Enhancing Sentiment Analysis in Dirección de Marketing with ChatGPT: A Game-changer in Technology
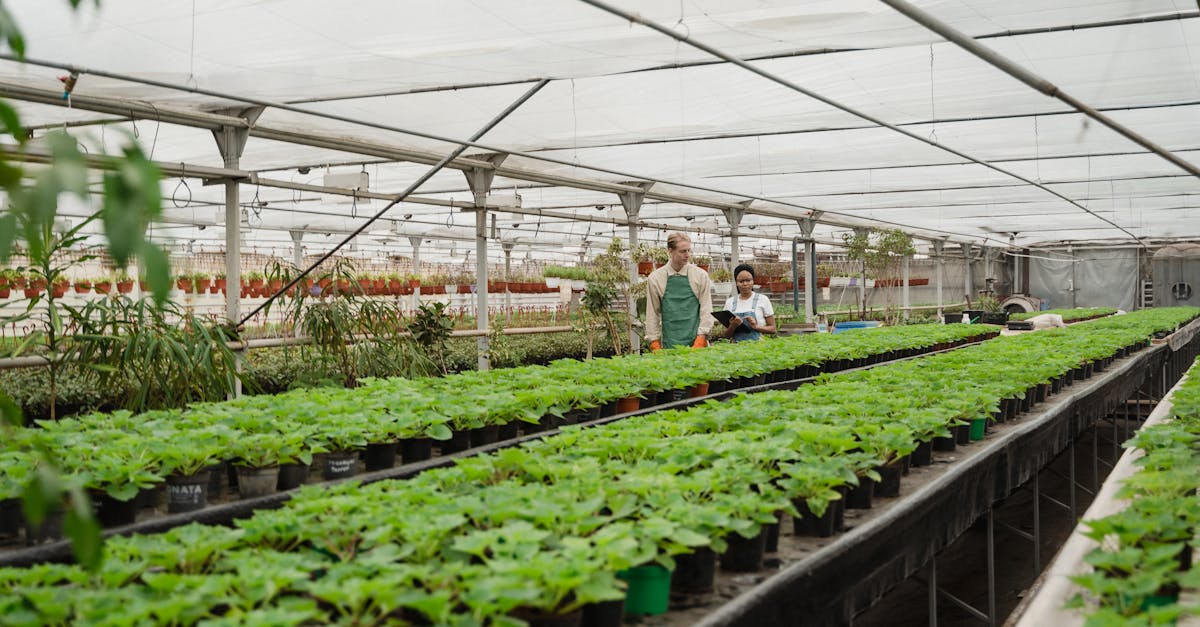
Dirección de marketing, or marketing management, is a critical component of any business strategy. It involves planning, implementing, and controlling activities related to the marketing of products or services. One important aspect of marketing management is understanding customer sentiment towards a product or brand.
What is Sentiment Analysis?
Sentiment analysis is a technology that utilizes natural language processing, computational linguistics, and text analytics to determine the emotional tone behind a series of words or text. It can analyze customer reviews, social media comments, surveys, and other textual data to identify the sentiment expressed by customers.
Usage in Dirección de Marketing
In the field of marketing management, sentiment analysis can be a valuable tool to gain insights into customer perceptions and opinions. By understanding how customers feel about a product or brand, marketers can make more informed decisions and develop targeted strategies.
Here are some specific ways sentiment analysis can be used in Dirección de Marketing:
1. Brand Perception
By analyzing customer reviews and social media comments, marketers can understand how customers perceive their brand. Sentiment analysis can help identify positive sentiments, negative sentiments, or even nuances in customer opinions. This information can guide marketers in improving brand messaging, addressing negative feedback, and enhancing overall brand perception.
2. Product Feedback
Sentiment analysis can analyze customer reviews or feedback regarding specific products. By categorizing sentiments expressed in these reviews, marketers can identify areas of improvement, discover product strengths, and make data-driven decisions regarding product development or modification.
3. Competitor Analysis
By monitoring sentiment towards competitors' products or brands, marketers can gain competitive intelligence. Sentiment analysis can reveal customer satisfaction levels, areas where competitors excel, and identify gaps in the market that can be targeted for competitive advantage.
4. Campaign Effectiveness
Sentiment analysis can gauge customer reactions to marketing campaigns, advertisements, or promotions. By analyzing social media comments or survey responses, marketers can assess the success of their campaigns and make necessary adjustments to improve effectiveness.
Overall, sentiment analysis is a powerful technology that can provide valuable insights for marketing management in Dirección de Marketing. By understanding customer sentiment towards a product or brand, marketers can make informed decisions, improve brand perception, enhance products, stay competitive, and run more effective marketing campaigns.
Comments:
Thank you all for reading my article on enhancing sentiment analysis with ChatGPT! I'm excited to hear your thoughts and engage in a discussion.
Great article, David! Sentiment analysis is such a crucial tool in marketing. How do you see ChatGPT specifically enhancing it?
Thanks, Mark! ChatGPT can enhance sentiment analysis by providing a more conversational approach. It allows for more dynamic interactions and can better capture the subtleties and nuances of sentiment.
I find sentiment analysis fascinating, especially in the context of marketing. David, could you give some real-world examples of how ChatGPT can improve existing sentiment analysis methods?
Certainly, Laura! One example is in analyzing customer reviews or feedback. ChatGPT can better understand the context and provide more accurate sentiment analysis by considering the entire conversation, rather than just individual sentences or phrases.
Another example is in social media monitoring. ChatGPT can handle the conversational nature of social media posts and comments, allowing for better sentiment analysis on platforms like Twitter or Facebook.
I'm curious about the potential limitations of using ChatGPT for sentiment analysis. David, what challenges should we be aware of?
Good question, Sophia! One limitation is that ChatGPT may sometimes generate responses that seem plausible but are not accurate when it comes to sentiment analysis. The model's reliance on patterns from its training data can occasionally result in false positives or negatives.
Additionally, ChatGPT may not handle sarcasm or irony effectively, which can impact the accuracy of sentiment analysis, as those are common in online communication.
This technology sounds promising, David. Do you think ChatGPT can also be used in analyzing sentiment for non-English languages?
Absolutely, Michael! ChatGPT can be fine-tuned for specific languages, making it suitable for sentiment analysis in multiple languages. However, the quality of analysis may vary based on the language and availability of training data.
For widely spoken languages like English, ChatGPT tends to perform better due to the larger dataset it was trained on.
I wonder if privacy concerns arise when using ChatGPT for sentiment analysis? How is user data handled?
Privacy is important, Emily. As an AI researcher, I prioritize data protection. It's crucial to use ChatGPT in a way that respects user privacy and complies with relevant regulations. Care should be taken when handling sensitive user data during sentiment analysis.
It's recommended to anonymize or aggregate data whenever possible to minimize privacy risks.
David, what do you think the future holds for sentiment analysis? How will ChatGPT continue to shape the field?
Great question, Sophie! I believe sentiment analysis will become more context-aware and accurate with advances in models like ChatGPT. As we continue to refine and fine-tune these models, they will become integral to understanding sentiment across various platforms and industries.
ChatGPT's ability to handle conversational data and its potential for multilingual sentiment analysis make it a game-changer in the field, paving the way for more sophisticated and effective sentiment analysis techniques.
David, how does ChatGPT handle varying tones and emotions in sentiment analysis? Can it differentiate between positive and negative sentiments expressed with similar word choices?
Excellent question, John! ChatGPT can infer meaning based on the context surrounding words, allowing it to differentiate between varying tones and emotions. It considers the overall conversation to make a more accurate sentiment analysis, even when dealing with similar word choices.
This contextual understanding helps ChatGPT capture the intended sentiment beyond just the literal meaning of individual words or phrases.
This article has certainly piqued my interest! David, could you recommend any resources for further reading on sentiment analysis using ChatGPT?
Absolutely, Emily! Here are a few resources to get you started: 1. OpenAI's documentation on fine-tuning: [URL] 2. Research papers on sentiment analysis with language models: [URL] 3. Online forums and communities dedicated to NLP and sentiment analysis discussions.
These resources should provide you with more in-depth information and help you explore the potential of sentiment analysis using ChatGPT.
David, do you have any insights regarding ChatGPT's performance with domain-specific language, such as marketing jargon or industry-specific terms?
Good question, Anna! ChatGPT can perform well in analyzing sentiment for domain-specific language. However, fine-tuning the model on relevant data from the specific domain or industry can further improve its performance and understanding of specialized terms and jargon.
By fine-tuning on domain-specific data, ChatGPT can be a powerful sentiment analysis tool for marketing professionals and others working in specific fields.
David, what are your thoughts on the potential biases ChatGPT might introduce to sentiment analysis?
Great question, Sophia! Biases can be a concern with AI language models like ChatGPT. It's essential to carefully curate and balance the training data to reduce biases as much as possible. Continued research and vigilance are necessary to ensure fair and unbiased sentiment analysis.
OpenAI is actively working towards addressing biases and making language models more fair and equitable.
David, I'd like to know how ChatGPT compares to other sentiment analysis tools on the market. What sets it apart?
Good question, Robert! ChatGPT's advantage lies in its ability to handle conversational data and its conversational nature. Its contextual understanding gives it an edge over more traditional approaches that analyze sentiment based on individual sentences or phrases.
Furthermore, ChatGPT's flexibility allows it to be fine-tuned for multiple languages and domains, making it a versatile tool for sentiment analysis in various industries.
David, can ChatGPT also identify and analyze sentiment in images or videos, or is it limited to text-based sentiment analysis?
Good question, Liam! ChatGPT is primarily designed for text-based sentiment analysis. While there are other AI models specifically developed for analyzing visual content, ChatGPT's strength lies in its ability to analyze sentiment in textual data.
For image or video sentiment analysis, other models that specialize in visual data would be more suitable.
David, I'm curious about the user interface for applying ChatGPT in sentiment analysis. Is it through APIs or do you have a separate platform?
Good question, Olivia! OpenAI provides an API through which developers can integrate ChatGPT into their own platforms or applications for sentiment analysis. The API facilitates easy implementation of the model for real-world applications.
Additionally, OpenAI is working on a platform called 'ChatGPT Plus' that provides a user-friendly interface for directly interacting with ChatGPT for various purposes, including sentiment analysis.
David, do you have any recommendations for preprocessing text data to improve sentiment analysis results when using ChatGPT?
Certainly, Ethan! A few preprocessing steps can help improve results. Firstly, removing noisy or irrelevant data can enhance the accuracy of sentiment analysis. Additionally, tokenization and removing stop words can be beneficial for better analysis.
Experimenting with different preprocessing techniques to suit the specific requirements of the task and dataset is often necessary for optimal sentiment analysis leveraging ChatGPT.
David, can you shed some light on the training process involved in fine-tuning ChatGPT for sentiment analysis?
Certainly, Natalie! Fine-tuning ChatGPT involves training the model with additional dataset containing examples of sentiment-labeled texts. This process helps the model learn to recognize sentiment patterns and make accurate predictions specific to sentiment analysis.
During fine-tuning, a balance needs to be struck to avoid overfitting the model to the training data while still capturing the necessary sentiment analysis capabilities.
David, how does the accuracy of ChatGPT's sentiment analysis compare to traditional rule-based approaches or other AI models?
Excellent question, Sophia! Compared to traditional rule-based approaches, ChatGPT's sentiment analysis can be more accurate due to its ability to understand and capture nuanced context, resulting in better sentiment predictions.
As for other AI models, comparative performance depends on the specific models being compared. However, ChatGPT's conversational nature often enables it to outperform models that focus solely on individual sentences or phrases.
David, what are some limitations when it comes to scaling sentiment analysis using ChatGPT in real-time applications or large-scale data analysis?
Great question, Dylan! Real-time applications or large-scale data analysis using ChatGPT for sentiment analysis may face challenges related to computational resources and response time. Scaling the infrastructure and ensuring timely predictions for a large volume of data is a consideration for optimal performance.