Enhancing Statistical Modeling with ChatGPT: A Breakthrough in Statistics Technology
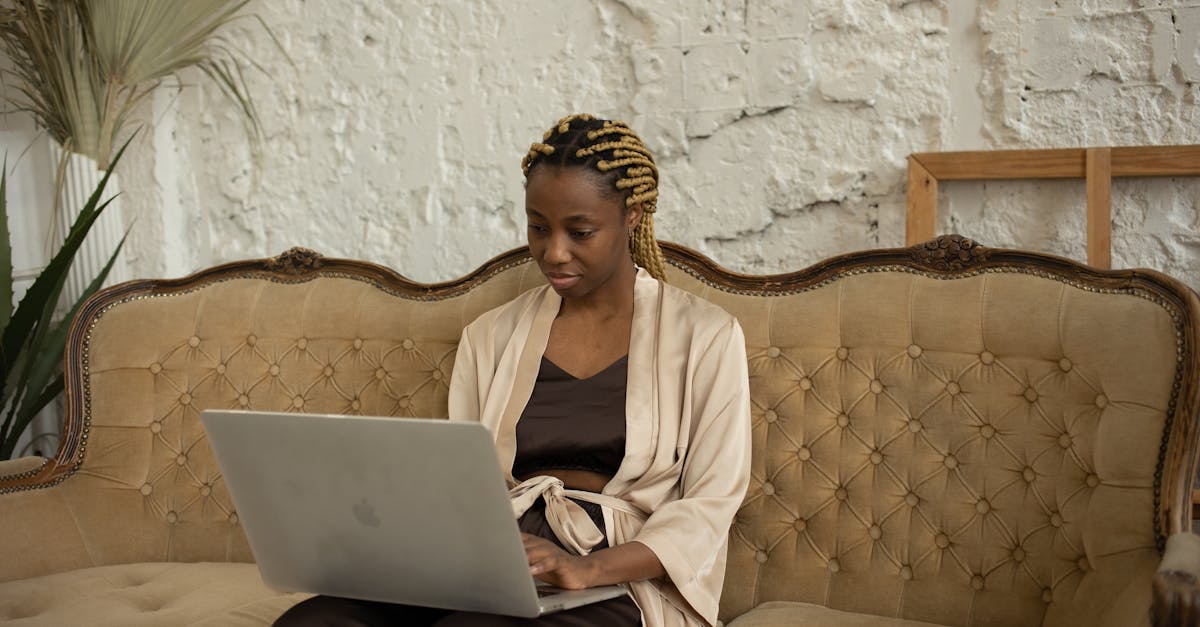
Statistical modeling plays a vital role in various domains to gain insights from data. With the emergence of advanced AI tools like ChatGPT-4, understanding and explaining statistical models have become much more accessible and user-friendly.
Introduction to Statistical Modeling
Statistical modeling is a technique used to describe the relationship between variables and to make predictions and inferences based on the available data. It involves the application of statistical concepts, theories, and methods to design and analyze data for various real-world scenarios.
The Role of ChatGPT-4
ChatGPT-4, powered by advanced deep learning algorithms, can assist users in comprehending and explaining various statistical models. It can provide valuable insights into linear models, generalized linear models, mixed-effects models, and their applications across different domains.
Linear Models
Linear models are the simplest form of statistical models that assume a linear relationship between the dependent variable and one or more independent variables. They are widely used in regression analysis to predict and explain continuous numerical outcomes. ChatGPT-4 can help users understand the underlying assumptions, estimation techniques, and interpretation of linear models.
Generalized Linear Models
Generalized linear models (GLMs) extend the concept of linear models to handle a broader range of response variables. Unlike linear models, GLMs can handle categorical, binary, and count data, making them suitable for various scenarios. With ChatGPT-4's assistance, users can explore the different types of GLMs (e.g., logistic regression, Poisson regression) and understand their specific use cases and interpretations.
Mixed-Effects Models
Mixed-effects models, also known as hierarchical models or multilevel models, are statistical models that capture the dependencies and variations within nested data structures. These models are extensively utilized in social sciences, medical research, and other domains where data exhibits clustering or hierarchical nature. ChatGPT-4 can help users grasp the concepts of random effects, fixed effects, and model selection in mixed-effects modeling.
Applications of Statistical Models
Statistical modeling finds applications in diverse domains such as healthcare, finance, marketing, social sciences, and more. Healthcare professionals can utilize statistical models to predict disease outcomes, assess treatment effectiveness, and identify risk factors. Marketing teams can leverage these models to analyze customer behavior and design effective marketing strategies. With the support of ChatGPT-4, users can explore specific use cases and gain a deeper understanding of how statistical modeling is applied in their respective fields.
Conclusion
ChatGPT-4's capabilities in facilitating the understanding of statistical models like linear models, generalized linear models, and mixed-effects models are invaluable for users across different domains. With its assistance, users can navigate complex statistical concepts, understand underlying assumptions, and apply the right modeling techniques for their data analysis needs. The fusion of advanced machine learning technologies and statistical modeling is empowering users to make data-driven decisions and extract meaningful insights from their data.
Comments:
This article on enhancing statistical modeling with ChatGPT is fascinating! It's incredible to see how technology is advancing in this field.
I completely agree, Michael. The potential for ChatGPT to revolutionize statistical modeling is huge. I'm excited to learn more about its applications.
As a statistician, I'm thrilled about the possibilities this technology offers. It could greatly enhance our ability to analyze complex datasets and make accurate predictions.
I wonder how ChatGPT compares to traditional statistical modeling methods. Are there any limitations or potential drawbacks to using it?
Great question, Emily! While ChatGPT has shown promising results, like any technology, it has limitations. One limitation is its reliance on large amounts of high-quality training data to produce accurate models.
Another potential drawback is the lack of interpretability in the models generated by ChatGPT. It's difficult to understand the underlying reasoning behind the predictions it makes.
Thank you, Virginia and Peter, for sharing the limitations. It's important to consider these factors when integrating ChatGPT into statistical modeling workflows.
I can definitely see the value of incorporating ChatGPT into statistical analysis. It could offer new insights and potentially speed up the modeling process.
I have some concerns about bias in the training data used for ChatGPT. How can we ensure the models it produces are fair and unbiased?
Valid point, Daniel. Bias in training data can indeed affect the fairness of the models. One approach is to carefully curate and diversify the training data to minimize bias as much as possible.
Another important step is thorough evaluation and testing. It's crucial to assess the models for any unintended biases before applying them in real-world scenarios.
I agree, Ethan. Regular audits and ongoing monitoring of the models' outputs can help identify and address any biases that may arise.
This article really got me excited about the future of statistics. ChatGPT seems like a powerful tool that can unlock new possibilities in data analysis.
I'm curious to know if there are specific use cases where ChatGPT has already been successful in enhancing statistical modeling. Does anyone have examples?
Absolutely, Benjamin! ChatGPT has been utilized in various domains, such as finance, healthcare, and marketing, to improve data-driven decision-making and predictive analytics.
In the healthcare field, ChatGPT has shown promise in analyzing patient data and assisting in diagnosis, helping medical professionals make evidence-based decisions.
That's correct, Emily. By leveraging ChatGPT's capabilities, healthcare providers can enhance their statistical models for more accurate predictions and personalized patient care.
That's an important point, Virginia. It's crucial to understand that ChatGPT should be used as a tool to assist statisticians, rather than replace their expertise and critical thinking.
I'm concerned about the potential ethical implications of using ChatGPT in statistical modeling. What steps are being taken to address this?
Ethical considerations are crucial, Jacob. Initiatives like responsible AI development and ensuring transparency in model deployment are being actively pursued to mitigate ethical concerns.
I couldn't agree more, Sophia. It's essential to prioritize ethical practices while leveraging the power of technologies like ChatGPT to ensure their responsible and beneficial use.
What are the key advantages of integrating ChatGPT with statistical modeling compared to traditional approaches?
One advantage is the ability of ChatGPT to handle unstructured data, such as text or natural language inputs, which can be challenging for traditional statistical approaches.
Additionally, ChatGPT's ability to learn from large amounts of diverse data can help uncover hidden patterns and relationships that traditional modeling approaches may miss.
The flexibility and adaptability of ChatGPT can also be advantageous. It can easily be fine-tuned and customized for specific use cases, making it highly versatile.
Are there any risks associated with relying heavily on ChatGPT for statistical modeling tasks?
Good question, Daniel. Overreliance on ChatGPT without proper validation and human expertise can lead to potential inaccuracies and incorrect conclusions.
I'm interested to know how ChatGPT can handle missing or incomplete data in statistical modeling. Is it able to adapt and still produce meaningful results?
ChatGPT does have the ability to handle missing or incomplete data to some extent. However, careful preprocessing and imputation techniques are often needed to ensure valid results.
It's important to note that while ChatGPT can help in handling missing data, depending solely on it may still introduce biases or inaccuracies. Human oversight is critical.
Can ChatGPT handle large-scale datasets in statistical modeling? Are there any practical limitations?
ChatGPT has been designed to handle large-scale datasets effectively. However, the computational resources required can be a practical limitation for certain setups.
Indeed, David. It's important to have sufficient computational power and infrastructure in place to leverage the full potential of ChatGPT for large-scale statistical modeling.
Could ChatGPT be used to enhance time series forecasting in statistics? It seems like it could have valuable applications in that area.
Absolutely, Ethan! ChatGPT's ability to capture complex patterns and dependencies can be beneficial for time series forecasting, enabling better predictions and insights.
Furthermore, by incorporating external factors and domain-specific knowledge, ChatGPT can improve the accuracy of time series models, especially in complex and dynamic environments.
I wonder if ChatGPT's performance is comparable to other advanced statistical modeling techniques like deep learning or Bayesian networks?
While ChatGPT can achieve impressive results, it's worth noting that its performance may vary depending on the specific use case and the availability of high-quality training data.
Deep learning and Bayesian networks have their own strengths and applications. The choice between these techniques ultimately depends on the problem at hand and the available resources.
Overall, it's undeniable that ChatGPT has the potential to significantly enhance statistical modeling. However, we must be aware of its limitations and ethical considerations for responsible usage.