Enhancing the RDF Landscape: Leveraging ChatGPT for Advanced Technological Interactions
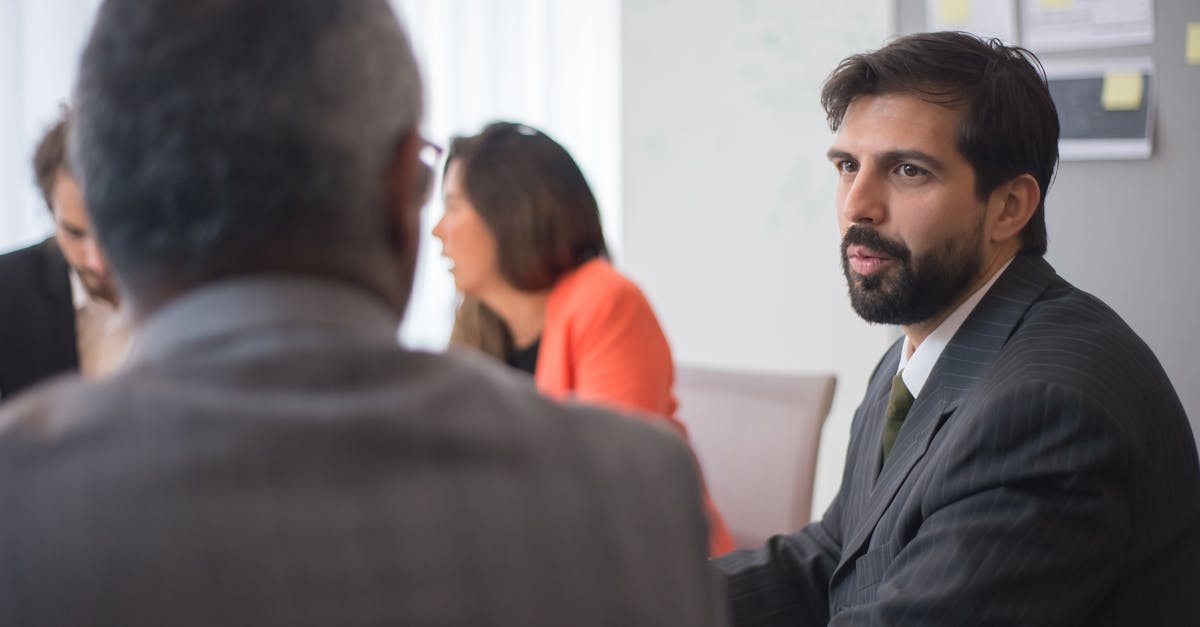
The world of technology is evolving at a fast pace, and so are the ways we extract and analyze data. One such revolutionary technology is the Resource Description Framework (RDF). It plays an essential role in data mining, particularly in the analysis of chatbots like GPT-4. The combination of RDF technology, data mining methods, and the potential of GPT-4 can work wonders to unleash meaningful insights hidden within data.
Understanding RDF in Data Mining
RDF, an acronym for Resource Description Framework, is a foundation for processing metadata. It provides interoperability between applications that exchange machine-understandable information. RDF highlights resources in the form of subject-predicate-object expressions, often known as triples.
Data mining, on the other hand, is a powerful technology aiming to discover unknown and potentially useful patterns lying within expansive datasets. It incorporates machine learning, statistical, and visualization techniques to discover and present knowledge in a form which is easily comprehensible to humans.
RDF and ChatGPT-4: A Powerful Union
Now let's delve into how these technologies interplay with ChatGPT-4. GPT-4 or Generative Pre-trained Transformer 4 is an autoregressive language prediction model. It uses deep learning to produce human-like text. RDF can be a potent tool to extract and analyze the data generated by ChatGPT-4.
The data produced by ChatGPT-4 can be extensive and extremely intricate due to the nuanced nature of human language, which could include a variety of inferences, speech acts, and emotions. This is where the RDF standards and data mining techniques can help. By translating these GPT-4 conversations into the form of RDF triples, you can inspect individual data points independently and within their larger conversational context. This makes data mining a much easier task.
Unleashing Insights with RDF and GPT-4
To extract meaningful insights from the dataset created by GPT-4, we can apply different data mining techniques. For instance, clustering can identify common themes or topics within the data. Classification can help sort the data based on predetermined categories. Association rules can identify if certain phrases or topics often appear together, implying a strong connection between them.
But the process doesn't end here. Analyzing the mined data is as crucial as extracting it. Analysts can visualize the mined data, inspect it manually, or use advanced algorithms for interpretation. With the scalability of RDF and GPT-4, this process can be automated to mine and analyze in real-time.
Conclusion
Understanding language can provide immense value, especially when it comes to evolving technologies like ChatGPT-4. Using RDF for data mining can help uncover meaningful patterns and connections. Whether it's for a deep language study or market trend analysis, integrating RDF, data mining, and GPT-4 can lead to significant business insights and enhancement of AI technologies over time.
As technologies continue to develop and intertwine, the potential for groundbreaking, data-driven insights only increases. RDF, data mining, and GPT-4 are undeniably a part of this future, promising a dynamic symbiosis of information extraction, analysis, and generation.
Comments:
Great article! The combination of RDF and ChatGPT is promising for advancing technological interactions.
I agree, David! It's exciting to see how natural language processing can enhance the capabilities of RDF.
Thank you, David and Susan! I'm glad you found the article interesting.
I'm not sure I fully understand RDF. Can someone explain it in simpler terms?
Sure, Mark! RDF stands for Resource Description Framework. It's a way to represent and exchange knowledge on the web using subject-predicate-object triples.
Well explained, Alice! RDF provides a structured way to describe relationships between different entities.
I'm curious, how can ChatGPT specifically enhance RDF interactions?
Good question, Andrew! ChatGPT can help with natural language understanding and generation, which can make interacting with RDF data more intuitive and user-friendly.
That sounds promising, Daniel. It could potentially attract more users to RDF-based applications.
I think leveraging ChatGPT for RDF is a great idea! It could make querying and exploring linked data much easier.
I'm curious to know if there are any limitations or challenges when combining RDF and ChatGPT.
Good point, William. One challenge could be ensuring semantic coherence and avoiding misinterpretation of user queries.
That's true, Susan. It requires robust natural language understanding to accurately capture user intent when querying RDF data.
Another challenge could be handling the complexity of RDF data and ensuring efficient retrieval of relevant information.
I agree, Alice. Navigating large RDF knowledge graphs can be overwhelming, so efficient retrieval is crucial.
Indeed, Alice and Emily! Overcoming these challenges will be essential for the successful integration of ChatGPT and RDF.
These are all great suggestions! Thank you, Alice, David, Susan, and Emily, for sharing valuable learning resources.
Are there any real-world applications already leveraging RDF and ChatGPT together?
There are a few ongoing projects exploring the combination of RDF and ChatGPT, but it's still a relatively new area of research.
I can see potential applications in personalized recommendation systems that leverage RDF knowledge and user interactions through ChatGPT.
That's a great point, Emily. ChatGPT could provide more conversational and tailored recommendations based on user preferences.
I completely agree, Emily. The combination of RDF and ChatGPT could revolutionize the recommendation systems we have today.
Thank you all for your insightful comments and discussions! It's been great to hear your perspectives.
Thank you, Ngina! Your article has sparked an interesting conversation.
My pleasure, Mark! I'm glad to have initiated this discussion and learned from all of you.
Thank you, Ngina! Your article has shed light on an exciting direction for the use of ChatGPT.
Ngina, your article has provided valuable information on the intersection of RDF and ChatGPT. Thank you!
You're most welcome, Mark! I appreciate your feedback and engagement.
Indeed, Ngina! Your article has sparked insightful conversations and increased awareness of RDF and ChatGPT.
I'm thrilled to hear that, Mark! It was my intention to create awareness and foster discussions around these exciting technologies.
I'm new to RDF, but this article has piqued my interest. Are there any resources you would recommend to learn more?
Certainly, Sophia! The W3C website is a great starting point for learning about RDF and its specifications.
Thanks, Alice! That clarifies the concept of RDF for me.
You're welcome, Mark! Feel free to ask if you have any more questions.
I would also recommend the book 'Learning SPARQL' by Bob DuCharme. It provides a practical introduction to querying RDF data.
If you prefer online courses, there are some excellent ones on platforms like Coursera and Udemy.
You can also find tutorials and examples on GitHub to get hands-on experience with RDF.
I'm excited to see the progress in integrating ChatGPT and RDF. It has the potential to make knowledge discovery more accessible to everyone.
I share your enthusiasm, Andrew! The combination of these technologies can democratize access to information and foster new discoveries.
How secure is ChatGPT when handling RDF data? Are there risks associated with it?
That's an important concern, Sarah. Ensuring data security and privacy would be crucial when applying ChatGPT to RDF interactions.
I agree, Alice. It's essential to implement appropriate measures to protect sensitive RDF data.
Absolutely, Sarah. Security measures like access controls and encryption should be in place to mitigate potential risks.
Efficient retrieval of relevant information is indeed a challenge. Do you have any recommendations or techniques to address this?
A common technique is to use SPARQL queries with appropriate filters and optimizations to retrieve specific subsets of RDF data efficiently.
Additionally, caching and indexing mechanisms can improve the retrieval performance of RDF data.
True, Mark. Caching frequently accessed RDF data can reduce the need for repeated retrieval and enhance the overall efficiency.
Indexing techniques like property tables or graph partitioning can also help optimize querying large RDF knowledge graphs.
ChatGPT's conversational recommendations sound promising. How could it handle diverse user preferences?
Good question, Sophia. ChatGPT could employ personalized learning mechanisms to understand and adapt to individual user preferences.
Sophia, ChatGPT could also take into account explicit user feedback, allowing users to influence the recommendations they receive.
Additionally, collaborative filtering techniques could be used to analyze user interactions and make recommendations based on similar users.
That's an interesting point, David. Incorporating collaborative filtering can help provide diverse recommendations beyond individual preferences.
These discussions have been fantastic! Thank you all for actively participating and sharing your insights.
Indeed, Ngina! It's been a pleasure engaging in this conversation with everyone.
Thank you, Ngina, for writing such an informative article and facilitating this valuable discussion.
You're most welcome, Sarah! I'm grateful for your kind words and enthusiasm.
Ngina, thank you for sharing your insights and expertise with us through this article.
Thank you, John! I'm humbled and grateful for your kind words.
Great question, John. There are various techniques and tools available for efficient retrieval of relevant information from large RDF datasets.
That's right, Daniel. Utilizing indexing and caching mechanisms, along with optimized SPARQL queries, can significantly improve performance.
Thank you, Alice and David! I'll check out the W3C website and the book you mentioned.
You're welcome, Sarah! It's a great starting point to dive into RDF and its specifications.
I'm excited about the future applications of RDF and ChatGPT. They have great potential to advance various fields.
Indeed, Michael! RDF and ChatGPT have far-reaching potential across industries and research domains.
Absolutely, Andrew! The synergistic combination of these technologies can unlock new opportunities.
Thank you, Daniel! Your explanations have provided valuable insights into the potential of ChatGPT and RDF.
Indeed, Andrew! The combination of these technologies can pave the way for transformative advancements in knowledge discovery.
Absolutely, Michael! The combination of these technologies can transform how we interact with and derive insights from data.
This article has sparked my interest in RDF and ChatGPT. I can't wait to explore their capabilities further.
That's fantastic, Jennifer! Dive in and enjoy discovering the possibilities.
Thanks, David, for recommending additional resources. I'm eager to enhance my knowledge in this domain.
You're most welcome, Sophia! Enjoy your learning journey in RDF and ChatGPT.
Great discussions everyone! It's refreshing to see the potential of RDF and ChatGPT being explored.
Indeed, Andrew! It's an exciting time for advancements in technology and data-driven interactions.
Absolutely, Emily! It's an exciting time for technological advancements and the exploration of new possibilities.
I couldn't agree more, Andrew and Emily! The future looks promising.
Thank you, Susan! I'll definitely reach out if I have more questions.
Thank you, Emily and Alice! I'll explore SPARQL queries and look into caching mechanisms for improved retrieval.
Thank you, Ngina! Your expertise and willingness to engage in discussions make this article even more valuable.
I'm grateful for your appreciation, John! I'm truly thrilled to be part of such insightful conversations.
Your article has sparked meaningful discussions, Ngina. Thank you for sharing your knowledge with us.
It's my pleasure, Susan! The engagement and enthusiasm from everyone have made these discussions truly valuable.
Your article has certainly achieved that, Ngina. Thank you for sharing your expertise with us.
You're most welcome, John! I'm grateful for the opportunity to share and learn from all of you.
Ngina, your vision of democratizing access to information is inspiring. Thank you for sharing your article.
Thank you for your kind words, John! It's my vision and hope to contribute to a more inclusive and accessible technological landscape.
Let's keep exploring and pushing the boundaries of RDF and ChatGPT. The possibilities are endless.
I couldn't agree more, Emily! Continuous exploration and innovation will drive the advancements in RDF and natural language processing.
Thank you all for your remarkable contributions and thoughtful discussions! It's been an absolute pleasure.
Your article has left me curious and excited, Ngina! Thank you for providing valuable insights.
Your enthusiasm means a lot, Sophia! I appreciate your kind words and participation in this discussion.
Caching and indexing mechanisms are indeed important. They can significantly improve the performance of RDF data retrieval.
Absolutely, David. Efficient retrieval and response times are key factors in providing a seamless user experience.
RDF and ChatGPT seem like a perfect match for enhancing technological interactions.
Absolutely, Alex! Their combination opens up new possibilities for more effective and intuitive interactions.
It's exciting to see how natural language processing is evolving and making complex technologies more accessible.
I couldn't agree more, Kevin! Natural language processing has the potential to bridge the gap between humans and technology.
RDF and ChatGPT have amazing potential for improving user experiences and knowledge discovery.
Indeed, Emma! The combination of these technologies can empower users and unlock new insights in a variety of domains.
Thank you all for your comments! I am glad you found the article interesting.
This is a great article, Ngina! I really enjoyed reading it. ChatGPT seems like a powerful tool for enhancing interactions. Do you have any ideas on how this technology can be applied in the real world?
Thank you, Sarah! ChatGPT indeed has numerous applications. One potential use case is in customer support systems, where it can assist in providing faster and more accurate responses to customer queries. It can also be integrated into educational platforms to enhance learning experiences.
I agree with Sarah, Ngina. Your article sheds light on an interesting advancement in technology. I believe that ChatGPT has the potential to revolutionize the way we interact with machines. However, do you think there are any limitations to this technology?
Thank you, Daniel! ChatGPT is indeed an exciting advancement. As for limitations, it can sometimes generate incorrect or nonsensical responses. It can also be sensitive to input phrasing, where a slight rephrase of a question might yield a different answer. However, OpenAI is actively working to address these limitations.
This article is very informative, Ngina. I had heard about ChatGPT but didn't know much about its potential. Thanks for sharing! Do you have any insights into the underlying technology behind ChatGPT?
Thank you, Olivia! ChatGPT is powered by a language model called GPT-3, which stands for 'Generative Pre-trained Transformer 3.' It is built on a transformer architecture and has been trained on a vast amount of text data. This allows it to generate coherent and contextually relevant responses based on the input.
Ngina, I appreciate your article. ChatGPT seems like a breakthrough. I'm curious to know if there are any privacy concerns associated with this technology.
Thank you, Emily! Privacy concerns are indeed important. OpenAI is aware of the potential risks and is working on improving the safety and privacy features of ChatGPT. They are also seeking external input and conducting red teaming to identify and address vulnerabilities.
Great article, Ngina! It's fascinating to see how AI is advancing. However, there might be cases where the output of ChatGPT could be misused or manipulated. Are there any measures in place to prevent such misuse?
Thank you, Michael! Preventing misuse is a significant concern. OpenAI has implemented usage policies and put measures in place to detect and mitigate malicious uses of its technology. They are actively developing AI safety techniques to minimize potential risks.
Ngina, I thoroughly enjoyed your article. It's incredible to see AI innovations. How do you think ChatGPT compares to other similar conversational AI models?
Thank you, David! ChatGPT is a significant step forward in conversational AI. Its predecessor, GPT-2, demonstrated impressive capabilities, but ChatGPT has even further improvements. It can engage in more interactive and context-aware conversations, making it a valuable tool for advanced technological interactions.
Ngina, your article is very well-written. I can see the potential of ChatGPT in various applications. However, have there been any studies on the ethical implications of using this technology?
Thank you, Sophia! Ethical implications are a critical aspect to consider. While there have been studies highlighting concerns related to bias and potential misinformation, researchers and organizations are actively working on addressing these issues to ensure responsible and ethical usage of ChatGPT.
Thanks for sharing your insights, Ngina. I find ChatGPT fascinating. Can you shed some light on how ChatGPT is trained on text data?
You're welcome, Liam! ChatGPT is trained using a method called unsupervised learning. It learns from a large corpus of publicly available text from the internet. By predicting the next word in a sentence, it develops an understanding of grammar, style, and context, enabling it to generate natural-sounding responses.
Ngina, your article is very informative. ChatGPT has tremendous potential. However, could you explain the challenges encountered during the training of ChatGPT?
Thank you, Sophie! Training ChatGPT posed several challenges. One challenge is the difficulty of scaling the model to handle large datasets. Another is the computational resources required for training such a vast language model. Additionally, fine-tuning models like ChatGPT can be a time-consuming and resource-intensive process.
Great article, Ngina! The advancements in AI are mind-blowing. However, I'm curious about the potential biases that might be present in the responses generated by ChatGPT.
Thank you, Ethan! Biases can indeed be a concern. ChatGPT's training data comes from the internet, which can contain biases present in the text. As a result, it might generate responses that reflect those biases. OpenAI is actively working on reducing both glaring and subtle biases in ChatGPT's responses.
Ngina, great job on the article! I'm amazed by how far AI has come. How accessible is ChatGPT for developers and businesses?
Thank you, Aiden! OpenAI offers an API that allows developers to access ChatGPT and integrate it into their applications. Businesses can leverage this API to build various AI-powered solutions. However, there are usage restrictions and costs associated with API access, so it's important to carefully consider the implementation.
Ngina, I found your article enlightening. It's impressive how AI is transforming various sectors. What kind of training does ChatGPT undergo to ensure its effectiveness?
Thank you, Ella! ChatGPT undergoes a two-step process. Initially, it is trained using a large corpus of publicly available text from the internet. Afterward, it goes through a fine-tuning phase where human reviewers follow guidelines provided by OpenAI to review and rate potential model outputs. This iterative feedback process hones the model's performance.
Ngina, your article provides a well-rounded view of ChatGPT. It seems like a powerful tool. However, have there been any instances where ChatGPT failed to provide accurate responses?
Thank you, Henry! ChatGPT has its limitations, and there are instances where it may provide responses that are incorrect or nonsensical. OpenAI actively encourages feedback on problematic outputs to further improve the model. Users can provide feedback through the user interface to report any issues encountered.
Great job on the article, Ngina! I found it very insightful. How do you see the future of ChatGPT in terms of advancements and applications?
Thank you, Emma! The future of ChatGPT looks promising. OpenAI is focusing on addressing its limitations, reducing biases, and improving its features. As the technology progresses, we can expect to see wider applications in various fields, such as medicine, research, and more advanced human-machine interactions.
Ngina, I appreciate your article. ChatGPT seems like a game-changer. However, I'm concerned about the potential for AI replacing human jobs. What are your thoughts on this?
Thank you, Jack! The concern about AI replacing human jobs is valid. However, technologies like ChatGPT should be seen as tools that can assist humans rather than replace them entirely. By automating certain tasks, it can free up humans to focus on more complex and meaningful work, leading to productivity and innovation.
Ngina, your article explores a fascinating application of AI. ChatGPT has immense potential. Do you think it will reach a point where it can pass the Turing test?
Thank you, Jonathan! The Turing test poses a significant challenge, as it requires an AI system to exhibit intelligence indistinguishable from a human. While ChatGPT showcases impressive capabilities, it still has room for improvement to reach the level of passing the Turing test. Many researchers and organizations continue to work towards achieving this goal.
Ngina, your article is informative. It's interesting to see how AI is evolving. Could you share any success stories where ChatGPT has been implemented effectively?
Thank you, Grace! Although ChatGPT is a recent development, there have been successful implementations in various areas. Some examples include using it to draft emails, brainstorm ideas, create Python code snippets, and even as a language learning tool. These applications showcase the versatility and potential of ChatGPT.
Great article, Ngina! I'm excited about the possibilities that ChatGPT offers. However, I'm curious to know if it can understand and respond to different languages.
Thank you, Lucas! While ChatGPT has been trained on a vast amount of English text, it can understand and generate responses in languages other than English. However, its performance might vary based on the language, as it hasn't undergone extensive training on specific languages.
Ngina, I found your article thought-provoking. ChatGPT has significant potential. However, have there been any incidents where it produced content that violates ethical guidelines?
Thank you, Isabella! OpenAI is committed to ensuring responsible AI usage. In the past, there have been instances where ChatGPT produced outputs that violated ethical guidelines. However, they are actively working to mitigate such risks and improve the system's behavior through guidelines and continuous feedback from users.
Ngina, this article is well-researched. ChatGPT is an exciting development indeed. Could you elaborate on how ChatGPT's responses are generated from given inputs?
Thank you, Valentina! ChatGPT generates responses by conditioning on both the input given by the user and the past conversation within a specific context window. It uses its predictive abilities, learned during training, to generate what it believes is the most likely next response given the input and context.
Thanks for sharing, Ngina. ChatGPT seems very promising. However, are there any constraints on using the technology due to computational limitations?
You're welcome, Robert! Indeed, computational limitations exist. Generating responses with ChatGPT can take time due to its complex architecture and resource requirements. Additionally, the API access for developers has usage restrictions and costs associated. These factors need careful consideration while incorporating ChatGPT into applications.
Ngina, I enjoyed your article. The potential of ChatGPT is impressive. However, could you explain how ChatGPT handles ambiguous or unclear queries?
Thank you, Victoria! ChatGPT tries to understand the user's intent even when queries are ambiguous or unclear. However, it may sometimes request clarification if the query lacks necessary details. It aims to provide relevant responses based on the given input and context.
Ngina, great article! AI advancements are fascinating. However, what measures are in place to ensure that ChatGPT's responses are safe and reliable?
Thank you, Jonathan! Safety and reliability are paramount. OpenAI has implemented safety mitigations to prevent the system from generating harmful or inappropriate outputs. They also have a strong feedback loop with human reviewers to address potential risks. ChatGPT's behavior is continually shaping to improve user safety.
Ngina, your article is well-articulated. ChatGPT seems like an impressive technology. However, are there any considerations to make regarding biased training data?
Thank you, Jasmine! Biased training data is a concern, as it can lead to biased responses. OpenAI recognizes this and is working toward reducing both glaring and subtle biases in how ChatGPT responds. They are actively seeking external input to address biases and improve the overall accuracy and fairness of the model.
Ngina, great insights. ChatGPT has incredible potential. Are there any plans to make it even more accessible in the future?
Thank you, Joseph! OpenAI's vision is to refine and expand access to ChatGPT. They plan to refine the offering based on user feedback and requirements. They are also actively considering options for lower-cost plans and business-oriented offerings to make it more accessible and beneficial for a wider audience.
Ngina, I learned a lot from your article. ChatGPT's advancements are inspiring. However, have there been any concerns regarding integrating ChatGPT into critical systems such as healthcare or autonomous vehicles?
Thank you, Lily! Integrating ChatGPT into critical systems poses unique challenges and requires careful considerations. Safety and robustness become crucial factors in such applications. OpenAI recognizes this and is actively collaborating with experts and the broader community to explore and address these concerns. Rigorous evaluation and safety measures need to be in place before deploying ChatGPT in critical systems.
Ngina, your article is insightful. ChatGPT offers exciting possibilities. However, could you share any limitations in terms of the length or complexity of queries it can handle?
Thank you, Mia! ChatGPT has some limitations in terms of query length and complexity. Extremely long queries might get truncated, resulting in incomplete responses. It's also more likely to generate accurate responses for queries within its training domain. Complex queries might sometimes confuse or produce less reliable results.
Ngina, your article paints a clear picture of ChatGPT's potential. I'm curious to know how ChatGPT handles ambiguous or multiple interpretations in questions.
Thank you, Christopher! ChatGPT tries its best to understand ambiguous queries or multiple interpretations. However, it may not always produce the desired answer in such cases. Providing additional context or rephrasing the question can help in reducing ambiguity and increasing the accuracy of responses.