Enhancing Yield Prediction in Plant Breeding: Harnessing the Power of ChatGPT
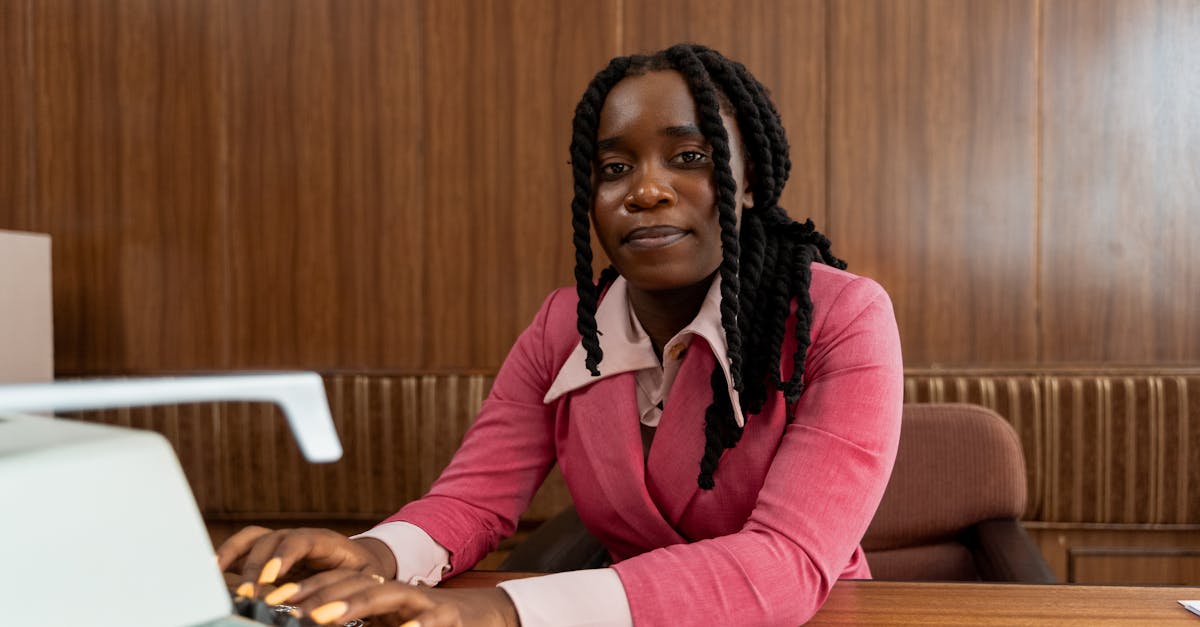
Introduction
Plant breeding is a technology that aims to improve the genetic traits of crops to enhance their productivity, quality, and resistance to various stresses. One of the significant challenges in agriculture is predicting the potential yield of crops accurately. Yield prediction plays a crucial role in decision-making for farmers, researchers, and policymakers. By utilizing historical data and advanced methodologies, plant breeders can now predict the probable yield of crops, leading to better planning and management strategies for maximizing agricultural productivity.
The Role of Yield Prediction
Yield prediction using plant breeding techniques allows breeders and agriculturists to estimate the potential harvest of a specific crop. This prediction involves analyzing various factors such as genetic traits, environmental conditions, disease prevalence, and agronomic practices. By integrating these data points, plant breeders can forecast the yield of a particular crop variety with a reasonable level of accuracy. This information is vital for farmers to plan their cultivation practices, decide on optimal planting dates, optimize fertilizer application, and make informed decisions regarding crop rotations.
Historical Data and Analysis
Yield prediction relies heavily on historical data, which includes information on past crop yields, soil characteristics, weather patterns, and management practices. By analyzing these datasets, plant breeders can identify patterns and correlations that impact crop productivity. With the help of advanced statistical models and machine learning algorithms, historical data can be processed to develop predictive models that accurately estimate future yields. The more comprehensive and reliable the historical data is, the more accurate the yield prediction becomes.
Advancements in Technology
Technological advancements have significantly improved the field of plant breeding and yield prediction. Traditional breeding methods, such as phenotypic selection, have been combined with modern tools like molecular markers, genomics, and bioinformatics. These technologies enable breeders to identify crop varieties with desirable traits more efficiently and accurately. Additionally, remote sensing techniques, such as satellite imagery and drones, provide valuable data on crop growth, disease prevalence, and stress conditions, which further enhances the accuracy of yield prediction models.
Usage and Benefits
The usage of yield prediction in plant breeding offers numerous benefits to various stakeholders in the agricultural sector. Farmers can make informed decisions regarding crop choices, planting strategies, resource allocation, and marketing plans based on predicted yields. For researchers, yield prediction allows for more targeted experimentation and efficient utilization of resources. Policymakers can also leverage yield prediction models to plan for food security, estimate production capacity, and design policies to support sustainable farming practices. Ultimately, the accurate estimation of crop yields contributes to improved agricultural productivity, reduced wastage, and increased profitability for farmers.
Conclusion
Plant breeding and yield prediction provide powerful tools to enhance agricultural productivity. By leveraging historical data and advanced technologies, breeders and agriculturists can predict the probable yield of crops, enabling effective decision-making and resource utilization. The accurate estimation of crop yields has far-reaching benefits for farmers, researchers, and policymakers, facilitating sustainable farming practices and ensuring food security.
Comments:
Thank you all for taking the time to read my article on enhancing yield prediction in plant breeding using ChatGPT. I'm excited to hear your thoughts and answer any questions you may have!
Great article, Je'quan! I found the concept of using ChatGPT for yield prediction in plant breeding fascinating. It opens up a whole new realm of possibilities. However, would this model work effectively with diverse plant species?
Thank you, Rachel! That's a valid question. While ChatGPT has been primarily trained on text data, it has shown promising results across various domains. However, it's essential to adapt and fine-tune the model to specific plant species to optimize its effectiveness.
Interesting read, Je'quan! I appreciate the potential of ChatGPT in streamlining and enhancing yield prediction in plant breeding. Do you think incorporating other data sources, like weather patterns or soil composition, could further improve the accuracy?
Thank you, Mitchell! Absolutely, integrating additional data sources such as weather patterns, soil composition, and even historical crop data can improve the accuracy of yield prediction models. ChatGPT can effectively incorporate these factors when provided during fine-tuning.
Great article, Je'quan! I think leveraging artificial intelligence models like ChatGPT to enhance yield prediction in plant breeding is a significant step forward. It holds immense potential for increasing agricultural productivity. However, what are the challenges in obtaining reliable and diverse training data for this model?
Thank you, Sophie! You bring up an important point. Obtaining reliable and diverse training data can be challenging. Collecting data from multiple sources, ensuring data quality, and covering various plant species, geographical regions, and environmental conditions are key challenges that need to be addressed to effectively train ChatGPT for yield prediction.
Je'quan, really enjoyed your article! I can see how ChatGPT can be a game-changer in plant breeding. However, what are the potential limitations of using this model? Are there any ethical concerns we should be aware of?
Thank you, Daniel! It's crucial to consider the limitations. ChatGPT may sometimes generate plausible-sounding but incorrect or misleading responses. Ethical concerns regarding bias in the training data and potential misuse of AI models are also important. It's essential to continuously refine and validate the model's outputs and be transparent about its limitations.
Great article, Je'quan! I enjoyed reading about the fusion of plant breeding and AI. I wonder, can ChatGPT also provide insights on optimal breeding techniques or just focus on yield prediction?
Thank you, Emily! ChatGPT can indeed provide insights on optimal breeding techniques, taking into account the vast knowledge it has accumulated during training. It can analyze patterns, suggest improvements, and assist in decision-making for various aspects of plant breeding, including techniques and maximizing yields.
Je'quan, your article was informative and thought-provoking! ChatGPT's potential in yield prediction is exciting. However, how would you address concerns about the model's interpretability? Will breeders be able to understand the reasoning behind the predictions?
Thank you, Nathan! Interpretability is indeed crucial. While ChatGPT is not inherently designed for transparency, techniques like attention mapping and explanation methods can aid in understanding the model's decision-making process. Providing breeders with interpretable outputs is an area of active research to ensure trust and effective collaboration.
Fascinating article, Je'quan! ChatGPT's potential for boosting yield prediction is impressive. However, can this technology be accessible for small-scale farmers who may not have extensive technological resources?
Thank you, Olivia! Accessibility is an important consideration. While small-scale farmers may not have the same technological resources as large-scale operations, efforts can be made to adapt and simplify the use of ChatGPT for them, potentially through user-friendly interfaces, offline functionality, or collaboration with agricultural extension services.
Great article, Je'quan! Integrating AI into plant breeding shows great promise. I'm curious, how extensively has ChatGPT been tested in the field, and what are some real-world success stories?
Thank you, David! While ChatGPT is a relatively new model, it has been tested and shown promising results in various fields. For example, it has been utilized to improve yield predictions, disease diagnostics, and crop management decisions. Collaborative efforts with plant breeders and researchers have significantly contributed to its development and application in real-world scenarios.
Je'quan, your article was insightful! The potential of ChatGPT in plant breeding seems vast. However, regarding privacy concerns, how can we ensure that sensitive breeding data remains secure when using AI models like ChatGPT?
Thank you, Sophia! Excellent point about privacy. When using AI models, data security and privacy should be prioritized. Implementing robust data management practices, secure infrastructure, and adhering to applicable data protection regulations are essential. Additionally, exploring privacy-preserving techniques like federated learning can help mitigate privacy concerns while still benefiting from AI models.
Well done, Je'quan! ChatGPT holds significant potential for improving yield prediction in plant breeding. How can plant breeders ensure that ChatGPT is effectively applied and integrated into their existing workflows?
Thank you, Amy! Integration into existing workflows is key. Plant breeders can start by collaborating with AI experts to fine-tune ChatGPT to their specific needs and datasets. Conducting pilot studies, validating the model's outputs against domain knowledge, and gradually incorporating it into the breeding process can help ensure effective integration while leveraging the expertise of breeders.
Excellent article, Je'quan! The intersection of AI and plant breeding is a fascinating subject. However, I'm curious about the computational resources required to train and deploy ChatGPT. Could this pose challenges for smaller research organizations?
Thank you, Benjamin! Computational resources can indeed be a challenge. Training large AI models like ChatGPT typically require substantial resources. However, efforts are being made to optimize resource usage, develop more efficient architectures, and explore cloud-based solutions for smaller research organizations to mitigate these challenges.
Impressive work, Je'quan! The potential applications of ChatGPT in plant breeding are exciting. How can the wider scientific community contribute to improving and expanding the capabilities of ChatGPT for this field?
Thank you, Liam! Collaboration and contribution from the scientific community are invaluable. Researchers can further improve ChatGPT's capabilities for plant breeding by sharing diverse and high-quality datasets, conducting benchmarking studies, exploring interpretability techniques, and developing domain-specific fine-tuning strategies. Together, we can accelerate progress and unlock the full potential of AI in plant breeding.
Wonderful article, Je'quan! The use of ChatGPT in plant breeding holds immense possibilities. However, can ChatGPT be used to assess the impact of breeding practices on sustainability and environmental factors?
Thank you, Ella! Assessing the impact of breeding practices on sustainability and environment is crucial. While ChatGPT can't directly assess environmental factors, it can assist in decision-making processes by considering relevant parameters like resource utilization, disease resistance, and adaptability. Integrating environmental data with ChatGPT outputs can provide breeders valuable insights for more sustainable practices.
Je'quan, your article shed light on an exciting application of AI. How can we ensure that the knowledge gained from ChatGPT benefits breeders and researchers worldwide, especially those in low-resource regions?
Thank you, Hannah! Knowledge dissemination and accessibility are vital. Efforts can be made to share research findings, methodologies, and successful implementations widely. Open access publications, collaborative platforms, and partnerships with organizations working in low-resource regions can help ensure that breeders and researchers worldwide, regardless of their resources, can benefit from the knowledge gained through ChatGPT.
Je'quan, your article showcases the potential of AI in plant breeding. Are there any ongoing research initiatives or organizations actively working on leveraging ChatGPT for yield prediction and related tasks?
Thank you, Andrew! Several research initiatives and organizations are actively exploring the application of ChatGPT in plant breeding. Collaborations between academia and industry, as well as partnerships among research institutions worldwide, are contributing to advancements in this field. Open-source frameworks and shared resources further facilitate the adoption and development of AI models like ChatGPT in plant breeding.
Informative article, Je'quan! ChatGPT's potential for yield prediction is huge. However, how can breeders effectively compare the predictions of ChatGPT with traditional breeding methods to validate its effectiveness?
Thank you, Sophia! Validating ChatGPT's predictions against traditional breeding methods is important. Breeders can first establish benchmarks using historical data and known breeding outcomes. By comparing ChatGPT's predictions with these benchmarks and conducting rigorous field trials, they can assess and refine the model's effectiveness for their specific plant species, environments, and breeding objectives.
Je'quan, your article on using ChatGPT for yield prediction is intriguing. How long does it typically take to train and fine-tune ChatGPT for adequate performance in plant breeding?
Thank you, Grace! Training and fine-tuning ChatGPT can vary based on factors like dataset size, computational resources, and the desired performance. Larger models with extensive fine-tuning may require several days to weeks. However, specific optimizations and architectures can reduce training time. It's an ongoing area of research, focusing on improving both efficiency and performance in various domains, including plant breeding.
Great post, Je'quan! The combination of ChatGPT and plant breeding seems very promising. Could you elaborate on how the model learns and adapts to different plant species during the fine-tuning process?
Thank you, Leo! During fine-tuning, ChatGPT is trained on a target dataset specific to the plant species of interest. This dataset includes examples of yield data, breeding objectives, and other relevant factors. By continually adjusting its parameters based on the provided target dataset, ChatGPT learns to adapt and make predictions tailored to the specific plant species' characteristics, optimizing its performance for that particular domain.
Je'quan, your article on leveraging ChatGPT for yield prediction is fascinating. Is there a plan to make ChatGPT's source code and pre-trained models available to the public, promoting transparency and collaboration?
Thank you, Zoe! Openness and collaboration are vital. While the details and availability are constantly evolving, there's a growing trend towards openness in AI research. The developers of ChatGPT are actively exploring ways to provide public access to the models and source code, ensuring transparency, openness, and enabling the research community and breeders to benefit from and contribute to its development.
Je'quan, your article sheds light on an exciting approach to yield prediction. Considering the dynamic nature of the agricultural domain, how can ChatGPT be continuously updated to adapt to changing conditions and new breeding practices?
Thank you, Maxwell! Continuous updates and adaptation are indeed essential. By collecting and incorporating new data, breeders can periodically retrain ChatGPT, enabling it to adapt to changing conditions, emerging breeding practices, and new challenges. This iterative process of training and updating helps ensure that ChatGPT remains relevant and effective in plant breeding, reflecting the latest knowledge and advancements.
Je'quan, your article on using ChatGPT in plant breeding is compelling. How flexible is this model when forecasting yields for different time horizons, from near-term to long-term predictions?
Thank you, Isaac! ChatGPT can be flexible in forecasting yields for different time horizons. By providing the model with historical yield data across various time scales, it can learn to capture patterns and make predictions for both near-term and long-term horizons. However, breeders should validate and refine the model's outputs against real-world observations and specific contexts to ensure accuracy for their specific needs.
Fascinating article, Je'quan! The potential of ChatGPT in plant breeding is immense. As a breeder, how can one effectively integrate this technology into their existing workflow without disturbing the established practices and knowledge?
Thank you, Ruby! Integrating ChatGPT into existing workflows while preserving established practices and knowledge is essential. Adoption can be gradual, starting with small-scale pilot studies to assess effectiveness, validating outputs against traditional methods, and gradually integrating the model's insights into decision-making processes. Collaboration between breeders, researchers, and AI experts can ensure a smooth integration, aligning the technology with existing practices.
Je'quan, your article provides valuable insights into the integration of ChatGPT in plant breeding. Considering biases that may exist in training data, how can we ensure that the model's predictions are fair and unbiased?
Thank you, Aiden! Addressing biases is crucial. Efforts are being made to ensure that training data for ChatGPT is diverse, representative, and free from biases as much as possible. Additionally, during the fine-tuning process, breeders can carefully curate and validate the training data to further reduce biases. Constant monitoring, feedback loops, and accountability help mitigate bias and ensure the model's predictions remain fair and unbiased.
Great article, Je'quan! The potential application of ChatGPT in plant breeding is exciting. Can you elaborate on the data requirements and challenges associated with training ChatGPT models for yield prediction?
Thank you, Naomi! Data requirements and challenges are significant. ChatGPT benefits from large and diverse datasets encompassing aspects like historical yield data, breeding objectives, environmental factors, and genomic information. Challenges include data collection, quality assurance, data standardization, and ensuring representation of various plant species and environments. Collaborative efforts and data sharing among breeders, researchers, and organizations play a vital role in addressing these challenges and training effective models.
Thank you all for engaging in this discussion! Your insightful questions and thoughts help enrich the conversation and drive advancements in the field. Feel free to continue sharing your perspectives and I'll be glad to provide further insights and clarifications.