Enhancing Speech Recognition with ChatGPT: Advancements in Cognitive Technology
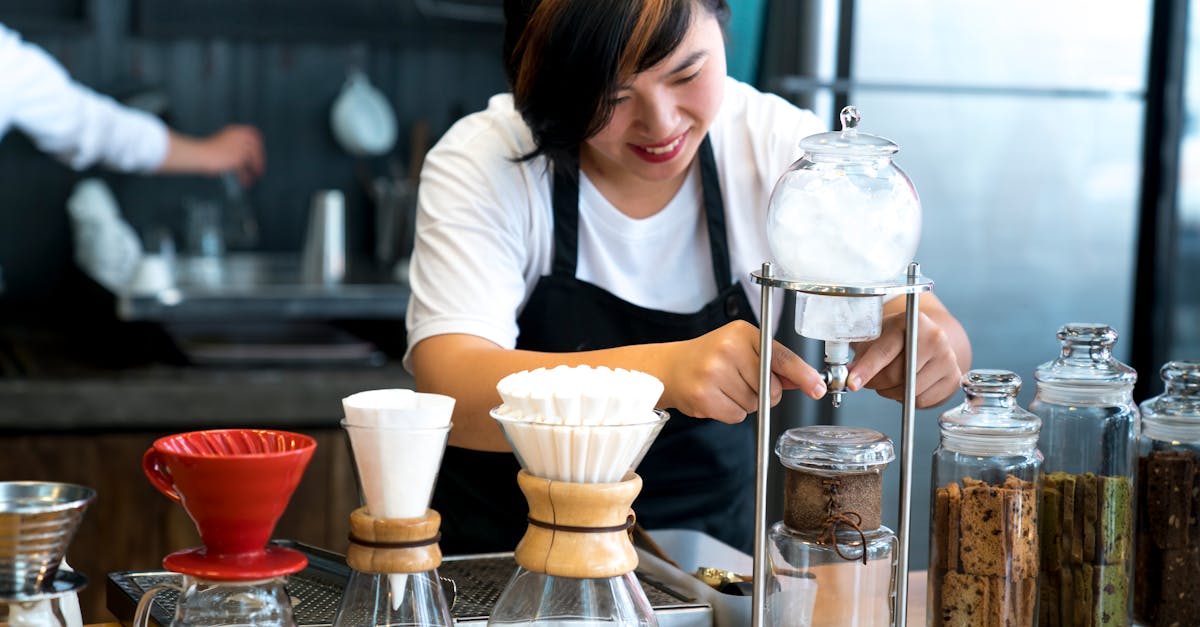
Cognition technology has opened up a plethora of possibilities in various fields, one of which is speech recognition. Speech recognition technology has come a long way in the past few years, but it still faces challenges when it comes to accurately understanding and transcribing spoken language. To overcome these challenges, researchers have been working on integrating advanced language understanding into automatic speech recognition (ASR) systems.
Speech recognition technology, in simple terms, converts spoken words into written text. ASR systems are used in a wide range of applications, including transcription services, voice assistants, and even accessibility tools for individuals with speech disabilities. However, perfect accuracy in transcribing spoken word can be challenging due to variations in accents, speech patterns, and environmental noise.
Advanced language understanding, a subfield of artificial intelligence, aims to enhance the capabilities of ASR systems by enabling them to comprehend language at a deeper level. By incorporating cognitive models, statistical machine learning techniques, and natural language processing algorithms into the ASR pipeline, researchers have made significant progress in achieving more accurate and context-aware speech recognition.
One of the key benefits of advanced language understanding in ASR is the ability to analyze and interpret the semantics of spoken language. Traditional ASR systems primarily focus on acoustic modeling and matching spoken input to a pre-defined set of words or phrases. However, they often struggle with handling homophones (words that sound the same but have different meanings) and contextual understanding. This is where advanced language understanding comes into play.
By leveraging cognitive models and semantic analysis techniques, ASR systems can now better understand the context in which words are used. This leads to improved accuracy in transcribing speech, as the system can make more informed decisions based on the surrounding words and phrases. For example, if the ASR system encounters the word "read," it can determine whether it should be transcribed as "reed" or "red" based on the context of the sentence.
Furthermore, advanced language understanding can also help address challenges posed by variations in accents and dialects. By integrating machine learning techniques, ASR systems can be trained on a diverse range of speech data, allowing them to adapt to different accents and speech patterns. This ensures that the ASR system can accurately transcribe speech regardless of the speaker's background.
Additionally, the incorporation of semantic analysis techniques enables ASR systems to better handle spoken language with multiple meanings and intents. For instance, if a speaker says, "I need some space," an ASR system with advanced language understanding can recognize that the speaker is referring to needing personal space rather than space in a physical sense. This level of comprehension significantly enhances the performance and usability of ASR systems in real-world scenarios.
In conclusion, the integration of advanced language understanding into automatic speech recognition systems holds immense potential for enhancing the accuracy, contextual understanding, and adaptability of ASR technology. By leveraging cognitive models, statistical learning techniques, and semantic analysis algorithms, ASR systems can better comprehend spoken language, leading to improved transcription accuracy and overall user experience. As research in this field continues to progress, we can expect ASR systems to become even more powerful and efficient, making speech recognition an indispensable tool in various applications and industries.
Comments:
Thank you for reading my article on enhancing speech recognition with ChatGPT! I'm excited to join this discussion and answer any questions you may have.
Great article, Terry! I found it really interesting how ChatGPT can help improve speech recognition. Do you think it will be able to handle accents and dialects effectively?
Thanks, Michael! ChatGPT has made significant progress in understanding accents and dialects. While it may still encounter challenges with some variations, ongoing training and fine-tuning efforts aim to improve its adaptability.
I enjoyed reading your article, Terry! How does ChatGPT handle background noise or interference? Can it filter out unwanted sounds effectively?
Thank you, Jessica! ChatGPT has been trained to handle some level of background noise, but loud or persistent interference can affect its performance. Noise reduction techniques and advanced models are being explored to address this limitation.
This is a fascinating topic, Terry! I wonder how ChatGPT performs with multiple speakers or overlapping conversations. Can it distinguish individual voices accurately?
Hi Daniel! ChatGPT still faces challenges in distinguishing multiple speakers and separating overlapping conversations. However, efforts are underway to enhance its capability in handling complex audio scenarios.
Impressive article, Terry! In real-life situations, people often use slang and informal language. Can ChatGPT understand and transcribe such speech accurately?
Thank you, Sophia! ChatGPT has been exposed to diverse language patterns, including informal speech and slang. While its performance is promising, it may still encounter difficulties with certain idiomatic expressions or highly context-dependent phrases.
I found your article very informative, Terry! How does ChatGPT handle interruptions, pauses, or fragmented speech? Can it handle natural conversational speech well?
Hi Emily! ChatGPT can handle pauses and minor interruptions to some extent. However, it might struggle with heavily fragmented or disfluent speech. Continuous improvements are being made to make its recognition more robust in natural conversations.
Interesting article, Terry! Does ChatGPT have limitations with specific languages or does it work well across various languages too?
Thanks, Jonathan! ChatGPT is designed to work with multiple languages, but initially, its proficiency is higher for English. However, efforts are being made to improve its performance in various language options.
Great article, Terry! How do you envision the future of speech recognition technology? What advancements can we expect in the coming years?
Thank you, David! In the future, we can expect speech recognition technology to become more accurate, efficient, and adaptable across diverse scenarios. Ongoing research and advancements aim to improve robustness, handling of complex audio, and support for multiple languages.
Terry, great job on the article! How can businesses benefit from enhancing speech recognition technology? Are there any specific industries that can leverage such advancements?
Hi Olivia! Businesses can benefit from enhanced speech recognition in various ways. Industries like customer service, transcription services, voice assistants, and healthcare can leverage these advancements to automate processes, improve user experiences, and enable better access to spoken content.
Fascinating read, Terry! With the increased reliance on remote work and virtual meetings, how can speech recognition contribute to improving online communication and collaboration?
Thanks, Liam! Speech recognition can enhance online communication and collaboration by providing accurate and real-time transcription during remote meetings, reducing the need for manual note-taking. It can also facilitate accessibility for individuals with hearing impairments.
Terry, your article was very insightful! However, are there any privacy concerns associated with the use of speech recognition technology?
Hi Alexandra! Privacy concerns are certainly important to consider. While speech recognition technology does involve audio processing, it's crucial to prioritize user privacy and ensure compliance with regulations. Transparency in data usage and strong security measures can help address these concerns.
Terry, great article! Do you think there will be a shift towards more voice-based interfaces in the future, replacing traditional text-based interactions?
Thank you, Sophie! While voice-based interfaces are gaining popularity, it's unlikely that they will completely replace traditional text-based interactions. Both have their strengths and complement each other. However, we can expect a greater integration of voice and text in future user interfaces.
Fantastic article, Terry! How can individuals contribute to the improvement of speech recognition technology? Are there ways to provide feedback or help with training models?
Thanks, Ethan! Individuals can contribute to the improvement of speech recognition technology by actively using and providing feedback on speech recognition systems. Some platforms also allow participation in data collection or model training initiatives, helping improve accuracy and versatility.
Great read, Terry! Are there any ethical considerations that should be taken into account while developing and deploying speech recognition technology?
Hi Natalie! Absolutely, ethical considerations are crucial in the development and deployment of speech recognition technology. Ensuring fairness, transparency, and avoiding biases in training data are key aspects. Privacy, user consent, and the responsible use of the technology should also be a priority.
Informative article, Terry! Is there ongoing research to make speech recognition technology more accessible for individuals with speech disabilities or impairments?
Thank you, Brian! Yes, ongoing research focuses on making speech recognition technology more accessible for individuals with speech disabilities or impairments. This includes training models on diverse voice samples and exploring dedicated assistive applications.
Terry, great article! Can ChatGPT be used in real-time applications, such as live transcription during speeches or lectures?
Thanks, Emma! ChatGPT can be used for real-time applications like live transcription, but it's important to note that it might have latency and accuracy limitations, especially in complex and fast-paced scenarios. Ongoing research aims to improve its suitability for real-time use cases.
Very interesting article, Terry! How can speech recognition technology contribute to the field of education?
Hi Victoria! Speech recognition technology can contribute to education in various ways. It can assist in transcribing and analyzing classroom discussions, provide real-time feedback during language learning, and even support students with note-taking or learning disabilities.
Fantastic article, Terry! Are there any notable challenges that remain in the field of speech recognition?
Thank you, Andrew! While significant progress has been made, challenges remain in areas like handling multiple speakers, noisy environments, and highly disfluent speech. Improving robustness and addressing biases are also important focus areas for the field.
Terry, your article was a great read! What are the key considerations for selecting or building speech recognition models for specific applications?
Thanks, Grace! When selecting or building speech recognition models, factors like accuracy, latency, adaptability to specific domains or languages, and available training data should be considered. It's crucial to evaluate models based on target use cases and ensure they align with the desired requirements.
Great insights, Terry! How do you foresee the integration of speech recognition technology with other emerging technologies like artificial intelligence and natural language processing?
Hi Max! Speech recognition technology is closely intertwined with artificial intelligence and natural language processing. Integration with these technologies enables automatic speech understanding, context-aware interactions, and enhances overall user experiences. We can expect further convergence and synergy between them in the future.
Very informative article, Terry! How does ChatGPT handle specialized or domain-specific vocabulary during speech recognition?
Thank you, Sophie! ChatGPT can handle specialized or domain-specific vocabulary to some extent, especially if it has been exposed to such language patterns during training. However, there might be limitations, and fine-tuning models with specific vocabulary can help improve accuracy in specialized domains.
Terry, great article! Can ChatGPT recognize and transcribe non-verbal vocal cues like laughter, hesitation, or emotion effectively?
Thanks, Ashley! ChatGPT can recognize and transcribe non-verbal vocal cues to some extent, but its ability to capture nuanced emotions, subtleties, or complex non-verbal cues is still limited. Further research and training efforts aim to improve these aspects.
Well-written article, Terry! Can ChatGPT handle speech with heavy accents, such as regional or non-native accents, effectively?
Thank you, Sophia! ChatGPT has been trained on diverse accents, including regional and non-native ones. While its performance is promising, challenges can still arise with some variations. The ongoing training and fine-tuning aim to address these issues and improve accent adaptation.
Terry, great insights in your article! How can individuals contribute to the training of speech recognition models?
Thanks, Alexander! Individuals can contribute to the training of speech recognition models by providing feedback on transcription accuracy, participating in data collection initiatives, or sharing voice samples representative of various dialects, accents, or speaking styles. These contributions help improve models' performance and adaptability.
Insightful article, Terry! How do advancements in speech recognition align with the broader goals of human-computer interaction research?
Hi Sarah! Advancements in speech recognition align with the broader goals of human-computer interaction research by striving to make interactions with technology more natural, intuitive, and efficient. It aims to bridge the gap between spoken and written language, enabling seamless communication with computers.
Great article, Terry! Do you think ChatGPT will be able to handle domain-specific jargon or technical terms effectively in the future?
Thanks, Brandon! While ChatGPT can already handle some domain-specific jargon, its effectiveness with technical terms heavily depends on the training data and fine-tuning. As models improve and more specialized data becomes available, the accuracy with domain-specific terms is expected to increase.