your thoughts.
Great article, Britton! I believe incorporating ChatGPT in root cause analysis can be a significant step forward in identifying and resolving technology issues. Have you applied this approach in a real-world scenario?
Thank you, Michael! Yes, I've had the opportunity to apply ChatGPT in a few real-world scenarios. It has proven to be quite effective in assisting with complex root cause analysis tasks. Happy to share more details!
Interesting read, Britton! How does ChatGPT compare to traditional methods of root cause analysis? Are there any particular limitations one should be aware of?
Thank you, Emily! ChatGPT offers a more dynamic and interactive approach compared to traditional methods. It allows for a natural conversation where additional questions can be asked to dig deeper. However, one limitation is that it relies on the data it has been trained on, so it may not have knowledge of very recent events or evolving systems.
Impressive work, Britton! How do you see ChatGPT assisting in situations where the root cause analysis involves multiple interconnected systems?
Thank you, Adam! When dealing with multiple interconnected systems, ChatGPT can help identify potential causes, dependencies, and interactions between them. It offers a holistic view by analyzing the relationships between different components and assisting in identifying the root cause more efficiently.
Fascinating article, Britton! How do you ensure that ChatGPT provides accurate insights and doesn't lead to false conclusions in root cause analysis?
Thank you, Sophia! Valid point. While ChatGPT is powerful, it's important to exercise caution. The accuracy relies on the quality and relevance of the training data. It's crucial to verify and validate the insights provided by ChatGPT using additional methods and subject matter expertise.
Excellent article, Britton! How can organizations integrate ChatGPT into their existing root cause analysis processes?
Thank you, Isabella! Integrating ChatGPT depends on the organization's setup. It can be used as a standalone tool or integrated into existing root cause analysis platforms. It's important to define clear guidelines on how to leverage ChatGPT effectively and combine its insights with human expertise.
Great article, Britton! How does ChatGPT handle the identification of root causes in situations where limited data is available?
Thank you, Liam! When dealing with limited data, ChatGPT relies on its training on diverse examples to make informed suggestions. However, it's important to note that the accuracy of its insights may be affected when there's insufficient data available. In such cases, human expertise becomes even more crucial.
Intriguing approach, Britton! Can ChatGPT assist in real-time root cause analysis during incidents, or is it primarily used for post-analysis?
Thank you, Chloe! ChatGPT can be used in real-time root cause analysis during incidents by providing insights and suggestions as the incident unfolds. It offers a valuable tool to support incident response teams and aid in faster identification of root causes.
Informative article, Britton! What are the key factors to consider when implementing ChatGPT for root cause analysis?
Thank you, Oliver! Key factors to consider include training ChatGPT on relevant data, ensuring data privacy and security, defining the scope of its use, providing clear guidelines to users, and regularly evaluating its effectiveness to make necessary improvements.
Great work, Britton! Could you share some use cases/examples where ChatGPT has significantly expedited root cause analysis?
Thank you, Sophie! One example is in identifying performance bottlenecks in large-scale distributed systems. ChatGPT helps in analyzing various metrics, suggesting potential causes, and assisting in identifying the root cause faster. It has also shown value in troubleshooting complex network issues and application failures.
Impressive article, Britton! Do you foresee any future developments or advancements in the field of root cause analysis with AI?
Thank you, Luke! Absolutely, the field of root cause analysis with AI is continuously evolving. We can expect advancements in more specialized and domain-specific models, improved training techniques, and integration of AI with existing analysis platforms. This will enhance the accuracy and effectiveness of root cause analysis in various industries.
Great insights, Britton! Were there any challenges you faced while implementing ChatGPT in root cause analysis, and how were they overcome?
Thank you, Samantha! One challenge was ensuring the quality and diversity of training data to cover a wide range of scenarios. Another challenge was managing user expectations and understanding the limitations of ChatGPT's insights. These challenges were addressed through careful data selection and user education about the tool's capabilities.
Fascinating approach, Britton! Could ChatGPT be used to assist in other problem-solving tasks beyond root cause analysis?
Thank you, Olivia! Absolutely, ChatGPT can assist in various problem-solving tasks beyond root cause analysis. Its ability to engage in natural language conversations makes it applicable in areas like troubleshooting, decision support, and providing recommendations based on complex datasets.
Insightful article, Britton! Have you encountered any ethical considerations or biases while using ChatGPT for root cause analysis?
Thank you, Aiden! Ethical considerations and biases are important aspects to address. ChatGPT can reflect and amplify biases present in the training data, leading to biased insights. To mitigate this, it's crucial to have diverse and representative training data and continuously evaluate and improve the model to reduce biases.
Informative article, Britton! How does the integration of ChatGPT in root cause analysis impact the overall time required for resolution?
Thank you, Ethan! ChatGPT can significantly reduce the time required for resolution by providing insights, suggestions, and narrowing down potential causes early in the analysis process. It assists analysts in identifying the root cause faster and enables them to focus their efforts on resolving the issue promptly.
Great read, Britton! Are there any privacy concerns related to using ChatGPT in root cause analysis?
Thank you, Jessica! Privacy concerns are important to address when using ChatGPT. It's crucial to handle sensitive data appropriately, especially if using ChatGPT in real-time analysis. Anonymizing and securing the data, adhering to privacy policies, and ensuring compliance with relevant regulations are key aspects to consider.
Impressive work, Britton! Have you measured the success rate of ChatGPT in accurately identifying root causes compared to traditional approaches?
Thank you, Lily! Measuring the success rate is an essential part of evaluation. In our experiments, ChatGPT has shown promising results in accurately identifying root causes. However, it's important to note that its effectiveness may vary depending on the complexity of the problem and availability of relevant training data.
Informative article, Britton! Are there any constraints or requirements for implementing ChatGPT in terms of computational resources?
Thank you, James! ChatGPT's computational resource requirements depend on the scale of the analysis and deployment setup. It can be resource-intensive for real-time analysis involving large volumes of data. However, there are techniques like optimizing model size, efficient data processing, and scalable infrastructure that can help manage resource constraints.
Great article, Britton! How do you handle situations where ChatGPT provides multiple possible causes that seem likely?
Thank you, Emma! When ChatGPT provides multiple possible causes, it becomes important to further analyze and validate the likelihood of each one. This can involve exploring additional data, consulting subject matter experts, or running automated tests to narrow down the possibilities and identify the most probable root cause.
Insightful approach, Britton! In what situations would you recommend using ChatGPT as the primary tool for root cause analysis, and when should it be used in combination with traditional methods?
Thank you, Benjamin! ChatGPT can be the primary tool for root cause analysis when dealing with known problem patterns or well-defined systems. However, in complex scenarios or for tackling novel issues, combining ChatGPT's insights with traditional methods and expert knowledge ensures a more comprehensive analysis, minimizing the risk of missing critical details.
Excellent article, Britton! How do you address potential biases introduced by analysts during the conversations with ChatGPT for root cause analysis?
Thank you, Emily! Analyst bias is an important consideration. Training analysts on the appropriate use of ChatGPT and providing guidelines for unbiased analysis helps mitigate this risk. Regular evaluations and feedback loops also play a critical role in identifying and addressing any unintended biases introduced during conversations.
Great work, Britton! How do you foresee ChatGPT evolving in the future to further enhance root cause analysis?
Thank you, Oliver! In the future, ChatGPT can evolve by integrating more contextual information, such as system event logs and historical data, to improve its insights. It can also benefit from advancements in explainable AI, enabling better understanding of its reasoning process and helping analysts trust its suggestions.
Informative article, Britton! How does ChatGPT handle situations where the root cause of a problem is a combination of multiple factors?
Thank you, Samantha! ChatGPT can handle situations involving multiple factors by identifying potential causes and interactions between them. However, when dealing with complex combinations of causes, human expertise is still valuable in understanding the interdependencies and identifying the multifactorial root cause.
Impressive work, Britton! Are there any specific industries or domains where ChatGPT has shown remarkable effectiveness in root cause analysis?
Thank you, Daniel! ChatGPT has shown remarkable effectiveness in industries that involve complex systems and extensive data analysis, such as IT operations, network infrastructure management, and cloud services. However, its applicability extends beyond these industries, and it can be valuable in various technology-dependent domains.
Great article, Britton! How do you address the challenge of explainability when using ChatGPT for root cause analysis?
Thank you, Emily! Explainability is an important aspect. ChatGPT's reasoning process can be enhanced by providing explanations along with its suggestions. Techniques like attention visualization and generating explanations from training data can help analysts understand how ChatGPT arrives at its insights, providing a clearer picture of the reasoning involved.
Insightful approach, Britton! Besides providing analysis assistance, can ChatGPT assist in documenting the root cause analysis process?
Thank you, Ella! ChatGPT can assist in documenting the root cause analysis process by generating summaries of conversations, capturing the key points discussed, and providing structured reports. This helps in maintaining a clear record of the analysis steps taken, aiding in knowledge sharing, and facilitating collaboration among analysts.
Great insights, Britton! How do you ensure the accuracy of the training data used for ChatGPT in root cause analysis?
Thank you, Ava! Ensuring the accuracy of the training data involves careful curation and validation. The data should cover a wide range of scenarios, encompassing both common and rare issues. It's important to annotate and validate the data to ensure accurate training and minimize the chances of incorrect or biased predictions.
Informative article, Britton! Can ChatGPT be vulnerable to adversarial attacks that might compromise the root cause analysis process?
Thank you, Leo! Adversarial attacks are a valid concern. ChatGPT can be susceptible to intentionally crafted inputs meant to mislead or manipulate its responses. Ensuring robustness against such attacks requires ongoing research and development of countermeasures, continuous evaluation of the model's performance, and secure implementation to prevent compromise.
Great work, Britton! How does ChatGPT handle situations where the root cause analysis involves time series data?
Thank you, Henry! ChatGPT can handle situations involving time series data by analyzing the trends, patterns, and anomalous behavior within the data. It can assist in identifying potential causes based on temporal relationships and suggest possible root causes that align with the observed patterns over time.
Impressive article, Britton! Can ChatGPT be trained to specialize in specific technology stacks or domains?
Thank you, Mia! Yes, ChatGPT can be trained to specialize in specific technology stacks or domains. By fine-tuning on domain-specific data and incorporating specialized knowledge, ChatGPT's insights can be tailored to address the nuances and specific challenges of the target technology stack or domain, enhancing the effectiveness of root cause analysis.
Great article, Britton! What are the potential challenges organizations might face when adopting ChatGPT for root cause analysis?
Thank you, Natalie! Some potential challenges organizations may face include integrating ChatGPT into their existing infrastructure, ensuring proper training and education of analysts, addressing data privacy and security concerns, and managing user expectations and reliance on ChatGPT's insights. Overcoming these challenges requires careful planning, training, and adaptation to the organization's needs.
Insightful work, Britton! Can ChatGPT provide recommendations or best practices to prevent recurring root causes in technology?
Thank you, Mason! ChatGPT can provide recommendations and best practices based on its training on historical data and patterns. It can suggest preventive actions, highlight areas of improvement, and propose strategies to mitigate the likelihood of recurring root causes. These recommendations can assist in building proactive approaches to technology maintenance and problem-solving.
Great insights, Britton! How do you ensure the secure deployment and use of ChatGPT in sensitive environments?
Thank you, Eva! Securing ChatGPT in sensitive environments involves measures like using secure communication channels, ensuring access control and authentication mechanisms, and implementing robust data privacy measures. Adhering to industry standards, conducting security audits, and following best practices in secure AI deployment are important steps to ensure the safe use of ChatGPT.
Impressive article, Britton! How can organizations measure the effectiveness of ChatGPT in their root cause analysis workflow?
Thank you, Oliver! Measuring effectiveness involves evaluating factors such as the accuracy of identified root causes, reduction in resolution time, analyst feedback, and comparing the outcomes with known incidents where ChatGPT was not used. Conducting post-implementation assessments and gathering feedback from analysts are crucial in determining the impact of ChatGPT in the overall analysis workflow.
Great work, Britton! How do you address potential language limitations or challenges when using ChatGPT?
Thank you, Ethan! Language limitations can arise due to the training data and the model's focus. Addressing these challenges involves training ChatGPT on diverse language samples and incorporating a wide range of technical terminology. Continuous evaluation and updates to the training data help in enhancing ChatGPT's language understanding and improving its ability to tackle technology-related queries.
Informative article, Britton! How does ChatGPT handle situations where the root cause analysis involves complex, non-linear relationships between components?
Thank you, Sophia! ChatGPT can handle complex, non-linear relationships between components by analyzing patterns, dependencies, and interactions within the available data. It can suggest potential causes based on the combined effects analyzed across multiple components, helping analysts navigate the non-linearity and narrowing down possible root causes more effectively.
Insightful approach, Britton! How do you ensure that ChatGPT remains up-to-date and knowledgeable about evolving systems?
Thank you, Aiden! Ensuring ChatGPT remains up-to-date involves regular updates to its training data to capture recent events and system changes. Incorporating real-time data feeds, system logs, and integration with monitoring tools can help keep ChatGPT knowledgeable about evolving systems. Continuous learning and feedback loops from analysts also contribute to its knowledge update process.
Great insights, Britton! Can ChatGPT be used as a collaborative tool, allowing multiple analysts to interact with it simultaneously for root cause analysis?
Thank you, Ella! Absolutely, ChatGPT can be used as a collaborative tool where multiple analysts can interact with it simultaneously. It enables real-time collaboration, fosters knowledge sharing and synergy among analysts, and provides a shared context for collective root cause analysis efforts.
Impressive work, Britton! How can organizations prepare their analysts to effectively leverage the capabilities of ChatGPT in root cause analysis?
Thank you, Olivia! Preparing analysts involves providing training on using ChatGPT, its capabilities, limitations, and its integration with existing analysis processes. Hands-on practice, simulated scenarios, and continuous feedback help analysts become proficient in leveraging ChatGPT effectively, combining its insights with their expertise, and making better-informed decisions during root cause analysis.
Informative article, Britton! Can ChatGPT be adapted to different organizational workflows and root cause analysis methodologies?
Thank you, Sophie! Yes, ChatGPT can be adapted to different organizational workflows and methodologies. Its flexibility allows integration into existing processes, such as incident management or problem-solving frameworks. By aligning its usage with specific methodologies and tailoring its training on relevant datasets, ChatGPT becomes an adaptable tool, enhancing the existing root cause analysis processes.
Great article, Britton! How do you manage the scalability of ChatGPT when dealing with a large number of simultaneous root cause analysis requests?
Thank you, Oliver! Managing scalability involves designing an infrastructure that can handle concurrent requests by distributing the load across multiple instances of ChatGPT. Techniques like load balancing, optimizing resource allocation, and utilizing cloud-based services contribute to ensuring the scalability and responsiveness of ChatGPT in large-scale root cause analysis scenarios.
Insightful work, Britton! How do you ensure the transparency of ChatGPT's decision-making process in root cause analysis?
Thank you, Aria! Ensuring transparency involves techniques like generating explanations for ChatGPT's responses, visualizing attention mechanisms to highlight relevant information, and fostering interpretability in its reasoning process. These measures help analysts understand how ChatGPT arrives at its suggestions, increasing transparency and building trust in its decision-making process.
Great insights, Britton! Can ChatGPT be integrated with automation tools to enable automatic resolution of identified root causes?
Thank you, Lily! ChatGPT's insights can be integrated with automation tools to enable automatic resolution of identified root causes. By connecting with orchestration systems, workflow automation, or support ticketing systems, ChatGPT's suggestions can trigger predefined actions and assist in faster resolution, reducing manual effort and accelerating the impact of root cause analysis.
Impressive article, Britton! Can ChatGPT adapt to the evolving jargon and technical language used in the technology industry?
Thank you, Oliver! ChatGPT can adapt to evolving jargon and technical language by incorporating up-to-date training data that reflects industry changes. Continuous learning and updates to its language model help ChatGPT stay current with industry terms and improve its understanding of the evolving language used in the technology industry.
Informative article, Britton! Can ChatGPT assist in identifying root causes for recurring issues in technology systems?
Thank you, Sophia! ChatGPT can assist in identifying root causes for recurring issues by analyzing historical data, identifying patterns, and suggesting potential causes that align with the recurring nature of the problems. By capturing the context of previous incidents, ChatGPT contributes to understanding and addressing the root causes leading to repetitive issues.
Great work, Britton! Can ChatGPT be integrated with data visualization tools to provide a more comprehensive analysis of root causes?
Thank you, Daniel! Absolutely, ChatGPT's insights can be combined with data visualization tools to provide a more comprehensive analysis of root causes. Visualizing ChatGPT's suggestions alongside relevant data and metrics enhances the understanding of the relationships and dependencies, facilitating a more holistic and interactive exploration of the root cause landscape.
Insightful article, Britton! How can organizations ensure the availability of sufficient training data for ChatGPT in their specific technology context?
Thank you, Emily! Ensuring sufficient training data involves a combination of using publicly available datasets, curating domain-specific data, and collecting anonymized data from internal incidents. Organizations should establish processes to regularly update and expand their training data to cover a wide range of scenarios, ensuring ChatGPT's relevance and accuracy in their specific technology context.
Great insights, Britton! How does ChatGPT handle situations where the root cause analysis requires deep knowledge of specific components or technologies?
Thank you, Oliver! ChatGPT handles situations requiring deep knowledge by leveraging its training on relevant data, including technical documentation, guidelines, and historical incidents. While it can provide valuable insights, domain experts' input plays a crucial role in addressing the complexity associated with deep knowledge of specific components or technologies during root cause analysis.
Impressive work, Britton! Have you encountered any limitations or challenges in the adoption of ChatGPT for root cause analysis?
Thank you, Sophie! Adoption challenges can include skepticism or resistance from analysts, integrating ChatGPT into existing workflows, managing user expectations, and addressing the limitations of the model. Proactive communication, training, monitoring, and continuous improvement help overcome these, making adoption smoother and ensuring effective utilization of ChatGPT's capabilities.
Informative article, Britton! Can ChatGPT learn from feedback provided by analysts to improve its performance?
Thank you, Olivia! Yes, ChatGPT can learn from feedback provided by analysts to improve its performance. Analyst feedback helps refine its responses, identify any biases, and enhance its reasoning process. Incorporating a feedback loop that includes continuous evaluation, updates, and model improvements helps ChatGPT adapt and deliver more accurate and valuable insights in root cause analysis.
Great article, Britton! What are the potential risks associated with over-reliance on ChatGPT in root cause analysis?
Thank you, Maxwell! Over-reliance on ChatGPT can pose risks like overlooking critical details specific to certain incidents, potential biases in the training data, or the model's limited coverage of recent events. It's important to maintain a balance between leveraging ChatGPT's capabilities and involving human expertise to safeguard against these risks and ensure comprehensive analysis.
Thank you all for taking the time to read my article on enhancing root cause analysis with ChatGPT! I'm excited to hear your thoughts and engage in discussion.
Great article, Britton! I really enjoyed reading it. The use of ChatGPT seems like a promising approach to improving root cause analysis in technology. I'm curious to know if there are any limitations or challenges when using this method?
Hi Sarah! Thank you for your positive feedback. While ChatGPT has proven to be effective in many cases, there are a few limitations we should consider. Firstly, it heavily relies on the quality of the training data, which means biases present in the data may influence its responses. Also, as with any AI model, there can always be cases where it fails to provide accurate or relevant answers. Continuous improvement and refinement of the model are crucial to mitigate these challenges.
Impressive article, Britton! ChatGPT does seem like a game-changer. How do you think the implementation of this approach will impact the efficiency of root cause analysis in practice?
Hi Jonathan! Thank you for your kind words. The implementation of ChatGPT can greatly enhance the efficiency of root cause analysis. By leveraging its conversational nature, analysts can explore different hypotheses and ask specific questions to uncover potential causes more effectively. It can also serve as a valuable tool for knowledge transfer among team members and facilitate collaborative problem-solving.
Interesting article, Britton! I'm wondering if ChatGPT is capable of handling complex technical concepts and jargon?
Hi Emily! That's a great question. ChatGPT has the ability to handle complex technical concepts and jargon to a certain extent. However, it's important to note that its responses are generated based on patterns learned from training data, so there might be cases where it struggles with highly specialized knowledge or niche technical details. It's always beneficial to combine the model's insights with human expertise for a comprehensive analysis.
Fantastic article, Britton! Do you have any recommendations on how to integrate ChatGPT into existing root cause analysis workflows?
Hi Daniel! Thank you for your kind words. Integrating ChatGPT into existing workflows can be done by incorporating it as a decision support tool. Analysts can use it to brainstorm ideas, validate hypotheses, or seek alternative perspectives during the root cause analysis process. It can be integrated into collaborative platforms or as a standalone tool with a user-friendly interface to provide real-time assistance to analysts.
Excellent article, Britton! I'm curious about the training data used for ChatGPT. Where does it come from, and how can we ensure its reliability and relevance?
Hi Sophia! Thank you for your question. The training data for ChatGPT is sourced from a wide range of internet text, which includes books, websites, and articles. To ensure its reliability and relevance, the data goes through extensive preprocessing and filtering. However, it's important to note that biases can still be present in the training data, which may impact the model's responses. Ongoing monitoring and updating of the training data are essential to maintain accuracy and mitigate biases.
Fascinating article, Britton! How would you recommend organizations approach implementing ChatGPT in terms of data privacy and security?
Hi Adam! Great question. Implementing ChatGPT in organizations should prioritize data privacy and security. Organizations should carefully evaluate the data they provide to the model and ensure sensitive information is not exposed. Depending on the requirements, deploying ChatGPT on-premises or using advanced encryption techniques to protect data in transit and at rest could be explored. It's crucial to follow best practices and adhere to relevant privacy regulations to safeguard sensitive information.
Well-written article, Britton! Have you considered any potential ethical concerns that might arise with the use of ChatGPT in root cause analysis?
Hi Olivia! Ethical concerns are indeed an important aspect to consider when using AI models like ChatGPT. One concern is the potential amplification of existing biases present in the training data. Careful attention should be given to ensure that the model's responses are fair, unbiased, and inclusive. Transparency in the deployment of the AI model and actively seeking user feedback can help address these concerns. Ongoing evaluation and audits of the model's performance are essential to identify and mitigate any ethical concerns.
Insightful article, Britton! Do you have any insights on how ChatGPT can handle real-time analysis during incidents?
Hi Liam! Thank you for your feedback. ChatGPT can indeed be valuable for real-time analysis during incidents. It can assist in identifying potential causes, providing guidance on troubleshooting steps, and offering relevant information to support incident response efforts. By quickly surfacing relevant knowledge and insights, ChatGPT can help incident responders make informed decisions and mitigate the impact of incidents more effectively.
Informative article, Britton! How would you recommend evaluating the effectiveness and success of ChatGPT in enhancing root cause analysis?
Hi Grace! Evaluating the effectiveness of ChatGPT in enhancing root cause analysis can be done through several approaches. Firstly, analysts can track the accuracy and relevance of the model's responses compared to manual analysis. They can also measure the time saved and the quality of insights gained by incorporating ChatGPT. Additionally, gathering feedback from analysts who use ChatGPT regularly can provide valuable insights into its impact and success. Continuous monitoring of its performance and addressing any limitations identified will ensure its continual improvement.
Really interesting article, Britton! How do you think the use of ChatGPT will impact the skill sets and roles of technology analysts?
Hi Ethan! Thank you for your comment. The use of ChatGPT can augment the skill sets and roles of technology analysts. It shifts their focus from repetitive and time-consuming tasks to higher-level analysis and problem-solving. Analysts can leverage ChatGPT's capabilities to explore broader ranges of possibilities and spend more time on critical thinking and decision-making. This technology can empower analysts to become more effective and strategic in their roles, enhancing their overall contribution to root cause analysis.
Well-argued article, Britton! Are there any specific industries or domains where ChatGPT is particularly well-suited for root cause analysis?
Hi Sophie! Thank you for your feedback. ChatGPT can be applied to a wide range of industries and domains for root cause analysis. However, it tends to be most effective in domains with well-defined problem structures and where textual data plays a significant role. It has shown promising results in technology, customer support, healthcare, and finance industries, but its applicability is not restricted to these areas. With proper training and customization, it can be tailored to specific industries and domains for more accurate and relevant analysis.
Engaging article, Britton! How does ChatGPT handle unstructured data, such as log files or system event data?
Hi Noah! Great question. ChatGPT can handle unstructured data like log files or system event data by utilizing the context provided in the conversation. Analysts can provide specific details or extracts from the unstructured data and ask relevant questions to gain insights. While it may not directly analyze the raw data itself, it can assist in understanding patterns, suggesting potential causes, or connecting relevant concepts based on the provided information.
Informative article, Britton! How would you recommend addressing the issue of explainability when using ChatGPT for root cause analysis?
Hi Lucy! Explainability is indeed an important consideration when using AI models like ChatGPT. To address this, organizations can adopt methodologies like rule-based post-processing or generating explanations alongside model responses. By incorporating interpretability techniques, analysts can understand the reasoning behind ChatGPT's suggestions and better validate its outputs. Ensuring transparency in the decision-making process and encouraging collaboration between ChatGPT and analysts can help address the issue of explainability.
Excellent article, Britton! How can organizations manage the risks associated with relying on an AI model like ChatGPT for root cause analysis?
Hi Daniel! Managing the risks associated with relying on ChatGPT for root cause analysis is vital. First, organizations should ensure human oversight and validation of its outputs to mitigate potential errors or biases. Regular validation against manual analysis should be conducted to evaluate the model's performance and identify any discrepancies. Additionally, maintaining a feedback loop with analysts and users of ChatGPT can help identify any emerging risks and address them promptly. Ongoing monitoring, auditing, and continuous improvement of the model will ensure better risk management.
Insightful article, Britton! How do you think ChatGPT will evolve in the future to further enhance root cause analysis?
Hi Amelia! Thank you for your comment. In the future, ChatGPT is likely to continue evolving to enhance root cause analysis. We can expect improvements in the model's understanding of niche technical knowledge, context-dependent responses, and the ability to handle a wider range of domains and industries. Increasing efforts in training data quality, reducing biases, and incorporating user feedback will contribute to refining the model's performance. Integration with other analytical tools and technologies may also enhance its capabilities in providing more comprehensive and accurate root cause analysis insights.
Fantastic article, Britton! How can organizations ensure the reliability and consistency of ChatGPT in root cause analysis over time?
Hi Joshua! Ensuring the reliability and consistency of ChatGPT in root cause analysis over time requires ongoing management and monitoring. Organizations should invest in regular model updates and retraining to keep the model up-to-date with the latest knowledge and reduce the risk of model drift. Gathering user feedback and conducting periodic evaluations can help identify any performance deviations or potential issues. Maintaining a feedback loop with analysts and incorporating their domain expertise is crucial for refining and fine-tuning the model's responses and ensuring its reliability and consistency.
Engaging article, Britton! Can you share some real-world use cases where ChatGPT has been successfully implemented for root cause analysis?
Hi Victoria! Absolutely. ChatGPT has been successfully implemented in various real-world use cases for root cause analysis. One example is in a technology support organization where analysts use ChatGPT to troubleshoot complex issues and identify underlying causes more efficiently. Another use case is in incident response teams, where ChatGPT assists in providing real-time insights during critical incidents, helping responders quickly understand the root cause and make informed decisions. These are just a few examples, and the applicability of ChatGPT can extend to various industries and domains.
Informative article, Britton! Are there any potential risks associated with relying solely on ChatGPT for root cause analysis?
Hi Isaac! Relying solely on ChatGPT for root cause analysis does come with potential risks. While it can provide valuable insights, there is always a possibility of incorrect or incomplete results due to the model's limitations. Organizations should ensure human oversight and validation to minimize the risk of inaccurate analysis. Additionally, clear communication with stakeholders about the model's capabilities and limitations is crucial to manage expectations. Combining ChatGPT with human expertise and utilizing it as a decision support tool will help mitigate these risks.
Well-analyzed article, Britton! Can you share any recommendations on how organizations can ensure a smooth adoption of ChatGPT for root cause analysis?
Hi James! Thank you for your feedback. Organizations can ensure a smooth adoption of ChatGPT for root cause analysis by following a few key recommendations. Firstly, providing proper user training and education on how to effectively utilize the tool will facilitate its adoption. Establishing clear guidelines and best practices on when and how to involve ChatGPT in the root cause analysis workflow is also essential. Lastly, fostering a culture of collaboration and feedback between analysts and the AI model will drive its successful adoption and integration into existing processes.
Informative article, Britton! How does ChatGPT handle situations where the root cause analysis requires domain-specific knowledge or expertise?
Hi Lily! ChatGPT can handle situations where domain-specific knowledge or expertise is required to some extent. However, in cases that demand highly specialized knowledge, it's important to combine ChatGPT's insights with human expertise. Analysts can provide additional context and guide the conversation to explore relevant domain-specific factors. By collaborating with human analysts, ChatGPT can assist in uncovering potential causes and provide valuable insights, even in complex scenarios that require domain knowledge beyond its training data.
Insightful article, Britton! How can organizations ensure the ethical and responsible use of ChatGPT in root cause analysis?
Hi Hannah! Ensuring the ethical and responsible use of ChatGPT in root cause analysis requires a few critical practices. Organizations should prioritize transparency in explaining the involvement of AI models and the limitations of ChatGPT. They should also establish clear guidelines and boundaries for the use of the tool to avoid overreliance or potential misuse. Additionally, organizations should actively seek user feedback, monitor the model's performance for biases, and proactively address any emerging ethical concerns. By fostering an ethical and responsible AI culture, organizations can utilize ChatGPT responsibly and address any ethical considerations.
Great article, Britton! What are the key prerequisites for implementing ChatGPT effectively in root cause analysis?
Hi Aiden! Thank you for your comment. Implementing ChatGPT effectively in root cause analysis requires a few key prerequisites. Firstly, organizations should have a well-defined root cause analysis process and an established knowledge base. Clear documentation and knowledge sharing practices are also important. Additionally, having relevant training data and resources to fine-tune the model to specific needs can enhance its accuracy and relevance. Having a strong feedback loop for continuous improvement and commitment to ongoing model management are crucial prerequisites to ensure its effectiveness in root cause analysis.
Fantastic article, Britton! How can organizations make ChatGPT more adaptable to different industries or organizational contexts for root cause analysis?
Hi Sophia! Making ChatGPT more adaptable to different industries or organizational contexts for root cause analysis can be achieved through customization and fine-tuning. By training the model on domain-specific data and incorporating industry-specific terminology, the relevance and accuracy of its responses can be improved. Feedback from analysts and continuous refinement of the model based on user needs will help align it with the specific requirements of each industry or organizational context. Being proactive in gathering user feedback and addressing customization needs will enhance the adaptability of ChatGPT.
Great article, Britton! Are there any potential biases that ChatGPT might introduce in the root cause analysis process?
Hi Thomas! ChatGPT has the potential to introduce biases if not carefully managed. Since it learns from large amounts of internet text, biases present in the training data can be reflected in its responses. Organizations should be vigilant in monitoring and addressing any biases that may arise to ensure fairness and impartiality in the root cause analysis process. By maintaining a diverse and representative training dataset, actively addressing biases in the responses, and encouraging diverse perspectives during analysis, organizations can mitigate the introduction of biases by ChatGPT.
Thank you all for your valuable comments and engaging in this discussion on the use of ChatGPT for enhancing root cause analysis in technology! Your insights and questions are greatly appreciated. If you have any further thoughts or questions, feel free to continue the conversation.