Enhancing Time Series Analysis in Mathematical Programming with ChatGPT
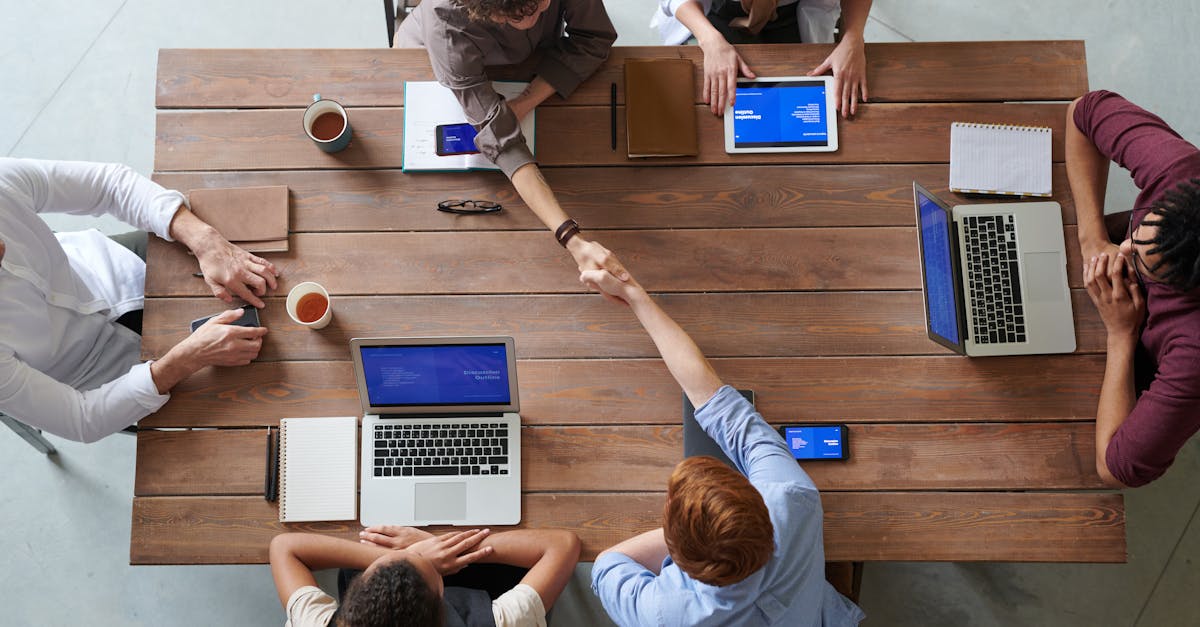
Time series data, consisting of observations taken at regular intervals over time, can provide valuable insights into various domains such as finance, economics, weather forecasting, and more. Analyzing and predicting time series data is essential for making informed decisions. One powerful technique used for this purpose is Mathematical Programming, which has gained significant popularity in recent years.
Mathematical Programming involves formulating a set of mathematical equations that describe the behavior of a time series and finding the optimal solutions to these equations. It combines mathematical optimization and statistical analysis to model and analyze time-dependent phenomena.
One application of Mathematical Programming in the field of Time Series Analysis is with the advent of advanced language models like ChatGPT-4. With its ability to understand and generate human-like language, ChatGPT-4 can build models to predict, forecast, understand, and explore future states of time series data.
The predictive power of ChatGPT-4 allows businesses and researchers to make accurate predictions and take proactive action based on the analyzed time series data. For example, in financial markets, ChatGPT-4 can analyze historical stock prices and predict future trends, enabling traders to make informed investment decisions.
Additionally, ChatGPT-4's forecasting capabilities make it valuable in various domains where accurate predictions of future states are crucial. For instance, in the field of meteorology, ChatGPT-4 can analyze historical weather data to forecast potential weather patterns, helping meteorologists issue timely warnings and manage resources accordingly.
Moreover, ChatGPT-4's ability to understand the underlying patterns and relationships in time series data enables researchers to gain a deeper understanding of complex systems. By exploring future states of time series data, it becomes possible to identify potential risks, explore various scenarios, and plan for contingencies more effectively.
In conclusion, Mathematical Programming, when combined with advanced language models like ChatGPT-4, offers a powerful approach for analyzing and predicting time series data. Its applications in various domains such as finance, meteorology, and more, make it an invaluable tool for decision-making and proactive action. With the ability to build accurate models for prediction, forecasting, and exploring future states, ChatGPT-4 opens up new avenues for leveraging the potential of time series analysis.
Comments:
Great article, Claire! I found the concept of combining time series analysis with mathematical programming really fascinating. It seems like this could have significant applications in various fields.
I agree, Michael! It's exciting to see how ChatGPT can enhance time series analysis. I wonder if there are any specific industries that could benefit the most from this integration.
Claire, your article provides a clear explanation of the benefits of using ChatGPT in time series analysis. I'm curious to know if there are any limitations or potential challenges associated with this approach.
Andrew, while ChatGPT shows promise in time series analysis, one potential limitation could be the requirement for a large amount of high-quality training data. Without sufficient data, the model's accuracy and reliability may be compromised.
That's a valid concern, Jennifer. Collecting and preparing reliable training data is crucial for any machine learning model, including ChatGPT. It's important to consider the data requirements and potential biases that might affect the analyses.
Andrew, you mentioned potential biases in the analysis. It's essential to carefully analyze and mitigate biases to ensure ethical and fair decision-making when using AI models like ChatGPT.
Absolutely, Gabriel. Bias in AI models can have detrimental impacts, perpetuating inequalities and unfair outcomes. Regular monitoring, transparency, and diverse representation in training data are crucial steps toward reducing biases in time series analyses.
Andrew and Gabriel, you bring up important points about biases. Addressing biases and ensuring fairness should be a continuous effort in AI research and application. It's crucial to recognize and mitigate biases to build trustworthy and inclusive models.
Thank you, Michael, Stephanie, and Andrew, for your comments! I appreciate your positive feedback and curiosity. Stephanie, to answer your question, industries like finance, energy, and manufacturing could greatly benefit from this integration, as they rely heavily on analyzing time series data for forecasting and decision-making.
I believe the healthcare industry could also benefit from integrating ChatGPT into time series analysis. It could help improve patient monitoring and disease prediction.
That's a great point, Daniel! The healthcare sector could definitely leverage the power of ChatGPT in analyzing medical data over time to enhance diagnoses and treatment plans.
Daniel and Stephanie, you both make excellent points! Healthcare is indeed an industry that can benefit from ChatGPT in time series analysis. The ability to monitor patient health and predict diseases accurately can save lives and improve healthcare outcomes.
Agreed, Claire. The potential applications of ChatGPT in healthcare are vast. It's exciting to think about the positive impact it could have on patient care and medical research.
I'm curious about the level of explainability that ChatGPT can provide in time series analysis. Understanding how the model arrives at its predictions is crucial for gaining trust and acceptance in critical domains.
That's an important consideration, David. Explainability is indeed crucial, especially in applications where decisions based on time series analyses can have significant consequences. It would be great to have insights into how ChatGPT forms its recommendations.
David and Stephanie, explainability is a valid concern. While ChatGPT is an advanced model, understanding its decision-making process can be challenging. Developing methods to provide insights into the model's reasoning is an active area of research globally.
In industries where regulatory compliance is crucial, explainability becomes even more critical. Companies need to provide evidence-based reasoning behind their decisions to fulfill legal and ethical requirements.
Absolutely, John. Explainability and transparency are essential for establishing trust and ensuring compliance in industries that heavily rely on time series analysis. Research efforts continue toward making AI models more interpretable.
Do you think there should be regulations or standards specifically addressing the use of AI models like ChatGPT in critical domains? It could help set guidelines for ensuring explainability and accountability.
I believe regulations would be beneficial, Evelyn. Establishing guidelines for AI model usage in critical domains can provide a framework for accountability, transparency, and ensure the responsible deployment of such powerful technologies.
In addition to addressing biases in the models, we should also consider the potential biases present in the data itself. Biased data can lead to skewed results and erroneous conclusions.
Absolutely, Sophia! Biases within the data can significantly impact the accuracy and reliability of time series analyses. Data preprocessing techniques and bias detection methods can help mitigate these challenges.
Well said, Sophia and Gabriel. Biases in data can be subtle yet influential. It's crucial to ensure proper data collection, cleaning, and validation to minimize biased results and enhance the reliability of time series analysis.
Absolutely, Claire. By addressing biases at the data level, we can significantly improve the accuracy and fairness of the insights derived from time series analysis.
I'm glad the issue of biases in data and their impact on time series analyses is being highlighted. It's a critical aspect that should not be overlooked in our pursuit of more powerful AI models.
Indeed, Michael. Recognizing and addressing biases in both models and data is crucial to ensure the ethical and accurate use of AI in time series analysis. It's an important aspect to consider in the development and deployment of such technologies.
Claire, your emphasis on the collaboration between humans and AI is spot on. It's the synergy of human intuition and machine learning capabilities that allows us to tackle complex problems effectively.
Absolutely, Michael. The collaboration between humans and AI can unlock new possibilities, enabling us to leverage the strengths of both for more insightful and accurate decision-making.
Claire, what challenges do you foresee in implementing ChatGPT for time series analysis? Are there any specific technical or practical hurdles one should be aware of?
Emily, implementing ChatGPT for time series analysis may face challenges in adapting the model to handle varying time intervals and irregular patterns in data. Additionally, long training times and computational resource requirements can pose practical hurdles. However, ongoing research aims to address these challenges and make the integration smoother.
Claire, how scalable would you say the integration of ChatGPT is for handling large-scale time series datasets? Are there any limitations in terms of model complexity or dataset size?
Good question, Emma. The scalability of ChatGPT for large-scale time series datasets depends on several factors like computational resources, training time, and model architecture. While there might be limitations with extremely large datasets, advancements in hardware and model optimization techniques contribute to improving scalability.
Claire, thank you for your insights! It's clear that combining ChatGPT with attention-based models has great potential. I'm excited to see how this integration can enhance time series analysis in various domains.
You're welcome, Emily! I'm also thrilled about the possibilities this integration offers. It's an exciting time for advancing time series analysis with the power of AI models like ChatGPT.
Claire, what future developments or research areas do you think hold promise for further improving time series analysis with AI models?
Sophia, there are several promising research areas for enhancing time series analysis with AI models. Improving interpretability, addressing biases, developing novel architectures tailored for time series data, and exploring robust ensemble methods are all avenues with potential for further advancements.
Claire, have there been any real-world case studies or practical implementations showcasing the effectiveness of ChatGPT in time series analysis? It would be interesting to see some examples.
Jessica, there has been growing interest and experimentation with ChatGPT in time series analysis; however, rigorous case studies and practical implementations are still emerging. Researchers and industry practitioners are actively exploring the full potential and refining approaches for specific applications.
I think ensuring diverse representation in the training data is an important step towards mitigating biases. It can help reduce the chances of biased predictions and ensure fairness across different demographic groups.
Great point, Rachel. Diverse representation in the training data helps create more inclusive and unbiased models. It plays a crucial role in ensuring that AI systems like ChatGPT produce fair and reliable results for everyone.
I fully agree, Claire. A diverse and representative training dataset can help mitigate the risk of inadvertently perpetuating biases and ensure that AI models are fair and inclusive in their behavior.
The role of data diversity shouldn't be underestimated. By considering diverse perspectives and understanding the different contexts, the models we develop can be more robust and reliable in a wider range of scenarios.
I believe it's crucial to also consider the potential ethical implications of relying too heavily on AI models like ChatGPT for decision-making. Human judgment and domain expertise should still play a significant role in critical analyses.
Well said, Nathan. Utilizing AI models like ChatGPT should be seen as augmenting human decision-making rather than replacing it. Human oversight and interpretation are crucial for maintaining accountability and detecting potential errors.
Nathan and Andrew, you raise an important aspect. AI models should be viewed as tools that assist and augment human decision-making, but human judgment, expertise, and accountability remain vital components in critical analyses.
I agree with what's been said. Human intuition and critical thinking cannot be replaced by AI models alone. It's crucial to have a collaborative approach that embraces both human and machine intelligence for optimal decision-making.
Considering the potential limitations of scalability, do you think there are alternative approaches or hybrid models that could complement ChatGPT in time series analysis?
Isabella, hybrid approaches that combine the strengths of different models or techniques can be beneficial. Ensemble methods or combining ChatGPT with other models specialized in time series analysis might enable handling more diverse datasets and improve accuracy.
Claire, what are your thoughts on using attention-based models in combination with ChatGPT for time series analysis? Could it help improve the model's ability to capture temporal patterns?
James, attention-based models can indeed be valuable when combined with ChatGPT for time series analysis. Attention mechanisms allow the model to focus on relevant temporal patterns, making it more capable of capturing long-range dependencies and improving overall performance.