Enhancing Technological 'Refrigeration' with Gemini: An Innovative Approach to Optimize Performance and Efficiency
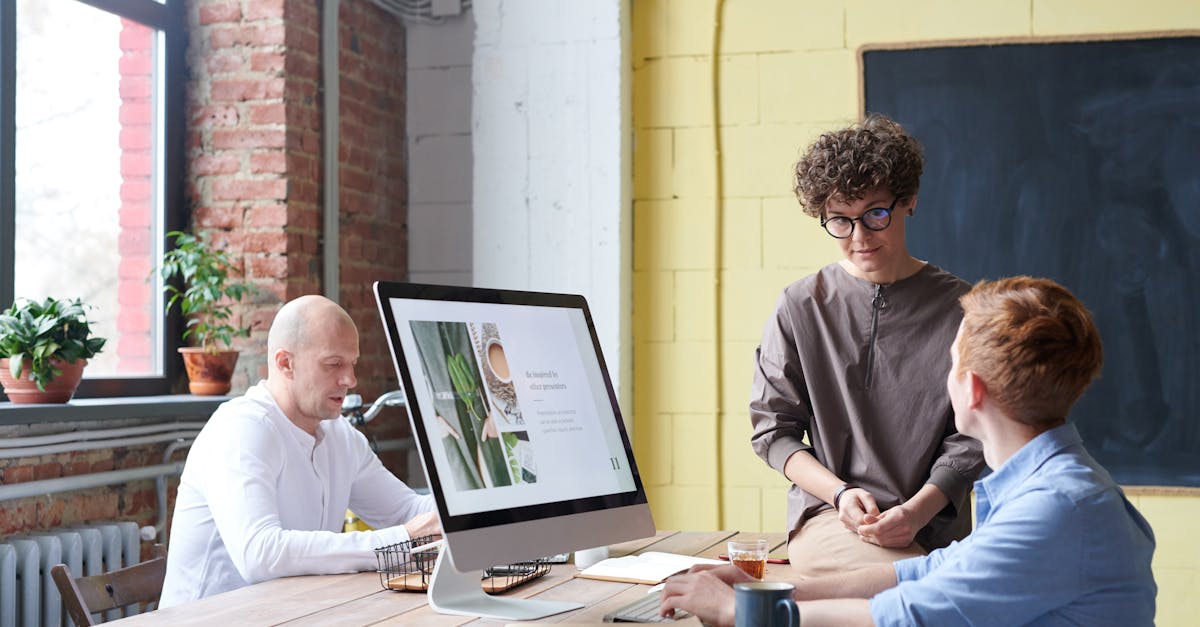
In today's fast-paced world, technology plays a crucial role in every aspect of our lives. From communication to transportation, technological advancements have transformed various industries. One such industry is refrigeration, which has seen significant improvements over the years. In this article, we explore how the integration of Gemini, an advanced language model, can enhance the performance and efficiency of refrigeration systems.
Technology at the Forefront
Refrigeration technology has come a long way since its inception. Traditional refrigeration systems have been effective in preserving perishable goods and maintaining low temperatures. However, there is always room for improvement. This is where Gemini comes in.
Gemini is an advanced language model developed by Google. Leveraging the power of deep learning and natural language understanding, Gemini can process and generate human-like responses based on input prompts. By utilizing Gemini's capabilities, we can optimize the control algorithms of refrigeration systems.
Optimizing Performance
Refrigeration systems rely on complex algorithms to determine the optimal setpoints for temperature, humidity, and other parameters. These algorithms are designed to maintain a stable and consistent environment within the refrigeration unit. However, traditional algorithms may not be capable of adapting to changing external factors or accurately predicting future conditions.
By integrating Gemini into the control system, refrigeration units can benefit from its ability to process large amounts of data and make intelligent predictions. Gemini can analyze historical data of temperature fluctuations, energy consumption, and environmental conditions to generate more precise control settings. This optimization leads to better performance in maintaining the ideal storage conditions for perishable goods.
Increasing Efficiency
In addition to optimizing performance, Gemini can aid in improving the overall efficiency of refrigeration systems. Energy consumption is a significant concern in refrigeration, and even small improvements in efficiency can result in substantial cost savings and reduced environmental impact.
Traditional refrigeration control systems often work on pre-determined setpoints that may not account for variations due to seasonality or changing energy costs. With Gemini, refrigeration units can adapt to real-time energy pricing, external environmental factors, and storage requirements. By dynamically adjusting the control parameters, energy consumption can be minimized without compromising the integrity of the stored goods.
Conclusion
Gemini brings a new dimension to the field of refrigeration technology. By integrating this advanced language model into control algorithms, refrigeration systems can benefit from optimized performance and increased efficiency. The ability to process vast amounts of data and make intelligent decisions allows for superior temperature control and energy management. As technology continues to advance, leveraging the power of AI models like Gemini will undoubtedly revolutionize the refrigeration industry.
Comments:
Thank you all for taking the time to read my article on enhancing technological 'refrigeration' with Gemini! I'm excited to hear your thoughts and answer any questions you may have.
This is a fascinating approach! I can see how incorporating Gemini into refrigeration systems can lead to improved performance and efficiency. The potential for real-time analysis and adjustments is intriguing. Great article, Katrina!
I agree with Jennifer, Katrina. Your article highlights the tremendous possibilities that emerge from combining AI with refrigeration technology. It seems like Gemini has the potential to revolutionize the industry.
I have some reservations about relying solely on AI for refrigeration optimization. What if there are malfunctions or errors in the Gemini system? How do we ensure the reliability and safety of such systems?
Good question, Samantha! While AI systems like Gemini can greatly enhance performance, it's crucial to have fail-safe mechanisms in place. These systems should always be monitored, and traditional control mechanisms should act as safety nets. AI should be seen as a tool that, when combined with human expertise, can achieve even greater results.
As with any new technology, there are risks and challenges. But I believe that with proper testing, validation, and oversight, the benefits of AI-based refrigeration optimization outweigh the potential downsides. We should explore this approach further!
I'm curious about the energy efficiency improvements that Gemini can bring to refrigeration systems. Can it help reduce electricity consumption and lower environmental impact?
Absolutely, Linda! By using AI-driven optimization, Gemini can analyze various factors like temperature, humidity, and system performance to achieve energy savings. It enables more precise control and adaptive adjustments, ultimately leading to reduced electricity consumption and a smaller carbon footprint. It's a win-win!
I'm concerned about the potential cost of implementing AI-powered 'refrigeration' solutions. Won't it require significant investments and modifications to existing systems?
Valid point, Michael. Implementing AI-powered optimization does involve upfront costs, but it's important to consider the long-term benefits. The improved efficiency and energy savings can result in reduced operational costs over time. Plus, as AI technology advances, the costs of implementation are likely to decrease. It's an investment in the future of refrigeration!
I love the idea of leveraging AI for 'refrigeration' optimization. It's clear that manual adjustments and traditional control methods have limitations. By combining technology and human expertise, we have the potential to create highly efficient refrigeration systems. Well done, Katrina, on shedding light on this innovative approach!
Thank you, Sophia! I'm glad you appreciate the potential of this approach. It's an exciting time for the refrigeration industry, as we explore ways to push the boundaries of efficiency and performance.
I wonder if Gemini has any limitations when it comes to complex refrigeration systems. Can it handle multiple variables and optimization objectives? Or are there situations where traditional control methods are still preferable?
Great question, Christopher. While Gemini can handle multiple variables and objectives, there may be cases where highly complex systems require a combination of AI and traditional control methods. It's important to assess the specific needs and optimize the approach accordingly. There's no one-size-fits-all solution, but Gemini offers valuable capabilities in many scenarios.
Katrina, could you provide an example of how Gemini could be applied practically in a refrigeration system? I'm having trouble picturing it in action.
Sure thing, Jason! Let's say we have a large cold storage facility. Gemini can continuously monitor temperature, humidity, and other key factors while taking into account varying external conditions. It can then provide real-time recommendations on adjusting cooling systems to achieve optimal performance and energy efficiency. It's like having an AI-assistant dedicated to maintaining the perfect refrigeration conditions.
Katrina, have there been any real-world implementations of Gemini in refrigeration systems? I'm curious to know if there are any success stories or practical results.
Great question, Emily! While Gemini is a relatively new technology, pilot implementations have shown promising results in smaller-scale refrigeration systems. The potential benefits in terms of energy savings and improved performance have been observed. It's still an emerging field, so we can expect more real-world implementations and success stories in the future.
Is privacy a concern when it comes to using Gemini for refrigeration optimization? Are there any risks associated with data collection and analysis?
Privacy is an important consideration when implementing AI technologies. It's crucial to ensure that any data collected and analyzed is done so in compliance with privacy regulations and best practices. Stricter data anonymization and secure storage methods can be used to mitigate these risks. The focus should always be on maintaining user and system privacy while harnessing the benefits of AI optimization.
Katrina, what are some of the potential challenges and roadblocks to widespread adoption of AI-driven refrigeration optimization?
Good question, Robert. One challenge is the integration of AI systems into existing infrastructure. Retrofitting or upgrading refrigeration systems to accommodate AI optimization can require investment and planning. Another challenge is gaining trust and acceptance from industry professionals who may be hesitant to rely on AI for critical tasks. Addressing these challenges will be crucial for the widespread adoption of AI-driven refrigeration optimization.
I love the concept, Katrina! Optimizing refrigeration systems with AI sounds like a game-changer. The potential for increased efficiency and reduced environmental impact is remarkable. Looking forward to seeing this technology evolve!
Thank you, Amy! I'm truly excited about the possibilities this technology holds. It's heartening to see so many others interested and supportive of AI-driven 'refrigeration' optimization.
What are some of the other applications where Gemini or similar AI systems can optimize performance and efficiency?
Great question, Elizabeth! Gemini can be applied to various domains where performance and efficiency are crucial. Examples include HVAC systems, energy management, industrial processes, and even transportation logistics, among others. The possibilities are vast, and optimizing operations with AI is a promising frontier across multiple industries.
I'm curious about the scalability of AI-driven optimization. Can Gemini handle large-scale refrigeration facilities, or are there limitations to its effectiveness in such scenarios?
Scalability is an important consideration, Thomas. While Gemini has shown promise in smaller-scale implementations, its effectiveness in larger facilities may have limitations. However, advancements in AI technology are rapid, and we can anticipate improvements that will enable more efficient and effective optimization of larger-scale refrigeration systems in the future.
Could Gemini be combined with predictive maintenance techniques to optimize refrigeration systems proactively?
Absolutely, Hannah! Combining Gemini with predictive maintenance techniques can enhance the overall efficiency and reliability of refrigeration systems. By proactively analyzing system data and making real-time recommendations, potential issues can be identified and addressed before they escalate, minimizing downtime and optimizing maintenance schedules.
I'm concerned about the potential impact on jobs. Could AI-driven optimization in the refrigeration industry lead to job displacements?
Job displacement is a valid concern, Emma. While AI optimization may change the nature of certain job roles, it also creates new opportunities. For example, skilled professionals will be needed to develop, implement, and monitor these AI-driven systems. Moreover, by improving efficiency and reducing operational costs, AI can potentially lead to industry growth and the creation of new jobs. It's important to approach these technological advancements with a focus on reskilling and adapting to change.
Katrina, great article! I'm curious about the potential impact of AI-driven 'refrigeration' optimization on food quality and safety. How can Gemini help in maintaining optimal conditions for perishable goods?
Thank you, Oliver! Gemini can play a vital role in maintaining optimal conditions for perishable goods. By continuously monitoring and analyzing various factors like temperature, humidity, and storage conditions, it can provide recommendations to prevent spoilage, extend shelf life, and mitigate the risk of bacterial growth. AI-driven optimization helps ensure that food quality and safety standards are maintained throughout the supply chain.
I agree with the potential benefits of AI optimization, but I'm concerned about the learning and adaptation process. How does Gemini handle changes in the refrigeration system or the environment to continuously optimize performance?
Great question, Chloe. Gemini can learn and adapt by continuously analyzing data and receiving feedback based on system performance. When changes occur in the refrigeration system or the environment, the system can incorporate these new variables and adjust its optimization recommendations accordingly. It's an iterative process where the AI system becomes more accurate and effective over time.
Katrina, what kind of data is required for Gemini to optimize refrigeration systems effectively? Are there any specific data collection requirements or challenges?
Data collection is crucial for effective optimization, Daniel. Ideally, the AI system would require data on refrigeration system variables (e.g., temperature, humidity), external factors (e.g., weather conditions), and performance indicators. Challenges may include ensuring data accuracy, reliability, and consistent availability. Accessing, organizing, and integrating these diverse data sources is an important aspect of implementing AI-driven 'refrigeration' optimization.
Katrina, have there been any studies comparing the performance and efficiency of refrigeration systems with and without AI-driven optimization?
Yes, Rachel! Comparative studies have been conducted, and they demonstrate significant improvements in performance and efficiency when AI-driven optimization is employed. While each system and its specific configuration may yield variable results, the overall trend shows that AI optimization can lead to energy savings, reduced costs, and improved system reliability. These studies serve as valuable proof points for the potential benefits of using Gemini in refrigeration systems.
Katrina, what do you foresee as the future of 'refrigeration' optimization? Any exciting developments or trends on the horizon?
Great question, Justin! The future of refrigeration optimization lies in a combination of AI technologies, big data, and advanced control systems. We can expect more sophisticated AI models tailored for specific refrigeration applications, increased integration with IoT devices, and enhanced adaptability to changing environmental conditions. Additionally, the development of standardized protocols and industry regulations will play a role in shaping the future of AI-driven 'refrigeration' optimization.
What kind of computational resources would be needed to implement Gemini for refrigeration optimization? Does it require high computing power?
Good question, Sarah! The computational resources required for Gemini implementation can vary depending on the scale of the refrigeration system and the complexity of optimization objectives. While it benefits from high computing power, recent advancements have allowed for more efficient models that can run on various hardware configurations. As AI technology progresses, we can expect optimized solutions that balance computational demands with practical implementation.
Katrina, what are some potential risks of relying heavily on AI for refrigeration optimization? Are there any concerns we should be aware of?
Excellent question, Jason. One risk is over-reliance on AI without proper human oversight. Technical failures or errors can occur, highlighting the importance of fallback mechanisms and continuous monitoring. Data security and privacy concerns we discussed earlier also need to be addressed. Ultimately, finding the right balance between human expertise and AI-driven optimization is key to mitigating these risks and maximizing the benefits.
Katrina, could Gemini be used to optimize refrigeration systems retroactively, analyzing past data to identify opportunities for improvement?
Certainly, Grace! Gemini could be leveraged to analyze historical data from refrigeration systems and identify patterns, inefficiencies, and opportunities for optimization. By learning from past data, AI models can provide recommendations for adjustments that would have resulted in improved performance. This is a valuable approach to retroactively optimize refrigeration systems and learn from historical experiences.
The potential benefits of AI-driven 'refrigeration' optimization are impressive. But are there any regulatory or legal challenges in implementing such systems? What kind of standards or guidelines would be necessary?
Regulation and standardization are vital components of implementing AI-driven optimization, Lucas. Clear guidelines should be established to ensure system safety, reliability, and compliance with privacy regulations. Regulatory bodies and industry associations should collaborate to define these standards, addressing ethical considerations, data privacy, and system performance. Having a framework that aligns technological advancements with legal and ethical requirements will facilitate safe and responsible implementation.
Thank you all for your interest in my article on enhancing technological refrigeration with Gemini! I'm excited to discuss this innovative approach with you.
This is a fascinating topic, Katrina. I can definitely see how Gemini can optimize the performance and efficiency of refrigeration systems. Do you have any real-world examples where this approach has been successfully implemented?
Great question, Mark! While Gemini is a relatively new technology, there have been successful implementations in large-scale cold storage warehouses. They used Gemini to analyze real-time data from refrigeration systems and make predictive maintenance suggestions, resulting in significant energy savings and improved equipment performance.
That's impressive, Katrina! I'm curious about the accuracy of the predictions made by Gemini. How reliable is the system in identifying potential issues and suggesting maintenance actions?
Hi Jennifer! Gemini has shown impressive accuracy in its predictions. The system is trained on vast amounts of data and uses advanced machine learning techniques to identify patterns and anomalies. However, it's important to note that it's still an evolving technology, and continuous human monitoring and validation are necessary to ensure optimal results.
Katrina, do you think the implementation of Gemini in refrigeration systems might lead to job losses in the industry? How would you address these concerns?
Valid concern, Michael. While Gemini can automate certain monitoring and maintenance tasks, it is more intended to assist human operators rather than replace them. By freeing up time spent on manual tasks, operators can focus on higher-value activities, such as equipment optimization and process improvements. It's all about finding the right balance between automation and human expertise.
I agree with Katrina. Gemini can be a valuable tool for professionals in the refrigeration industry. It can provide insights and recommendations that improve overall system performance. It's all about leveraging technology to enhance our capabilities rather than viewing it as a threat to job security.
I have some concerns regarding data privacy and security with this approach. How is the data collected by Gemini handled, and what measures are in place to protect sensitive information?
John, data privacy and security are of utmost importance. When implementing Gemini, it's crucial to follow best practices and strict protocols to safeguard sensitive information. Data encryption, access controls, and compliance with privacy regulations are key considerations. Additionally, it's recommended to anonymize and aggregate data whenever possible to protect individual user privacy.
Katrina, I wonder if there are any limitations or challenges associated with using Gemini in refrigeration systems. Could you shed some light on that?
Absolutely, Emma. One limitation of Gemini is its reliance on training data. If the training data doesn't adequately represent all possible scenarios, the system may struggle to provide accurate predictions. Additionally, Gemini may struggle with understanding ambiguous queries or unusual situations that the training data hasn't covered. Ongoing monitoring and close collaboration with domain experts are necessary to overcome these challenges.
Hi Katrina, what are the potential cost savings that can be achieved by implementing Gemini in refrigeration systems? Is it worth the investment?
Hi Lisa! The potential cost savings can be significant. By optimizing equipment performance, reducing energy consumption, and minimizing downtime through predictive maintenance, businesses can achieve substantial savings in operational and maintenance costs. However, the exact return on investment would depend on factors such as the size of the refrigeration system, energy rates, and the specific implementation approach.
Katrina, I have concerns about the accessibility of this technology for smaller businesses. Is chatLLM only suitable for large-scale refrigeration systems, or can it be adapted for smaller setups as well?
Good question, Phillip. While large-scale systems are more likely to have the resources to implement Gemini extensively, the technology can still be adaptable for smaller setups. It may require customization and integration with existing monitoring systems, but the potential benefits, such as improved performance and efficiency, can still be achieved on a smaller scale. It's important to assess the specific needs and constraints of each business.
Katrina, thanks for shedding light on this innovative approach. I can see the potential of Gemini in revolutionizing refrigeration systems. How do you envision the future of this technology in the industry?
You're welcome, Daniel! I believe the future of Gemini in the refrigeration industry is promising. As the technology advances, we can expect increased accuracy, efficiency, and customization. The integration of artificial intelligence and machine learning algorithms with human expertise will lead to more intelligent and optimized refrigeration systems, positively impacting energy consumption, cost savings, and overall sustainability.
Katrina, do you see any potential risks or downsides to implementing Gemini in refrigeration systems?
Hi Liam! While the benefits of Gemini implementation are significant, there are potential risks to be considered. Reliance on AI systems introduces the risk of false positives/negatives, which could affect decision-making. Additionally, proper system training and data validation are essential to ensure accuracy and avoid biases. On the technical side, system failures or vulnerabilities could pose security risks. It's crucial to address these concerns through continuous monitoring, refining algorithms, and following best practices for deployment.
Katrina, I find this integration of AI and refrigeration systems truly exciting. Are there any other potential applications where Gemini can optimize performance and efficiency?
Certainly, Sophia! Gemini has a wide range of potential applications beyond refrigeration. Industries such as manufacturing, energy, logistics, and healthcare can benefit from optimizing performance and efficiency through AI-driven insights and predictive maintenance. By leveraging similar approaches, businesses can unlock new opportunities for cost savings, improved productivity, and sustainability.
Katrina, what are the challenges of implementing Gemini in an existing refrigeration system? Are there any compatibility issues to consider?
Hi Amy! One of the challenges is the compatibility and integration of Gemini with existing monitoring and control systems. It may require adapting data formats, communication protocols, or even hardware upgrades to ensure seamless integration. The availability and quality of historical data for training can also be a factor to consider. Overall, careful planning and collaboration with technical experts are necessary during the implementation phase.
Katrina, I'm curious if Gemini could also help optimize energy consumption in household refrigerators? It would be great if we could improve efficiency at a smaller scale as well.
That's an interesting idea, Oliver! While the current focus has been on large-scale refrigeration systems, there is potential for similar applications in household refrigerators. By analyzing data from individual units, Gemini could provide tips on energy-saving behaviors, like adjusting temperature settings or suggesting optimal usage patterns. To make it more accessible, however, the challenge would be developing a cost-effective solution that can be easily integrated into existing appliances.
Katrina, thank you for sharing your expertise on this topic. I believe Gemini has the potential to transform refrigeration systems and drive efficiency. It's exciting to see how AI continues to revolutionize various industries!
You're welcome, Emily! I'm glad you found the discussion valuable. AI is indeed transforming multiple sectors, and its integration with refrigeration systems opens up new possibilities for improved performance and sustainability. Thank you all for your insightful comments and questions!
This idea of optimizing refrigeration with Gemini is truly innovative! I can't wait to see the impact it will have on energy efficiency and cost savings in the industry.
Katrina, what kind of training data is needed to teach Gemini about refrigeration systems? Is it a complex and time-consuming process?
Hi Jessica! The training process involves feeding Gemini with a large and diverse dataset of refrigeration system data, including sensor readings, maintenance logs, and historical performance. While obtaining and preparing the training data can be time-consuming, the actual training itself is handled by powerful computing systems. The complex machine learning algorithms learn to recognize patterns and make predictions based on the provided data. It requires expertise in AI and domain knowledge collaboration to ensure the best possible training outcomes.
Katrina, have there been any studies or research papers published on the application of Gemini in refrigeration systems? I'd be interested in exploring the results and findings in more detail.
Absolutely, Sophie! There have been research studies and papers published on the topic. I can share some references with you after this discussion. It's a rapidly evolving field, and these studies provide valuable insights into the implementation, challenges, and benefits of integrating Gemini with refrigeration systems.
Katrina, could Gemini potentially improve the response time to malfunctions or system failures in refrigeration systems? It would be crucial to minimize downtime in such cases.
Definitely, Andrew. Gemini's ability to analyze real-time data and identify anomalies enables it to detect malfunctions or deviations from normal system behavior promptly. By providing early warnings and specific guidance, it helps minimize downtime and allows for faster response and resolution. Proactive maintenance based on predictive insights can significantly reduce the impact of system failures and increase overall uptime.
Katrina, how does Gemini handle situations where the training data is limited or unavailable? Can it still provide accurate recommendations?
Hi Ethan! While limited or unavailable training data can pose challenges, Gemini's generalization capability allows it to extrapolate from the available data to a certain extent. However, the accuracy of recommendations in such cases may be lower compared to situations with robust training data. It emphasizes the importance of continuously updating and expanding the training dataset to cover various scenarios, ideally in collaboration with industry experts, to ensure more reliable predictions.
Katrina, what kind of infrastructure is required to implement Gemini in a refrigeration system? Are there any hardware or software prerequisites that businesses need to consider?
Good question, Benjamin. Implementing Gemini in a refrigeration system would typically require a robust computing infrastructure capable of handling the computational demands of the machine learning algorithms. Businesses should also consider the compatibility of their existing monitoring and control systems with Gemini, which may require additional software integration or hardware upgrades. It's essential to assess the technical requirements and consult with experts to ensure a smooth and effective implementation.
Thank you all for your engaging comments and questions! It has been a pleasure discussing the potential benefits and considerations of using Gemini to optimize refrigeration systems. If you have any further inquiries or would like to explore this topic in more detail, feel free to reach out to me. Have a great day!