Enhancing Visual Effects: Exploring Denoising Techniques with ChatGPT
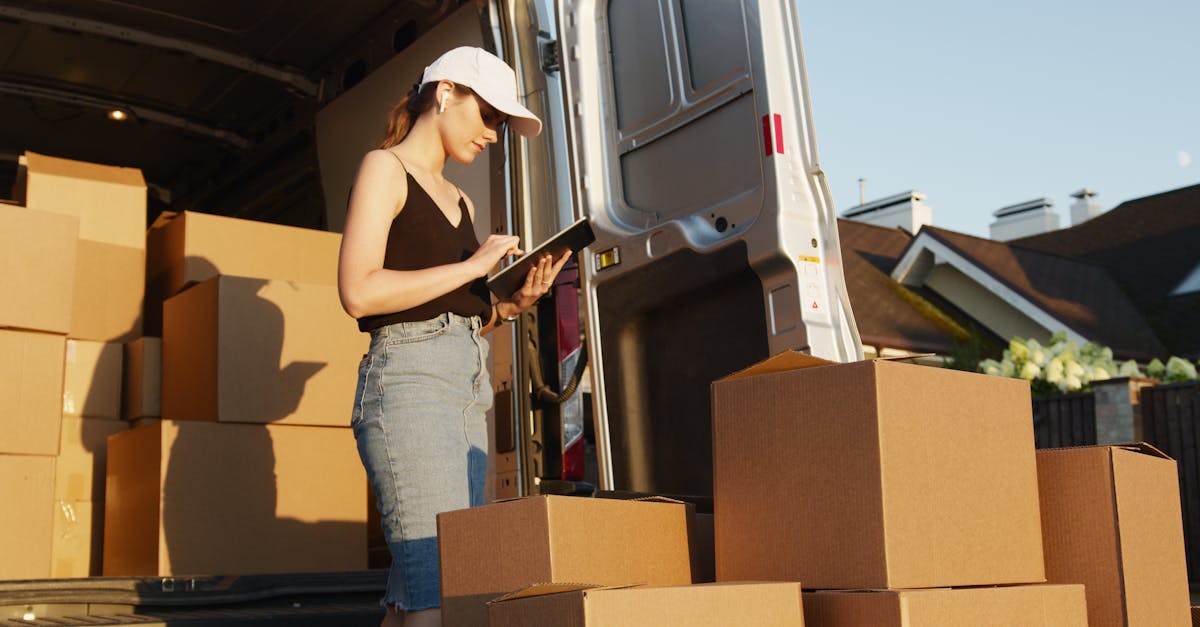
Visual effects play a significant role in enhancing the overall visual experience in various forms of media, including movies, video games, and virtual reality. One of the challenges in achieving high-quality visuals is removing noise or unwanted artifacts from the rendered images or videos. Denoising techniques have become essential in the visual effects industry to provide clearer visuals, and recent advancements in artificial intelligence have made it even more accessible.
Denoising Techniques
Denoising techniques aim to reduce or eliminate noise, which refers to random variations in brightness or color information present in images or videos. These variations can be caused by factors such as low-light conditions, sensor limitations, or compression algorithms. Traditional denoising methods involve applying filters or statistical algorithms to smooth out the noise while preserving the desired details.
With the advent of deep learning and artificial neural networks, advanced denoising techniques have emerged. These techniques leverage the power of machine learning to train models that can effectively denoise images or videos. The models are trained on large datasets that contain both clean and noisy samples, helping them learn the patterns and characteristics of noise as well as the corresponding clean images. Once trained, these models can be used to denoise new images or videos by inputting the noisy data and obtaining a cleaner version as output.
ChatGPT-4 and Advanced Denoising
OpenAI's ChatGPT-4 is an AI language model that is designed to provide intelligent responses and suggestions. While primarily focused on natural language processing, ChatGPT-4 also possesses the capability to understand and assist with visual-related tasks, such as denoising. By leveraging its advanced understanding of visual effects and deep learning algorithms, ChatGPT-4 can suggest advanced denoising techniques for achieving clearer visuals in images or videos.
Imagine a scenario where a visual effects artist is working on a scene for a movie, but the rendered images appear noisy due to certain rendering parameters or limitations. The artist can simply interact with ChatGPT-4, describe the issue, and seek its assistance. ChatGPT-4 would analyze the problem and provide suggestions on specific denoising techniques that could be applied to improve the visual quality. These suggestions could include using specific deep learning models or fine-tuning existing ones to align with the characteristics of the noisy data.
The ability of ChatGPT-4 to understand the context and intricacies of denoising techniques enables it to provide tailored solutions based on the particular requirements of the visual effects artist. It can recommend denoising algorithms or even generate code snippets to simplify and accelerate the implementation process. By providing instant and accurate guidance, ChatGPT-4 empowers visual effects artists to create visually stunning outputs with reduced noise and enhanced overall quality.
Conclusion
Visual effects are a powerful tool that significantly impacts the quality of media content. Denoising techniques, both traditional and advanced, play a crucial role in enhancing the visual experience by reducing noise and artifacts. With the introduction of AI models like ChatGPT-4, denoising has become more accessible and convenient, providing artists and designers with intelligent suggestions tailored to their specific needs. As technology continues to advance, we can expect even more exciting developments in visual effects and denoising techniques.
Comments:
Thank you all for reading my article on enhancing visual effects with ChatGPT! I'd love to hear your thoughts and opinions.
Yes, I found it very informative as well. John did a great job explaining the different denoising techniques.
Great article, John! I found the explanation of denoising techniques to be really helpful.
I agree, Michael! The article was well-written and easy to understand.
I've been looking for ways to improve visual effects in my projects. This article came just at the right time!
I'm glad to hear that, Sophia! Let me know if you have any specific questions or need further clarification on any technique.
Thank you, John! I'll give those a try.
I found the article to be quite technical. It might be helpful to include some practical examples or case studies.
Thank you for the feedback, David! Including practical examples is a great suggestion. I'll make sure to include them in future articles.
I have a question about one of the denoising algorithms mentioned. Can you explain more about how it works, John?
Of course, Sam! Which algorithm are you referring to?
That makes sense. Thanks for clarifying, John!
The article was really interesting! I never knew there were so many denoising techniques available.
I'm a beginner in visual effects, and this article provided a good introduction to denoising. Thanks, John!
I have some experience with denoising, and it's always interesting to learn about new techniques. Thanks for sharing, John.
The article was detailed and well-researched. It's evident that you put a lot of effort into it, John.
I'm specifically curious about the non-local means algorithm.
The non-local means algorithm compares similar patches in the image to estimate the value for each patch. It takes advantage of the redundancy present in natural images to remove noise effectively.
I'm impressed with the advancements in denoising techniques. They really help to enhance the visual quality of images and videos.
I'm curious, John, do these denoising techniques have any limitations or trade-offs?
Great question, Brian! While denoising techniques can improve visual quality, they can also introduce artifacts or blur in some cases. It's important to find the right balance based on the specific application.
I liked the comparison of different denoising techniques in the article. It helped in understanding their strengths and weaknesses.
Are there any denoising techniques that are more suitable for certain types of images or videos?
Yes, Adam! Some techniques like wavelet denoising are more effective for images with high-frequency noise, while others like bilateral filtering work well for color images with structured noise.
That's good to know! I'll keep that in mind when working on different types of projects.
I would love to see some sample code or implementation examples for these denoising techniques.
Thanks for the suggestion, Sophie! I'll consider including code snippets or references to implementation examples in future articles.
I appreciate the explanations of the mathematical concepts behind the denoising techniques. It really adds depth to the article.
The article was well-structured, making it easy to follow along and understand the different techniques.
I'm excited to try out some of these denoising techniques in my upcoming projects. Thanks for sharing, John!
I've been struggling with noise in my images. This article has given me some valuable insights. Much appreciated, John!
John, have you personally used these denoising techniques in any of your projects?
Yes, Liam! I have employed various denoising techniques in several visual effects projects, and they have significantly improved the quality of the final output.
It would have been helpful to include some visual examples illustrating the differences between noisy and denoised images.
That's a valid point, Emma. Including visual examples would definitely enhance the understanding of the denoising techniques. I'll make sure to include them in future articles.
I enjoyed reading the article, John! It was both educational and engaging.
John, which denoising technique would you recommend for beginners to start with?
For beginners, I would suggest starting with basic techniques like median filtering or simple averaging. They are easy to implement and provide decent results.
John, are there any denoising techniques that can handle real-time applications or video processing effectively?
Absolutely, Daniel! Techniques like temporal filtering and block-matching algorithms are commonly used for real-time applications and video denoising.
This article has inspired me to explore denoising techniques further. Thanks for sharing your knowledge, John!
John, have you come across any denoising techniques that are specifically designed for deep learning applications?
Yes, Jacob! Deep learning-based techniques like autoencoders and convolutional neural networks often provide state-of-the-art denoising performance.
That's interesting! I'm interested in exploring the applications of deep learning in denoising.
John, do these denoising techniques only apply to images, or can they also be used for video denoising?
Great question, Karen! While some techniques are tailored specifically for images, many of them can be extended to handle video denoising as well.
That's good to know! It's fascinating how these techniques can be applied to various multimedia tasks.
I appreciate the practical tips and recommendations provided alongside each denoising technique. It's helpful for implementing them in real-world scenarios.
The article was well-referenced, allowing readers to explore further on specific denoising techniques.
Thank you all for your valuable feedback and comments! I'm glad you found the article informative. Stay tuned for more articles on visual effects techniques!