Enhancing Sentiment Analysis in Social Network Analysis with ChatGPT
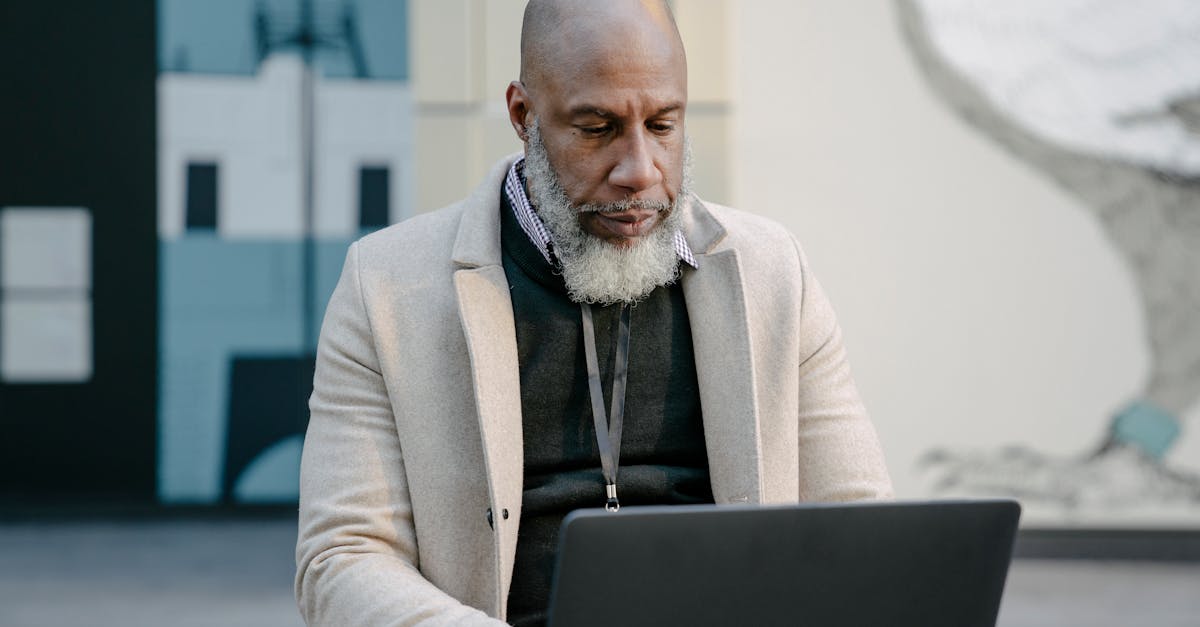
Social Network Analysis is a powerful technology that can be applied in various areas to derive valuable insights from social media data. One such area is Sentiment Analysis, where Social Network Analysis can assess the mood or tone of comments and posts, giving an insight into the general sentiment within the social network.
The Power of Social Network Analysis
Social Network Analysis (SNA) is the process of analyzing the relationships and connections between individuals or entities within a social network. With the proliferation of social media platforms, there is a wealth of user-generated content available for analysis. By leveraging SNA techniques, we can gain a deeper understanding of the social interactions, influence, and sentiment present within these networks.
Application in Sentiment Analysis
Sentiment Analysis involves determining the emotional tone or attitude expressed in a piece of text. Traditionally, sentiment analysis has focused on analyzing individual posts or comments, but Social Network Analysis takes it a step further by considering the relationships and connections between users.
By analyzing the social network, we can capture the sentiment spread across the network, identifying influential users, and discovering sentiment clusters or communities. This information provides a comprehensive view of the overall sentiment within the social network, helping businesses and researchers better understand the mood of their target audience.
Benefits of Social Network Analysis in Sentiment Analysis
1. Identify Influential Users: By analyzing the social connections within the network, SNA can identify influential users who have a significant impact on shaping the sentiment of others. This information can be leveraged for targeted marketing campaigns or engaging influencers in brand promotion.
2. Discover Sentiment Clusters: SNA can uncover communities or groups of users with similar sentiment patterns. This knowledge can be helpful in identifying niche markets, understanding the preferences of specific user groups, or even detecting potential sentiment shifts within the network.
3. Analyze Sentiment Dynamics: By monitoring sentiment patterns over time, SNA can capture changes in sentiment and identify the factors influencing these changes. This allows for a proactive approach in addressing issues or enhancing positive sentiment within the social network.
Challenges and Future Directions
While Social Network Analysis has proven to be a valuable tool in Sentiment Analysis, several challenges exist. These include the sheer volume of data, data quality, privacy concerns, and the need for efficient algorithms to handle large-scale social network data.
As technology and analytical capabilities continue to evolve, there is immense potential for the further development of Social Network Analysis in the field of Sentiment Analysis. Future research and advancements in machine learning, natural language processing, and network analysis techniques will undoubtedly contribute to enhancing the accuracy and applicability of sentiment analysis within social networks.
Conclusion
Social Network Analysis plays a crucial role in Sentiment Analysis, allowing us to assess the mood or tone of comments and posts within a social network. By analyzing the relationships and connections between users, SNA provides a holistic view of the sentiment spread across the network, enabling businesses and researchers to gain valuable insights into the preferences and attitudes of their target audience. As technology continues to advance, Social Network Analysis will undoubtedly play an even more significant role in understanding and leveraging social media data.
Comments:
Thank you all for reading my article on enhancing sentiment analysis with ChatGPT. I'm excited to hear your thoughts and opinions!
Great article, Jeff! I really enjoyed how you explained the challenges of sentiment analysis in social network analysis and how ChatGPT can help overcome them. Looking forward to seeing more advancements in this field!
I agree, Mark. Sentiment analysis is crucial in understanding public opinion, especially on social media platforms. ChatGPT seems like a promising tool to improve accuracy. Jeff, do you have any thoughts on its limitations?
Thanks, Michelle! While ChatGPT has shown impressive results, it still has some limitations. One challenge is handling sarcasm and irony effectively. Another limitation is the potential for bias in the training data. Continual improvement and training can help address these issues.
Jeff, I found your article insightful. Sentiment analysis is vital for businesses to understand customer feedback. I'm curious, though, how would you suggest integrating ChatGPT with existing sentiment analysis tools?
Thanks, Sara! Integrating ChatGPT with existing sentiment analysis tools can be beneficial. One approach could be using ChatGPT's generated responses as additional features for training existing models. This could potentially enhance the accuracy and resolution of sentiment analysis.
I appreciate your article, Jeff! Sentiment analysis is a fascinating area, and I can see the potential of ChatGPT in improving it. With its ability to understand context and generate human-like responses, it could be a game-changer in sentiment analysis. What are your thoughts on privacy concerns when using ChatGPT for sentiment analysis?
Thank you, Emily! Privacy is indeed a concern when working with text data in sentiment analysis. It's important to handle user data responsibly and ensure compliance with privacy regulations. Anonymization, aggregation, and obtaining appropriate consent are important steps in addressing privacy concerns.
Jeff, your article was well-written and informative. I have a question about bias in sentiment analysis. Can ChatGPT be used to identify and mitigate bias in social network analysis?
Thank you, Alex! ChatGPT can be used to help identify and mitigate bias. By analyzing and generating responses, social network analysis researchers can get insights into the potential bias in sentiment analysis algorithms and develop techniques to reduce it. OpenAI encourages researchers to focus on bias mitigation in their work.
Jeff, your article shed light on the importance of sentiment analysis in social network analysis. It's fascinating how ChatGPT can understand the emotional context and respond accordingly. Do you foresee any ethical concerns in the widespread use of ChatGPT in sentiment analysis?
Thank you, Lisa! With any AI technology, there are ethical concerns to consider. Ensuring transparency, avoiding misuse, and being mindful of potential bias or harm are crucial. Ethical guidelines and governance frameworks can guide the responsible use of ChatGPT and other sentiment analysis tools.
Great article, Jeff! I found ChatGPT's potential in sentiment analysis quite fascinating. Are there any plans to expand its capabilities to languages other than English?
Thanks, Robert! OpenAI has plans to expand ChatGPT's capabilities to support more languages. While currently focused on English, there is ongoing research and development to make it accessible to a wider range of languages, enabling broader cross-cultural sentiment analysis.
Jeff, I enjoyed reading your article. Sentiment analysis is crucial for many applications, and ChatGPT seems promising. However, do you think it can accurately handle short and informal social media text, which often includes slang and emojis?
Thank you, Daniel! Short and informal social media text can pose a challenge, particularly with slang and emojis. While ChatGPT can understand and generate responses in such contexts, it can still have limitations in accurately capturing the sentiment. Fine-tuning and more training on social media data would be important to address this issue.
Great article, Jeff! Sentiment analysis is important, and ChatGPT seems like a powerful tool to enhance it. How would you recommend training ChatGPT to improve its sentiment analysis capabilities?
Thanks, Ryan! Training ChatGPT to improve its sentiment analysis capabilities would involve using labeled sentiment analysis datasets for fine-tuning. Incorporating a wide range of publicly available text data and balancing positive and negative examples can help it better understand sentiments and improve accuracy.
Jeff, I found your article very informative. Sentiment analysis is an interesting field, and ChatGPT's potential is exciting. What could be the main challenges in implementing ChatGPT for sentiment analysis in large-scale social networks?
Thank you, Sophia! Implementing ChatGPT for sentiment analysis in large-scale social networks could face challenges in terms of computational resources, scalability, and real-time analysis. Optimizing the processing and parallelization of ChatGPT's architecture can help address these challenges and make it viable for large-scale analysis.
Jeff, your article was a great read. Sentiment analysis is crucial in many domains, and ChatGPT seems like a useful tool. What are the potential applications of ChatGPT-enhanced sentiment analysis beyond social networks?
Thanks, Tom! ChatGPT-enhanced sentiment analysis can have applications in various domains. Market research, brand monitoring, customer feedback analysis, and public opinion measurement are just a few examples where understanding sentiment at scale can provide valuable insights.
Jeff, your article provided a good overview of sentiment analysis and ChatGPT's potential. In terms of accuracy, how does ChatGPT compare to traditional sentiment analysis methods?
Thank you, Amanda! ChatGPT has shown promising results in sentiment analysis, but its accuracy may vary compared to traditional methods. While traditional methods are often purpose-built for sentiment analysis, ChatGPT's generative nature allows it to understand context better, but it may have limitations with certain nuances. A hybrid approach that combines the strengths of both methods could be effective.
Jeff, your article highlighted the potential of ChatGPT in sentiment analysis. How would you suggest addressing the challenges of dealing with the vast amount of noisy and biased data present in social media for sentiment analysis?
Thanks, Sam! Dealing with noisy and biased data is indeed a challenge in sentiment analysis. It's important to preprocess the data, remove duplicates, and handle outliers. Additionally, using techniques like data augmentation, active learning, and leveraging external resources can help in more robust sentiment analysis despite noisy and biased social media data.
I enjoyed reading your article, Jeff! Sentiment analysis plays a crucial role in media monitoring. With ChatGPT's capabilities, it can provide valuable insights into the public's perception and sentiment towards different topics. Are there any specific industries that can greatly benefit from this technology?
Thank you, Olivia! ChatGPT-enhanced sentiment analysis can benefit various industries. Advertising and marketing, e-commerce, public relations, political campaigns, and media broadcasting are some industries where understanding sentiment towards products, services, or topics can drive informed decision-making and improve communication strategies.
Jeff, great article! Sentiment analysis is an exciting area, and ChatGPT's potential is intriguing. Can ChatGPT also be trained to analyze sentiment based on specific domains or topics?
Thanks, Joshua! ChatGPT can indeed be trained for sentiment analysis based on specific domains or topics. By fine-tuning the model on targeted datasets related to the desired domain, it can learn domain-specific sentiment nuances and provide more accurate results.
Jeff, I found your article on sentiment analysis quite interesting. ChatGPT's capabilities offer exciting possibilities. How do you see sentiment analysis evolving in the future, and what role will AI technologies like ChatGPT play in it?
Thank you, Sophie! Sentiment analysis will continue to evolve with advancements in AI technologies. AI models like ChatGPT will play a significant role in improving the accuracy and efficiency of sentiment analysis. With ongoing research, fine-tuning, and advancements in language understanding, we can expect sentiment analysis to provide even more valuable insights in the future.
Jeff, great article! Sentiment analysis is crucial in understanding public opinion. ChatGPT's potential to enhance it is fascinating. Have you come across any real-world use cases where ChatGPT has been successfully employed in sentiment analysis?
Thanks, Benjamin! ChatGPT is still relatively new, but there are real-world use cases where it has shown promise in sentiment analysis. Some early applications include analyzing user sentiment in online reviews, analyzing social media sentiment during product launches, and monitoring sentiment towards political candidates during election campaigns.
Jeff, I enjoyed your article on enhancing sentiment analysis with ChatGPT. Sentiment analysis is fascinating, and the potential to improve it with models like ChatGPT is exciting. Are there any plans to release a more fine-tuned version of ChatGPT specifically for sentiment analysis?
Thank you, Liam! While I can't speak for OpenAI's specific plans, it's possible that in the future, OpenAI or researchers may release more fine-tuned versions of ChatGPT specifically designed for sentiment analysis tasks. Continual research and development can lead to more specialized models that excel at sentiment analysis.
Jeff, your article provided valuable insights into enhancing sentiment analysis. ChatGPT's potential in this context is exciting. What are the key factors to consider when evaluating the performance of ChatGPT for sentiment analysis?
Thank you, Grace! When evaluating ChatGPT's performance for sentiment analysis, key factors to consider include accuracy, precision, recall, and F1 score. It's also important to assess how well it handles sentiment polarity and if it captures the intended sentiment accurately in a variety of contexts and topics.
Jeff, great article! Sentiment analysis is vital, and ChatGPT's potential in improving it is exciting. What are some open challenges that researchers still need to address in sentiment analysis?
Thanks, Ethan! Researchers still face open challenges in sentiment analysis, including handling subjectivity, analyzing sentiment in multilingual settings, addressing cultural differences, dealing with facet-level sentiment, and improving the generalization across domains. These challenges represent opportunities for further research and innovation.
Jeff, I found your article quite insightful. Sentiment analysis is a fascinating field, and ChatGPT's capabilities seem promising. How do you see sentiment analysis contributing to social media moderation?
Thank you, Lucy! Sentiment analysis can play a crucial role in social media moderation. By automatically classifying sentiment, it can help identify and flag potentially offensive or harmful content, enabling more efficient content moderation and community management. It can be an invaluable tool for maintaining positive and healthy online spaces.
Jeff, excellent article on sentiment analysis and ChatGPT's potential to enhance it. Can ChatGPT be used to analyze sentiment in real-time social media feeds, given the dynamic nature of platforms like Twitter?
Thanks, Emma! ChatGPT can be used for sentiment analysis in real-time social media feeds. While the dynamic nature of platforms like Twitter poses challenges, efficient data processing and stream processing techniques can enable near real-time sentiment analysis using ChatGPT to capture trends and understand public sentiment as it evolves.
Jeff, your article on sentiment analysis with ChatGPT was informative. Sentiment analysis can provide valuable insights. How do you envision ChatGPT's role in sentiment analysis evolving over time?
Thank you, Max! ChatGPT's role in sentiment analysis will likely evolve with continued research and advancements. We can expect it to become more accurate, versatile, and scalable, enabling comprehensive sentiment analysis across various domains, languages, and social media platforms. The ongoing collaboration between researchers and developers will be vital in driving these improvements.
Jeff, your article on enhancing sentiment analysis with ChatGPT was thought-provoking. ChatGPT's capabilities offer exciting possibilities. How can one fine-tune ChatGPT for specific sentiment analysis tasks?
Thanks, Sophie! Fine-tuning ChatGPT for sentiment analysis involves training the model on a dataset labeled with sentiment annotations. Researchers can use text classification datasets, create their own labeled data, or leverage existing sentiment analysis benchmarks. The fine-tuning process tunes ChatGPT's parameters specifically for sentiment analysis and enables better sentiment understanding and generation.
Jeff, your article provided valuable insights into sentiment analysis and ChatGPT's potential in enhancing it. What are some additional applications of ChatGPT or similar models that you foresee in the field of natural language processing?
Thank you, Nathan! Besides sentiment analysis, ChatGPT and similar models have potential applications in several areas of natural language processing. These include dialogue systems, content generation, language translation, text summarization, and more. The advancements in AI models like ChatGPT can significantly impact the way we interact with and process textual data across various domains.
Jeff, your article on sentiment analysis and ChatGPT was insightful. Sentiment analysis is crucial for businesses and decision-making. Do you have any recommendations for organizations looking to adopt ChatGPT for sentiment analysis?
Thank you, Laura! For organizations considering adopting ChatGPT for sentiment analysis, it's important to start with a clear understanding of their specific requirements and the limitations of the model. Collaborating with AI experts, using fine-tuning techniques, constantly evaluating performance, and considering user feedback can help optimize the integration and maximize the benefits of ChatGPT in sentiment analysis.
Jeff, your article shed light on the potential of ChatGPT in sentiment analysis. Sentiment analysis has wide applications, and incorporating AI models like ChatGPT can greatly enhance it. Are there any efforts underway to make ChatGPT more accessible to non-technical users?
Thanks, Sophia! Making ChatGPT more accessible to non-technical users is a priority. Efforts are underway to develop user-friendly interfaces, intuitive APIs, and provide documentation and tutorials that can enable users with varying technical backgrounds to leverage ChatGPT's capabilities in sentiment analysis and other NLP tasks.
Jeff, your article was well-written and informative. Sentiment analysis has numerous potential applications, and ChatGPT's capabilities can enhance its accuracy. How can one deploy ChatGPT for sentiment analysis in a production environment?
Thank you, Lucas! Deploying ChatGPT for sentiment analysis in a production environment involves setting up a backend infrastructure to handle API requests, managing model versions, and ensuring scalability and availability. It also requires monitoring and maintaining the model's performance and incorporating user feedback to iterate and improve the sentiment analysis capabilities.
Jeff, I found your article on sentiment analysis quite interesting. ChatGPT's enhancements hold promise. What are some limitations of traditional sentiment analysis methods that ChatGPT can potentially overcome?
Thank you, Aaron! Traditional sentiment analysis methods often struggle with understanding context, handling sarcasm, and capturing nuanced sentiments. ChatGPT's generative nature and language understanding capabilities allow it to better comprehend the meaning behind text and provide contextually appropriate responses, enabling more accurate sentiment analysis.
Jeff, great article on sentiment analysis and ChatGPT. Sentiment analysis has far-reaching implications. Are there any plans to incorporate reinforcement learning techniques to improve ChatGPT's sentiment analysis capabilities?
Thanks, Julia! Reinforcement learning techniques can certainly be valuable for improving sentiment analysis capabilities. While I don't have insights into specific plans, it's likely that reinforcement learning and advanced techniques will be explored to further enhance ChatGPT's sentiment analysis performance, enabling more accurate and context-aware sentiment understanding.
Jeff, I enjoyed your article on sentiment analysis with ChatGPT. Sentiment analysis is a fascinating topic, and ChatGPT's potential to enhance it is exciting. How do you see ChatGPT's role in sentiment analysis evolving in the context of emerging social media platforms?
Thank you, Adam! As new social media platforms emerge, ChatGPT can play a crucial role in sentiment analysis. With customization and training on platform-specific data, ChatGPT can adapt to the evolving patterns, themes, and sentiment analysis requirements of these platforms. It can provide valuable insights into users' sentiment and contribute to a better understanding of emerging social media trends.
Jeff, your article on enhancing sentiment analysis with ChatGPT was informative. Sentiment analysis is vital, especially for businesses. How effective is ChatGPT in capturing sentiment from different demographic groups with diverse cultural backgrounds?
Thank you, Michael! ChatGPT's effectiveness in capturing sentiment from different demographic groups with diverse cultural backgrounds relies on the diversity and representativeness of the training data. By including diverse datasets during fine-tuning, researchers can help improve the model's ability to understand sentiments across demographic groups, thus enhancing its effectiveness in capturing varied cultural sentiment.
Jeff, your article provided valuable insights. Sentiment analysis is a critical aspect of social network analysis, and ChatGPT seems to offer useful capabilities. How can one verify the accuracy and reliability of sentiment analysis results obtained using ChatGPT?
Thank you, Sophie! Verifying the accuracy and reliability of sentiment analysis results obtained using ChatGPT involves comparing the model's predictions against annotated ground truth data, setting up evaluation metrics, and performing extensive testing on diverse datasets. Conducting human evaluations with domain experts can help validate the sentiment analysis quality and ensure its reliability for real-world applications.
Jeff, your article was an interesting read. ChatGPT's potential in sentiment analysis is intriguing. Do you think ChatGPT could also be employed in sentiment analysis for languages with complex grammatical structures, such as Mandarin or Arabic?
Thanks, Jonathan! ChatGPT's language understanding capabilities can be adapted to sentiment analysis tasks for languages with complex grammatical structures. However, it would require extensive training and fine-tuning on large-scale datasets of the specific language to ensure accurate sentiment analysis in contexts with different sentence structures and nuances.
Jeff, great article on ChatGPT and sentiment analysis. Sentiment analysis is valuable in various domains. How can one address the concern of biased sentiment analysis results influenced by the biases in training datasets?
Thank you, Lily! Addressing biases in sentiment analysis results is crucial. It starts with carefully curating and diversifying training datasets to minimize biases. Additionally, employing bias mitigation techniques, conducting thorough post-training analysis, and involving diverse evaluators can help identify and mitigate potential bias in sentiment analysis results obtained using ChatGPT or other models.
Jeff, your article on sentiment analysis with ChatGPT was insightful. Sentiment analysis has wide-ranging applications. How can ChatGPT's sentiment analysis capabilities be fine-tuned to specific industry domains and jargon?
Thanks, George! Fine-tuning ChatGPT for specific industry domains involves training on labeled datasets that reflect the industry-specific sentiment and jargon. By leveraging industry-specific language and incorporating relevant domain knowledge during fine-tuning, ChatGPT can capture nuanced sentiments and provide more accurate sentiment analysis tailored to specific industry requirements.
Jeff, your article was informative. Sentiment analysis plays a crucial role in understanding public opinion. How scalable is ChatGPT's sentiment analysis in handling large volumes of textual data?
Thank you, Emma! ChatGPT's sentiment analysis scalability depends on the computational resources available. Efficient implementation, parallelization techniques, and optimization can help scale sentiment analysis to handle large volumes of textual data and achieve real-time or near real-time performance in analyzing sentiments from social media feeds or other sources with high data throughput.
Jeff, I found your article quite insightful. Sentiment analysis is crucial in understanding public sentiment. Can ChatGPT be used in sentiment analysis of audiovisual content, such as analyzing sentiments expressed in videos or podcasts?
Thanks, Evelyn! ChatGPT's capabilities can be extended to analyze sentiments expressed in audiovisual content. By converting speech or transcriptions to text, ChatGPT can process the textual component and provide sentiment analysis insights for audiovisual media, helping understand sentiments expressed in videos, podcasts, or other multimedia formats.
Jeff, your article shed light on sentiment analysis and ChatGPT's potential. Sentiment analysis is crucial for understanding user behavior. How important is user feedback in improving the performance of ChatGPT for sentiment analysis?
Thank you, David! User feedback is invaluable in improving ChatGPT's sentiment analysis performance. Incorporating user concerns, suggestions, and real-world use cases provides insights for ongoing research and development. OpenAI actively seeks user feedback to iterate on models like ChatGPT, enhancing their effectiveness and addressing their limitations.
Jeff, your article on sentiment analysis was well-explained. ChatGPT's potential in enhancing it is exciting. How would you recommend organizations prepare their data for sentiment analysis using ChatGPT?
Thanks, Amy! Organizations can prepare their data for sentiment analysis using ChatGPT by annotating it with sentiment labels, creating training and testing datasets, and ensuring a diverse representation of sentiments within the data. Cleaning the data, removing noise, handling missing values, and preprocessing text for analysis can further enhance the quality of sentiment analysis using ChatGPT.
Jeff, your article on enhancing sentiment analysis with ChatGPT was informative. Sentiment analysis is critical in understanding user sentiment. What factors influence ChatGPT's sentiment analysis accuracy when applied to social network data?
Thank you, Eric! Several factors influence ChatGPT's sentiment analysis accuracy when applied to social network data. The diversity, representativeness, and quality of the training data, fine-tuning techniques, data preprocessing steps, and the model's understanding of context and language nuances are some factors that can impact the sentiment analysis accuracy in social network data applications.
Jeff, great article on sentiment analysis and ChatGPT. Sentiment analysis is crucial in various fields. How can one effectively validate the performance of ChatGPT for sentiment analysis across different use cases?
Thanks, Hannah! Validating the performance of ChatGPT for sentiment analysis across different use cases involves setting up specific evaluation metrics, comparing against known ground truth sentiment labels, and conducting extensive testing on representative datasets from different domains and contexts. It's important to evaluate performance across a range of use cases to ensure the reliability and accuracy of ChatGPT's sentiment analysis capabilities.
Jeff, your article offered valuable insights. Sentiment analysis has numerous applications, and ChatGPT's capabilities can significantly enhance it. Are there any efforts underway to address the challenges of domain adaptation in sentiment analysis with ChatGPT?
Thank you, Mike! Domain adaptation is an important challenge in sentiment analysis with ChatGPT. While I don't have specific insights into ongoing efforts, researchers are actively exploring techniques like unsupervised domain adaptation, transfer learning, and domain-specific fine-tuning to address this challenge and improve ChatGPT's adaptability to different sentiment analysis domains.
Jeff, your article on sentiment analysis and ChatGPT was fascinating. Sentiment analysis has wide applications. How can one leverage ChatGPT to analyze sentiment in real-time streams, such as live social media updates or news feeds?
Thanks, Sophie! Leveraging ChatGPT for sentiment analysis in real-time streams involves setting up a stream processing pipeline to receive and process the updates, connecting it with ChatGPT's sentiment analysis capabilities, and continuously analyzing and categorizing sentiment from the incoming stream. This way, sentiment insights can be obtained in near real-time from live social media updates or news feeds.
Jeff, your article was insightful. Sentiment analysis is crucial for businesses and decision-making. How can one handle the evolving lexical and contextual changes in sentiment expression when using ChatGPT for sentiment analysis?
Thank you, Kevin! Handling evolving lexical and contextual changes in sentiment expression involves continually updating the training data, incorporating recent linguistic trends and expressions, and fine-tuning ChatGPT with sentiment annotations from more current datasets. By keeping the model up-to-date with the language shifts and evolving sentiment expressions, it can provide accurate sentiment analysis across lexical and contextual changes.
Jeff, your article on enhancing sentiment analysis with ChatGPT was thought-provoking. Sentiment analysis has numerous applications. Can ChatGPT be used to analyze sentiment in offline text sources, such as historical documents or books?
Thanks, Claire! ChatGPT can indeed be used to analyze sentiment in offline text sources like historical documents or books. By providing the textual content as input, ChatGPT can process the data and generate sentiment analysis insights, helping understand sentiment expressed in the text and its historical context.
Jeff, great article on sentiment analysis with ChatGPT. Sentiment analysis is vital for understanding public opinions and sentiments. How can ChatGPT be fine-tuned to improve its recognition of various sentiment intensities, such as identifying subtle or moderate sentiments?
Thank you, Liam! Fine-tuning ChatGPT to improve its recognition of various sentiment intensities involves using datasets that provide nuanced sentiment annotations, ensuring a balanced representation of subtle, moderate, and strong sentiments. By training the model with such data, it can learn to capture the varying intensities and provide more nuanced sentiment analysis.
Jeff, your article provided valuable insights into sentiment analysis and ChatGPT's potential in enhancing it. Across different platforms and industries, what are the key challenges organizations may face when using ChatGPT for sentiment analysis?
Thanks, Andrew! Key challenges organizations may face when using ChatGPT for sentiment analysis include handling biased or noisy training data, ensuring privacy and compliant data usage, addressing cultural sensitivities, handling evolving language trends, dealing with real-time analysis requirements, and managing computational resources for large-scale sentiment analysis. Overcoming these challenges will be crucial for successful sentiment analysis deployments.
Jeff, your article was insightful. Sentiment analysis can contribute to various domains. How can one integrate domain-specific knowledge or ontologies with ChatGPT to improve its sentiment analysis for specialized industries?
Thank you, Joshua! Integrating domain-specific knowledge or ontologies with ChatGPT involves incorporating industry-specific terminologies, sentiment lexicons, or domain knowledge during fine-tuning. By training the model with a combination of sentiment-related datasets and domain-specific resources, it can learn to recognize sentiment nuances specific to specialized industries, improving the sentiment analysis for those domains.
Jeff, your article shed light on sentiment analysis and ChatGPT's capabilities. Sentiment analysis is important for understanding public perception. What are some potential ways to make ChatGPT's sentiment analysis more interpretable and explainable?
Thanks, Julia! Making ChatGPT's sentiment analysis more interpretable and explainable can involve techniques like attention mechanisms, visualization of sentiment cues, or generating explanations alongside sentiment predictions. By providing insights into which parts of the input text influenced the sentiment analysis output, it becomes easier to understand and evaluate the reasoning behind ChatGPT's sentiment analysis decisions.