Enhancing Sentiment Analysis in Process Analysis Technology with ChatGPT
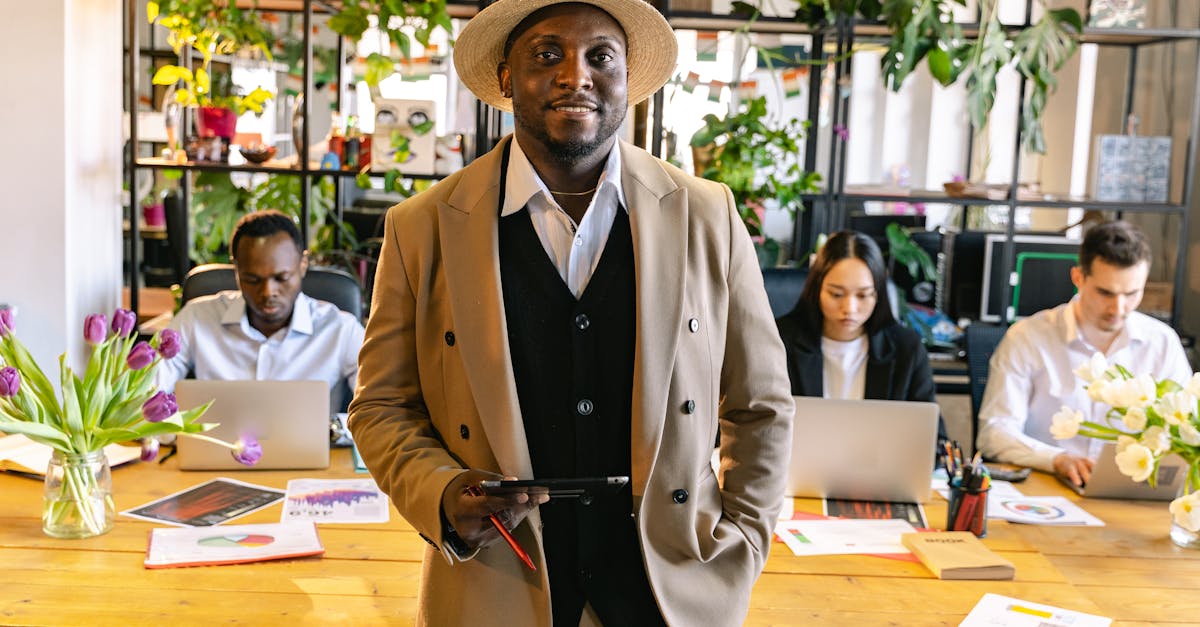
Technology: Process Analysis
Area: Sentiment Analysis
Usage: ChatGPT-4 can process text data, helping to understand public sentiment towards a topic, brand or product.
When it comes to understanding public sentiment towards a particular topic, brand, or product, sentiment analysis plays a crucial role. Sentiment analysis involves the use of natural language processing techniques to identify and extract subjective information from text data. One effective approach within sentiment analysis is process analysis.
What is Process Analysis?
Process analysis, also known as sequence analysis, is a method used to analyze the steps or processes involved in a particular task or activity. It aims to understand the order and dependencies of actions that occur to accomplish a goal. While process analysis is commonly associated with business process management and optimization, it has proven to be valuable in the field of sentiment analysis as well.
The Role of Process Analysis in Sentiment Analysis
In sentiment analysis, process analysis helps to uncover the sentiment expressed at each stage or step of a process. By analyzing the chronological order of actions taken in response to a topic, brand, or product, we can gain valuable insights into the sentiment evolution. This provides a deeper understanding of how people's emotions change over time in relation to the subject of interest.
With the advancement of technologies like ChatGPT-4, we can now leverage process analysis to analyze large volumes of text data and gain comprehensive insights into public sentiment. ChatGPT-4 is a language model that can process text data and understand the sentiments expressed towards a particular topic, brand, or product.
How ChatGPT-4 Utilizes Process Analysis
ChatGPT-4 utilizes process analysis techniques by analyzing the sequence of text inputs and extracting sentiment-related information from them. It can identify the sentiment expressed at different stages of a conversation, allowing for a nuanced understanding of sentiment dynamics.
By processing text data in real-time, ChatGPT-4 can provide valuable insights on how public sentiment evolves throughout a conversation. For example, it can detect when users transition from a negative sentiment towards a positive one or identify moments of frustration or satisfaction.
Benefits and Applications
The benefits of using process analysis in sentiment analysis are numerous. It allows businesses to track sentiment changes over time, identify critical points in a process that influence sentiment, and make informed decisions based on these insights.
By understanding public sentiment towards a topic, brand, or product, businesses can gauge customer satisfaction, predict trends, evaluate marketing campaigns, and even mitigate potential crisis situations.
Conclusion
Process analysis, when applied to sentiment analysis, provides a powerful framework for understanding public sentiment towards a topic, brand, or product. Technologies like ChatGPT-4 bring innovative capabilities to the field, allowing for real-time analysis of sentiment dynamics. By leveraging process analysis, businesses can gain valuable insights and make informed decisions that align with public sentiment.
Comments:
Thank you all for reading my article on enhancing sentiment analysis with ChatGPT! I'm excited to hear your thoughts and answer any questions you may have.
Great article, Mike! I've been using sentiment analysis in my work, but I haven't explored ChatGPT yet. How do you see ChatGPT improving the process analysis technology?
Thanks, Sarah! ChatGPT brings a conversational aspect to sentiment analysis. It can better understand the context of text, handle ambiguous language, and provide more accurate sentiment analysis in complex scenarios.
I agree, ChatGPT's ability to understand and generate human-like text makes it promising for sentiment analysis. It can capture subtle nuances and improve the overall accuracy of sentiment classification.
I'm curious about the training process for ChatGPT. How was it trained to understand sentiment and context effectively?
Good question, Emily. ChatGPT was pre-trained on a large corpus of text data and then fine-tuned using methods that involved reinforcement learning from human feedback. It learned to understand sentiment by using annotated data and can now effectively analyze sentiment in various contexts.
That's fascinating, Mike. How was the reinforcement learning process implemented in the training of ChatGPT?
During reinforcement learning, ChatGPT was given prompts and generated responses. These responses were then ranked by humans based on quality. The model was then fine-tuned using Proximal Policy Optimization to maximize the chances of generating high-quality responses with sentiment understanding.
I've used sentiment analysis in social media monitoring. How well does ChatGPT handle analyzing sentiment in shorter text snippets, like tweets or social media posts?
ChatGPT can handle analyzing sentiment in shorter text snippets quite well! In fact, it has been specifically fine-tuned to handle social media posts, tweets, and other similar content. It can account for the informal language, abbreviations, and emojis often found in those contexts.
That's impressive, Mike! Sentiment analysis on social media can be challenging due to the use of sarcasm and ambiguity. It would be interesting to see samples of ChatGPT's performance in those cases.
Absolutely, Sophie! I can provide you with some samples where ChatGPT successfully detects sarcasm and handles ambiguous language. It's a valuable feature that ensures accurate sentiment analysis in real-world social media applications.
Do you see any limitations or challenges that developers may encounter when implementing ChatGPT for enhanced sentiment analysis?
Certainly, Kate. One challenge is handling explicit and implicit biases present in the training data. Developers need to be cautious and ensure they mitigate biases to prevent unfair or unreliable sentiment analysis results. It requires careful data selection, augmentation, and post-training techniques to address this issue.
Are there any privacy concerns when using ChatGPT for sentiment analysis? How does it handle sensitive or personal data?
Great question, Olivia! ChatGPT does not store any user inputs and the decoding process is stateless, ensuring privacy. However, it's important for developers to handle sensitive or personal data before feeding it to the model, following proper privacy guidelines and regulations.
Congratulations on the article, Mike! How do you see the future of sentiment analysis evolving with the advancements in language models like ChatGPT?
Thank you, Jacob! With language models like ChatGPT, sentiment analysis will continue to improve in accuracy and applicability. As models become more fine-tuned and capable of understanding complex human language, we can uncover more insights from textual data, leading to better decision-making and enhanced user experiences.
I agree, Mike. Sentiment analysis has enormous potential in various domains like customer feedback analysis, brand monitoring, and market research. The advancements in language models will only amplify its impact and usefulness.
Mike, how can businesses and developers effectively incorporate ChatGPT for sentiment analysis? Any best practices you recommend?
Sarah, it's essential to start with a well-defined goal and dataset. Developers should evaluate the model's performance on their specific use cases and domain. Iterative testing and evaluation are crucial to identify areas for improvement. Keep in mind the importance of addressing biases and ensuring privacy throughout the implementation process.
What are the limitations of ChatGPT when it comes to sentiment analysis? Are there any scenarios where it might struggle?
Emily, while ChatGPT performs well, there are contexts where it might struggle. It can still be sensitive to nuances and might not grasp highly specific domain-related sentiments. Additionally, very long documents or those with excessive noise might pose challenges. It's crucial to monitor and evaluate performance based on the specific application.
Mike, can you provide more details about the technology stack behind ChatGPT that enables effective sentiment analysis?
Certainly, David! ChatGPT utilizes a transformer-based deep learning model, specifically the GPT (Generative Pre-trained Transformer) architecture. It leverages attention mechanisms and fine-tuning methods to interpret and analyze text for sentiment. The overall technology stack involves libraries like TensorFlow and PyTorch.
Thanks for the details, Mike. How resource-intensive is ChatGPT when deployed for sentiment analysis? Does it need significant computational power?
Jessica, deploying ChatGPT for sentiment analysis usually requires significant computational power due to the size and complexity of the model. It's recommended to utilize high-performance servers or cloud services with robust hardware specifications to ensure smooth and efficient processing.
How can businesses measure the accuracy or performance of sentiment analysis models like ChatGPT? Are there any standard evaluation metrics?
Olivia, businesses can measure the accuracy and performance of sentiment analysis models using evaluation metrics like precision, recall, and F1-score. These metrics help assess how well the model predicts positive, negative, and neutral sentiments. The choice of metrics may vary based on the specific use case and requirements.
Mike, what are some potential applications of sentiment analysis enhanced by ChatGPT that we can expect in the future?
Alex, the potential applications are vast. We can expect enhanced sentiment analysis to be used in areas like social media monitoring, brand reputation management, customer feedback analysis, market research, product recommendations, and sentiment-driven chatbots. It will enable businesses to better understand their audience and make data-driven decisions.
How does ChatGPT handle sentiment analysis in multiple languages? Can it perform equally well across different languages?
Good question, Jacob. While ChatGPT can handle sentiment analysis in multiple languages, its performance might vary across different languages. It depends on the availability of training data and how well it covers the specific language's nuances. Language-specific fine-tuning can help improve performance for individual languages.
Do you see any potential ethical concerns when deploying ChatGPT for sentiment analysis in real-world applications?
Ethical concerns can arise, Sarah. Bias in the training data can lead to unfair sentiment analysis results. It's important to address and mitigate biases by carefully designing evaluation strategies, diverse data selection, and algorithmic fairness techniques. Transparency and responsible AI practices should be prioritized to ensure ethical deployment.
How can developers improve the accuracy of sentiment analysis with ChatGPT? Any tips to fine-tune the models for better results?
To improve accuracy, John, developers can fine-tune ChatGPT by using specific datasets relevant to their domain and tasks. By incorporating feedback and annotations from human experts, the model can adapt and learn for more accurate sentiment analysis. Experimenting with different hyperparameters and testing against a validation set can further optimize performance.
How scalable is ChatGPT for sentiment analysis? Can it handle processing large amounts of text in real-time?
ChatGPT's scalability for sentiment analysis depends on the system resources and computational power available. With sufficient resources, it can handle processing large amounts of text in real-time. However, scaling horizontally using multiple instances might be necessary for extremely high volumes or tight response time requirements.
Mike, have you encountered any surprising or interesting findings during your research and experiments with ChatGPT for sentiment analysis?
Indeed, David. It was fascinating to see how ChatGPT could understand and interpret sentiments even in cases where humans might disagree or find it challenging. The ability to capture subtle nuances and context has been impressive, and it opens up exciting possibilities for sentiment analysis in various applications.
Are there any specific industries or sectors where you believe ChatGPT's enhanced sentiment analysis can have a significant impact?
Sophie, ChatGPT's enhanced sentiment analysis can have a significant impact in industries like e-commerce, social media, customer service, public relations, and market research. By accurately understanding sentiments and emotions, businesses can improve customer satisfaction, brand reputation, and make data-driven decisions to stay ahead of the competition.
How do you envision the integration of ChatGPT with other NLP techniques to enhance sentiment analysis even further?
Jessica, integrating ChatGPT with other NLP techniques can unlock new possibilities. Combining it with entity recognition, aspect-based sentiment analysis, or emotion detection can provide a more comprehensive understanding of sentiment-related aspects. By leveraging complementary NLP techniques, we can achieve even higher accuracy and granularity in sentiment analysis.
Could you share some real-world examples where ChatGPT has been successfully used for sentiment analysis?
Certainly, Olivia! ChatGPT has been successfully used for sentiment analysis in social media monitoring, customer feedback analysis for product improvements, brand reputation management, and sentiment-driven chatbots or virtual assistants. Its ability to handle real-world complexities and deliver accurate sentiment analysis has made it a valuable tool in these domains.
Do you have any recommendations for developers who are just starting to explore ChatGPT for sentiment analysis?
Absolutely, Matt! I recommend developers starting by familiarizing themselves with the existing literature on sentiment analysis and NLP techniques. They should experiment with ChatGPT using small datasets and gradually scale up for specific use cases. Actively seek feedback from users to refine and improve the model's performance over time.
I want to thank everyone for participating in this discussion. Your questions and insights have been valuable. If anyone has further inquiries or wants to explore ChatGPT's applications in more detail, feel free to reach out to me. Have a great day!