Enhancing System Monitoring with ChatGPT: Revolutionizing Hardware Health Monitoring
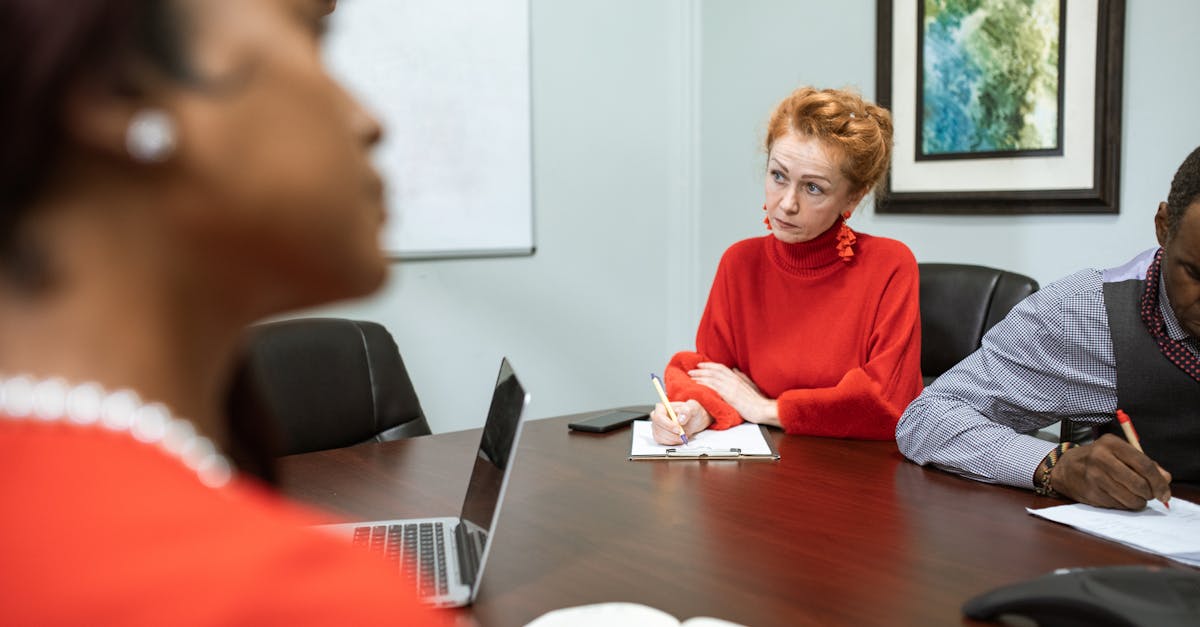
In today's world, where technology plays a crucial role in almost every aspect of our lives, maintaining the health and functionality of our systems and hardware is of utmost importance. System monitoring and hardware health monitoring are the key practices that help us ensure the smooth operation of our devices and prevent unexpected failures.
One of the latest advancements in system monitoring is the use of machine learning algorithms to predict hardware failures. This has been made possible by incorporating artificial intelligence into monitoring tools like ChatGPT-4, which can analyze data trends to identify potential hardware issues before they turn into critical failures.
Hardware health monitoring involves constantly monitoring various parameters like temperature, voltage, fan speed, and many others to evaluate the overall health of hardware components. Traditionally, system administrators and IT professionals relied on manual inspection and periodic checks to identify potential hardware failures. However, this approach had limitations, as some issues were difficult to detect or required continuous monitoring.
With the advent of machine learning algorithms, hardware health monitoring has become more efficient and reliable. By analyzing historical data trends and patterns, machine learning models can learn to identify anomalies or deviations that may indicate an impending hardware failure. This proactive approach allows for preventive actions to be taken, reducing the risk of catastrophic system failures and minimizing downtime.
ChatGPT-4, powered by machine learning, can process large volumes of data generated by hardware sensors and devices to predict potential failures. It can analyze the relationships between different variables and detect patterns that may lead to hardware malfunctions. By providing real-time insights and alerts, ChatGPT-4 empowers system administrators and IT professionals to take proactive measures, such as replacing faulty components or performing maintenance tasks, before failures occur.
The usage of machine learning in hardware health monitoring has revolutionized the way we approach system maintenance and increased the reliability and performance of our devices. By leveraging predictive analytics, we can ensure the longevity and optimal functioning of our systems, avoiding costly downtime and improving user experience.
In conclusion, system monitoring and hardware health monitoring are essential practices to maintain the efficiency and reliability of our devices. With the integration of machine learning algorithms, tools like ChatGPT-4 have the capability to predict hardware failures by analyzing data trends. This proactive approach enables system administrators and IT professionals to take timely actions, reducing the impact of potential failures and ensuring uninterrupted system operation.
For more information on system monitoring and hardware health monitoring, visit www.example.com.
Comments:
Great article! I can see how ChatGPT can greatly improve system monitoring by providing real-time insights into hardware health.
I agree, Michael. It's amazing to see how AI technology like ChatGPT is being utilized to enhance system monitoring and diagnostics.
Thank you both for your kind words! I'm glad you recognize the potential of ChatGPT in revolutionizing hardware health monitoring.
This is fascinating! The ability to leverage AI for real-time hardware health monitoring could prevent costly breakdowns and improve overall system reliability.
Absolutely, Robert! Proactive monitoring can minimize downtime and improve maintenance efforts. I'm excited to see how this technology progresses in the future.
I can see the potential benefits, but what about the risks associated with relying on AI for critical system monitoring? How do we ensure the reliability and accuracy of the data provided?
Valid concerns, Nikhil. While AI technologies like ChatGPT can greatly enhance monitoring, it's crucial to have robust validation processes, regular model updates, and human oversight to ensure accuracy and reliability.
That's a great point, Nikhil. Implementing fail-safes and redundancy measures will be essential to mitigate any risks associated with AI-driven hardware health monitoring.
Indeed, Olivia. AI should augment human expertise rather than completely replace it. Human intervention and critical thinking play a vital role in maintaining system integrity.
I can imagine ChatGPT facilitating more efficient troubleshooting processes. It could save a lot of time by quickly identifying the root causes of hardware issues.
Absolutely, Samantha. With a well-trained AI system like ChatGPT, system administrators can gain valuable insights and resolve issues faster.
While this sounds promising, I wonder how accessible this technology will be for small businesses with limited resources.
Valid concern, Emily. As AI technology continues to evolve, we hope to see more cost-effective solutions that cater to businesses of all sizes.
I think it's only a matter of time before AI-driven monitoring becomes the standard in the industry. The benefits it brings are too significant to ignore.
I agree, Robert. Companies that embrace this technology will gain a competitive edge by maintaining reliable systems and minimizing downtime.
I have concerns about the potential job displacement due to automation. Will AI monitoring systems replace human roles in the future?
I understand your concern, Sara. While AI monitoring systems can automate certain tasks, human expertise will still be crucial for decision-making and complex problem-solving.
Well said, Olivia. AI should be seen as a tool to support and enhance human capabilities, rather than a replacement for skilled professionals.
I wonder how ChatGPT specifically handles anomalies in hardware health monitoring. Can it adapt to new or unusual patterns and alert system administrators?
That's a great question, Michael. ChatGPT can adapt to new patterns with continual training, but it's important to establish robust anomaly detection mechanisms to ensure accurate alerts.
The potential here is immense! I can imagine a future where AI-driven monitoring enables predictive maintenance, preventing system failures before they even occur.
Absolutely, Daniel! By analyzing data and identifying early warning signs, AI monitoring can significantly reduce costly downtime and improve overall system reliability.
Wouldn't the implementation of ChatGPT for system monitoring require significant computational resources? How would that impact its accessibility?
Valid concern, Emily. While AI models can be resource-intensive, advancements in cloud computing and edge computing are making them increasingly accessible and cost-effective.
I'm curious about the limitations of ChatGPT in hardware health monitoring. Are there specific scenarios where it may not be as effective or accurate?
Great question, Sara. ChatGPT's effectiveness can depend on the quality and diversity of the training data. In complex scenarios or rare edge cases, it may benefit from additional training or human intervention.
The ethical considerations surrounding AI monitoring are crucial. How do we ensure data privacy and prevent any misuse of sensitive information?
Excellent point, Emma. Data privacy should be a top priority. Implementing strict security measures, anonymizing sensitive data, and obtaining user consent are essential to address these concerns.
What happens when ChatGPT encounters a hardware issue it has never been trained on? Could it still provide useful insights or would it fail to respond appropriately?
That's an interesting question, John. While ChatGPT may not have specific training for a rare issue, it can still offer insights based on its understanding of similar cases. However, human expertise should also be sought for unfamiliar problems.
ChatGPT seems promising, but have any real-world implementations successfully utilized this technology for hardware health monitoring?
Great question, Jessica. While ChatGPT is relatively new, there have been successful pilot projects where it has demonstrated its value in enhancing system monitoring, detecting anomalies, and providing real-time insights.
I'm excited about the potential benefits of AI-driven hardware health monitoring. How soon do you envision widespread adoption of this technology?
It's hard to predict the exact timeline, Brian. However, as AI technology evolves, becomes more accessible, and organizations witness its value, we can expect to see accelerated adoption in the coming years.
I have concerns about the reliance on AI for critical system monitoring. What if the AI system malfunctions or provides inaccurate information?
Valid concerns, Mary. Implementing thorough testing, validation, and continuous monitoring of AI systems can help mitigate the risks associated with malfunctions or inaccurate outputs.
ChatGPT sounds promising, but will it be compatible with existing monitoring tools and infrastructure, or would it require a complete overhaul?
Good question, David. Ideally, AI systems like ChatGPT should be designed to integrate seamlessly with existing tools and infrastructure to facilitate a smooth transition without a complete overhaul.
How customizable is ChatGPT? Can it be tailored to different hardware configurations and monitoring requirements?
Customizability is an important aspect, Emily. ChatGPT can be fine-tuned and trained on specific hardware configurations and requirements to ensure its effectiveness in various monitoring scenarios.
I can't help but wonder about the power consumption of AI-driven monitoring systems. Could it lead to increased energy consumption?
Good point, Oliver. AI systems do require computational resources, but with optimization techniques and advancements in hardware efficiency, efforts can be made to minimize any potential increase in energy consumption.
The role of interpretability is crucial in AI systems. How can we ensure transparency and understand the reasoning behind ChatGPT's monitoring insights?
You're absolutely right, Sophia. Ensuring interpretability is important. Techniques like explainable AI and model explanation frameworks can provide insights into ChatGPT's decision-making and promote transparency.
One concern I have is the potential bias in AI models. How do we ensure that hardware health monitoring systems powered by ChatGPT don't exhibit biased behavior?
Valid concern, Robert. Bias detection and mitigation techniques, diverse training data sources, and inclusive development practices can help minimize the risk of biased behavior in AI systems.
Will organizations need to undergo significant training or hire AI experts to effectively implement ChatGPT for hardware health monitoring?
Training or hiring AI experts can be beneficial, Nikhil, especially during the initial stages of implementation. However, user-friendly tools and vendor support can also aid organizations in effectively utilizing ChatGPT without extensive training requirements.
I'm curious about the potential cost savings associated with AI-driven hardware health monitoring. Has any research focused on quantifying the financial benefits?
Excellent question, Daniel. While research is ongoing, preliminary studies have shown that AI-driven monitoring can lead to significant cost savings by minimizing system failures, reducing downtime, and optimizing maintenance efforts.