Enhancing WiFi Efficiency: Harnessing ChatGPT for Data Usage Monitoring in Wireless Networks
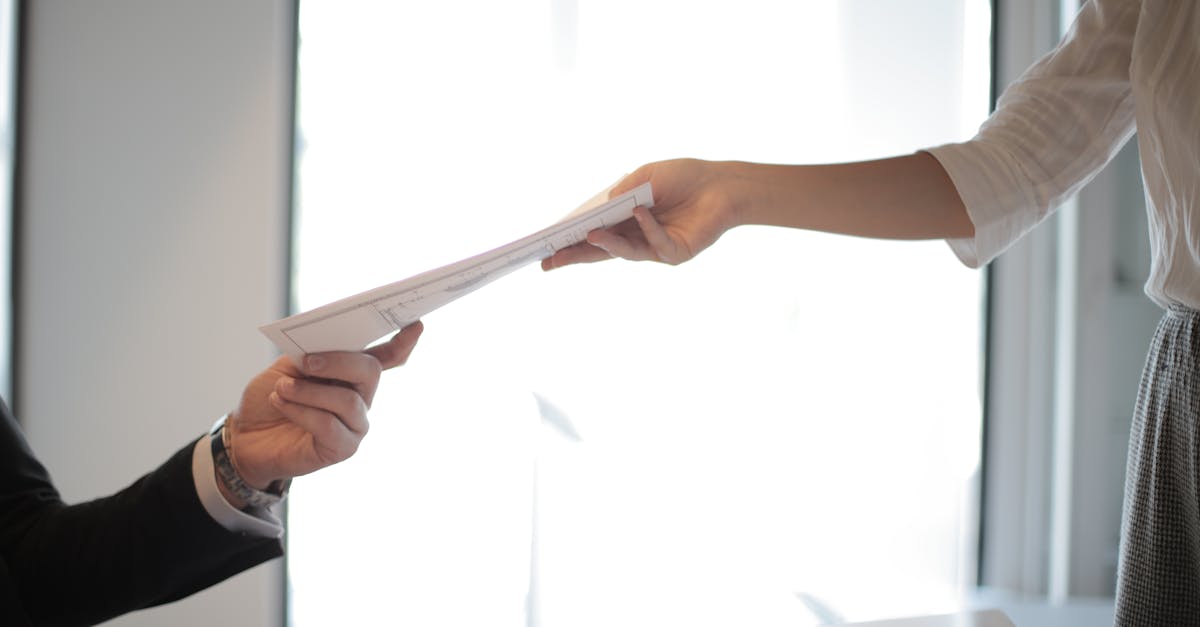
With the increasing dependence on WiFi for our day-to-day activities, it is crucial to have a proper understanding of our data usage in order to optimize network costs. WiFi data usage monitoring technology provides invaluable insights into how our devices utilize data, allowing us to make informed decisions and potentially save money.
The Importance of Data Usage Monitoring
Whether you are an individual, a small business, or a large enterprise, monitoring data usage is essential to understanding how much data your devices consume. Data usage monitoring technology can help you keep track of the amount of data each device consumes, identify heavy data users, and be aware of any unusual spikes in usage.
By having a clear understanding of your data consumption patterns, you can make informed decisions on the allocation of your network resources. This can help you optimize network costs by identifying areas where data consumption can be reduced or where additional resources may be required.
Insights from WiFi Data Usage Monitoring
WiFi data usage monitoring provides detailed insights into your network's data consumption, allowing you to make data-driven decisions. Some of the key insights that can be gained include:
- Usage Patterns: Data usage monitoring technology provides information about when and how data is being utilized. This can help identify peak usage periods and areas of high data consumption, allowing you to allocate resources more efficiently.
- Device-Specific Consumption: Monitoring data usage on a device-level basis enables you to identify which devices consume the most data. This information can be valuable in identifying potential data-hogging devices and taking appropriate actions to optimize their data usage.
- Application Usage: Understanding which applications consume the most data can help identify areas where data consumption can be reduced or optimized. This can lead to cost savings by identifying applications that may not be essential or finding alternatives that consume less data.
- Historical Data Trends: Analyzing historical data usage patterns can help identify long-term trends and make predictions about future data consumption. This information can be critical for capacity planning and ensuring that your network can handle future growth without incurring additional costs.
Cost Optimization with WiFi Data Usage Monitoring
By leveraging the insights provided by WiFi data usage monitoring technology, you can take proactive steps to optimize network costs. Here are some strategies that can be implemented based on the insights gained:
- Data Usage Policies: Implementing data usage policies based on the information gathered can help regulate data consumption and ensure that resources are allocated efficiently.
- Device Management: Identifying and managing devices that consume excessive data can help optimize their usage and reduce unnecessary costs.
- Application Optimization: By understanding which applications consume the most data, you can explore alternatives or consider implementing measures to optimize their data usage.
- Capacity Planning: Using historical data trends, you can plan for future growth and avoid over-provisioning or under-provisioning of your network capacity, ultimately saving costs.
WiFi data usage monitoring provides the necessary insights to actively manage network costs and ensure efficient resource allocation. By making data-driven decisions and implementing appropriate strategies, you can optimize network costs and make the most out of your WiFi network.
In Conclusion
WiFi data usage monitoring technology plays a vital role in providing insights into data usage and optimizing network costs. By monitoring data usage patterns, identifying heavy data users, and understanding application-specific consumption, you can take proactive steps to optimize network resources and reduce costs.
Take advantage of WiFi data usage monitoring technology to gain valuable insights into your network's data consumption. Optimize your network costs and ensure efficient resource allocation by implementing data-driven strategies based on the insights provided.
Comments:
Thank you all for visiting my blog post on enhancing WiFi efficiency! I hope you find the information useful.
Great article, Remi! The use of ChatGPT for data usage monitoring in wireless networks sounds promising. Are there any specific use cases you can share?
Hi Alice! Thank you for your comment. Absolutely, ChatGPT can be used for various use cases, such as detecting abnormal data usage patterns, identifying bandwidth-hogging applications, and optimizing network configurations to enhance WiFi performance.
This is fascinating, Remi! I can see how ChatGPT can make data usage monitoring more efficient. How does it handle real-time monitoring and alerts?
Hi Bob! Thanks for your interest. ChatGPT can continuously analyze data usage patterns in real-time. It can generate alerts based on thresholds set by administrators, enabling proactive identification of potential issues and facilitating prompt actions for efficient management.
This technology holds great potential for businesses and organizations. Remi, do you have any recommendations on implementing ChatGPT for data usage monitoring?
Hi Carol! Absolutely, implementing ChatGPT for data usage monitoring requires considering a few key points. It is essential to have a robust network infrastructure, adequate computational resources, and scalable data storage. Additionally, defining clear monitoring objectives and fine-tuning the model based on specific network characteristics can further enhance its effectiveness.
What are the potential limitations of using ChatGPT for data usage monitoring? How accurate can it be?
Hi David! While ChatGPT offers promising results, it may have certain limitations. It heavily relies on training data and might struggle with uncommon or evolving network patterns. Accuracy can vary based on the quality and size of training data, so continuous data updates and model validation are vital to maintain accuracy levels.
I'm curious to know about the implementation complexity. Is it a complex process to integrate ChatGPT into existing wireless networks?
Hi Eva! Implementing ChatGPT into existing wireless networks may require some initial effort. Significant dependencies include data pre-processing, model training, and fine-tuning for specific network characteristics. However, there are libraries and frameworks available that can simplify the integration process, making it more accessible for network administrators.
Excellent article, Remi! This technology could revolutionize the way we monitor and optimize data usage in wireless networks. I'm excited to see its potential unfold in the near future!
Thank you, Frank! I share your excitement. The application of ChatGPT for data usage monitoring indeed holds promise, and I believe it can greatly contribute to improving WiFi efficiency and user experience.
I'd love to know more about the computational resources required for implementing ChatGPT. Does it demand significant processing power?
Hi Grace! ChatGPT does require a substantial amount of computational resources, especially during the training phase. However, after the initial training, the inference process is less resource-intensive, making it more efficient for real-time data usage monitoring in wireless networks.
What are some potential challenges that network administrators might face in implementing ChatGPT?
Hi Hannah! Network administrators may face challenges regarding data privacy, model interpretability, and system integration. Privacy concerns are paramount, and it's important to ensure user data remains confidential. Additionally, understanding and interpreting model outputs for decision-making is crucial. Lastly, integrating ChatGPT into existing network infrastructure and workflows may require careful planning and coordination.
I'm curious about the scalability of ChatGPT for large-scale wireless networks. Can it handle networks with thousands of connected devices?
Hi Isaac! ChatGPT's scalability depends on the computational resources available. With sufficient resources, it can handle large-scale wireless networks effectively. However, it's crucial to ensure the infrastructure supporting ChatGPT is scalable to accommodate the increasing number of connected devices and the growing network demands.
I have seen similar approaches using machine learning for WiFi optimization. What sets ChatGPT apart from other methods?
Hi Jack! One significant aspect of ChatGPT is its conversational nature, allowing network administrators to obtain actionable insights and recommendations through an interactive interface. The ability to communicate with the model can provide a more intuitive approach to understand and address data usage issues, making it stand out among other machine learning methods.
I'm concerned about potential biases in data usage monitoring when employing a language model like ChatGPT. How can we address this issue?
Hi Karen! Biases can indeed exist when using a language model like ChatGPT for data usage monitoring. It's important to incorporate diverse and representative training data that considers various demographics and network usage patterns to minimize biases. Regular evaluation and validation of the model's outputs can help in detecting and mitigating any potential biases during the monitoring process.
Impressive article, Remi! I'm wondering if ChatGPT can adapt to different network environments and configurations?
Hi Lisa! Absolutely, ChatGPT can adapt to different network environments and configurations. Through fine-tuning and customization based on specific network characteristics, it can provide accurate and tailored insights for diverse wireless network setups, ensuring efficient data usage monitoring and optimization.
How can ChatGPT assist in identifying network security threats related to data usage?
Hi Michael! ChatGPT can help in identifying network security threats related to data usage by monitoring abnormal usage patterns and detecting potential malicious activities. By continuously analyzing data flows, it can assist in identifying suspicious behaviors and alerting network administrators for further investigation and mitigation.
Considering the evolving nature of network technologies, how adaptable is ChatGPT for future advancements?
Hi Nancy! ChatGPT's adaptability relies on regular updates and retraining with new data to keep up with the evolving network technologies. By incorporating new training data that reflects the latest advancements, the model can continue to provide accurate insights and recommendations, ensuring its relevance and applicability for future wireless network advancements.
I'm curious about the model's chat interface. How intuitive is it for network administrators to interact with ChatGPT?
Hi Oliver! The chat interface is designed to be intuitive for network administrators. It enables natural language conversations where administrators can ask questions, provide prompts, or seek recommendations related to data usage monitoring in wireless networks. This interactive approach makes it easy to seek insights and take appropriate actions based on the model's responses.
What kind of computational infrastructure is needed to support ChatGPT's training and deployment in wireless networks?
Hi Pamela! To support ChatGPT's training and deployment, a robust computational infrastructure is essential. High-performance computing resources, including GPUs or TPUs, are usually required for efficient model training. Additionally, reliable servers or cloud platforms are needed to deploy the trained model for inference and real-time data usage monitoring in wireless networks.
How can network administrators ensure the accuracy and reliability of data usage insights provided by ChatGPT?
Hi Quincy! Ensuring accuracy and reliability starts with comprehensive training data that covers a wide range of network scenarios. Regular model validation against ground truth data is crucial to maintain accuracy. Incorporating feedback loops where administrators verify and provide input on the model's outputs can also help improve reliability over time, making data usage insights from ChatGPT more trustworthy.
I'm curious about the potential impact on energy consumption when using ChatGPT for real-time data usage monitoring in wireless networks.
Hi Robert! Real-time data usage monitoring using ChatGPT can involve additional computational processes, which may impact energy consumption. However, efficient hardware utilization and optimized model architectures can mitigate this impact. Innovative energy-saving techniques and utilization of low-power hardware can be explored to balance accuracy and energy efficiency in data usage monitoring.
Are there any potential disadvantages of integrating ChatGPT into wireless networks?
Hi Samantha! While there are potential benefits, some disadvantages should be considered. One is the initial setup and integration effort required, as it may involve changes to existing network infrastructure and workflows. Additionally, there may be a learning curve for network administrators to effectively use the chat interface and interpret the model's responses. Regular updates and maintenance are also necessary to address evolving network patterns and challenges.
Can ChatGPT help identify network congestion issues and optimize traffic flow in wireless networks?
Hi Sara! ChatGPT can aid in identifying network congestion issues by analyzing data usage patterns and providing insights on potential bottlenecks. By understanding the traffic flow dynamics, network administrators can optimize routing, adjust bandwidth allocations, or apply Quality of Service (QoS) measures to alleviate congestion and improve wireless network performance.
In terms of data privacy, what precautions should be taken when implementing ChatGPT for data usage monitoring?
Hi Thomas! Data privacy is crucial when implementing ChatGPT. It's essential to ensure user data is protected and confidential. Anonymization techniques, appropriate access controls, and encryption can be utilized to safeguard user privacy. Complying with relevant data protection regulations and obtaining informed consent from users is also necessary to maintain ethical data usage practices.
What kind of data sources does ChatGPT rely on for data usage monitoring in wireless networks?
Hi Victoria! ChatGPT can utilize various data sources for data usage monitoring in wireless networks. These may include network flow records, packet captures, device metadata, communication protocols, and application-level data. Integration with network monitoring tools and network device APIs can provide a comprehensive data source for ChatGPT's analysis and insights.
Can ChatGPT handle unique network configurations and unconventional wireless network setups effectively?
Hi William! ChatGPT's effectiveness in handling unique network configurations relies on adequate training data that covers diverse network scenarios. By incorporating such data, it can adapt and provide insights for unconventional wireless network setups. However, it's important to fine-tune the model according to specific network characteristics to maximize its effectiveness in such cases.
Are there any potential security risks when deploying ChatGPT for data usage monitoring in wireless networks?
Hi Xavier! Deploying ChatGPT for data usage monitoring should consider potential security risks. Adversarial attacks targeting the model, model poisoning, or unauthorized system access can pose risks. Employing robust cybersecurity practices, ensuring secure model deployment, and regularly updating security measures can help mitigate these risks and safeguard the wireless network environment.
Can ChatGPT be trained to detect and adapt to changing network environments, such as dynamic network topologies?
Hi Yvonne! With sufficient training data that covers dynamic network topologies, ChatGPT can detect and adapt to changing network environments effectively. By incorporating temporal aspects and training the model with data reflecting varying network topologies, it can provide accurate insights and recommendations for optimizing data usage in dynamic wireless network scenarios.