Enhancing Signal Integrity: Leveraging ChatGPT for Cross Talk Reduction
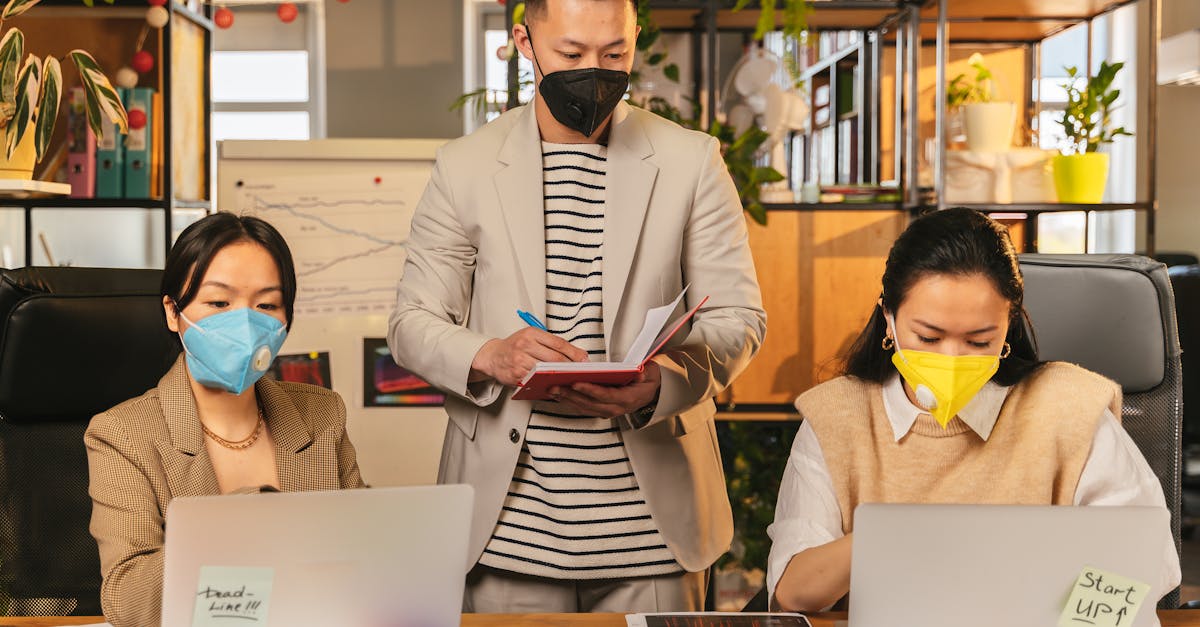
Signal integrity is a crucial aspect in circuit design that ensures reliable and accurate transmission of signals. Cross talk is a common issue that can degrade signal integrity and cause unwanted interference. To mitigate the effects of cross talk, several techniques can be employed in circuit design.
1. Proper Grounding and Shielding
One of the most effective ways to reduce cross talk is to provide proper grounding and shielding techniques. Adequate grounding ensures that the signals have a reference point and helps in reducing noise coupling between different signal paths. Shielding techniques involve enclosing sensitive circuits or wires within conductive material to minimize electromagnetic radiation and external interference.
2. Signal Isolation
Signal isolation techniques are employed to physically separate sensitive signals from noisy signals, minimizing the chances of cross talk. This can be achieved by using isolation transformers, optocouplers, or dedicated ground planes for different signal types.
3. Routing and Tracing
Proper routing and tracing techniques play a crucial role in reducing cross talk. It is important to avoid parallel running of signal traces, especially over longer distances. Adequate spacing between traces and the use of differential signaling can also help minimize cross talk.
4. Capacitive Coupling Reduction
Capacitive coupling is a common cause of cross talk in circuit design. By reducing the coupling capacitance, the effects of cross talk can be minimized. Techniques such as increasing the distance between traces, adding guard traces, or using grounded shields can help mitigate the capacitive coupling effect.
5. Impedance Matching
Impedance mismatches can lead to signal reflections, which can result in cross talk. Proper impedance matching techniques, such as using termination resistors or impedance-controlled routing, help minimize signal reflections and reduce cross talk.
6. Signal Integrity Analysis
Performing signal integrity analysis and simulation using specialized software tools can provide valuable insights into potential cross talk issues. These tools can analyze the circuit design, identify potential areas of cross talk, and suggest suitable solutions to mitigate them.
Conclusion
Reducing cross talk is essential for maintaining signal integrity in circuit design. By implementing appropriate techniques such as proper grounding and shielding, signal isolation, careful routing, capacitive coupling reduction, impedance matching, and thorough signal integrity analysis, engineers can ensure reliable and accurate signal transmission, minimizing the adverse effects of cross talk.
Comments:
Great article, Philip! I found the insights about leveraging ChatGPT for cross-talk reduction fascinating. It's amazing how AI technology can be applied to improve signal integrity.
Thank you, Nancy! I'm glad you found it interesting. AI has definitely opened up new possibilities for enhancing signal integrity. Do you have any specific thoughts or experiences related to cross-talk reduction?
This is a very informative article, Philip. I appreciate the practical examples and explanations provided. It helps me understand how ChatGPT can be utilized in reducing cross-talk.
Thank you, Robert! Giving practical examples and explanations is important to bridge the gap between theory and application. Have you encountered any challenges when dealing with cross-talk in your work?
I've just started learning about signal integrity and this article was extremely helpful, Philip. The concept of using ChatGPT for cross-talk reduction is intriguing. Are there any limitations or risks we need to consider when implementing this approach?
Thanks for your comment, Laura! Implementing AI techniques like ChatGPT for cross-talk reduction does come with its limitations. One limitation is the need for a large dataset to train the model effectively. Additionally, performance may vary based on the specific characteristics of the signals involved. It's essential to thoroughly test and validate the results before deployment.
I'm impressed by the potential of leveraging ChatGPT for cross-talk reduction. Philip, do you have any advice on how to choose the right parameters or input data for achieving optimal results?
Thanks for your question, Mark! When selecting parameters for ChatGPT, it's crucial to consider factors like the size of the training dataset, model architecture, and even hyperparameters like learning rate or batch size. Experimentation and validation are key to finding the optimal configuration for a given application.
Philip, your article provides a clear understanding of the challenges associated with cross-talk and how ChatGPT can aid in reducing it. Are there any drawbacks or trade-offs we should be aware of when implementing this approach?
Thanks for your feedback, Anna! One potential trade-off when using ChatGPT is the possibility of generating inaccurate or nonsensical responses if the training data is not representative or if the model is not properly fine-tuned. Careful preprocessing and domain-specific training can help mitigate this issue.
Intriguing article, Philip! I'm curious about the computational resources required to implement ChatGPT for cross-talk reduction. Are there any guidelines or recommendations for managing resource allocation?
Thank you, Michael! Implementing ChatGPT for cross-talk reduction can be computationally intensive, especially when dealing with large datasets or complex models. It's important to consider factors like available processing power, memory requirements, and implementation scalability. Optimizing both hardware and software resources can help manage the computational load.
This article has opened my eyes to the potential of AI in signal integrity. Philip, what do you see as the future of using ChatGPT or similar models for cross-talk reduction?
I'm glad you found it eye-opening, Eric! The future of using models like ChatGPT for cross-talk reduction is promising. As AI technology advances, we can expect more sophisticated models, better training approaches, and increased accuracy. However, it's important to also consider ethical implications and ensure responsible use of AI in signal integrity applications.
Philip, your article has shed light on the potential benefits of leveraging ChatGPT for cross-talk reduction. Are there any industry-specific applications where this approach has shown significant promise?
Thank you, Matthew! ChatGPT or similar approaches have shown promise in various industries where signal integrity is crucial, such as telecommunications, high-speed data transmission, and electronics manufacturing. The ability to effectively reduce cross-talk can lead to enhanced performance and reliability in these domains.
Philip, your article provides valuable insights into leveraging ChatGPT for cross-talk reduction. Have there been any real-world applications or success stories you can share?
Thanks for your comment, Sophia! There have been successful real-world applications of AI, including ChatGPT, in cross-talk reduction. For instance, in telecommunications, AI models have been used to optimize routing and minimize cross-talk in complex network infrastructures. These applications demonstrate the potential impact of AI in improving signal integrity.
As a beginner in signal integrity, this article gave me a comprehensive understanding of cross-talk reduction using ChatGPT. Philip, do you have any recommendations for additional resources or references?
Thank you, Oliver! For additional resources, I would recommend exploring research papers and publications in the signal integrity field. Additionally, keeping up with advancements in AI research, especially in natural language processing, can provide valuable insights into potential improvements in ChatGPT and similar models for cross-talk reduction.
The concept of leveraging ChatGPT for cross-talk reduction is fascinating. Philip, what are some key considerations to ensure the implementation doesn't introduce new noise or signal integrity issues?
Thanks for your question, Emily! When implementing ChatGPT or any AI approach for cross-talk reduction, it's important to meticulously test and validate the results. Thorough analysis of potential noise or integrity issues is crucial, and adjustments to the training process or model architecture may be necessary to mitigate any negative impact on signal integrity.
Philip, your article highlights the significance of leveraging ChatGPT for cross-talk reduction. Are there any specific challenges or considerations when implementing AI in environments with varying signal characteristics?
Thank you, David! Implementing AI in environments with varying signal characteristics does present challenges. It's important to train the model with diverse and representative datasets to capture different signal behaviors. Additionally, adapting the model or training approaches to be robust against varying characteristics can help maintain a high level of performance in different environments.
This article has given me a deeper understanding of cross-talk reduction and the potential of leveraging ChatGPT for this purpose. Philip, do you foresee any potential legal or ethical considerations when applying AI in signal integrity?
Thanks for your comment, Grace! Implementing AI, including ChatGPT, in signal integrity comes with legal and ethical considerations. Privacy, data protection, and ensuring responsible use of AI are important factors to consider. It's crucial to comply with relevant regulations and conduct ethical assessments to avoid any unintended consequences or misuse.
I'm impressed by the potential impact of leveraging ChatGPT for cross-talk reduction. Philip, can you elaborate on the training process and how it contributes to improved signal integrity?
Thank you, Jonathan! The training process for ChatGPT involves leveraging large datasets and using approaches like unsupervised learning. By exposing the model to various examples of cross-talk and non-interfering signals, it learns patterns and correlations that aid in cross-talk reduction. This training process helps improve signal integrity by enabling the model to produce more accurate responses.
Philip, your article provides valuable insights into ChatGPT's role in cross-talk reduction. How feasible is it to incorporate real-time signal analysis and response generation using this approach?
Thanks for your question, Daniel! Incorporating real-time signal analysis and response generation using ChatGPT is feasible, but it requires considerations regarding the computational requirements and responsiveness of the system. Optimizing the model, infrastructure, and processing pipeline is essential to ensure efficient and timely signal analysis and response generation.
Philip, your article provides excellent insights into leveraging ChatGPT for enhancing signal integrity. Are there any alternative AI approaches or models that can be utilized in cross-talk reduction?
Thank you, Sarah! While ChatGPT is a powerful model, there are alternative AI approaches and models that can be used for cross-talk reduction. Recurrent neural networks (RNNs) and transformers, such as the popular BERT model, have also shown promising results in various natural language processing tasks. Exploring these alternatives and comparing their performance can help identify the most suitable approach for a specific application.
This article has increased my understanding of cross-talk reduction and the potential of leveraging ChatGPT. Philip, have you encountered any specific challenges or limitations when utilizing ChatGPT in signal integrity applications?
Thanks for your comment, Joshua! With ChatGPT, one notable challenge is the potential generation of incorrect responses if the model encounters inputs that deviate significantly from the training data. Fine-tuning the model with diverse and relevant scenarios can help mitigate this challenge. It's essential to carefully consider these limitations when utilizing ChatGPT in signal integrity applications.
Philip, your article provides valuable insights into using ChatGPT for cross-talk reduction. Can this approach be combined with traditional signal integrity techniques for even better results?
Thank you, Liam! Combining ChatGPT with traditional signal integrity techniques can indeed lead to even better results. By integrating AI approaches with well-established practices, it's possible to leverage the strengths of both and achieve enhanced cross-talk reduction. This hybrid approach has the potential to further improve signal integrity in complex scenarios.
I found your article on leveraging ChatGPT for cross-talk reduction thought-provoking, Philip. Given the evolving nature of AI technology, how do you anticipate improvements or advancements in ChatGPT specifically for signal integrity?
Thanks for your feedback, Ava! As AI technology advances, improvements in ChatGPT for signal integrity can be expected. Fine-tuning the model architecture, training approaches, and incorporating domain-specific knowledge can lead to advancements in accuracy, speed, and adaptability. Keeping up with the latest research and continuously refining the methodology will play a crucial role in the future advancements of ChatGPT for signal integrity.
I appreciate your article's insights into ChatGPT's role in cross-talk reduction. Philip, how important is the quality and diversity of the training dataset for achieving optimal results?
Thank you, Christopher! The quality and diversity of the training dataset are crucial for achieving optimal results with ChatGPT. A high-quality dataset that covers a wide range of signal characteristics and potential cross-talk scenarios helps the model learn robust representations. The more diverse and representative the dataset, the better the model's performance in real-world applications.
Philip, your article sheds light on the potential of leveraging ChatGPT for cross-talk reduction. Are there any considerations or challenges related to implementing this approach in safety-critical systems?
Thanks for your question, Victoria! Implementing ChatGPT or any AI approach in safety-critical systems requires careful considerations. The accuracy, reliability, and certification requirements for safety-critical applications are of utmost importance. Thorough testing, validation, and adherence to safety standards should be carried out to ensure the safe and reliable operation of the system.
I found your article on ChatGPT's role in cross-talk reduction intriguing, Philip. How can industry professionals embrace and incorporate AI technology to leverage its benefits in signal integrity?
Thank you, Jacob! Embracing and incorporating AI technology requires industry professionals to stay updated with the latest advancements in the field. Investing in AI research and development, fostering collaboration between AI experts and domain specialists, and conducting proof-of-concept projects can help organizations identify specific applications of AI in signal integrity and realize its benefits.
Philip, your article provides valuable insights into leveraging ChatGPT for cross-talk reduction. Are there any signal types or scenarios where using ChatGPT may not yield satisfactory results?
Thanks for your comment, Olivia! While ChatGPT can be effective in various signal types and scenarios, there may be instances where it may not yield satisfactory results. For example, if the cross-talk behavior deviates significantly from the training data or the model encounters unprecedented signal characteristics, the accuracy of the generated responses may be compromised. It's important to thoroughly test and analyze the model's performance in different scenarios.
Philip, your article provides an informative overview of utilizing ChatGPT for cross-talk reduction. Do you have any recommendations for organizations looking to adopt AI approaches like ChatGPT in their signal integrity workflows?
Thank you for your question, Isabella! Organizations planning to adopt AI approaches should start by thoroughly understanding their signal integrity requirements and defining specific use cases. Building the necessary technical expertise or partnering with AI specialists can aid in developing effective solutions. Conducting pilot projects, analyzing results, and gradually integrating the AI approaches into existing workflows ensures a smooth adoption process.
Philip, your article sheds light on the potential of using ChatGPT for cross-talk reduction. What are some common misconceptions or myths surrounding this approach that you would like to address?
Thanks for your comment, William! One common misconception is that AI approaches like ChatGPT can entirely eliminate cross-talk. While they can significantly contribute to reducing cross-talk, complete elimination may not always be feasible due to various factors like signal characteristics, noise, or system constraints. It's important to set realistic expectations and view AI as a powerful tool that complements traditional signal integrity techniques.