Enhancing Sentiment Understanding in Computational Linguistics with ChatGPT
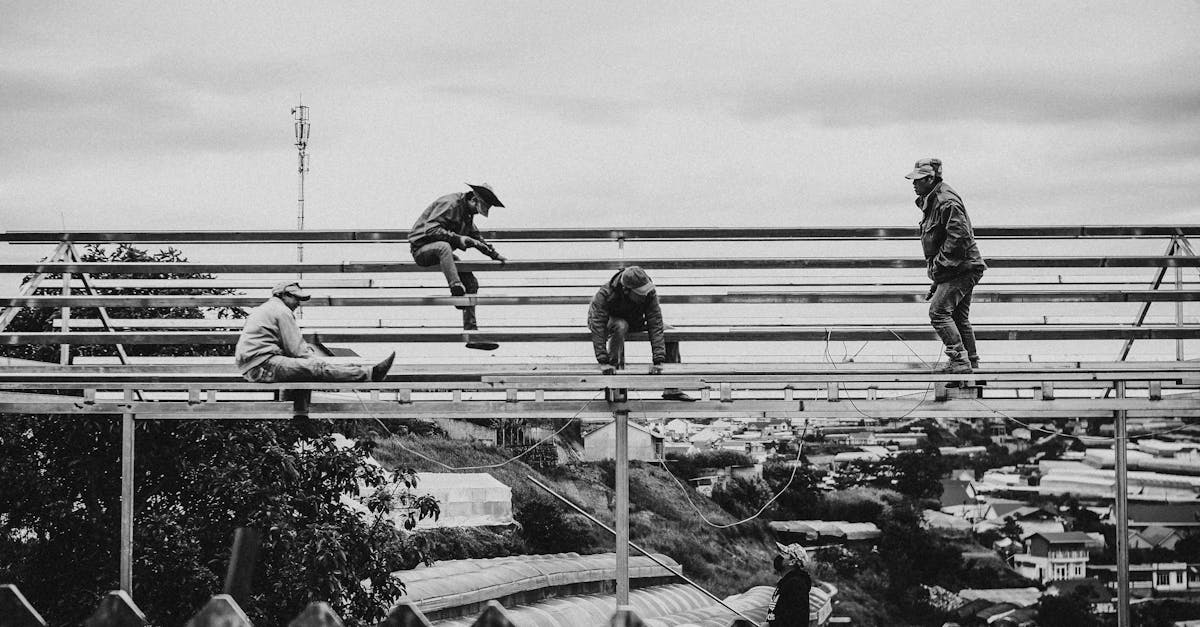
Computational Linguistics is a field of study that combines linguistics and computer science to process and analyze human language with the help of technology. One of the key areas in computational linguistics is Sentiment Understanding, which focuses on determining the sentiment or emotion behind a piece of text.
What is Sentiment Understanding?
Sentiment Understanding involves analyzing written text and identifying the underlying sentiment expressed by the author. It aims to go beyond just identifying positive, negative, or neutral sentiments but also tries to capture nuances and subtle emotions. Sentiment analysis can be applied to a variety of texts, including social media posts, product reviews, news articles, and customer feedback.
How does it Work?
Computational Linguistics employs various techniques to analyze the sentiment in text. Natural language processing (NLP) algorithms are used to preprocess and tokenize the text, breaking it down into smaller units like sentences, phrases, or words. These units are then analyzed using methods like machine learning and pattern recognition to determine the overall sentiment.
One common approach in sentiment analysis is to use a labeled dataset, where each text is annotated with its corresponding sentiment or emotional label. Machine learning models are then trained on this dataset, enabling them to accurately classify new, unlabeled texts based on the patterns in the training data.
Applications of Sentiment Understanding
Sentiment Understanding has wide-ranging applications in various fields:
- Brand Monitoring: Companies can use sentiment analysis to monitor online mentions of their brand and products. This helps them understand how customers perceive their offerings and allows them to make informed business decisions.
- Social Media Analysis: Sentiment analysis is extensively used to analyze social media platforms to gauge public opinion on different topics or events. It helps in monitoring public sentiment towards political campaigns, social movements, or product launches.
- Crisis Management: Sentiment analysis can be crucial for organizations during a crisis. By monitoring social media and news platforms, companies can quickly identify negative sentiments and take appropriate actions to manage the situation and maintain their reputation.
- Customer Feedback Analysis: Sentiment analysis can automatically categorize and analyze customer feedback, allowing companies to identify common pain points and areas of improvement. This helps in enhancing customer satisfaction and making data-driven business decisions.
- Market Research: Sentiment analysis can provide valuable insights for market research. By analyzing the sentiment towards a specific product or service, companies can understand customer preferences, spot emerging trends, and improve their offerings accordingly.
Challenges and Future Scope
Despite significant advancements in Sentiment Understanding, there are still several challenges to overcome. Understanding sarcasm, irony, and context-dependent sentiments remains a complex task. Additionally, the analysis may differ across different languages and cultural contexts.
However, ongoing research and advancements in natural language processing, machine learning, and deep learning techniques continue to improve sentiment analysis accuracy. Future developments in this field might focus on multilingual sentiment analysis, emotion detection, and context-aware sentiment understanding.
In conclusion, Computational Linguistics and Sentiment Understanding play a crucial role in analyzing text and determining the sentiment behind it. Sentiment analysis has a wide range of applications, from brand monitoring to market research. As technology evolves, we can expect even more accurate and nuanced sentiment analysis, enabling us to harness the power of language in a deeper and more meaningful way.
Comments:
This article on enhancing sentiment understanding using ChatGPT is fascinating! I believe natural language processing has come a long way, and this could greatly improve sentiment analysis in various applications.
Thank you, Alex! I'm glad you find the topic interesting. Sentiment analysis is indeed important, and with the advancements in natural language processing, we can expect significant improvements in understanding sentiment. Are there any specific aspects you'd like me to discuss about ChatGPT?
I agree, Alex! Sentiment analysis is crucial in understanding customer feedback and user opinions. ChatGPT seems like a promising approach to enhance this field. It would be interesting to see its performance compared to other methods.
I've been following the progress of sentiment analysis in computational linguistics, and it's exciting to see new techniques like ChatGPT being explored. However, I wonder how ChatGPT handles sarcasm and context-dependent sentiment. These aspects have often been challenging for sentiment analysis models.
Great question, Michael! Context is indeed crucial in sentiment analysis, and capturing sarcasm can be challenging. One approach ChatGPT uses is by leveraging large pre-training datasets that expose it to various forms of language use, which can help handle contextual information. However, sarcasm detection remains an active area of research.
Thank you for your response, Carine! It's good to know that addressing sarcasm and context-dependent sentiment is an ongoing research area. I look forward to seeing the advancements made in this direction.
Carine, it's impressive to hear about the successful applications of ChatGPT in sentiment analysis. The fact that it can adapt to various domains is a major advantage. I can see how it would be beneficial to gain insights from customer feedback and social media sentiment.
Michael, I share your concern about data security. It would be great to have more insights into how OpenAI ensures data confidentiality and prevents the misuse of information in sentiment analysis tasks.
Olivia, privacy and data security are of utmost importance. OpenAI follows strict protocols and standards to ensure data confidentiality and prevent the misuse of information. All user data is anonymized and treated with the highest level of security throughout the development and usage processes of ChatGPT in sentiment analysis and other tasks.
Carine, thank you for elaborating on OpenAI's protocols to ensure data confidentiality. It's reassuring to know that user privacy is a top priority, especially in sentiment analysis where personal opinions and feedback are involved. This transparency inspires trust!
You're welcome, Olivia. Trust is indeed a crucial aspect in AI applications, especially when sensitive data is involved. OpenAI strives for transparency and user trust in all aspects of model development, training, and usage in sentiment analysis and other domains.
Indeed, Michael! ChatGPT's ability to adapt to different domains enables businesses to gain a better understanding of customer sentiment, thus providing opportunities for improving products and services. It's exciting to see the advancements in this field!
I'm curious to know how ChatGPT differs from other sentiment analysis models. What specific features or techniques make it stand out in this field?
Good question, Emily! One aspect that distinguishes ChatGPT is its ability to generate coherent and contextually grounded responses, which can be beneficial in understanding sentiment. Additionally, it can handle a wide range of topics and conversational scenarios, allowing for more versatile sentiment analysis applications.
Emily, one of the reasons ChatGPT stands out is because of its capability to not only understand the sentiment in a given text but also generate informative responses based on that understanding. Its combination of context awareness and coherent output makes it a valuable tool in sentiment analysis applications.
Thank you for the insight, Carine! The ability of ChatGPT to generate coherent responses based on sentiment understanding makes it a promising solution in sentiment analysis. I'm looking forward to exploring it further!
I'm impressed with the potential of ChatGPT for sentiment understanding. However, I'm concerned about potential biases in the training data. How is ChatGPT addressing this issue, especially from a sentiment analysis perspective?
That's an important point, Daniel. Biases in training data can indeed impact the accuracy and fairness of sentiment analysis models. Carine, could you please shed some light on the steps taken to mitigate bias in ChatGPT?
Great concern, Daniel. Addressing biases is essential in natural language processing models. OpenAI is actively working on reducing biases in ChatGPT by using diverse datasets during training, gathering user feedback to inform improvements, and developing ways to allow users to customize the behavior of the model within certain limits to avoid amplifying biases in sentiment analysis.
That's reassuring to hear, Carine. It's important to ensure sentiment analysis models can provide unbiased results, especially in applications involving critical decision-making. Thank you for the clarification!
It's great to see the efforts OpenAI is putting into data privacy and reducing biases in ChatGPT. These are crucial factors to ensure fair and ethical sentiment analysis outcomes. Thank you, Carine, for addressing those concerns!
I couldn't agree more, Daniel. Privacy and fairness are essential in sentiment analysis, and it's heartening to see OpenAI actively working on those aspects. The responsible development and usage of AI models like ChatGPT should be a priority.
Thank you both, Daniel and Alex, for your valuable insights! OpenAI is committed to addressing concerns related to privacy, fairness, and ethics in AI applications. By continuously improving the models and involving users in the feedback loop, we aim to create a responsible and trustworthy sentiment analysis tool.
As a researcher in sentiment analysis, I'm excited about the potential of ChatGPT. However, I'm curious about its limitations. Are there any specific domains or types of sentiments where ChatGPT might struggle?
It's commendable to see OpenAI actively addressing biases in ChatGPT. This kind of transparency during the model development process inspires confidence in its outcomes. Great work!
I'm impressed by the potential of ChatGPT in sentiment analysis. However, I'm also concerned about user privacy when using such models. How does OpenAI ensure the privacy of users' data in sentiment analysis tasks?
Excellent question, Olivia. Privacy is indeed a concern when it comes to using models like ChatGPT. OpenAI has implemented strict privacy policies to safeguard user data. ChatGPT is designed to prioritize user privacy, and steps like anonymizing and securing data are taken during the model development and usage.
I completely agree, Olivia. User privacy is a paramount factor in today's technological advancements. OpenAI's commitment to privacy and their transparent approach instills confidence in using ChatGPT for sentiment analysis and other tasks.
Olivia, I share your concern about privacy. It's crucial to balance the advantages of advanced sentiment analysis models like ChatGPT with user privacy protection. It would be great to know how OpenAI ensures data security and confidentiality.
It's great to know that OpenAI is striving to reduce biases in ChatGPT. Ensuring fairness and accuracy is crucial in sentiment analysis. Thank you for the efforts!
This article highlights an exciting application of ChatGPT in sentiment understanding. Carine, can you share any real-world examples or use cases where ChatGPT has been successfully employed in sentiment analysis?
Certainly, Sophia! ChatGPT has shown promising results in sentiment analysis across various domains. It has been successfully employed in analyzing customer feedback in e-commerce, social media sentiment monitoring, and even in understanding political sentiment during elections. Its versatility and contextual understanding make it adaptable to different scenarios.
Thank you, Carine! It's impressive to see the wide range of applications ChatGPT has been successfully employed in sentiment analysis. I can envision it being immensely useful for businesses and organizations.
Absolutely, Sophia! ChatGPT's ability to analyze sentiment across different domains and platforms can provide valuable insights for decision-making processes and informed business strategies.
Sophia, there are numerous real-world applications for ChatGPT in sentiment analysis. Some examples include brand monitoring to analyze customer sentiment, analyzing user reviews to understand product perception, and analyzing social media sentiments for marketing campaigns. Its broad applicability makes it a valuable tool in various industries.
One question that comes to mind is the scalability of ChatGPT in sentiment analysis. Can it handle large volumes of data efficiently? High-performance and scalability are crucial factors in sentiment analysis applications.
Ryan, scalability is indeed an important consideration when it comes to sentiment analysis applications. ChatGPT has been designed to handle large volumes of data efficiently, thanks to the training processes and infrastructure used. It can process and analyze data at scale, making it suitable for various high-performance use cases.
Carine, that's reassuring to know that ChatGPT can efficiently handle large volumes of data for sentiment analysis tasks. Scalability is indeed crucial for real-world applications. Thank you for addressing the concern!
I'm excited about the advancements in sentiment analysis using ChatGPT. However, I'm curious about potential limitations in multi-lingual sentiment analysis. Can ChatGPT effectively handle sentiment understanding in languages other than English?
Luke, multi-lingual sentiment analysis is an important aspect to consider. While ChatGPT's proficiency in non-English languages is improving, its performance might still vary across different languages. However, OpenAI actively works towards enhancing language support and expanding ChatGPT's capabilities in sentiment analysis across various languages.