Enhancing Software Prototyping in Full SDLC Technology with ChatGPT
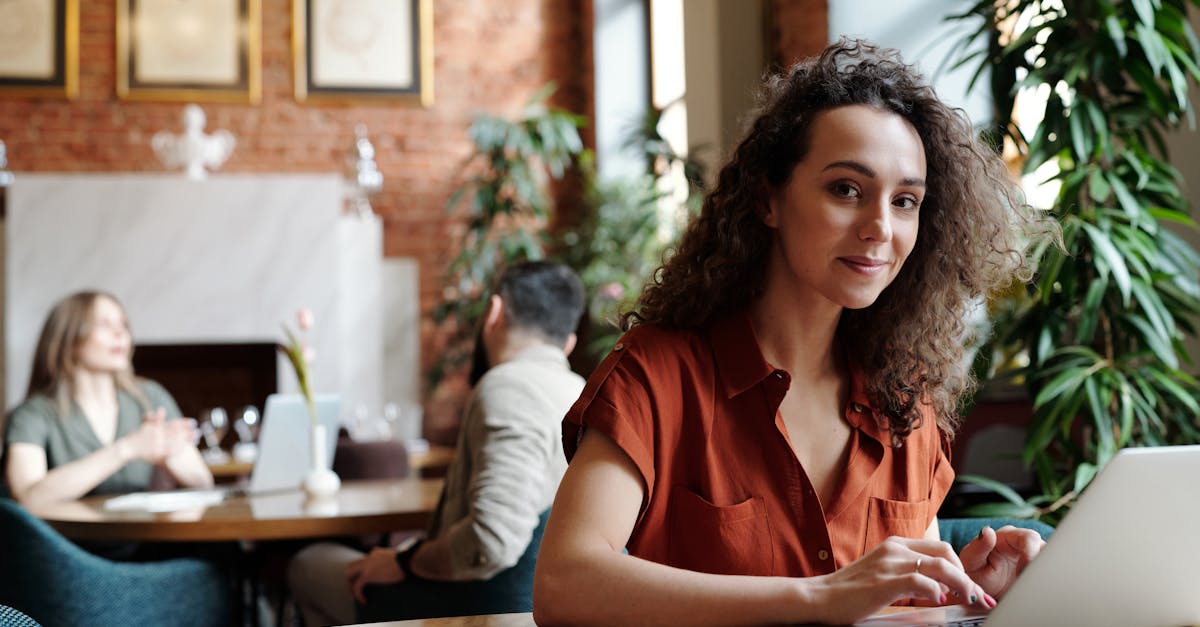
The software development life cycle (SDLC) plays a crucial role in the development of software applications, ensuring quality, efficiency, and success. In the context of software prototyping, SDLC provides a structured approach to developing and testing prototypes before they are deployed in a live environment. This article aims to explore how the full SDLC methodology contributes to software prototyping and its usage in generating simulations and demos for showcasing application functionality.
What is Software Prototyping?
Software prototyping is an iterative process of developing a scaled-down version of a software application. It allows stakeholders, developers, and users to visualize and test the functionality of the final product before investing significant time and resources in its development. Prototyping enables early feedback, identifies potential issues, and helps align the vision of the application with the desired outcomes.
The Phases of Full SDLC
The full SDLC encompasses several well-defined phases that ensure a systematic approach to software development. These phases include:
- Requirements Gathering: Gathering and documenting user requirements and expectations for the application.
- Analysis and Design: Analyzing the requirements and defining the application's architecture, data models, and user interfaces.
- Implementation: Developing the prototype by translating the design into code.
- Testing: Conducting rigorous testing of the prototype to identify and rectify any defects or functional gaps.
- Deployment: Deploying the prototype in a controlled environment for further evaluation.
- Maintenance: Continuously monitoring and enhancing the prototype based on user feedback and changing requirements.
How Full SDLC Benefits Software Prototyping
The full SDLC methodology brings several benefits to the software prototyping process:
- Structured Approach: SDLC provides a structured framework to manage the development process, ensuring that all critical aspects are addressed.
- Clear Requirements: Requirements gathering phase helps define clear goals and expectations for the prototype, minimizing miscommunication and ensuring that the final product meets the users' needs.
- Efficient Design: Analysis and design phase allows developers to create a robust and scalable architecture, ensuring the prototype's stability and flexibility.
- Thorough Testing: Testing phase enables identification and resolution of potential defects early in the development cycle, reducing the risk of issues in the final product.
- Evaluation and Feedback: Deployment phase allows stakeholders to evaluate the prototype's functionality in a controlled environment and provide valuable feedback.
- Continuous Improvement: Maintenance phase allows for continuous enhancements and refinements based on user feedback, ensuring the prototype evolves with changing requirements.
Usage of Full SDLC in Generating Simulations and Demos
ChatGPT-4, an advanced language model, leverages the full SDLC methodology to generate simulations and demos showcasing how an application would function. By following the SDLC phases, ChatGPT-4 can simulate a comprehensive prototype, allowing users to interact with the application's functionalities and experience its potential benefits.
During the requirements gathering phase, ChatGPT-4 can collect user input and discern the specific functionality that needs to be included in the simulation. It then moves on to the analysis and design phase to define the application's architecture, user interfaces, and data models, presenting an accurate representation of the intended application.
The implementation phase involves translating the design into code, generating the necessary logic and interactions for the simulation. ChatGPT-4 can emulate user interactions and provide real-time responses, making the simulation feel interactive and dynamic.
Thorough testing is conducted to ensure the reliability and accuracy of the simulation. By rigorously testing the prototype, ChatGPT-4 can identify and rectify any defects, providing a smooth and functional experience to users.
Once the simulation is ready, it can be deployed in a controlled environment where users can interact with the application's features and functionalities. Stakeholders, developers, and users can evaluate the simulation's usability, provide feedback, and suggest improvements to enhance the final product.
Through the maintenance phase, ChatGPT-4 can incorporate user feedback and evolving requirements to continuously refine and improve the simulation. This iterative approach ensures that the simulation remains up to date and aligned with users' expectations.
Conclusion
The full SDLC methodology plays a critical role in software prototyping, providing a structured approach to developing and testing prototypes before they reach the production stage. The usage of full SDLC in generating simulations and demos, such as those powered by ChatGPT-4, allows stakeholders and users to experience the application's functionalities and envision its potential benefits. By embracing the full SDLC methodology, organizations can ensure that their prototypes are robust, scalable, and aligned with user expectations, leading to the successful development of high-quality software applications.
Comments:
Thank you all for taking the time to read my article on enhancing software prototyping with ChatGPT. I'm excited to discuss this topic with you!
ChatGPT seems like a promising tool for software prototyping. Has anyone here used it before?
Hi Lisa, I've used ChatGPT for prototyping and found it to be quite helpful. It provides quick responses based on the prompts you give it.
I haven't used ChatGPT myself, but I've heard positive things about it. It's great to see it being applied to software prototyping.
I'm curious about the potential limitations of using ChatGPT for software prototyping. Are there any specific challenges?
One challenge could be ensuring that the output provided by ChatGPT aligns with the desired requirements and specifications. It might require careful validation.
Another challenge could be the lack of understanding context-specific domain knowledge. ChatGPT may generate responses that are not accurate for specific industries.
I'm interested in seeing how ChatGPT can integrate with other prototyping tools or platforms. Any insights on that?
Great question, Emily! ChatGPT can be integrated with existing prototyping tools through APIs. A developer can initiate conversations and receive responses programmatically.
That's really convenient. It would allow for a seamless experience within the existing prototyping workflow.
Are there any ethical concerns to consider when using an AI-powered tool like ChatGPT?
One concern could be bias in the generated responses. It's crucial to train and fine-tune AI models like ChatGPT to minimize any potential biases.
Privacy is another aspect to consider. Users need to ensure that sensitive information is not inadvertently shared in conversations with ChatGPT.
Thank you, Sarah and Michael, for bringing up these important points. Addressing bias and privacy concerns is indeed crucial for responsible AI adoption.
How can ChatGPT be extended to support collaborative prototyping?
To support collaborative prototyping, ChatGPT can be used as a shared interface where multiple stakeholders can input prompts and discuss in real-time.
That sounds really useful, especially for remote teams working together on software projects.
Have there been any case studies on the effectiveness of using ChatGPT for software prototyping in a full SDLC?
Yes, Mark. There have been several case studies that showcase the effectiveness of integrating ChatGPT in the software prototyping phase. I can share some links if you're interested.
It would be great to see those case studies. Real-world examples can help us understand the practical benefits of using ChatGPT in the SDLC.
I wonder if there are any alternatives to ChatGPT for software prototyping? Has anyone explored other tools or approaches?
There are other AI-powered tools like OpenAI Codex or IBM Watson that can be explored for software prototyping. Each has its own strengths and limitations.
How easy is it to train ChatGPT for specific business domains or customized scenarios?
Training ChatGPT for specific domains requires domain-specific datasets and fine-tuning techniques. It can be done with proper data collection and preparation.
It's important to note that the quality of training data will significantly impact the accuracy and relevance of the responses.
I'm concerned about potential trust issues when using ChatGPT in the software prototyping process. How can those be addressed?
One way to address trust issues is through extensive testing and validation of the model's outputs against established requirements and expectations.
Transparency is also vital. Providing users with information about the limitations of ChatGPT and its training data can help set realistic expectations.
What are the key advantages of using ChatGPT over traditional prototyping methods?
ChatGPT offers quick and interactive prototyping capabilities, allowing for rapid iterations and feedback. It can speed up the concept validation process.
Additionally, the ability to generate realistic user interactions using ChatGPT can help in visualizing the user experience early on in the prototyping phase.
I'm curious about the learning curve required to effectively use ChatGPT for software prototyping. Can it be easily adopted by non-technical stakeholders?
While non-technical stakeholders might require some initial guidance, ChatGPT can be designed with user-friendly interfaces to enable easy adoption.
Moreover, as the tool evolves and matures, it will likely become more intuitive and accessible to a wider range of users.
I'd love to hear about any success stories or specific use cases where ChatGPT has helped improve software prototyping outcomes.
There have been instances where ChatGPT has assisted in rapidly ideating and shaping new software features. These have significantly accelerated time-to-market.
I remember a case where ChatGPT aided in identifying potential user pain points early on, leading to more user-centered designs.
As a developer, I'm curious about the technical requirements and any potential integration challenges when using ChatGPT in the SDLC.
To integrate ChatGPT, developers would typically require an API key, and there might be some considerations for managing API requests and rate limits.
In terms of requirements, an internet connection is needed to access the ChatGPT model, and there might be some hardware constraints for larger workloads.
Thank you all for your valuable comments and questions. If you have further queries or want to dive deeper into any aspect, feel free to let me know!