Enhancing Stochastic Modeling in Technology with ChatGPT
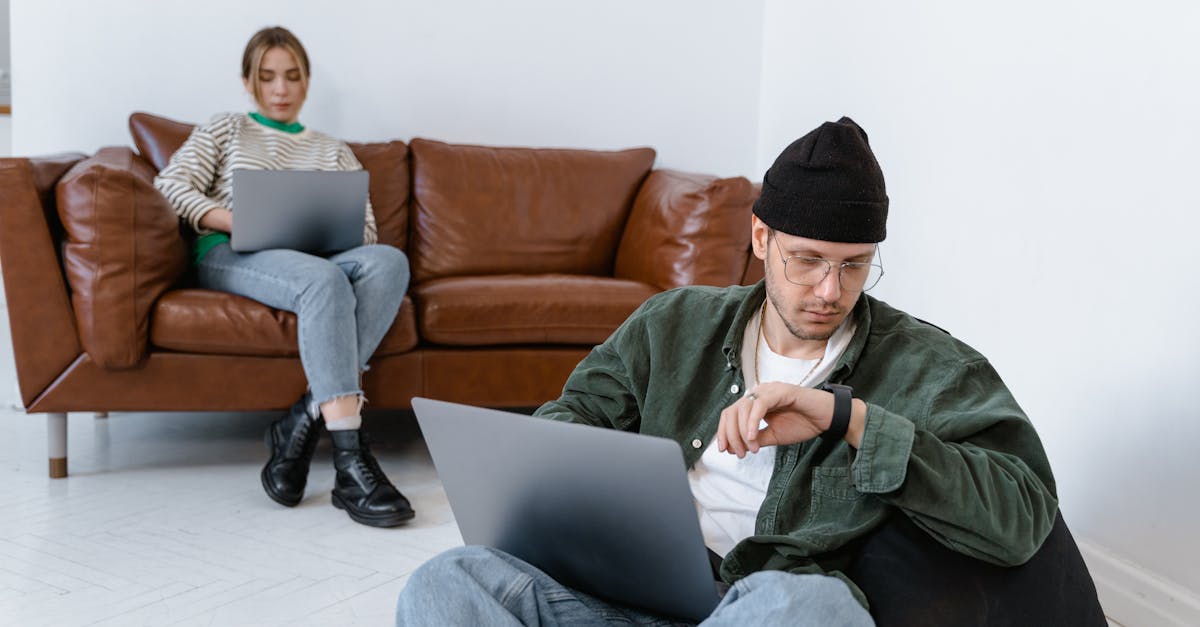
In this modern era of evolving technology, computational techniques become increasingly significant in various fields. The area of finance, in particular, sees considerable implementations of advanced technologies. One such technology is Stochastic Modeling. Stochastic Modeling is a mathematical approach used to estimate probabilities. It involves the use of random variables to model different potential outcomes in a process. The most simplistic definition may be that Stochastic Modeling is the generation of random variables to evaluate and predict outcomes. For our purposes, we will focus on the area of financial forecasting.
Stochastic Modeling in Financial Forecasting
In financial forecasting, Stochastic Models give a comprehensive framework to study the market dynamics that cannot be perfectly predicted. Stochastic modeling enables businesses to evaluate investing and pricing strategies, make predictions about market trends, better understand uncertainty, and make informed decisions.
We use these models to generate different possible future outcomes for stock prices, interest rates, inflation rates, returns on investments, etc. Their powerful analysis abilities help businesses to optimize their operational strategies as per the current market patterns to maximize their profits and minimize their potential losses.
Supercharging Financial Forecasting with ChatGPT-4
ChatGPT-4, an evolution of the popular AI model developed by OpenAI, brings this usage of Stochastic Modeling to a new level. Integrated with sophisticated learning capabilities, the model provides holistic predictions and insights into the financial world.
More than just predictions, ChatGPT-4 can learn from and adapt to changes in market scenarios, providing timely and more accurate forecasts. It can also provide a descriptive analysis of the reasons behind the predicted trends giving its users a more comprehensive understanding of the underlying factors influencing the market.
The key idea behind using ChatGPT-4 with Stochastic Models is its high adaptability to constantly evolving markets. It can learn from the past and present market trends and accordingly adjust its internal parameters to give more precise forecasts for future trends.
This helps in reducing the uncertainty in the market predictions and aids in making more informed decisions regarding investments. Thus, ChatGPT-4 when combined with Stochastic Modeling can assist users in understanding financial markets better.
Conclusion
Stochastic Modeling is a powerful approach when it comes to financial forecasting due to its ability to model uncertain outcomes and provide valuable insights for decision making. Its integration with ChatGPT-4 is likely to revolutionize not just how businesses predict financial trends but also how they react to them.
By streamlining financial forecasting and providing more accurate and timely predictions, these technologies can hugely impact the field of finance. It is a clear indication of how rapidly evolving AI models are starting to permeate various aspects of our lives and industries, promising better efficiency and accuracy.
It'll be wise for anyone interested or involved in financial forecasting to keep an eye on these evolving trends and technologies. Surely, the optimal use of such models would lead us to a future where financial management becomes significantly easier and efficient.
Comments:
Thank you all for reading my article on Enhancing Stochastic Modeling in Technology with ChatGPT. I'm excited to hear your thoughts and answer any questions you may have!
Great article, Mike! I found your insights on leveraging ChatGPT for stochastic modeling really interesting. I can see how this approach can add value in various tech domains.
Thanks, Samuel! I appreciate your kind words. ChatGPT's ability to generate realistic text opens up new possibilities for refining stochastic models.
I have some concerns about the accuracy of using ChatGPT for stochastic modeling. How can we ensure that the generated text aligns with real-world probabilities?
That's a valid concern, Emily. To enhance accuracy, it's crucial to fine-tune the model on relevant data and validate the generated outputs against real-world probabilities.
Mike, I really enjoyed your article! ChatGPT seems like a wonderful tool to augment stochastic modeling. Have you encountered any limitations or challenges when using it in practice?
Thanks, John! While ChatGPT is powerful, it can sometimes produce responses that are contextually accurate but factually incorrect. It's important to carefully validate the generated outputs and complement them with other techniques.
Thank you, Mike, for actively participating in the discussion and providing insightful responses. Your expertise has been enlightening.
You're welcome, John! I'm glad I could contribute to the discussion and provide valuable insights. Your kind words are appreciated. Feel free to connect if you have more questions or ideas to explore.
I loved the practical examples you provided in the article, Mike! It helped me understand how ChatGPT can be applied in stochastic modeling. Do you have any recommended resources to learn more about fine-tuning the model?
Thank you, Natalie! For fine-tuning ChatGPT, OpenAI provides detailed documentation and guides on their website. They also offer pre-trained models and datasets for various domains, which can be a great starting point.
Mike, excellent article! I'm curious about the computational requirements when using ChatGPT for stochastic modeling. Does it demand significant computational resources?
Thanks, Daniel! The computational requirements can depend on the size of the model and the complexity of the tasks you're working on. Generally, larger models require more computational resources. However, OpenAI has introduced smaller versions of ChatGPT that can be more accessible.
Great article, Mike! I'm wondering how ChatGPT handles uncertainty in stochastic modeling. Can it generate probabilistic outputs or quantify its confidence in the predictions?
Thank you, Olivia! ChatGPT focuses on generating coherent responses rather than producing probabilistic outputs. However, by sampling multiple responses, you can get a sense of the model's variability and assess the uncertainty.
Thank you, Mike, for engaging with us and patiently answering our questions about ChatGPT in stochastic modeling. Your input has been highly valuable.
You're welcome, Olivia! I'm glad I could engage with you all and provide valuable insights. Your appreciation is greatly appreciated. If there's anything else you'd like to discuss, feel free to let me know.
Thank you, Mike, for your contributions to this discussion. Your guidance on the topic of using ChatGPT in stochastic modeling has been invaluable.
You're welcome, Andrew! I'm pleased that my contributions were helpful to you. If you have further queries or need further assistance, feel free to reach out.
Hi Mike, I'm impressed with the potential of using ChatGPT for stochastic modeling. Are there any ethical considerations we should keep in mind while leveraging this technology?
Hi Sophia, ethical considerations are crucial. As with any AI technology, we should be mindful of bias in the training data, potential misuse, and understand the limits of the model's expertise. Regular audits and diverse perspectives can help mitigate these concerns.
Mike, thanks for sharing your insights on enhancing stochastic modeling with ChatGPT. Are there any alternative approaches you would recommend alongside using ChatGPT?
You're welcome, Jacob! ChatGPT can be complemented with traditional modeling techniques, expert domain knowledge, and real-world data validation. A hybrid approach often yields robust results.
Hi Mike, great article! How easy is it to integrate ChatGPT with existing stochastic modeling frameworks or software tools?
Thank you, Sara! While integration specifics may vary depending on the framework or software, general integration involves utilizing the model's API and adapting it to fit your existing pipeline. OpenAI provides support and resources to facilitate this process.
Mike, thanks for shedding light on the potential of ChatGPT in stochastic modeling. Can it handle complex scenarios with multiple variables and dependencies?
You're welcome, Alex! ChatGPT can handle complex scenarios, but it's important to note that the model's responses are based on patterns learned from training data. Ensuring high-quality training data and appropriate fine-tuning can help capture the desired complexity.
Mike, I loved your article! How do you see the future of stochastic modeling evolving with advancements in AI language models like ChatGPT?
Thank you, Jasmine! I believe AI language models like ChatGPT will play a significant role in advancing stochastic modeling. They have the potential to offer better accuracy, improved understanding of context, and more efficient exploration of probabilistic spaces.
Mike, your article was quite informative. I'm curious about the computational costs associated with fine-tuning ChatGPT for stochastic modeling. Are they significant?
Thanks, Liam! Fine-tuning ChatGPT can be computationally expensive, especially when working with large-scale models. However, you can leverage cloud computing resources or pre-trained models as a starting point to mitigate these costs.
Hi Mike, your article discusses the benefits of using ChatGPT in stochastic modeling, but are there any potential downsides we should be aware of?
Hi Ella, while ChatGPT offers valuable capabilities, it's essential to be cautious regarding the accuracy of the generated text and potential biases. Additionally, it requires careful monitoring and validation to ensure its suitability for the intended tasks.
Thank you, Mike, for sharing your knowledge and guiding us through the nuances of leveraging ChatGPT in stochastic modeling. Your expertise is invaluable.
You're welcome, Ella! It was my pleasure to share my knowledge and assist you throughout the discussion. Your appreciation means a lot. If you have further inquiries, feel free to ask.
Mike, your article was insightful! Have you tested ChatGPT on any specific applications within stochastic modeling? I would love to hear about any practical implementations.
Thank you, Robert! ChatGPT has shown promise in various stochastic modeling applications, such as generating synthetic data for simulations, addressing missing data issues, and refining probabilistic forecasts. Its versatility makes it valuable across different domains.
Mike, excellent article! I'm curious about the limitations of ChatGPT in terms of the complexity it can handle in stochastic modeling. Are there any trade-offs?
Thanks, Sophie! ChatGPT can handle considerable complexity, but it's vital to consider the model's training data and the trade-offs between generating coherent responses and capturing nuanced probabilistic behaviors. Balancing these aspects is crucial for optimal results.
Mike, I really enjoyed your article! As ChatGPT relies heavily on training data, how do you address potential biases in the generated text?
Thank you, David! Addressing biases requires careful curation and diversification of the training data to avoid over-representation of certain perspectives. It's an ongoing concern that should be continually monitored and actively managed.
Mike, your participation in this discussion has been truly insightful. Thank you for sharing your expertise and addressing our concerns.
You're welcome, David! I'm glad I could provide you with insightful information and address your concerns. If you have any more questions or thoughts to discuss, feel free to reach out.
Fantastic article, Mike! I'm interested in the scalability of using ChatGPT for large-scale stochastic modeling problems. Are there any considerations or limitations when applying it to extensive systems?
I'm glad you liked it, Lucy! When dealing with large-scale systems, computational resources can become a significant consideration. It's important to assess the trade-offs between model complexity, available resources, and the desired level of accuracy for efficient application.
Mike, your article showcased the potential of ChatGPT in stochastic modeling. I'm curious about the interpretability of the model's responses. How can we ensure transparency and understand the underlying logic?
Great question, Henry! The interpretability of ChatGPT's responses can be challenging. Techniques like attention maps and rule-based post-processing can help gain insights into its decisions, but complete transparency may be difficult to achieve due to its language-based nature.
Hi, Mike! Your article was fascinating. What are your thoughts on potential biases and ethical implications when using ChatGPT for stochastic modeling, considering its training data sources?
Hi Alice! Mitigating biases and ethical concerns should be a priority. OpenAI is actively promoting research and engineering efforts to reduce both glaring and subtle biases in AI models. They're working toward increasing transparency and involving diverse perspectives in model development.
Mike, your expertise and prompt responses in this discussion have made the topic of using ChatGPT in stochastic modeling much clearer. Thank you!
You're welcome, Alice! I'm delighted to hear that my expertise and prompt responses have clarified the topic for you. Your kind words mean a lot to me. If you have more questions or need further clarification, don't hesitate to ask.
Thank you, Mike, for your engagement and insightful responses. Your expertise has enhanced our understanding of using ChatGPT for stochastic modeling.
You're welcome, Jennifer! I'm glad I could contribute to the discussion and enhance your understanding. Your appreciation is valued. If you ever need further insights or have more questions, feel free to reach out.
Mike, your article shed light on an exciting application of ChatGPT. Can you recommend any specific areas or industries where leveraging ChatGPT for stochastic modeling can be particularly beneficial?
Absolutely, Connor! ChatGPT can be valuable in industries such as finance, supply chain management, energy systems, and healthcare, where robust stochastic modeling contributes to informed decision-making and risk analysis.
Hi Mike, your article was very insightful! Are there any limitations of ChatGPT in terms of its ability to understand and handle stochastic processes with non-linear dynamics?
Thank you, Erica! ChatGPT's ability to understand and handle stochastic processes with non-linear dynamics is limited. For such cases, it's beneficial to combine ChatGPT with traditional modeling approaches to capture complex dynamics.
Mike, I enjoyed reading your article. How do you see the role of human experts alongside ChatGPT in stochastic modeling?
I'm glad you enjoyed it, Melissa! Human experts play a critical role in stochastic modeling. While ChatGPT can enhance the process, it's important to have domain experts validate the outputs, provide guidance, and interpret the results to ensure accuracy.
Mike, your article was both informative and thought-provoking. Are there any regulatory or legal considerations we should be aware of when using ChatGPT in stochastic modeling applications?
Thank you, Laura! Regulatory and legal considerations are essential. Depending on the specific application and industry, there may be requirements related to privacy, data usage, and accountability. It's crucial to adhere to relevant regulations and seek legal guidance when necessary.
Mike, I found your article quite engaging! How can we quantify the uncertainty or confidence in the outputs generated by ChatGPT?
Thanks, Lucas! Quantifying uncertainty or confidence in ChatGPT's outputs can be challenging since it doesn't produce explicit probability estimates. However, you can explore techniques like ensemble sampling or calibration to gain insights into the model's reliability.
Mike, your active participation and guidance throughout this discussion have been highly beneficial. Thank you for sharing your expertise.
Mike, your article was enlightening! Can ChatGPT be used for real-time stochastic modeling, or does it have significant response time limitations?
Thank you, Maria! ChatGPT's response time can be a limiting factor for real-time stochastic modeling. The inference time depends on the model size, available computational resources, and the complexity of the request. For real-time tasks, optimizing the system's architecture and employing efficient computation can help overcome these limitations.
Mike, great article! How do you address the issue of bias in training data, especially when it comes to capturing diverse perspectives and accounting for rare events?
Thanks, Benjamin! Addressing bias in training data requires an inclusive and diverse dataset that accounts for a broad range of perspectives. Actively seeking out and collecting data that represents rare events or underrepresented groups is crucial for mitigating bias.
Mike, I enjoyed your article on incorporating ChatGPT into stochastic modeling. Could you shed some light on the model's training process and the fine-tuning steps involved?
I'm glad you enjoyed it, Lily! ChatGPT's training process involves pretraining on a large corpus of publicly available text. Fine-tuning is done on custom datasets, created with human reviewers using guidelines provided by OpenAI. Iterative feedback and experimentation make up a crucial part of the fine-tuning process.
Mike, your article provided great insights into using ChatGPT for stochastic modeling. Can you elaborate on how to ensure model consistency and stability when working with dynamic data?
Thank you, Julia! Ensuring model consistency and stability with dynamic data requires regular updates to the model's training and fine-tuning process. As new data becomes available, retraining the model periodically helps to capture the evolving patterns and maintain performance.
Mike, I found your article on integrating ChatGPT in stochastic modeling quite informative. Can you share any best practices to improve the model's performance in this context?
I'm glad you found it informative, Daniel! To improve ChatGPT's performance in stochastic modeling, fine-tuning on relevant and high-quality datasets, leveraging ensemble methods, and actively validating the model's outputs with real-world data can lead to better results.
Thank you, Mike! Your insights have been valuable, and your responses addressed my questions effectively.
You're welcome, Daniel! I'm glad I could provide you with valuable insights and address your questions. If you have any further inquiries, feel free to ask.
Thank you, Mike, for sharing your expertise and clarifying various aspects related to using ChatGPT in stochastic modeling. Much appreciated!
You're welcome, Amelia! I'm glad I could assist in clarifying the nuances. Your appreciation means a lot. If you have any more questions in the future, don't hesitate to reach out.
Mike, your article presented a compelling use case. How do you approach challenges related to capturing long-term dependencies and complex patterns in stochastic modeling using ChatGPT?
Thank you, Oliver! Capturing long-term dependencies and complex patterns can be challenging with ChatGPT. Augmenting the model with attention mechanisms and leveraging larger contexts during training can help in addressing these challenges.
Great article, Mike! Have you encountered scenarios where the generated responses from ChatGPT have significantly deviated from the expected behavior in stochastic modeling?
Thanks, Emily! Yes, there have been instances where ChatGPT's responses deviated from expected behavior. Careful monitoring, validation, and validating against real-world probabilities can help identify and address such deviations to ensure the desired performance.
Mike, your article was well-written and informative. I'm curious about the computational requirements when fine-tuning ChatGPT. Is it feasible to fine-tune on modest hardware or limited resources?
Thank you, Sarah! Fine-tuning ChatGPT can be resource-intensive, especially when working with larger models. While fine-tuning on modest hardware or limited resources is possible, it's beneficial to leverage cloud computing or pre-trained models as a starting point to ease computational demands.
Mike, fascinating article! How do you ensure that ChatGPT doesn't generate outputs that sound plausible but are factually incorrect in stochastic modeling?
I'm glad you found it fascinating, Emma! Ensuring ChatGPT's outputs are factually correct requires validation against real-world probabilities, cross-referencing with domain knowledge, and utilizing techniques like adversarial evaluation and data augmentation to enhance validity.
Mike, your article was impressive! In your experience, what are the major benefits of using ChatGPT in stochastic modeling compared to traditional techniques?
Thank you, Jack! ChatGPT brings the advantage of generating realistic and contextually accurate responses, enhancing the exploration of probabilistic spaces. Its ability to understand and generate text naturally makes it a valuable addition to traditional stochastic modeling techniques.
Mike, great article! Are there any concerns related to data privacy or confidentiality when using ChatGPT for stochastic modeling, especially when dealing with sensitive data?
Thanks, Sophie! Data privacy and confidentiality are indeed vital considerations. It's crucial to handle sensitive data responsibly, follow relevant data protection measures, and ensure that ChatGPT's usage aligns with the required privacy and security standards.
Mike, I enjoyed reading your article! How can one ensure that ChatGPT doesn't generate biased or misleading outputs in stochastic modeling tasks?
I'm glad you enjoyed it, Grace! Avoiding biased or misleading outputs requires continuous monitoring, active mitigation of biases in training data, diverse representation among reviewers, and soliciting external perspectives. OpenAI is actively working to improve these aspects in their models.
Mike, your article provided valuable insights into using ChatGPT for stochastic modeling. Are there any limitations in terms of the model's ability to handle rare events or sparse data?
Thank you, Jason! ChatGPT's ability to handle rare events or sparse data is limited by the exposure and representation of such scenarios in the training data. To overcome this limitation, utilizing techniques like data augmentation and active learning can be valuable.
Hi Mike, your article was informative! How do you ensure that the fine-tuned ChatGPT model remains updated and reliable as new data becomes available?
Hi Amy! Keeping the fine-tuned ChatGPT model updated requires continuous evaluation, retraining with relevant and recent data, and incorporating suitable mechanisms to adapt to new patterns and trends. Regularly validating against real-world outcomes helps ensure reliability.
Mike, your article was thought-provoking. Do you have any suggestions for evaluating the performance and accuracy of ChatGPT in stochastic models, apart from real-world validation?
Thank you, Tom! Apart from real-world validation, additional evaluation techniques include comparing against baseline models or expert-generated responses, conducting adversarial testing to identify weaknesses, and leveraging value-at-risk metrics or error analysis for quantitative assessment.
Mike, great article! How can ChatGPT benefit from using large-scale datasets during fine-tuning, and what are the considerations when working with such datasets for stochastic modeling?
Thanks, Sophia! Large-scale datasets during fine-tuning help capture diverse patterns and improve the model's robustness. When using such datasets, it's crucial to ensure data quality, relevance to the domain, and regularly review and curate them for optimal fine-tuning outcomes.
Thank you, Mike! Your article provided a great understanding of integrating ChatGPT into stochastic modeling. We appreciate your prompt responses.
You're welcome, Sophia! I'm glad you found the article helpful. It was my pleasure to engage in these discussions and address your queries.
Mike, your article presented a compelling use case of ChatGPT in stochastic modeling. Can the model also provide explanations or reasoning behind its probabilistic outputs?
Thank you, Emily! Generating explanations or reasoning behind probabilistic outputs is an ongoing area of research. While it may not be a core capability of ChatGPT, techniques like rule-based post-processing or attention visualization can provide some insights into the model's decision-making process.
Mike, great article with valuable insights! When fine-tuning ChatGPT, what are the challenges to consider when dealing with domain-specific jargon or technical terms in stochastic modeling?
Thanks, Anthony! Dealing with domain-specific jargon or technical terms during fine-tuning requires careful domain-specific data curation, exposure to relevant literature and best practices, and working closely with domain experts to ensure accurate representation and understanding of the terminologies.
Mike, your article was very insightful! Can you highlight any potential shortcomings of using ChatGPT in stochastic modeling, especially in highly regulated industries?
I'm glad you found it insightful, Victoria! When working with highly regulated industries, potential shortcomings of ChatGPT can include the need for rigorous validation processes, addressing concerns about privacy and data security, and ensuring compliance with industry-specific regulations, which may pose additional challenges.
Mike, great article! Does ChatGPT support interactions with external databases or other tools commonly used in stochastic modeling workflows?
Thank you, Jacob! ChatGPT can integrate with external databases or tools in stochastic modeling workflows via suitable APIs or data connectors. This allows leveraging additional data sources and tools to enhance the model's functionality and access domain-specific information.
Mike, your article provided excellent insights into using ChatGPT in stochastic modeling. How can we ensure that fine-tuning on limited or domain-specific data leads to reliable performance?
I'm glad you found it excellent, Eleanor! When fine-tuning on limited or domain-specific data, employing techniques like transfer learning from related domains, leveraging pre-trained models as starting points, and iterative validation with real-world data can help ensure reliable performance.
Mike, your article was informative and well-structured. Can ChatGPT handle stochastic modeling tasks involving time series analysis and forecasting effectively?
Thank you, Lucy! While ChatGPT can provide value in time series analysis and forecasting, its effectiveness depends on the availability of training data that captures relevant temporal patterns and the effort put into aligning the model's outputs with established forecasting methods.
Mike, great article! How does one deal with the challenge of reducing ambiguity and incorporating contextual constraints when using ChatGPT for stochastic modeling?
Thanks, Gabriel! Reducing ambiguity and incorporating contextual constraints can be addressed by fine-tuning ChatGPT on task-specific data, employing rule-based post-processing methods, or developing custom prompts or interventions to guide the model's responses within the desired context.
Hi Mike! I enjoyed reading your article on ChatGPT in stochastic modeling. Are there any potential pitfalls or challenges one should be aware of when adopting this approach?
Hi Sophie! Adopting ChatGPT in stochastic modeling comes with challenges, such as ensuring the model doesn't generate incorrect responses, addressing biases, and considering the computational resources required. Regular validation, diverse perspectives, and balancing the model's outputs with other techniques can help mitigate these challenges.
Thank you, Mike! Your article and detailed responses have been immensely helpful in grasping the potential of ChatGPT in stochastic modeling.
You're welcome, Sophie! I'm delighted to hear that the article and responses were helpful to you. If you ever need further assistance or have more discussions, feel free to reach out.
Mike, your article was eye-opening! How do you approach continuous learning and model updating when incorporating ChatGPT into stochastic modeling workflows?
I'm glad you found it eye-opening, Oliver! Continuous learning and model updating involve monitoring the model's performance, regularly integrating new data, and retraining or fine-tuning to capture evolving patterns. It's an iterative process that ensures the model remains up-to-date and aligned with real-world changes.
Mike, your article provided a fresh perspective on using ChatGPT in stochastic modeling. Can you share any tips for effectively fine-tuning the model to specific industries or use cases?
Thank you, Grace! When fine-tuning ChatGPT for specific industries or use cases, it's beneficial to carefully curate the training data, involving domain experts or reviewers, and iterate through fine-tuning with proper evaluation and validation cycles to refine the model's performance specifically for the desired applications.
Thank you all for your engaging discussions and valuable questions! I appreciate your time and insights.
Thank you all for taking the time to read my article on enhancing stochastic modeling with ChatGPT. I'm excited to hear your thoughts and insights!
Great article, Mike! I've been exploring stochastic modeling lately, and it's fascinating to see how AI can enhance it. I'm curious to know which specific applications do you think ChatGPT can have in this field?
Laura Thompson, ChatGPT can have numerous applications in stochastic modeling. For example, it can be used to simulate complex scenarios, estimate probabilities, and generate realistic scenarios for risk analysis. It adds a new level of interactivity to the modeling process.
Hey Mike, thanks for sharing this interesting piece. In your opinion, what are the main advantages of using ChatGPT over other language models for stochastic modeling? I'd love to know your thoughts.
This article was a great read, Mike. I've been using stochastic modeling for financial forecasting. Could ChatGPT help with generating more accurate and flexible financial models?
Absolutely, Nathan! ChatGPT can assist in generating more accurate financial models by incorporating real-time data and allowing you to interactively explore different scenarios. It can provide valuable insights into the potential outcomes of various financial strategies.
Thanks for sharing your knowledge, Mike! I'm relatively new to stochastic modeling, and the potential of incorporating AI into it sounds promising. Are there any limitations to consider when using ChatGPT in this context?
Julia Adams, while ChatGPT is a powerful tool, it also has some limitations. One limitation is that it may generate outputs that seem reasonable but are incorrect or misleading. It's crucial to carefully validate and interpret the generated results. Additionally, it's important to ensure that the training data used for ChatGPT includes a relevant and diverse set of stochastic models.
This article is quite insightful, Mike. I've used other language models for stochastic modeling, but ChatGPT seems more interactive. Do you have any recommendations on how to get started with using ChatGPT for this purpose?
David Smith, I'm glad you found the article insightful. To get started with ChatGPT for stochastic modeling, it's helpful to first familiarize yourself with the model's capabilities and limitations. Then, you can start experimenting with small-scale scenarios and gradually increase complexity. It's recommended to keep the model's outputs in the context of their probabilistic nature to leverage its full potential.
Interesting article, Mike! I'm curious about the size of the training data required for ChatGPT to perform well in stochastic modeling. Is there an optimal amount of data?
Emily Brown, the optimal amount of training data for ChatGPT can depend on various factors such as the complexity of the stochastic models you want to simulate and the level of accuracy you seek. Generally, having a diverse and extensive dataset can improve the model's performance, but in practice, it's often a trade-off between resource requirements and the desired level of fidelity.
Great article, Mike! I'm particularly interested in the real-time applications of ChatGPT in stochastic modeling. Can it handle data streams and adapt its predictions dynamically?
Daniel Green, ChatGPT can indeed be adapted for real-time applications in stochastic modeling. By continuously feeding it with new data, you can help it make predictions and adapt its outputs based on the evolving information. However, it's crucial to carefully design and implement the data streaming process to ensure accuracy and validity.
Thanks for sharing your insights, Mike. It's great to see the potential of AI in enhancing stochastic modeling. Do you think ChatGPT can eventually replace traditional approaches in certain domains?
Sophie Anderson, while ChatGPT can offer valuable enhancements to stochastic modeling, I don't think it can completely replace traditional approaches in all domains. It can be seen as a powerful tool that complements and augments existing methodologies. The expertise and domain knowledge of human experts remain important in interpreting and validating the results generated by the model.
Fascinating article, Mike! As stochastic modeling is often used for risk analysis, do you think ChatGPT can also contribute to risk management in technology-based industries?
Robert Johnson, definitely! ChatGPT can play a significant role in risk management for technology-based industries. By generating realistic scenarios and simulating various outcomes, it can help identify potential risks and evaluate the effectiveness of risk mitigation strategies. It adds an interactivity aspect that can enhance decision-making in risk management processes.
Thanks for the insightful article, Mike. I'm wondering if there are any ethical considerations when using AI like ChatGPT in stochastic modeling?
Chris Thompson, ethical considerations are indeed important when using AI in any context, including stochastic modeling. It's crucial to ensure the responsible use of AI and consider potential biases or unfair consequences that may arise from the generated outputs. Transparency, accountability, and ethical guidelines should be followed to mitigate such issues.
Great article, Mike! I'm wondering if there are any online resources or tutorials you would recommend to learn more about incorporating ChatGPT into stochastic modeling?
Amy Wilson, there are several online resources available to learn more about incorporating ChatGPT into stochastic modeling. OpenAI's website provides documentation, tutorials, and examples that can help you get started. Additionally, there are various online communities and forums where practitioners share their experiences and insights. Don't hesitate to explore and leverage these resources.
Interesting read, Mike! Are there any known challenges or limitations when it comes to training ChatGPT for stochastic modeling? How can we address them?
William Davis, training ChatGPT for stochastic modeling can present a few challenges. One notable challenge is acquiring diverse and relevant training data that covers a broad range of stochastic models. It's crucial to carefully curate and preprocess the data to ensure its usefulness. Additionally, addressing the model's limitations and biases through fine-tuning and ongoing validation can help mitigate their impact.
Thanks, Mike, for sharing this article. I was wondering if ChatGPT can handle data with different levels of uncertainty in stochastic modeling?
Olivia Brown, ChatGPT can handle data with different levels of uncertainty in stochastic modeling. By considering probabilistic distributions and incorporating uncertainty measures, it can generate outputs that account for the varying levels of uncertainty associated with the input data. This flexibility can be valuable when dealing with stochastic models.
Great article, Mike! What are the key factors to consider when deciding whether to use ChatGPT or traditional models for stochastic modeling?
Sophia Phillips, the choice between ChatGPT and traditional models for stochastic modeling depends on various factors. Some key considerations include the specific requirements of the problem, complexity of the stochastic models, availability of relevant training data, and the desired level of interactivity and flexibility. It's crucial to evaluate these factors to determine the most suitable approach for a given scenario.
Thanks for this informative article, Mike. I'm curious about the computational resources required to make use of ChatGPT effectively in stochastic modeling. Are there any resource constraints to be aware of?
Daniel Cooper, ChatGPT can indeed have computational resource requirements. Training the model and utilizing it for complex stochastic modeling tasks can demand substantial computational power and time. It's important to assess the available resources, such as CPU/GPU capabilities, memory, and running time, to ensure a feasible and efficient application of ChatGPT in stochastic modeling workflows.
Really enjoyed this article, Mike! As ChatGPT offers interactivity, I'm curious if it can also help identify potential data anomalies or outliers during the modeling process.
Emma Wilson, ChatGPT's interactivity can indeed be leveraged to identify potential data anomalies or outliers. By generating simulated scenarios, it becomes easier to notice unexpected patterns or outliers that can help guide the data analysis and identify potential issues or areas for further investigation.
Thank you for sharing your expertise, Mike! I'm interested in understanding how ChatGPT handles the exploration-exploitation trade-off when it comes to stochastic modeling.
Lucas Martin, ChatGPT can be used to balance the exploration-exploitation trade-off in stochastic modeling by considering different scenarios and iteratively adjusting the model's inputs or parameters based on the generated outputs. By exploring multiple paths, you can exploit the valuable insights provided by the model and make informed decisions while accounting for the inherent randomness and uncertainty of stochastic models.
This article was a great read, Mike. Could you provide an example of how ChatGPT can enhance stochastic modeling in technology-driven industries?
Sophie Turner, certainly! In technology-driven industries, ChatGPT can assist in stochastic modeling for various purposes. For example, it can be used to predict failure rates of components in a system, optimize resource allocation based on probabilistic demand forecasts, or simulate the behavior of network traffic under different conditions. These applications leverage ChatGPT's ability to generate insights and simulate realistic scenarios.
Hey Mike, great article! I'm wondering if ChatGPT can help address issues like overfitting or bias that may arise during the development of stochastic models.
Ethan Adams, ChatGPT can indeed help address issues like overfitting or bias in stochastic modeling. By providing alternative perspectives and generating diverse scenarios, it can mitigate the risk of over-reliance on specific patterns or biased data. However, it's crucial to carefully validate and interpret the model's outputs, especially when it comes to sensitive or critical applications.
Thanks for sharing this informative article, Mike. I'm curious if there are any ongoing research efforts to further improve the integration of AI, like ChatGPT, with stochastic modeling.
Liam Roberts, there are indeed ongoing research efforts to enhance the integration of AI, including ChatGPT, with stochastic modeling. Researchers are continuously working on improving the model's capabilities, addressing limitations, and exploring new techniques to enable more efficient and accurate stochastic modeling processes. It's an exciting area of development!