Expanding the Frontiers of Technology: Harnessing Gemini in the Microarray Domain
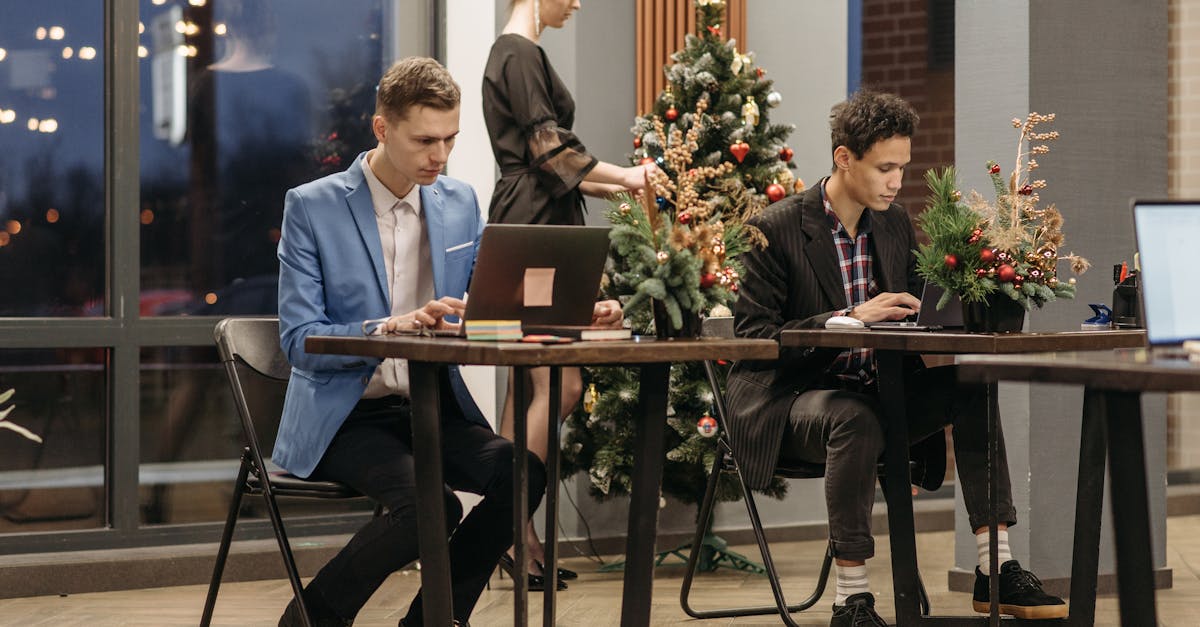
In recent years, the field of technology has witnessed remarkable advancements, pushing the boundaries of what was once thought possible. One such breakthrough is the development of Gemini, a state-of-the-art language model that has revolutionized natural language processing. While initially intended for conversation generation, the potential applications of this technology extend far beyond its original purpose.
The Microarray Domain
The microarray domain refers to the field of genetic research that involves analyzing the expression of thousands of genes simultaneously. Microarrays are powerful tools used to study the activity of genes and understand their role in various biological processes. They offer a high-throughput approach to gather large amounts of genetic data quickly, allowing researchers to perform extensive analyses and make significant discoveries.
Utilizing Gemini in the Microarray Domain
With its powerful language processing capabilities, Gemini can contribute substantially to the field of microarray analysis. By integrating Gemini with existing technologies and frameworks in the domain, researchers can leverage its ability to understand and generate human-like text to enhance their analytical processes.
One potential application of Gemini in the microarray domain is in the preprocessing and data cleaning phase. Microarray data often contains noise, outliers, and missing values, which can greatly affect the quality and reliability of subsequent analyses. By utilizing Gemini's language understanding capabilities, researchers can develop intelligent models to identify and handle these data issues effectively, streamlining the preprocessing workflow.
Furthermore, Gemini can also be employed in the interpretation and analysis of microarray results. After performing statistical analyses and generating a list of differentially expressed genes, researchers need to make sense of the obtained results, identify potential biological pathways, and uncover hidden relationships. Gemini can assist in this process by generating summaries, suggesting relevant literature, or providing additional insights that researchers might overlook.
The Future of Gemini in the Microarray Domain
The integration of Gemini and microarray analysis opens up numerous exciting possibilities for advancing research in genetics and molecular biology. As the technology continues to evolve, future developments may include the creation of intelligent chat-based systems that interact with researchers, answer queries, and assist in the design of experiments. These systems could save time, accelerate discoveries, and facilitate collaboration among scientists.
However, it is important to note that while Gemini demonstrates remarkable language processing capabilities, it is not a substitute for human expertise. The insights provided by Gemini should always be critically evaluated and validated by domain experts.
Conclusion
Expanding the frontiers of technology through the integration of Gemini in the microarray domain presents exciting prospects for genetic research. By harnessing Gemini's language understanding capabilities, researchers can enhance the preprocessing and interpretation phases of microarray analysis. As researchers continue to explore and leverage this technology, new opportunities for collaboration, discovery, and advancement in the field of genetics are within reach.
Comments:
This article presents an interesting application of Gemini in the microarray domain. It's fascinating to see how technology is being utilized to enhance the analysis of gene expression data.
I agree, Sarah. Leveraging Gemini to tackle complex bioinformatics problems like analyzing microarray data can potentially yield valuable insights and speed up research in the field.
The possibilities of applying AI in genomics are truly exciting! Gemini could provide researchers with a powerful tool to assist in data interpretation and hypothesis generation.
I wonder if Gemini can handle the large volumes of data typically associated with microarray experiments. Processing such high-dimensional datasets efficiently is crucial for meaningful analysis.
That's a valid point, Lucas. Scaling up Gemini to handle large-scale genomic data can be challenging. It would be interesting to know more about the model's performance on different data sizes.
Thank you all for your comments! Sophia, you bring up an important aspect. Gemini has shown promising results on smaller datasets, and while scalability is a concern, advancements in AI infrastructure could potentially address these challenges.
Thank you for elaborating, Donald. Overcoming those challenges and leveraging Gemini for gene expression analysis could lead to significant advancements in disease understanding, personalized medicine, and drug discovery.
Absolutely, Sophia. Scaling up AI models is an ongoing area of research. It's crucial to explore how Gemini can handle the volume and complexity of microarray data to make it a viable tool for genomics research.
I completely agree, Michael. As Gemini continues to evolve, it's important to validate its performance on larger datasets and further refine it for genomics applications.
While the potential for Gemini in genomics is intriguing, we should also consider the ethical implications. AI-driven analysis should not replace the expertise and careful interpretation that domain experts bring to the table.
Well said, Oliver. AI should augment human expertise, not replace it. Researchers should be cautious of over-reliance on AI models and ensure a balanced approach to leverage their benefits while considering the limitations.
Great points, Oliver, Alexandra, Emily, and Sophia. Ethical considerations and the integration of AI with human expertise are indeed vital aspects when adopting AI technologies in genomics research.
Donald, could you provide more insights into the challenges currently faced in implementing Gemini for microarray analysis? It would be interesting to know the specific obstacles researchers might encounter.
Certainly, Michael. One challenge is fine-tuning Gemini on domain-specific microarray data due to limited annotated samples. Another is optimizing the model's architecture to handle the complexity of gene expression patterns.
Donald, what are the potential benefits that Gemini can offer in the microarray domain? It would be helpful to understand the specific use-cases where this technology can make an impact.
Indeed, Lucas. Gemini can assist researchers in tasks like feature selection, clustering analysis, and identifying potential biomarkers or gene expression signatures associated with certain conditions. Its interactive nature allows for exploration and hypothesis generation.
I agree, Alexandra. AI can be a valuable tool, but human intuition and domain knowledge are irreplaceable. It's crucial to strike a balance between leveraging AI capabilities and expert interpretation.
Exactly, Emily. AI can assist researchers by identifying patterns and potential relationships in large-scale datasets, but it should always be complemented with the expertise of domain specialists.
I'm curious about the interpretability aspect of Gemini. Can it provide explanations for its predictions in the microarray domain? Interpretability is crucial for gaining the trust of researchers and clinicians.
That's an important question, Liam. While Gemini's internal workings might be complex, being able to understand and explain the logic behind its predictions is critical for adoption in scientific research.
I agree, Sarah. Explainability is key in genomics, where researchers need to understand the underlying rationale behind AI-driven predictions. Incorporating interpretability techniques becomes crucial for adoption.
Indeed, interpretability can't be overlooked. As the field progresses, models like Gemini need to provide transparent explanations to foster trust and enable researchers to validate and build upon their findings.
Absolutely, Thomas. Explainable AI not only promotes trust but also helps researchers identify potential biases or limitations in the model, allowing for better decision-making in genomics and related areas.
The integration of explainable AI in genomics research also means being able to communicate the findings effectively to medical professionals, policymakers, and the public. Clear explanations bridge the gap between complex AI models and practical applicability.
Absolutely, Oliver. Effectively communicating the insights and implications derived from AI models like Gemini is paramount to ensure a broader understanding and acceptance of AI-driven genomics research.
I completely agree, Emily. Bridging the gap between AI and different stakeholders is crucial for driving the adoption of AI technologies in genomics and ensuring its potential benefits reach the wider society.
Talking about scalability, what are the computational requirements for running Gemini on microarray data? Are there any limitations or computational bottlenecks to be aware of?
Good question, Alex. The computational requirements depend on the specific architecture and implementation. While there might be challenges in handling large datasets efficiently, leveraging distributed computing and optimizations can help mitigate bottlenecks.
It would also be interesting to see how Gemini performs when confronted with real-time or streaming microarray data, where processing speed becomes critical for prompt analysis and decision-making.
That's a valid point, Sophia. Handling real-time data is crucial for enabling rapid interpretation of microarray experiments, especially in time-sensitive clinical or research settings.
Considering the rapidly evolving nature of genomics research, how do you foresee the integration of AI technologies like Gemini improving in the future? Are there any specific challenges to address?
Great question, Liam. Looking ahead, the integration of AI technologies in genomics research will require addressing challenges like data privacy, ensuring unbiased model outputs, and addressing the limitations of models in handling complex biological systems.
Another area to consider is the continuous training and updating of AI models like Gemini to keep up with the rapidly expanding genomics knowledge. Adapting models to emerging research findings will be crucial.
Absolutely, Oliver. AI models should be adaptable, continuously learning, and updatable to incorporate new findings and ensure they stay relevant in a rapidly evolving genomics landscape.
I agree, Sophia. Regular updates and collaborations between AI researchers and genomics experts will drive the refinement of models like Gemini and ensure they stay relevant and aligned with the latest scientific advancements.
While AI-driven technologies like Gemini hold significant promise, it's important to acknowledge the potential limitations and biases that could arise from training data or model design. Robust validation is needed to ensure the reliability and generalizability of AI tools.
You're absolutely right, David. Addressing biases and data limitations is crucial. Careful validation on diverse and representative datasets is essential to ensure AI tools like Gemini are reliable and unbiased in their predictions.
Validation should also involve proper evaluation metrics to assess the model's performance accurately. Transparency in reporting the limitations and potential biases of AI models fosters trust and helps guide their responsible use in genomics research.
I couldn't agree more, Alexandra. Ensuring transparency, standardized evaluation, and reporting of AI models will play a vital role in building trust, promoting reproducibility, and advancing responsible AI adoption in genomics.
Alongside addressing technical and ethical challenges, educating and training researchers on the responsible use of AI in genomics research is crucial. It will empower them to harness the full potential while minimizing risks.
You raise an important point, Thomas. Bridging the gap between AI specialists and domain experts through education and interdisciplinary collaborations will facilitate responsible and effective use of AI in genomics.
I couldn't agree more, Donald and Thomas. Encouraging open dialogue and fostering interdisciplinary collaborations will enable researchers to leverage AI tools like Gemini responsibly and make impactful advancements in genomics.
Adding to the interpretability aspect, would it be possible to incorporate prior biological knowledge or constraints into Gemini to guide its predictions and make them more biologically meaningful?
That's a great suggestion, Liam. Integrating prior biological knowledge or incorporating domain-specific constraints in Gemini can enhance the biological interpretability of its predictions, making them more aligned with existing knowledge.
Including domain knowledge in AI models can not only improve predictions but also aid in hypothesis generation. The combination of AI-driven insights and expert intuition can lead to more focused and targeted research directions.
Absolutely, Sophia. Combining the power of AI with the expertise of researchers can drive discovery and expedite the identification of meaningful biological markers or potential therapeutic targets.
Having the means to incorporate prior knowledge would not only aid in interpretability but also help prevent AI models from making implausible predictions that contradict established biological principles.
One of the challenges is also ensuring that AI models are designed and validated across diverse populations to avoid biases and limitations that might exist in the training data. Representation matters in genomics research.
Absolutely, Oliver. Achieving diversity and inclusivity in AI models is crucial to avoid biases and ensure equitable and impactful genomics research that benefits all populations.
Diverse representation in AI models also allows for the identification of population-specific patterns or risk factors that might be missed if the data primarily represents a specific population.
Thank you all for visiting my blog post on harnessing Gemini in the microarray domain. I hope you find the article informative and interesting. Please feel free to share your thoughts and opinions!
Great article, Donald! It's fascinating how AI technologies like Gemini can be applied to various domains. This could really revolutionize the field of microarray analysis.
Thank you, Sarah! I agree, the potential applications of AI in microarray analysis are immense. It opens up new possibilities for data interpretation and discovery of patterns.
AI advancements are truly remarkable. This article gives a clear overview of how Gemini can aid in the analysis of microarray data. Well written, Donald!
Thank you for your kind words, Robert! I'm glad you found the article informative. AI technologies like Gemini have the potential to enhance our understanding of complex biological data.
I never realized the potential of AI in the microarray domain until I read this article. The ability of Gemini to uncover hidden patterns and provide insights is impressive!
Glad to hear that, Sophia! AI technologies can indeed reveal hidden patterns in microarray data, leading to new discoveries and advancements in the field. It's an exciting time for research!
The article highlights how AI can potentially assist in the identification of biomarkers through microarray analysis. This could greatly impact precision medicine and personalized treatments.
Absolutely, Mark! AI-powered analysis of microarray data can aid in identifying biomarkers and facilitate personalized medicine. It has the potential to significantly improve patient outcomes.
Gemini seems to be a powerful tool for data analysis! The article provides a comprehensive understanding of its capabilities in the context of microarray research.
Thank you, Emily! Gemini indeed offers valuable insights for data analysis across various domains. In the microarray field, it can potentially uncover hidden patterns and assist in the interpretation of complex datasets.
I wonder if Gemini can be integrated into existing microarray analysis software. It could be a game-changer for researchers in terms of efficiency and accuracy.
That's a great point, Michael! Integrating Gemini into existing microarray analysis software could enhance researchers' capabilities and streamline the analysis process. It's an exciting prospect!
As promising as AI is, we shouldn't overlook the importance of human expertise in interpreting microarray data. Gemini can be a valuable tool, but the human touch is still crucial.
You're absolutely right, Olivia! AI technologies like Gemini should be seen as complementing human expertise rather than replacing it. The human touch is vital for critical analysis, validation, and decision-making.
I'm curious about the potential limitations of Gemini in the microarray domain. Could it face challenges in accurately analyzing complex datasets?
Good question, Adam! While Gemini is a powerful tool, it does have limitations. Complex datasets and noise in microarray data might pose challenges for accurate analysis. It requires careful validation and domain-specific tuning.
This article made me realize the immense potential of AI in accelerating research in the microarray domain. It can help researchers focus their efforts on the most promising avenues.
Indeed, Lily! AI can assist researchers in prioritizing their work and identifying promising areas for further exploration. It can save valuable time and resources in the research process.
Gemini's ability to understand and respond to natural language makes it a user-friendly tool for researchers, even those without extensive programming skills.
Absolutely, Joshua! Gemini's natural language capabilities make it accessible to researchers from various backgrounds. It reduces the barrier to entry and encourages interdisciplinary collaboration.
The article suggests that Gemini can extract meaningful insights from microarray data. I'm excited to see its potential impact on research discoveries.
I share your excitement, Grace! Gemini has the potential to reveal previously unnoticed patterns and relationships in microarray data, leading to breakthroughs in research and discoveries.
One concern with AI tools like Gemini is the potential bias in the training data and resulting analysis. How can we address this issue in microarray analysis?
That's an important concern, Jacob. Addressing bias requires a careful approach to training data selection and diversity. It's crucial to focus on representative data and ongoing validation to ensure fair and reliable analysis.
I appreciate the author's emphasis on the ethical considerations associated with AI adoption in the microarray domain. It's essential to prioritize responsible and transparent practices.
Thank you, Sophie! Ethical aspects of AI adoption should always be at the forefront. Responsible and transparent practices are vital to ensure the trustworthy and beneficial application of AI in the microarray domain.
AI advancements like Gemini have immense potential, but we should also be mindful of their limitations. Human expertise and validation are crucial to avoid unfounded conclusions.
Absolutely, Ethan! AI should be viewed as a supportive tool that enhances human expertise rather than replacing it. Human validation and critical analysis are vital for reliable and accurate conclusions.
Donald, do you foresee any challenges in the adoption of Gemini or similar AI technologies in the microarray domain?
Good question, Sarah! Adoption challenges may include integration into existing workflows, addressing data privacy concerns, and ensuring user-friendly interfaces for researchers. Overcoming these challenges would be crucial for successful adoption.
Do you think Gemini could eventually evolve into a tool that assists researchers in designing microarray experiments?
That's an interesting thought, Robert! While Gemini's primary focus is on data analysis, advancements in AI could potentially extend its capabilities to assist researchers in experimental design by suggesting optimal strategies and parameter settings.
I'm curious about the scalability of Gemini in handling large-scale microarray datasets. Could it handle the vast amount of data generated in research projects?
Scalability is an important consideration, Emily. While Gemini can handle substantial amounts of data, very large-scale datasets might pose challenges. However, continued advancements in AI technology are likely to improve scalability in the future.
I appreciate how the article highlights the need for interpretability in AI-driven analysis. Having clear explanations of the rationale behind Gemini's insights is crucial for researchers to trust and validate the results.
Interpretability is indeed a significant aspect, Olivia. Providing clear explanations and transparent insights helps researchers understand, validate, and trust the results generated by AI tools like Gemini. It's essential for effective collaboration between AI and humans.
I wonder if Gemini can be extended to other types of -omics data analysis besides microarrays, such as RNA-Seq or proteomics. The possibilities seem endless!
You're right, Adam! The potential applications of AI in -omics data analysis are vast. While Gemini is primarily showcased in the microarray domain in this article, similar approaches could be explored for other -omics data types like RNA-Seq and proteomics.
I appreciate the author's emphasis on the need for collaboration between AI and domain experts. Combining their strengths can lead to transformative advancements in various research fields.
Absolutely, Michael! Collaboration between AI and domain experts is key for harnessing the full potential of AI technologies. By combining their expertise, we can achieve breakthroughs in research and make significant advancements.
The integration of AI in the microarray domain could also lead to more reproducible research outcomes by reducing the impact of manual biases and variations.
That's an excellent point, Sophie! AI integration can contribute to more reproducible research outcomes by minimizing biases and variations introduced by manual analysis. It promotes consistency and reliability in research findings.
What are the potential challenges in training Gemini for microarray analysis? Is large labeled training data required for optimal performance?
Training Gemini for microarray analysis does require labeled data, Jacob. However, the size of the training dataset can vary depending on the desired performance. Large labeled datasets generally tend to yield better results, but suitable performance can be achieved with carefully curated smaller datasets too.
The discussion around AI adoption in the microarray domain needs to involve broader stakeholders like clinicians to ensure that the insights derived have practical clinical implications.
You raise a crucial point, Grace! Involvement of clinicians and other stakeholders is essential to bridge the gap between research insights and practical clinical implications. By collaborating with them, AI technologies can be tailored to address real clinical needs.
The increasing integration of AI in research domains emphasizes the need for researchers to develop a deeper understanding of AI techniques and their limitations.
Absolutely, Ethan! Researchers should strive to develop a deeper understanding of AI techniques to leverage their potential effectively. This knowledge enables them to make informed decisions, interpret the results accurately, and overcome potential limitations.
Thank you for sharing this insightful article, Donald! It's been a great discussion, and it's exciting to see how Gemini can contribute to the microarray domain.
You're welcome, Sarah! I'm glad you found the article and the discussion valuable. Gemini indeed holds immense promise for the microarray domain, and I'm excited about the possibilities it brings. Thank you all for your engaging comments!