Enhancing User Behavior Prediction in Social Network Analysis with ChatGPT
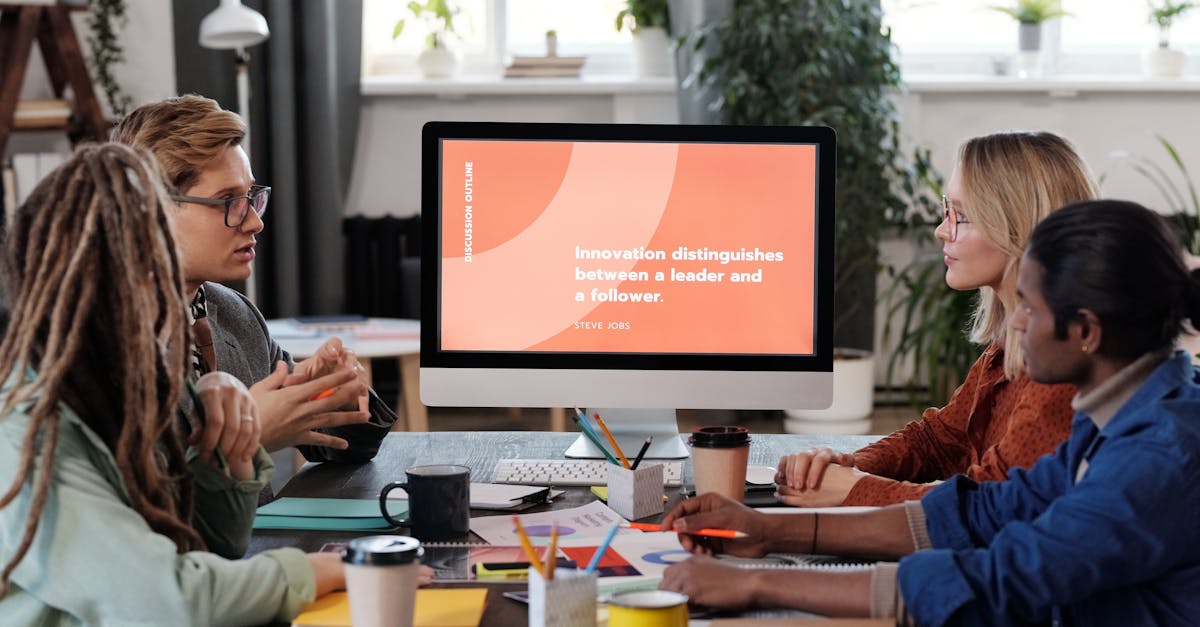
Social Network Analysis (SNA) is a technology that focuses on analyzing social interactions and relationships among individuals or groups. In recent years, SNA has gained popularity in predicting user behavior on various social media platforms. By applying machine learning algorithms, SNA can provide valuable insights to marketers, helping them understand and predict user actions.
Understanding Social Network Analysis
Social Network Analysis involves the collection, analysis, and interpretation of data to study how individuals or organizations are connected within a social network. It explores the relationships between entities and identifies patterns to gain insights into human behavior. In the context of predicting user behavior, SNA plays a crucial role in interpreting social media data to understand user preferences, interests, and interactions.
Prediction of User Behavior
One of the primary applications of Social Network Analysis is the prediction of user behavior. Marketers can leverage SNA to analyze user interactions, identify influencers, and predict user actions such as purchasing decisions, content engagement, or even social behavior. By understanding the underlying relationships and patterns within a social network, marketers can tailor their strategies, campaigns, and offerings to better target their audience.
Applying Machine Learning Algorithms
Machine learning algorithms play a vital role in predicting user behavior using SNA. By analyzing vast amounts of social media data, these algorithms can uncover hidden patterns and correlations. Some common machine learning techniques include classification, clustering, and regression. These algorithms learn from historical data to make predictions about future user behavior.
For example, with classification algorithms, marketers can predict whether a user is likely to purchase a specific product based on their past behavior, interests, or social connections. Clustering algorithms can group users based on similar characteristics, allowing marketers to create targeted advertising campaigns. Regression algorithms can be used to forecast user engagement metrics, such as the number of likes, shares, or comments a particular post will receive.
Valuable Insights for Marketers
The insights provided by SNA and machine learning algorithms are invaluable for marketers. By accurately predicting user behavior, marketers can optimize their marketing strategies, enhance customer segmentation, and personalize content to improve customer engagement and conversion rates.
Additionally, SNA can help marketers identify and leverage influential users within a social network. By targeting these influencers, marketers can spread their brand messages effectively and organically, reaching a wider audience.
Conclusion
Social Network Analysis, in combination with machine learning algorithms, has revolutionized the way marketers predict and understand user behavior. The ability to analyze social interactions, identify patterns, and make accurate predictions allows marketers to optimize their strategies and improve overall campaign performance. With the rapid growth of social media platforms and the increasing reliance on digital marketing, SNA has become an essential tool for marketers seeking to stay ahead in the competitive landscape.
Comments:
Thank you everyone for your interest in my article 'Enhancing User Behavior Prediction in Social Network Analysis with ChatGPT'. I'm excited to join this discussion and answer any questions you may have!
Great article, Jeff Snook! I found your research on ChatGPT intriguing. Can you highlight any potential limitations of using ChatGPT for user behavior prediction?
Thank you, Sarah Roberts, for your kind words. When it comes to limitations, one important aspect to consider is the potential bias present in the training data used to train ChatGPT. Bias in the data could lead to biased predictions or reinforce existing biases in user behavior. Additionally, ChatGPT's responses are generated based on patterns in the training data, so it may not always provide accurate predictions for novel situations.
Jeff Snook, your article was a fascinating read. I'm curious, can ChatGPT be used to predict user behavior in online shopping platforms? If so, how accurate is it compared to other methods?
Thank you, Adam Thompson, for your interest. Yes, ChatGPT can be applied to predict user behavior in online shopping platforms. Its natural language processing capabilities allow it to analyze user conversations and interactions, providing insights for behavior prediction. In terms of accuracy, ChatGPT performs well compared to other methods, but its effectiveness ultimately depends on the quality and relevance of the training data used.
Hi Jeff Snook! Your article presents a fascinating application of ChatGPT. Are there any ethical concerns associated with using AI models like ChatGPT for user behavior prediction in social network analysis?
Good question, Emily Adams! Ethical concerns do arise when using AI models like ChatGPT for user behavior prediction. The potential for privacy invasion, data misuse, and the reinforcement of harmful biases are important considerations. It's crucial to ensure responsible and transparent use of such technologies to mitigate these ethical concerns.
Jeff Snook, your article shed light on the potential of ChatGPT. How can businesses leverage this technology to enhance their social media marketing strategies?
Thank you, Michael Cooper! Businesses can leverage ChatGPT to enhance their social media marketing strategies by analyzing user conversations and interactions to gain insights into their preferences, interests, and needs. This information can inform targeted advertising, personalized recommendations, and tailored customer support to optimize social media marketing efforts.
Great article, Jeff Snook! I'm curious about the computational requirements for implementing ChatGPT for user behavior prediction. Is it resource-intensive?
Thank you, Lisa Johnson! Implementing ChatGPT can indeed be resource-intensive, especially for large-scale social network analysis. Training the model requires substantial computational power, and the inference phase may also require GPU accelerators for efficient processing. However, with advancements in hardware and distributed computing, these computational requirements can be managed effectively.
Jeff Snook, your article opened up exciting possibilities. How would you handle cases where user behavior is highly unpredictable or influenced by external factors?
Thank you, David Smith! Handling highly unpredictable or externally influenced user behavior can be challenging. In such cases, it's important to continuously update and adapt the training data used for ChatGPT, incorporating recent data to capture evolving trends and dynamics. Additionally, integrating external factors like current events, trends, or market conditions can help improve the accuracy of behavior predictions.
Jeff Snook, I really enjoyed your article. What are the key prerequisites for successfully implementing ChatGPT for user behavior prediction in social network analysis?
Thank you, Sophie Turner! Successful implementation of ChatGPT for user behavior prediction requires a few key prerequisites. Firstly, a diverse and representative training dataset is essential to ensure accuracy and minimize biases. Secondly, businesses must have proper data governance and ethical frameworks in place to address privacy concerns. Lastly, ongoing monitoring and evaluation of ChatGPT's predictions are necessary to fine-tune the model and address any shortcomings.
Jeff Snook, great work! What are the potential real-world applications for enhancing user behavior prediction through ChatGPT, apart from social network analysis?
Thank you, Mark Davis! ChatGPT's enhanced user behavior prediction can be applied in various real-world scenarios. Apart from social network analysis, it can assist in targeted advertising, content recommendation systems, fraud detection, customer support automation, and personalized user experiences across different domains such as e-commerce, finance, and healthcare.
Jeff Snook, your article was enlightening. Are there any potential concerns about the interpretability of ChatGPT's predictions for user behavior?
Thank you, Olivia Anderson! Interpretability of ChatGPT's predictions is indeed a concern. Deep learning models like ChatGPT often lack interpretability as they operate as black boxes, making it challenging to understand the decision-making process. Addressing this concern requires exploring techniques like attention mechanisms, counterfactual explanations, and model-agnostic interpretability methods to shed light on the factors influencing the predictions.
Jeff Snook, I'm intrigued by your research. How do you see the future of ChatGPT and user behavior prediction evolving?
Thank you, Kevin Lee! The future of ChatGPT and user behavior prediction looks promising. As AI technologies advance, we can expect improvements in natural language processing and the ability to handle more complex social network analysis scenarios. Moreover, addressing ethical considerations, ensuring transparency, and building trustworthy AI systems will be crucial for widespread adoption and positive social impact.
Jeff Snook, thank you for your response! I appreciate your insights on the limitations of ChatGPT. It's essential to be aware of potential biases and limitations when implementing AI models in real-world applications.
Thank you for clarifying, Jeff Snook. Considering the potential of ChatGPT for user behavior prediction in online shopping platforms, I can see how it can revolutionize personalized marketing strategies.
The ethical concerns you raised, Jeff Snook, are important reminders. Striving for responsible and transparent use of AI models is crucial for building trust and ensuring positive societal outcomes.
Appreciate your insights, Jeff Snook. Leveraging ChatGPT for social media marketing strategies can undoubtedly help businesses better understand and cater to their customers' needs.
Understanding the computational requirements, as you mentioned, Jeff Snook, is vital for organizations planning to implement ChatGPT for user behavior prediction at scale.
Thank you for your response, Jeff Snook. Adapting training data and incorporating external factors seem like practical solutions to handle unpredictable user behavior.
Thanks for sharing the key prerequisites, Jeff Snook. Considering factors like diverse training data and ethical frameworks is crucial for successful deployment of AI models.
The real-world applications you've mentioned, Jeff Snook, highlight the versatility and potential impact of ChatGPT beyond social network analysis.
Addressing the interpretability concern, as you suggested Jeff Snook, will be key to gaining trust in AI models like ChatGPT and its predictions for user behavior.
Exciting to hear your insights, Jeff Snook. The future of ChatGPT and its role in user behavior prediction holds great promise for advancements in AI technologies.
Jeff Snook, I really appreciate the article you wrote. It raises interesting questions about the boundaries of AI assistance in social network analysis. What potential challenges do you see in implementing ChatGPT at scale?
Thank you, Paul Wilson! Implementing ChatGPT at scale does present challenges. One challenge is managing computational resources as the model's size and complexity increase, requiring significant computational power. Addressing biases in training data, ensuring model robustness, and integrating with existing infrastructure are additional challenges for large-scale deployment. However, continued research and advancements in AI engineering can help overcome these challenges.
Jeff Snook, your article was thought-provoking. Can ChatGPT be applied to individual user behavior prediction, such as personalized recommendations on e-commerce platforms?
Thank you, Linda Thompson! Yes, ChatGPT can indeed be applied to individual user behavior prediction. Its ability to analyze conversations and interactions can enable personalized recommendations on e-commerce platforms, improving the user experience and increasing customer engagement.
Hi Jeff Snook! Your research on ChatGPT's application to user behavior prediction is fascinating. Can it be used to identify potential security threats in social networks?
Thank you, Emma Roberts! Yes, ChatGPT can be utilized to identify potential security threats in social networks. By analyzing user conversations and interactions, it can detect suspicious or malicious activities, helping improve the security and safety of online social platforms.
Jeff Snook, your work on ChatGPT is impressive. How do you envision the collaboration between AI models like ChatGPT and human analysts in social network analysis?
Thank you, Daniel Brown! The collaboration between AI models like ChatGPT and human analysts is crucial. While AI models can assist in processing large volumes of data, identifying patterns, and generating insights, human analysts bring domain expertise, critical thinking, and context understanding to interpret the results. The collaboration aims to leverage the strengths of both, enhancing the effectiveness and accuracy of social network analysis.
Jeff Snook, your article intrigued me. How can we ensure the fairness and impartiality of ChatGPT's predictions, especially in diverse social networks?
Thank you, Sophia Johnson! Ensuring fairness and impartiality in ChatGPT's predictions is crucial. It requires using representative training data that captures diversity and addressing biases that may exist in the model. Regular audits, evaluation against fairness metrics, and involving diverse stakeholders in the training process can help identify and mitigate biases, ensuring more equitable predictions in diverse social networks.
Jeff Snook, your research broadens the possibilities of AI. Can ChatGPT be used to identify emerging trends or changes in user behavior within social networks?
Thank you, John Anderson! Yes, ChatGPT can be leveraged to identify emerging trends and changes in user behavior within social networks. By analyzing conversations and interactions, it can detect shifts in preferences, interests, or emerging patterns of behavior, providing valuable insights for monitoring and adapting strategies in real-time.
Jeff Snook, your article is insightful. How can ChatGPT's predictions be validated or verified in real-world scenarios?
Thank you, Sophie Davis! Validating and verifying ChatGPT's predictions in real-world scenarios is essential for building trust. Conducting A/B tests, comparing predicted and observed behavior, and obtaining feedback from users and domain experts are effective ways to evaluate the predictions. Continuous monitoring and refinement based on performance metrics help improve accuracy and reliability.
Jeff Snook, the potential applications of ChatGPT are exciting. Could it help predict user sentiment or emotional responses within social networks?
Thank you, Jasmine Wilson! ChatGPT can indeed be used to predict user sentiment or emotional responses within social networks. By analyzing conversations and interactions, it can identify patterns and cues indicative of different emotions, providing insights into the sentiment of users and helping gauge their emotional responses.
Jeff Snook, your article sparked my interest. How do you see the integration of AI models like ChatGPT with existing social network analysis tools and platforms?
Thank you, Jason Thompson! The integration of AI models like ChatGPT with existing social network analysis tools and platforms can enhance their capabilities. By leveraging the insights and predictions generated by ChatGPT, these tools can offer improved user behavior analysis, more accurate predictions, and better support for decision-making processes. Integration should focus on seamless data flow, efficient processing, and meaningful visualizations to maximize the value of AI in social network analysis.
Jeff Snook, your research is impressive. How can businesses ensure the privacy and security of user data when implementing ChatGPT for user behavior prediction?
Thank you, Ella Brown! Ensuring privacy and security of user data is of paramount importance when implementing ChatGPT or any AI system. Businesses should establish robust data governance policies, employ secure data storage and transmission practices, and follow privacy regulations. Adopting privacy-preserving techniques like differential privacy and providing transparent user consent and control over data usage can help safeguard user privacy while reaping the benefits of user behavior predictions.