Enhancing Sentiment Analysis in Technology with Gemini
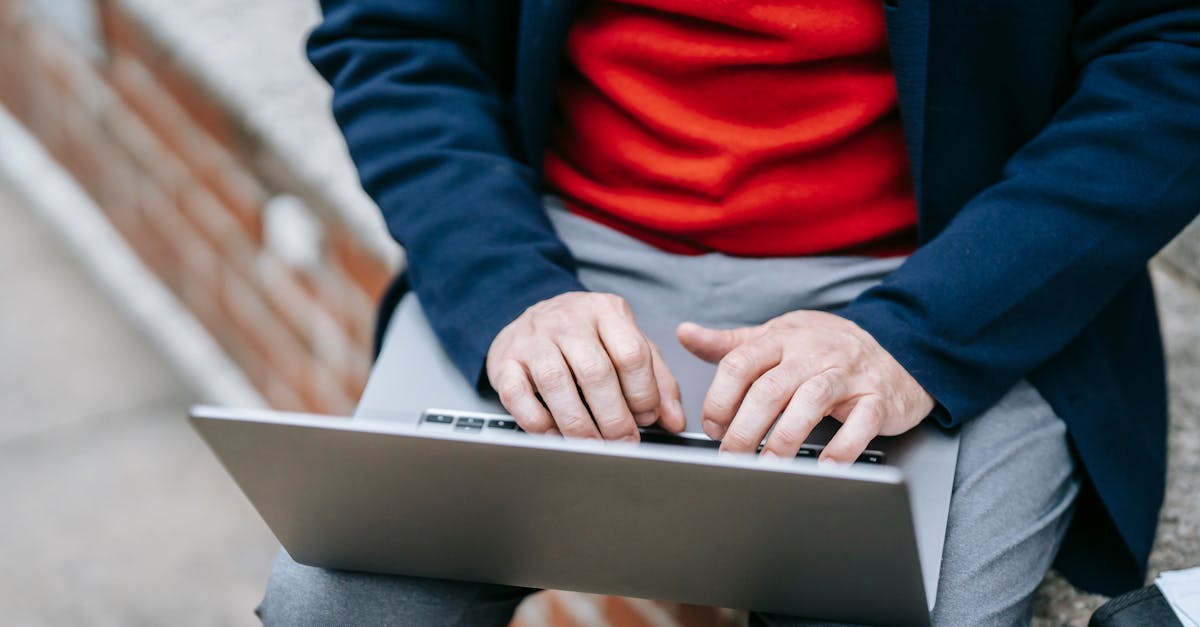
Sentiment analysis, the process of determining the emotional tone behind a series of words, plays a crucial role in understanding public opinion and customer feedback. With the exponential growth of digital platforms and the increasing reliance on online communication, sentiment analysis has become a critical component for businesses and organizations in various industries. In the field of technology, sentiment analysis holds immense significance as it enables companies to gauge the user response towards their products and services.
Traditionally, sentiment analysis was performed using rule-based and statistical techniques that often fell short in accurately capturing the intricacies of human emotions and nuances in language. However, recent advancements in artificial intelligence (AI) and natural language processing (NLP) have revolutionized sentiment analysis, paving the way for more accurate and sophisticated approaches.
The Emergence of Gemini
One of the most notable advancements in AI that has significantly enhanced sentiment analysis in technology is the development of Gemini. Gemini, based on the LLM architecture, is a conversational AI model that has been trained on a large corpus of internet text, making it capable of understanding and generating human-like responses.
Gemini's ability to comprehend context, complex language structures, and coherent conversations makes it a powerful tool for analyzing sentiment in technology. Its machine learning capabilities allow it to learn from vast amounts of textual data, enabling it to accurately discern sentiment and understand the sentiment-laden phrases specific to the technology domain.
Utilizing Gemini for Sentiment Analysis
By harnessing the power of Gemini, sentiment analysis in technology can be taken to new heights. Companies can use Gemini to analyze customer reviews, social media posts, and other user-generated content to gain valuable insights into public perception and sentiment towards their products and services.
Gemini's advanced language understanding capabilities help it identify not just positive or negative sentiments, but also detect the intensity of emotions expressed in the text. This level of granularity allows companies to understand not only whether users are happy or dissatisfied but also the reasons behind their emotions.
Additionally, Gemini can be integrated with chatbots and virtual assistants to provide real-time sentiment analysis during customer interactions. By analyzing user queries, Gemini can gauge the sentiment of customers and provide personalized responses based on their emotional state, improving customer satisfaction and engagement.
Challenges and Ethical Considerations
While Gemini offers tremendous potential for enhancing sentiment analysis in technology, there are certain challenges and ethical considerations that need to be addressed. The potential for biases and unfair treatment must be carefully monitored and controlled to ensure that the sentiment analysis provided by Gemini is fair and unbiased.
Moreover, the privacy of individuals should be respected, and appropriate measures should be in place to handle sensitive user data. Transparency in how sentiment analysis is performed using Gemini is also crucial to maintain the trust of users.
In Conclusion
Gemini represents a significant breakthrough in sentiment analysis in the field of technology. Its advanced language understanding capabilities, combined with its conversational AI functionalities, provide a powerful tool for accurately gauging public sentiment and understanding the emotional responses of users. While leveraging Gemini for sentiment analysis, it is important for organizations to address ethical considerations and ensure the fair and responsible use of this technology. With the continuous advancements in AI, sentiment analysis in technology is set to evolve further, allowing businesses to make informed decisions based on user sentiment and feedback.
Comments:
Thank you all for your interest in my article on enhancing sentiment analysis with Gemini! I'm excited to hear your thoughts and answer any questions you may have.
Great article, Ujjwal! I find sentiment analysis fascinating, and Gemini seems like a promising addition. Do you think it can accurately capture emotional nuances in text?
Thanks, Sarah! Gemini has shown good results in sentiment analysis, but like any tool, it has limitations. While it can capture emotions to a certain extent, it's important to train it on diverse data for better accuracy.
I'm curious about potential biases in sentiment analysis. Can Gemini handle them effectively?
Valid concern, Alex! Bias is a significant challenge in sentiment analysis. Gemini can adopt techniques like debiasing of training data and fine-tuning to mitigate biases. However, continuous monitoring and improvement are essential to minimize such biases.
I've seen sentiment analysis tools struggle with sarcasm and context. Can Gemini overcome these difficulties?
Good point, Emily! Sarcasm and contextual understanding can be challenging for sentiment analysis. Gemini has improved in this regard but may still have limitations. Incorporating contextual clues and fine-tuning with sarcasm-rich datasets can help to some extent.
What are the potential applications of enhancing sentiment analysis? Can it benefit businesses in any specific ways?
Thank you for your question, Mark! Enhancing sentiment analysis can have numerous applications. It can help businesses analyze customer feedback, assess brand reputation, understand market trends, and even identify potential crises. It enables them to make data-driven decisions and improve customer satisfaction.
Do you think Gemini can be beneficial for sentiment analysis in social media monitoring?
Absolutely, Jennifer! Social media monitoring relies heavily on sentiment analysis to understand public opinion, identify trends, and monitor brand sentiment. Gemini can assist in this domain by providing more accurate and nuanced sentiment analysis.
How does Gemini compare to other sentiment analysis tools available?
Good question, Michael! Gemini has shown promising results, especially with its conversational capabilities. However, it's not a one-size-fits-all solution. Its performance can vary based on the data it was trained on and specific use cases. It's always advisable to evaluate different tools and choose the one that best fits the requirements.
Ujjwal, have you come across any challenges in implementing Gemini for sentiment analysis?
Yes, Sarah! One of the main challenges is handling ambiguous or vague text. Sentiment analysis requires clear context to provide accurate results, but when text lacks clarity, it becomes more challenging. Additionally, managing and addressing bias is a significant challenge that needs constant attention.
Are there any privacy concerns related to using Gemini for sentiment analysis?
Privacy concerns are essential, David. Gemini processes user inputs, but as an AI model, it doesn't retain any information beyond the interaction. However, it's crucial to handle user data responsibly and follow appropriate privacy guidelines when integrating Gemini into sentiment analysis workflows.
Ujjwal, in your experience, have you witnessed any interesting real-life applications of Gemini for sentiment analysis?
Absolutely, Emily! One interesting application is using Gemini for analyzing customer feedback in the hospitality industry. It allows hotels and resorts to understand guests' sentiments, identify areas of improvement, and take proactive measures to enhance guest experiences.
How can Gemini be used effectively in sentiment analysis? Any tips or best practices?
Great question, Tom! To use Gemini effectively, it's crucial to fine-tune the model on relevant sentiment analysis datasets. It helps the model align better with the desired outcomes. Additionally, ongoing monitoring and evaluation, along with iterating on the training process, can optimize the results.
What are the limitations of using Gemini for sentiment analysis?
Good question, Emma! Gemini might struggle with rare or domain-specific language, leading to reduced accuracy. Additionally, it can be sensitive to noisy or misleading inputs, affecting the sentiment analysis results. A diversified training dataset and continuous improvement efforts can help mitigate such limitations.
Ujjwal, how do you envision the future of sentiment analysis with the help of AI models like Gemini?
Great question, Alex! AI models like Gemini can enable more accurate and nuanced sentiment analysis, helping businesses and organizations gain deeper insights into public opinion, customer feedback, and market trends. Continued research and improvement in this field holds the potential for significant advancements.
Ujjwal, do you recommend using Gemini as a standalone tool or integrating it with existing sentiment analysis systems?
Good question, Sarah! It largely depends on the specific requirements and capabilities of the existing sentiment analysis systems. Gemini can be used both ways, either as a standalone tool or to enhance the existing systems. Assessing individual needs and conducting experiments can help determine the ideal approach.
What future developments do you anticipate in sentiment analysis that can further enhance its capabilities?
Interesting question, Michael! I believe advancements in natural language processing, incorporating more contextual understanding, and addressing biases will continue to enhance sentiment analysis. Additionally, the integration of multimodal inputs like images and videos can provide a more comprehensive sentiment analysis experience.
Ujjwal, how can businesses overcome the challenge of false positives or false negatives in sentiment analysis results?
Excellent question, Emily! False positives and negatives can be tackled by fine-tuning the sentiment analysis models, leveraging more labeled data, and setting appropriate thresholds based on specific use cases. Continuous monitoring and iterating on the analysis process play a crucial role in improving the accuracy and reducing false results.
Ujjwal, what are some potential challenges while deploying Gemini for sentiment analysis in real-world scenarios?
Thanks for asking, Jennifer! Some challenges in deploying Gemini for sentiment analysis include system integration, handling large volumes of data efficiently, real-time response requirements, and addressing scalability. Deployment considerations should also include data privacy, security, and ongoing model maintenance.
Does the amount of training data significantly affect the performance of Gemini in sentiment analysis?
Yes, David, the quantity and quality of training data play a vital role in Gemini's performance. Sufficient training data helps the model to generalize better and improve accuracy. However, it's important to ensure the training data represents the desired domain and sentiment variations to achieve the desired results.
How can Gemini assist in sentiment analysis for non-English languages?
Great question, Sophia! Gemini can be fine-tuned on non-English sentiments analysis datasets to support sentiment analysis in multiple languages. While it might require language-specific training, fine-tuning can leverage Gemini's underlying capabilities to provide accurate sentiment analysis results for non-English texts.
Ujjwal, how does Gemini handle ambiguous sentiment expressions or cases where sentiment is mixed?
Good question, Sarah! Gemini can face challenges with ambiguous sentiment expressions or mixed sentiment cases. Ideally, training it on diverse and well-labeled data that covers such scenarios can improve its confidence in dealing with mixed or ambiguous sentiment expressions.
Can Gemini be adapted to work with industry-specific sentiment analysis, like healthcare or finance?
Absolutely, Mark! Gemini's flexibility allows it to be adapted to industry-specific sentiment analysis tasks. With sufficient domain-specific training data, fine-tuning the model can help it understand the specific nuances and expressions relevant to healthcare, finance, or any other industry.
Ujjwal, how can Gemini handle sentiment analysis in short texts, like social media posts or product reviews?
Great question, Emma! Short texts present challenges due to limited context. However, Gemini can still provide sentiment analysis in such cases. Depending on the use case, it's beneficial to fine-tune the model with short-text specific datasets, ensuring it can capture sentiment effectively despite the constrained context.
Ujjwal, do you see any ethical implications associated with the widespread use of AI models like Gemini in sentiment analysis?
Ethical implications are a crucial consideration, Michael. AI models, including Gemini, require responsible use to mitigate any biases, protect privacy, and avoid harmful consequences. Transparent integration, explaining limitations, and addressing biases are steps toward ethical deployment of such models in sentiment analysis and AI in general.
Ujjwal, how can businesses ensure data privacy and security when using Gemini for sentiment analysis?
Data privacy and security should be a top priority, Emily. Businesses can ensure privacy by anonymizing or encrypting sensitive information before feeding it to Gemini. Implementing secure data storage, access controls, and following relevant privacy regulations can help maintain data privacy and security while using Gemini for sentiment analysis.
Ujjwal, what resources or tools do you recommend for training and fine-tuning sentiment analysis models like Gemini?
There are various resources available, Tom. Some popular ones include publicly available sentiment analysis datasets like IMDb, Amazon Reviews, and Twitter datasets. Additionally, tools like Hugging Face's Transformers library and industry-specific sentiment analysis datasets can also be valuable resources for training and fine-tuning models like Gemini.
Ujjwal, what can we expect from future iterations of Gemini in the context of sentiment analysis?
The future iterations of Gemini are expected to have improved sentiment analysis capabilities, thanks to ongoing research and advancements in NLP. We can anticipate better contextual understanding, reduced bias, and enhanced domain-specific sentiment analysis support. Continuous iteration and feedback from users will be vital for refining and evolving the model.
Thank you all for taking the time to read my article on Enhancing Sentiment Analysis with Gemini. I hope you found it informative and interesting!
Great article, Ujjwal! Sentiment analysis is crucial in understanding the emotions and opinions of users, and Gemini seems like a promising tool to improve it.
I agree, Alice. Incorporating natural language processing and machine learning techniques into sentiment analysis can lead to more accurate results. Do you have any examples of how Gemini can enhance sentiment analysis?
Absolutely, Bob! Gemini can help in capturing nuanced sentiments by understanding context and generating more human-like responses. It can also handle conversational data, allowing for a deeper analysis of user sentiment over a conversation.
I think incorporating Gemini into sentiment analysis tools could make them more adaptable to different industries. Sentiments can be industry-specific, so having a tool that can understand and adapt to different domains would be beneficial.
That's an excellent point, Eva. Gemini's ability to adapt to different domains and understand industry-specific sentiments can indeed be a game-changer for sentiment analysis in various applications.
I'm curious about the limitations of using Gemini for sentiment analysis. Are there any potential challenges or drawbacks to consider?
Good question, Dan. Gemini, like any AI model, can sometimes generate responses that may be plausible but incorrect. It is important to carefully evaluate the sentiment analysis results and consider potential biases in the training data.
I wonder if integrating Gemini with sentiment analysis tools could help detect and mitigate online harassment or hate speech. Emotion detection can be crucial in identifying harmful content.
Absolutely, Grace. Combining Gemini with sentiment analysis can enhance the identification of harmful content and enable more effective moderation and intervention in online platforms.
Do you think Gemini's performance in sentiment analysis could improve over time as it is exposed to more conversational data?
Indeed, Carlos. As Gemini is exposed to more data and continuously refined, its performance in sentiment analysis will likely improve. This is one of the great advantages of utilizing machine learning models.
I wonder if there are any privacy concerns when using Gemini for sentiment analysis. Will user data be stored or used for other purposes?
Valid concern, Sophia. It's important to ensure the necessary privacy measures are in place if user data is involved. Organizations must be transparent about data usage and comply with privacy regulations to maintain user trust.
I think the integration of Gemini with sentiment analysis tools could greatly benefit customer satisfaction analysis. Companies can gain valuable insights into their customers' sentiments and improve their products and services accordingly.
Absolutely, Liam. Understanding customer sentiment can help businesses drive improvements, make better-informed decisions, and ultimately enhance customer satisfaction and loyalty.
What about multilingual sentiment analysis? Can Gemini handle sentiment analysis for different languages?
Good question, Emily. While Gemini's initial release primarily focused on English, it has the potential to handle sentiments in multiple languages. However, further research and development are required to make it more proficient in languages other than English.
Gemini sounds like a powerful tool for sentiment analysis. Are there any specific industries or sectors where it has shown particularly promising results?
Indeed, Jack. Gemini's potential spans across various sectors, including customer service, brand monitoring, social media analysis, and market research. Its adaptability makes it a versatile tool for sentiment analysis in multiple industries.
I'm excited about the potential of Gemini in sentiment analysis, but I'm concerned about the ethical implications. How can we ensure its usage doesn't result in unintended consequences?
Valid concern, Olivia. Ethical considerations are crucial. Organizations should implement responsible AI practices, conduct regular audits, and have human oversight to prevent unintended consequences or biases in sentiment analysis outcomes.
I'm curious about the training data used for Gemini's sentiment analysis capabilities. How diverse and representative is the training set?
Great question, Isabella. The training data for Gemini's sentiment analysis covers a wide range of sources to ensure diversity. However, it's important to continually evaluate and improve the models to avoid biases and improve representation.
Gemini's ability to understand and generate human-like responses is impressive. How does it handle sarcasm or more complex sentiments?
Indeed, William. While Gemini has shown promising results in capturing complex sentiments, including sarcasm, it may still have limitations in fully understanding nuances. Ongoing research aims to improve its performance in handling such complexities.
Can Gemini be integrated into existing sentiment analysis tools, or does it require a separate implementation?
Good question, Ava. Gemini can be integrated into existing sentiment analysis tools, enhancing their capabilities. Its flexibility allows for integration into different systems and workflows, making adoption easier for organizations.
Do you think Gemini has potential applications beyond sentiment analysis in the field of technology?
Absolutely, Noah. Gemini has wide-ranging applications, including virtual assistance, content generation, and brainstorming. Its versatility provides opportunities beyond sentiment analysis in various technological domains.
This article has sparked my interest in sentiment analysis. Are there any resources you would recommend for further reading on this topic?
Certainly, Victoria. Here are a few resources to delve deeper into sentiment analysis: 'Sentiment Analysis and Opinion Mining' by Bing Liu, 'Natural Language Processing with Python' by Steven Bird and Ewan Klein, and 'Advances in Sentiment Analysis' edited by Thanh-Nghi Do et al.
How can we overcome the challenge of scalability when applying Gemini to large volumes of data in sentiment analysis tasks?
Great question, Jake. Scaling Gemini for large volumes of data can be achieved through efficient cloud computing resources and parallel processing techniques. Distributing the workload and optimizing the implementation can help overcome scalability challenges.
Are there any visualization techniques or tools that can aid in interpreting the results of sentiment analysis performed using Gemini?
Indeed, Sophie. There are various visualization techniques available to interpret sentiment analysis results. Word clouds, sentiment score distributions, and sentiment trend graphs are commonly used to gain insights and present the findings effectively.
How can businesses leverage Gemini to gain a competitive advantage in sentiment analysis?
Great question, Michael. By leveraging Gemini's sophisticated sentiment analysis capabilities, businesses can gain valuable insights from customer feedback, social media posts, and other textual data. Understanding sentiment allows them to make data-driven decisions and stay ahead of their competitors.
Are there any known biases that Gemini might exhibit in sentiment analysis, and how can we address them?
Valid concern, Emma. Biases in sentiment analysis could arise due to imbalances in the training data. It is crucial to identify and mitigate these biases by continuously evaluating and refining the models, incorporating diverse data sources, and employing fairness metrics to ensure fair and unbiased analysis.