Enhancing Topology Optimization in Hypermesh with ChatGPT: Revolutionizing the Design Process
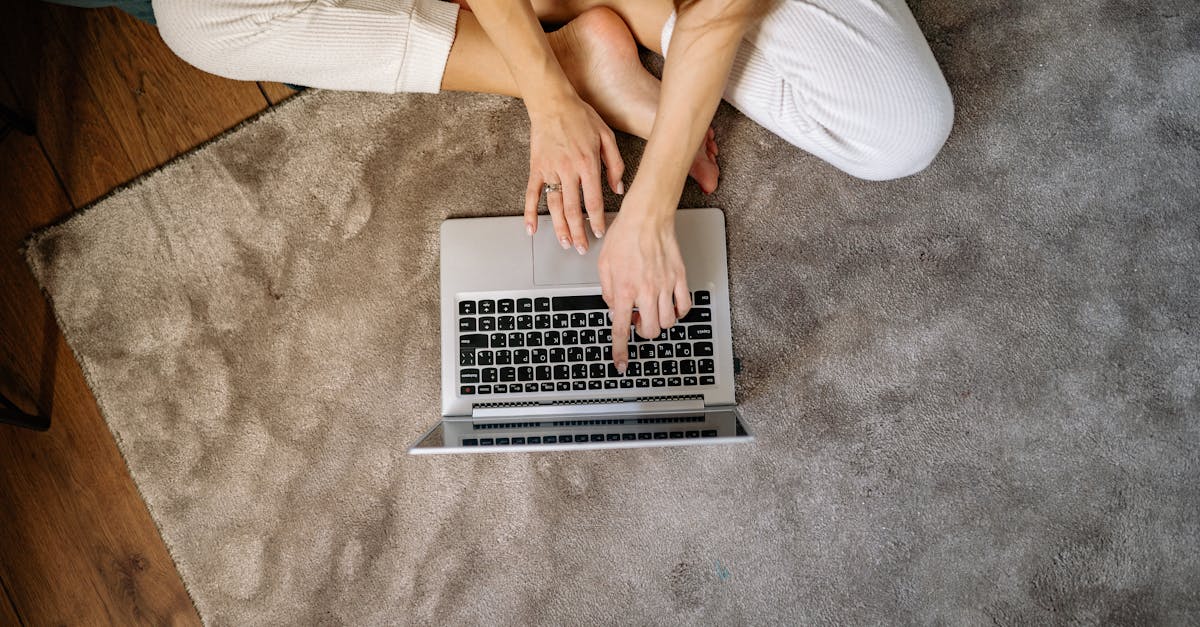
Topology optimization is a powerful technique used in engineering design to find the optimal material distribution within a given design domain. It is often used to optimize structural components by minimizing their weight while maintaining sufficient strength and stiffness.
Introduction to Hypermesh
Hypermesh is a leading software tool used in the field of finite element analysis (FEA) and is widely used for conducting topology optimization. It provides efficient and effective meshing capabilities, enabling engineers to create complex finite element models.
Procedures for Topology Optimization
Topology optimization involves several key steps that need to be carried out in order to obtain the desired results. These steps include:
- Design Domain Definition: The first step is to define the design domain or the region within the structure where the optimization will be performed. This can be done using CAD tools or by creating a solid model directly within Hypermesh.
- Loads and Constraints: Once the design domain is defined, the next step is to apply loads and constraints to the model. These represent the conditions under which the structure will be analyzed. Loads can include forces, moments, and pressure, while constraints can be in the form of fixed displacements or constrained degrees of freedom.
- Material Properties and Optimization Criteria: In this step, the material properties for the design domain are defined. This includes the Young's modulus, Poisson's ratio, and density. Additionally, the optimization criteria are established, which define the objective function to be minimized. Common objectives include minimizing compliance (strains and stresses) or maximizing stiffness while keeping the weight within certain limits.
- Mesh Generation: Once the design domain, loads, and material properties are defined, the next step is to generate a mesh using Hypermesh. The mesh should be fine enough to capture the structural behavior accurately but not too fine to avoid unnecessary computational costs.
- Topology Optimization: With the mesh in place, Hypermesh provides tools to perform topology optimization. These tools use mathematical algorithms to iteratively remove or redistribute material from the initial design to find the optimal configuration. Engineers can set various parameters such as the volume fraction, minimum member size, and maximum iteration count to guide the optimization process.
- Post-processing: Once the topology optimization is complete, engineers need to analyze and interpret the results. Hypermesh allows for visualization of the optimized topology and provides tools to extract numerical data such as stiffness, mass, and stress distribution.
- Iterative Refinement: In many cases, the initial topology obtained from the optimization process may require further refinement. Engineers can iterate through the optimization and post-processing steps to fine-tune the design and achieve the desired compromise between weight and structural performance.
Considerations for Topology Optimization
While performing topology optimization using Hypermesh, there are several important considerations to keep in mind:
- Feasibility: The optimized topology obtained from the software should be manufacturable within the given constraints such as material availability, manufacturing processes, and cost.
- Mesh Quality: The quality of the mesh created in Hypermesh plays a crucial role in the accuracy of the optimization results. A well-structured and fine mesh is necessary to capture the structural behavior effectively.
- Boundary Conditions: Proper definition of loads and constraints is essential for obtaining realistic optimization results. Engineers should carefully consider the application-specific boundary conditions during the modeling process.
- Objective Function: Choosing an appropriate objective function is important to achieve the desired design goals. Engineers should carefully define the objective function based on the specific requirements of the structure.
- Optimization Parameters: The selection of optimization parameters in Hypermesh can greatly influence the final results. Engineers should experiment with different settings to achieve the optimal design while considering computational efficiency.
Conclusion
Topology optimization with Hypermesh is a valuable tool for engineers involved in structural design. By following the procedures and considerations outlined above, engineers can leverage the power of Hypermesh to optimize the material distribution and achieve lightweight yet structurally efficient designs.
With its powerful meshing capabilities and optimization algorithms, Hypermesh greatly facilitates the topology optimization process. By accurately defining design domains, applying appropriate loads and constraints, and selecting optimal material properties and criteria, engineers can successfully utilize Hypermesh to create innovative and optimized designs.
Comments:
Thank you all for your interest in my article on Enhancing Topology Optimization in Hypermesh with ChatGPT! I'm excited to hear your thoughts and answer any questions you may have.
Great article, Ethan! Topology optimization is an essential aspect of the design process, and it's fascinating to see how AI-powered tools like ChatGPT can revolutionize it.
I absolutely agree, Matthew! The combination of topology optimization and AI has the potential to significantly improve the efficiency and effectiveness of the design process.
Indeed, Sophia! I can imagine that using a tool like ChatGPT would not only streamline the optimization process but also provide valuable insights and suggestions throughout the design iterations.
The integration of AI in design sounds promising! Ethan, could you provide some practical examples or case studies where you have applied ChatGPT to enhance topology optimization in Hypermesh?
Absolutely, Alice! We have successfully used ChatGPT in various engineering applications, including topology optimization. One particular example involved designing a lightweight aircraft frame while satisfying structural constraints and minimizing material usage. ChatGPT helped in generating innovative and efficient designs.
Additionally, we applied ChatGPT to optimize the layout of internal cooling channels in a turbine blade, maximizing cooling efficiency while minimizing pressure drop. The generated designs provided superior performance compared to traditional methods.
That's impressive, Ethan! I'm curious about the training data used for ChatGPT. How did you ensure that the AI model understands the design constraints and requirements specific to topology optimization?
Good question, Oliver! We trained ChatGPT on a large dataset of engineering designs along with their corresponding constraints and objectives. This allowed the model to learn the patterns and relationships between design parameters, constraints, and optimization goals.
During the training process, we fine-tuned the model by exposing it to simulated design scenarios while providing feedback based on engineering principles. This iterative process helped the AI model grasp the unique considerations of topology optimization.
As an industrial designer, I'm intrigued by the potential of ChatGPT in the design process. Ethan, do you foresee any limitations or challenges in leveraging AI for topology optimization?
Great question, Maria! While AI can greatly benefit topology optimization, there are challenges to overcome. The generated designs need to be feasible in terms of manufacturing and practicality. Incorporating production constraints and process-specific considerations into the optimization process is an ongoing research area.
Another challenge is the interpretability of AI-generated designs. We need to ensure that the optimization results can be understood and validated by engineers. Efforts are being made to develop methods that balance design performance and interpretability.
Ethan, it's fascinating how AI can improve design efficiency. However, what are the potential risks or ethical considerations when relying heavily on AI-powered tools like ChatGPT in the design process?
Excellent question, Rebecca! Ethical considerations are crucial when using AI in design. We must guard against biases in the training data and ensure that the generated designs meet ethical standards. Regular human oversight and validation of the AI-generated designs are essential.
Moreover, open communication and collaboration among designers, engineers, and AI experts are necessary to ensure responsible and transparent use of AI in the design process.
This article opens up a world of possibilities! I'm excited about the potential impact of AI-powered topology optimization. Great work, Ethan!
I couldn't agree more, Justin! The integration of ChatGPT with Hypermesh for topology optimization can truly revolutionize the way we approach design challenges.
I completely agree, Sophie! The possibilities are exciting, and I can't wait to see how AI shapes the future of design.
Ethan, I'm curious about the computational requirements of applying ChatGPT to topology optimization. Does it significantly increase the computational time or resources needed for the design process?
That's a valid concern, Daniel. While AI-assisted topology optimization introduces some computational overhead, recent advancements in hardware and optimization algorithms have mitigated the impact. The benefits gained from improved designs generally outweigh the additional computational cost.
Ethan, in your experience, how does ChatGPT compare to traditional optimization methods in terms of convergence speed and quality of the final designs?
Great question, Daniel! ChatGPT, combined with topology optimization, often converges faster compared to traditional methods. It can quickly explore design variations and provide rapid feedback, enabling designers to efficiently iterate and converge towards high-quality designs.
Thank you for addressing the computational concerns, Ethan! It's reassuring to know that the benefits outweigh the overhead of applying ChatGPT.
Ethan, how does ChatGPT assist engineers and designers in making decisions during the topology optimization process?
Great question, Olivia! ChatGPT interacts with engineers during the design process, suggesting possible design modifications, providing insights into trade-offs between different objectives, and explaining the reasoning behind recommendations. It acts as a helpful virtual assistant in decision-making.
Furthermore, engineers can ask ChatGPT questions about design choices, design constraints, or clarify ambiguities, enabling a more interactive and intuitive topology optimization experience.
Ethan, do you see ChatGPT as a complementary tool to human designers, or could it potentially replace human involvement in topology optimization entirely?
An excellent question, Lucas! ChatGPT is designed to augment human designers and engineers, providing them with enhanced capabilities and efficiency. It serves as a valuable tool, but human involvement and expertise remain crucial in ensuring the final designs meet all requirements.
I appreciate your emphasis on human involvement in the design process, Ethan. The collaboration between AI and human designers seems to be the ideal approach.
I'm thrilled about the potential of AI in topology optimization! Ethan, how do you envision the future development and integration of AI technologies in the design field?
Glad to hear your excitement, Emily! I believe AI technologies will continue to advance and play an increasingly important role in design. We can anticipate even more sophisticated AI models and seamless integrations with existing design software, enabling a more intuitive and efficient design process.
It's exciting to envision a future where AI-driven design processes become more accessible and widespread. Thanks for your insights, Ethan!
Moreover, the democratization of AI-powered tools and resources will empower more designers and engineers to leverage the benefits of topology optimization and other AI-driven design approaches.
Ethan, fantastic article! How can interested individuals or companies get started with integrating ChatGPT into their topology optimization workflows?
Thank you, Adam! To get started with ChatGPT, interested individuals and companies can reach out to our team at [email protected] We provide guidance and support for integrating ChatGPT into existing topology optimization workflows and tailoring it to specific design requirements.
Thank you, Ethan! I will definitely reach out to your team to explore integrating ChatGPT into our topology optimization workflow.
I'm impressed by the potential of ChatGPT! Ethan, what are some of the practical benefits or success stories your clients have experienced after adopting ChatGPT for topology optimization?
Great question, Lily! Our clients have reported significant improvements in the designs' structural performance, reduced material usage, and shortened design cycles. By leveraging the AI-powered capabilities of ChatGPT, they achieved better results in terms of both efficiency and effectiveness.
That's impressive, Ethan! The reported benefits sound very promising for industries that heavily rely on efficient and optimized designs.
Ethan, I'm curious about the scalability of using ChatGPT for large-scale or complex topology optimization problems. Have you encountered any limitations in terms of problem size or complexity?
Good point, Oscar! While ChatGPT can handle various problem sizes and complexities, there are practical limitations when dealing with extremely large-scale problems or cases with many nuanced design constraints. However, advancements in AI and optimization algorithms continue to push these boundaries.
Ethan, as an AI enthusiast, I'm curious about the underlying AI model used in ChatGPT. Can you give us some insights into the architecture or methodology behind it?
Absolutely, Elijah! ChatGPT is powered by the GPT (Generative Pre-trained Transformer) architecture. It employs a transformer model that is trained on a vast amount of text data to predict and generate coherent responses. This architecture allows ChatGPT to understand and generate text in a conversational manner.
Ethan, your article has indeed sparked my interest! Are there any resources or documentation available for developers who want to explore AI-powered topology optimization further?
Certainly, Sarah! Our website offers comprehensive resources, including technical documentation, code examples, and tutorials, to support developers in exploring AI-powered topology optimization and integrating ChatGPT into their workflows. Feel free to visit our website for more information!
Moreover, ChatGPT's ability to generate creative and out-of-the-box designs can lead to innovative solutions that may have been overlooked by conventional optimization methods.
Ethan, can ChatGPT assist in addressing multi-objective optimization problems by considering trade-offs between conflicting objectives?
Absolutely, Matthew! ChatGPT is designed to handle multi-objective optimization problems. It can suggest design modifications that provide trade-offs between conflicting objectives, helping engineers make informed decisions based on their priorities and constraints.
By interacting with ChatGPT, designers can explore the design space and understand the trade-offs between, for example, weight reduction and structural integrity or cooling efficiency and pressure drop.