Exploring the Impact of ChatGPT on Technological Statistics
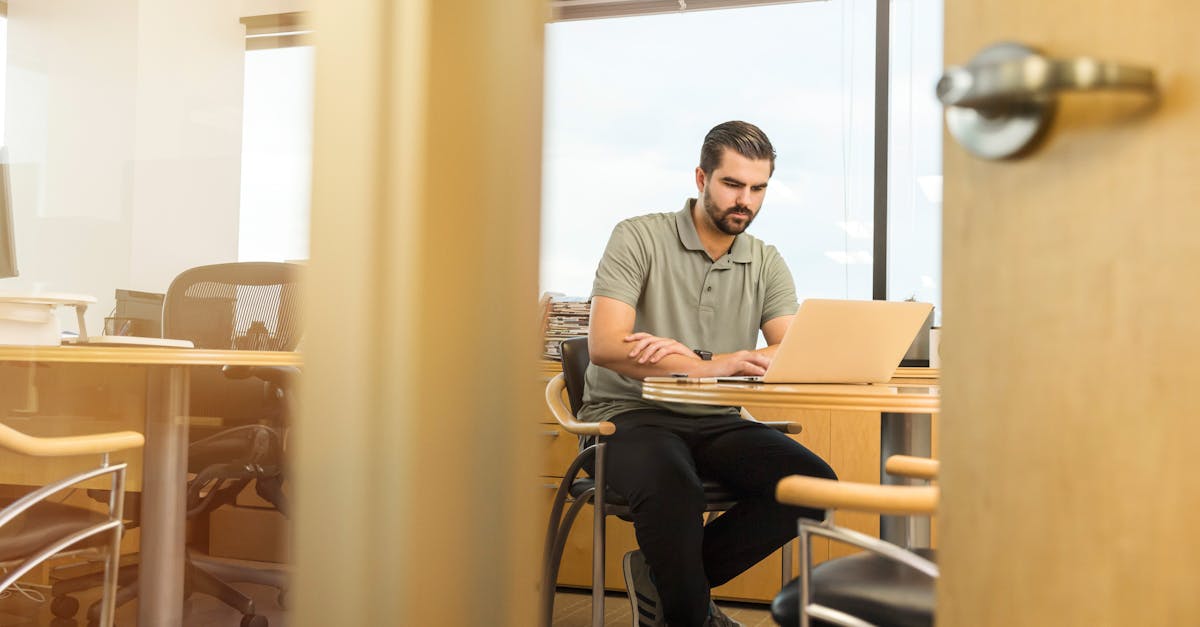
Introduction
Sampling is an essential aspect of statistical analysis as it allows researchers to gather valuable insights about a population without studying every individual within that population. By selecting a representative sample, researchers can make accurate inferences and draw conclusions that reflect the entire population. In statistical analysis, different sampling techniques are employed to ensure the sample represents the population effectively.
Simple Random Sampling
Simple random sampling is one of the most basic sampling techniques. It involves randomly selecting individuals from a population without any specific criteria. Each member of the population has equal chances of being included in the sample. This technique ensures that the sample is unbiased and allows for generalizations to the entire population if done correctly.
Stratified Sampling
Stratified sampling divides the population into distinct subgroups, known as strata, based on specific characteristics. Each stratum is then sampled proportionally, according to their representation within the population. This technique ensures that each subgroup is adequately represented in the sample, making it particularly useful when studying populations with heterogeneous characteristics.
Cluster Sampling
Cluster sampling involves dividing the population into separate clusters or groups and randomly selecting a few clusters to include in the sample. This technique is often used when it is impractical or expensive to access every member of the population individually. Cluster sampling is particularly useful when the clusters are similar internally but differ from each other, allowing for efficient data collection.
Systematic Sampling
Systematic sampling involves selecting individuals from a population at regular intervals. For instance, if researchers want to sample every 10th person within a population, they would select the first individual randomly and then select every subsequent 10th person. This technique provides a representative sample by providing an equal chance of selection to each individual within the population.
Applications
The understanding of different sampling techniques is crucial in various statistical applications. These techniques are widely employed in market research, where data about customer preferences, demographics, and behavior is collected. Additionally, government surveys often utilize sampling techniques to estimate unemployment rates, population sizes, or political opinions.
One specific application of sampling techniques is in the field of data analysis for Artificial Intelligence (AI) models such as ChatGPT-4. As AI models require vast amounts of data to learn and make accurate predictions, using the entire population is often unfeasible. By applying appropriate sampling techniques, AI models can learn from representative samples, leading to more efficient training processes and reliable predictions.
Conclusion
Sampling techniques play a vital role in statistical analysis, ensuring that reliable conclusions can be drawn from representative samples. Simple random sampling, stratified sampling, cluster sampling, and systematic sampling are among the techniques used. These techniques have applications in various industries, including market research, government surveys, and AI data analysis. By understanding and utilizing these techniques effectively, researchers and data analysts can make accurate inferences about populations and improve decision-making processes.
Comments:
Thank you all for joining this discussion on the impact of ChatGPT on technological statistics. I'm excited to hear your thoughts and opinions!
ChatGPT has definitely revolutionized the way we interact with technology. It has greatly improved natural language understanding and response generation. However, it also raises concerns about the potential biases in its responses. We need to ensure that the data it learns from is diverse and representative. What do you all think?
I agree with you, Alice. While ChatGPT is impressive, it's crucial to address biases. Diversity in training data is vital to avoid perpetuating stereotypes or misinformation. Developers should continuously monitor and work towards reducing biases through feedback and improvements.
Absolutely, Robert. Bias mitigation should be a priority. I suggest implementing robust evaluation processes to identify and rectify biases. Additionally, involving domain experts and diverse user feedback can help in making the system more inclusive and accurate.
I completely agree, Emma. Feedback from users and domain experts is crucial for continuous improvement. Bias mitigation should be an ongoing effort to ensure fairness and inclusivity in the technology.
While ChatGPT is impressive in many ways, there are concerns about its reliability. It can sometimes generate misleading or incorrect answers, which can be misleading to users who rely on it for accurate information. We should focus on improving its fact-checking capabilities.
That's a valid point, James. Fact-checking is a crucial aspect, and improvements in accuracy are necessary. It's important to strike a balance between generating creative responses and providing accurate information to enhance user trust.
Thank you, Virginia. It's been a thought-provoking discussion, and I've gained new insights into the role of AI models like ChatGPT in technological statistics.
I believe ChatGPT has immense potential in various fields. It can assist in data analysis, research, and even content creation. However, to leverage its benefits fully, it should be combined with human oversight to ensure the quality and reliability of its outputs.
Great point, Sophia! Human oversight is essential to maintain quality and prevent the propagation of inaccurate or misleading information. Combining human expertise with AI technologies like ChatGPT can lead to more reliable and informed outcomes.
I'm concerned about the potential misuse of ChatGPT technology. As it becomes more powerful and sophisticated, malicious actors could manipulate it to spread misinformation, deceive users, or even conduct social engineering attacks. Security measures should be a top priority.
You raise an important concern, Daniel. Security measures are indeed crucial to minimize the risk of misuse. It's essential to have safeguards in place to detect and prevent malicious activities and protect users from potential harm.
ChatGPT has brought some exciting advancements, but there are also ethical concerns. As the technology becomes more advanced and indistinguishable from human responses, there is a need for guidelines and regulations to address issues like privacy, consent, and transparency.
I couldn't agree more, Olivia. Ethical considerations should be at the forefront of AI development. Clear guidelines, regulations, and standards are necessary to ensure responsible and transparent deployment of ChatGPT and similar technologies.
It's fascinating to see how ChatGPT can assist in language translation, customer support, and even creative writing. However, we must also address potential job displacement concerns caused by the automation of tasks traditionally performed by humans.
You raise an important point, Grace. Automation can lead to job displacement in certain areas. It's crucial to have measures in place to mitigate the impact, such as reskilling programs and creating new opportunities within the evolving technological landscape.
While ChatGPT is undeniably impressive, it also highlights the need for responsible use. Users should be aware of its limitations and understand that it's an AI system. Promoting digital literacy and educating users about AI can empower them to make informed decisions.
Absolutely, Mike. Digital literacy is key in today's technology-driven world. By fostering awareness and understanding about AI systems like ChatGPT, we can empower users to engage responsibly and make informed judgments about the information they receive.
To maximize the positive impact of ChatGPT, it's crucial to ensure accessibility. The technology should be designed in a way that accommodates different languages, cultures, and abilities, enabling a more inclusive and equitable user experience.
Well said, Emily. Accessibility is a fundamental aspect of technology design. Adapting ChatGPT to cater to diverse languages, cultures, and abilities will enhance its reach and impact, enabling more individuals to benefit from its capabilities.
ChatGPT is undoubtedly impressive, but as we explore its potential, we should also be mindful of the energy consumption involved in large-scale AI systems. Developing sustainable AI technologies should be a priority for a greener future.
You bring up a very valid point, David. Energy consumption and environmental impact are important considerations in AI development. Promoting research and implementing sustainable practices can contribute to a greener future while enjoying the benefits of AI technologies like ChatGPT.
Thank you, Virginia, for facilitating this engaging discussion. It's been a pleasure exchanging ideas and learning from everyone's valuable insights.
As advancements continue, the accountability of AI systems like ChatGPT becomes essential. Transparent disclosure of the technology's limitations, capabilities, and decision-making processes is crucial to build trust and enable users to make informed choices.
I completely agree, Jennifer. Trust-building is essential, and transparent disclosure of the technology's behavior and limitations is necessary. Openly sharing information about ChatGPT's decision-making processes can empower users to gauge its reliability and usefulness.
One of the exciting prospects of ChatGPT is its ability to assist in research and development. It can accelerate innovation by generating new ideas and possibilities, helping researchers and scientists explore uncharted territories.
Absolutely, Erica. The research potential of ChatGPT is immense. By assisting researchers and scientists in generating new ideas and exploring uncharted territories, it can contribute to breakthroughs and advancements across various fields.
ChatGPT has opened up numerous opportunities for businesses. It can enhance customer support, automate tasks, and even customize user experiences. Its potential for improving efficiency and productivity is noteworthy.
Well said, Alex. The business applications of ChatGPT are indeed valuable. By leveraging its capabilities for tasks like customer support and automation, businesses can streamline processes, enhance efficiency, and provide personalized user experiences.
The development of ChatGPT is a testament to AI progress. While it has some limitations, the continuous advancements in natural language processing and generation are exciting. I'm eager to see how ChatGPT and similar technologies evolve in the future.
I share your excitement, Claire. The progress in AI, particularly in natural language processing, is truly fascinating. The future holds incredible possibilities as technologies like ChatGPT evolve and empower us to achieve more with the aid of AI.
ChatGPT's impact in the education sector is worth exploring. It can supplement learning, provide instant feedback, and even assist students in their assignments. Integrating it effectively with educational systems can enhance the learning experience.
Valid point, Mark. ChatGPT's potential in education is immense. By incorporating it thoughtfully, we can augment learning, provide personalized feedback, and foster greater engagement. It can become a valuable tool for both students and educators.
ChatGPT has opened new doors for exploring conversational AI. It has the potential to simulate human-like conversations and improve human-machine interaction. Continued research and development in this area can lead to even more impressive breakthroughs.
Absolutely, Sarah. Conversational AI has progressed significantly with the advent of ChatGPT. Simulating human-like conversations and enhancing human-machine interaction are remarkable achievements. Continued research will undoubtedly push the boundaries and unlock further potential.
ChatGPT's potential for content creation is exciting. It can generate written content for various purposes, like news articles, blog posts, and marketing copy. However, it raises concerns about originality and plagiarism. Ensuring ethical content generation should be a focus.
You raise a valid concern, Lucas. Content generation capabilities of ChatGPT are impressive, but maintaining ethical content creation is crucial. Balancing automation and ensuring originality while avoiding plagiarism is essential to uphold integrity in various domains.
The impact of ChatGPT on language translation is noteworthy. It can bridge communication gaps by providing real-time translation services. However, it's important to continuously refine its accuracy and handle complex linguistic nuances.
Indeed, Sarah. Language translation is an area empowered by ChatGPT. Real-time translation services can facilitate global communication. Refining its accuracy, addressing linguistic complexities, and considering cultural nuances will lead to more effective and reliable translations.
While the impact of ChatGPT is tremendous, we must remember that it's an AI tool. Human intelligence and intuition are equally important. Using ChatGPT as an assistant to augment, rather than replace, our abilities can result in more robust and meaningful outcomes.
Well said, Lisa. ChatGPT's role as an assistant rather than a replacement is crucial. Combining the strengths of AI tools like ChatGPT with human intelligence and intuition can lead to more holistic and impactful solutions across various domains.
One area where ChatGPT can have a significant impact is in healthcare. It can assist with diagnosing symptoms, providing medical information, and even supporting mental health conversations. Ethical considerations and security measures must be prioritized in this domain.
You make a great point, Oliver. Healthcare is one of the areas where ChatGPT's potential is immense. Nevertheless, prioritizing ethics, privacy, and security is crucial to ensure the accuracy, reliability, and confidentiality needed for healthcare-related interactions.
Yes, Virginia, I second that. Bias in AI can have serious consequences. It is essential to have rigorous evaluation processes to identify and mitigate biases.
Thank you, Virginia. This discussion has shed light on the opportunities, challenges, and ethical considerations surrounding the impact of ChatGPT on technological statistics.
The collaborative potential of ChatGPT is intriguing. It can facilitate teamwork, brainstorming, and problem-solving among individuals from different locations. Optimizing its collaboration features can unlock new possibilities for remote work and beyond.
Absolutely, Sophie. The collaboration aspect of ChatGPT holds immense promise. By enhancing its capabilities for teamwork and remote collaboration, we can foster creativity, problem-solving, and innovation beyond the constraints of physical proximity.
ChatGPT is undoubtedly impressive, but we should not overlook the importance of user privacy. As AI systems learn from user interactions, safeguarding personal information and ensuring consent are vital in maintaining user trust.
You're absolutely right, Ethan. User privacy and consent are paramount in the development and deployment of AI systems like ChatGPT. Implementing robust privacy measures and giving users control over their data are crucial for building and maintaining trust.
Ethics in AI is a critical topic, and ChatGPT's impact highlights the need for guidelines and regulations. Establishing ethical frameworks, standards, and accountability processes can help navigate the complex landscape and ensure responsible AI development.
I couldn't agree more, Natalie. Ethical guidelines and regulations are essential to guide AI development. By establishing responsible frameworks and fostering accountability, we can ensure that technologies like ChatGPT are developed and deployed in a way that benefits society while minimizing potential harm.
The potential of ChatGPT in assisting individuals with disabilities is immense. By customizing its interfaces and incorporating accessibility features, we can empower people with different abilities to participate fully, ensuring inclusivity across digital platforms.
Well said, Jack. Accessibility should be at the forefront of AI technologies. Adapting ChatGPT to accommodate diverse abilities and providing inclusive interfaces can enable a more accessible digital landscape, ensuring equal participation and opportunities.
One potential area of concern is the addictiveness of ChatGPT. Its interactive nature and ability to engage users for prolonged periods can lead to overreliance and potential negative impacts on interpersonal connections and mental well-being.
That's an important point, Noah. Balancing engagement and preventing potential negative impacts on mental well-being is crucial. Encouraging mindful usage, setting limits, and promoting a healthy balance between technology and interpersonal connections are essential considerations.
The impact of ChatGPT on human creativity is intriguing. By assisting with idea generation, brainstorming, and creative writing, it can potentially augment our creative processes and lead to novel and innovative outcomes.
Absolutely, Sophie. ChatGPT's role in augmenting human creativity is remarkable. By serving as a creative assistant, it can unlock new ideas, enhance brainstorming sessions, and fuel innovation across various creative domains.
ChatGPT's potential impact in the gaming industry is worth exploring. It can serve as an interactive game master, adapt to player choices, and provide dynamic storytelling experiences. It opens up exciting possibilities for immersive gameplay.
That's an interesting perspective, Amy. ChatGPT's integration into the gaming industry can indeed transform gaming experiences. As an interactive game master, it can create dynamic and immersive storytelling, leading to more engaging and personalized gameplay.
As the use of ChatGPT becomes more widespread, responsible deployment becomes crucial. Developers, organizations, and policymakers should work together to establish ethical guidelines and frameworks that prioritize the well-being of individuals and society.
I completely agree, Liam. Responsible deployment of ChatGPT, along with robust ethical guidelines, is essential to prevent misuse and prioritize societal well-being. Collaboration between developers, organizations, and policymakers can ensure its positive impact while mitigating potential risks.
ChatGPT's impact on data analysis and insights is worth discussing. It can assist in analyzing complex datasets, identifying patterns, and generating valuable insights. Its ability to handle large volumes of data can save time and enhance decision-making.
Great point, Chris. ChatGPT's potential in data analysis and insights is tremendous. By leveraging its capabilities to process and analyze complex datasets, we can gain valuable insights and make informed decisions more efficiently and effectively.
While ChatGPT is impressive, the potential unintended consequences should be carefully considered. As AI systems become more prevalent, we should be mindful of biases, privacy concerns, and the impact on human relationships within various contexts.
You bring up important considerations, Julia. The widespread use of AI systems like ChatGPT necessitates a holistic perspective. Careful evaluation of potential consequences, including biases, privacy, and impacts on human relationships, is crucial to maximize benefits and minimize harm.
ChatGPT's capabilities in virtual assistants and smart homes are fascinating. It can automate tasks, personalize interactions, and enhance user experiences. However, we must address the challenges of privacy, security, and building user trust in these contexts.
Absolutely, Anna. ChatGPT's integration into virtual assistants and smart homes unlocks new possibilities. Balancing automation, privacy, and security with personalized and trustworthy interactions is essential to fully leverage its potential in these contexts.
ChatGPT's role in content moderation is an area that requires attention. While it can assist in identifying problematic content, human oversight is crucial to avoid false positives or negatives. Striking the right balance is key to maintaining user trust and freedom of expression.
Well said, Kayla. Content moderation is a delicate balance, and human oversight is essential. Augmenting human moderators with the capabilities of ChatGPT can help identify problematic content, but robust measures must be in place to avoid false judgments and uphold freedom of expression.
The impact of ChatGPT on customer support and service is significant. It can streamline communication, address FAQs, and provide personalized assistance. Its ability to understand and respond to user queries enhances the overall customer experience.
Indeed, Alex. In the realm of customer support and service, ChatGPT can be a valuable tool. Optimizing its capabilities to streamline communication, address common queries, and provide personalized assistance can enhance the customer experience and improve overall satisfaction.
As AI systems like ChatGPT advance, it's crucial to ensure transparency in their decision-making processes. Understanding how decisions are reached can increase user trust, improve accountability, and facilitate audits, particularly in critical areas like finance or healthcare.
Absolutely, Paul. Transparent decision-making is essential, particularly in critical domains. AI systems like ChatGPT should be capable of providing explanations and justifications for their outputs, leading to increased trust, accountability, and opportunities for auditing and validation.
The potential of ChatGPT in creative writing and storytelling is fascinating. It can assist authors, screenwriters, and content creators in generating ideas and enhancing their creative processes. The interplay between human creativity and AI technologies can lead to exciting outcomes.
Well said, Jason. The collaboration between human creativity and AI technologies like ChatGPT holds immense potential, particularly in creative fields. Assisting authors, screenwriters, and content creators can enhance the creative process, generate novel ideas, and spark imaginative storytelling.
The potential bias in ChatGPT's responses is a valid concern. Developers should actively work to address and mitigate biases through diverse training datasets and continuous feedback loops that incorporate insights from a wide range of perspectives.
You're absolutely right, Robert. Bias mitigation is crucial, and developers should adopt a proactive approach. Incorporating diverse training data, feedback loops, and perspectives can help in the continuous improvement and reduction of biases in ChatGPT's responses.
Indeed, Virginia. The diverse perspectives shared in this discussion have broadened my viewpoint on the impact of ChatGPT on technological statistics.
While ChatGPT is impressive, we shouldn't overlook the importance of user consent in data collection and usage. Ensuring users have control over their data and the ability to opt-in or opt-out can alleviate privacy concerns and enhance user trust.
Absolutely, Sophia. User consent and control over their data are key considerations. Respecting user privacy, providing transparency, and empowering users to make informed choices regarding data collection and usage are vital for fostering trust and maintaining ethical practices.
The potential of ChatGPT in research and analysis is intriguing. It can assist in literature reviews, data exploration, and hypothesis generation. Combining machine intelligence with human expertise can lead to more comprehensive and insightful research outcomes.
Well said, Emily. ChatGPT's assistance in research and analysis can be transformative. By leveraging its capabilities for tasks like literature reviews, data exploration, and hypothesis generation, researchers can augment their expertise, leading to more robust and insightful outcomes.
ChatGPT has potential applications in personalized learning. It can adapt to individual learning styles, provide tailored explanations, and offer personalized recommendations. This can revolutionize the educational experience and enhance student engagement and outcomes.
Absolutely, Luke. Personalized learning is an area where ChatGPT can make a significant impact. Adapting to individual learning styles, providing tailored explanations, and offering personalized recommendations can foster greater student engagement and improve learning outcomes.
The potential of ChatGPT in generating code and assisting developers is exciting. From providing code snippets to automating repetitive tasks, it can accelerate development and enhance productivity. Its role as a programming assistant is promising.
Well said, Amelia. ChatGPT's potential in code generation and developer assistance is remarkable. By automating repetitive tasks, providing code suggestions, and enhancing productivity, it can serve as a valuable programming assistant, thereby speeding up development processes.
While the capabilities of ChatGPT are fascinating, it's important to recognize its limitations. It's an AI model trained on data and lacks true understanding. We should engage with it mindfully and acknowledge its responses may not always align with reality.
You make a crucial point, Jacob. Mindful engagement with ChatGPT, keeping in mind its limitations, is essential. Understanding that it's an AI model trained on data and may not always align with reality helps us use it as a tool while leveraging human judgment and expertise.
The potential of ChatGPT in simulating human-like conversations is impressive. It can assist in language learning, interview preparation, and social skills development. Its ability to provide interactive and engaging conversations can be immensely beneficial.
Absolutely, Claire. ChatGPT's role in simulating human-like conversations is remarkable. Leveraging it for language learning, interview preparation, and social skills development can provide interactive and engaging learning experiences, fostering growth and proficiency in various domains.
ChatGPT's applications in virtual reality and augmented reality are worth exploring. It can enhance immersive experiences, enable real-time information access, and create interactive virtual environments. Its integration with extended reality technologies can unlock new possibilities.
You're absolutely right, Jessica. The integration of ChatGPT with virtual reality and augmented reality can revolutionize immersive experiences. Real-time information access, interactive environments, and seamless integration can create captivating user experiences and unlock new digital frontiers.
ChatGPT's potential in market research and customer insights is notable. It can analyze consumer preferences, identify trends, and assist in product development. By leveraging its capabilities, businesses can make informed decisions and better understand their target audience.
Great point, Anna. ChatGPT's potential in market research and consumer insights is indeed noteworthy. By leveraging its capabilities for analyzing preferences, identifying trends, and assisting in product development, businesses can gain valuable insights to make informed decisions and better serve their target audience.
The potential of ChatGPT in legal research and analysis is significant. It can assist in reviewing case law, legal documents, and contract analysis. Incorporating AI-powered tools like ChatGPT can enhance legal workflows and provide more efficient legal services.
Absolutely, Anthony. ChatGPT's integration into legal research and analysis is promising. By assisting in reviewing case law, legal documents, and contract analysis, it can enhance efficiency and accuracy in legal workflows, ultimately providing more effective and efficient legal services.
The potential impact of ChatGPT in journalism and content creation is intriguing. It can assist in article writing, fact-checking, and research. Integrating it effectively can augment journalists' abilities and improve the quality and speed of content creation.
Well said, Joshua. ChatGPT's potential in journalism and content creation is remarkable. By assisting in article writing, fact-checking, and research, it can augment journalists' capabilities and lead to more efficient and accurate content creation processes.
ChatGPT's impact on social media interactions and content generation is noteworthy. It can assist in generating engaging posts, recommending content, and managing interactions. Its integration into social media platforms can enhance user experiences and boost engagement.
Absolutely, Gabriel. ChatGPT's integration into social media platforms can result in exciting possibilities. By assisting in content generation, recommending personalized posts, and managing interactions, it can contribute to more engaging social media experiences, fostering better connections and boosting engagement.
The impact of ChatGPT on data privacy is a significant concern. As it processes and learns from user interactions, ensuring robust privacy measures, data protection, and transparency about data handling practices should be prioritized to maintain user trust.
You raise an important point, William. Protecting user data, implementing robust privacy measures, and being transparent about data handling practices are crucial for maintaining user trust. Safeguarding data privacy should be a priority in chatbot technology like ChatGPT.
ChatGPT's potential to encourage critical thinking and knowledge exploration is intriguing. By challenging assumptions, providing alternative perspectives, and supporting information discovery, it can foster intellectual curiosity and greater exploration in various domains.
Well said, Daniel. Encouraging critical thinking and knowledge exploration is a valuable aspect of ChatGPT's potential. By challenging assumptions, providing diverse perspectives, and facilitating information discovery, it can foster intellectual curiosity and inspire continuous learning and exploration.
The interdisciplinary potential of ChatGPT is incredibly exciting. Its applications span numerous fields, from healthcare to education, research, marketing, and beyond. Collaborations across disciplines can unlock new ways of leveraging ChatGPT's capabilities.
Indeed, Sophia. The interdisciplinary potential of ChatGPT is extensive. By fostering collaborations across various fields, we can leverage its capabilities to tackle complex challenges and find innovative solutions, ultimately benefiting multiple domains to achieve greater societal impact.
Thank you, Virginia. This discussion has emphasized the importance of collaborative efforts between AI and human experts in advancing technological statistics.
This article provides an interesting perspective on the impact of ChatGPT on technological statistics. It's fascinating how AI advancements like this can affect data analysis.
I agree with David too. AI advancements have indeed created new opportunities, but there is also a need to be cautious about potential ethical concerns that may arise.
I completely agree, David! AI technologies like ChatGPT have the potential to revolutionize how we analyze and interpret data in various fields.
Thank you, David and Karen, for your comments. I'm glad you find the topic interesting. AI indeed plays a significant role in shaping the future of data analytics.
While the potential benefits are undeniable, we must also consider the limitations of AI algorithms like ChatGPT. Bias and interpretability can be major challenges in utilizing these models effectively.
Great point, Martin! Addressing bias and ensuring transparency in AI algorithms is crucial for their responsible implementation. These are important topics to further explore.
Thank you, Virginia, for moderating this discussion. It's inspiring to see the level of engagement and the collective understanding we have developed.
I find it fascinating how ChatGPT can contribute to technological statistics. The ability to generate human-like responses opens up possibilities for more complex data analysis tasks.
Absolutely, James! AI language models like ChatGPT have immense potential in assisting researchers and analysts in data-driven decision making.
Exactly, Patricia! With ChatGPT, we can explore large datasets more efficiently and gain valuable insights.
That's true, James. The natural language understanding capabilities of ChatGPT enable users to extract meaningful information from texts that were previously challenging to analyze.
I'm curious to know if there are any specific examples or case studies that demonstrate the impact of ChatGPT on technological statistics.
Great question, Emily! There are ongoing studies in various domains, such as financial analysis and healthcare, where ChatGPT has shown promise in extracting insights from complex data.
Thank you for the response, Virginia. I'll explore those case studies to get a better understanding of how ChatGPT can enhance technological statistics.
I have a concern regarding the potential misuse of ChatGPT. In the wrong hands, such AI models can be used to manipulate data and distort statistical analysis.
You make a valid point, Samuel. Ensuring responsible use and regulation of AI technologies like ChatGPT is crucial to prevent any misuse.
I agree with both Martin and Samuel. As we explore the impact of ChatGPT on technological statistics, it's vital to establish ethical guidelines and robust governance.
I think it's worth discussing the limitations of ChatGPT when applied to technological statistics. How accurate and reliable are its generated responses in complex analytical scenarios?
That's an important aspect to consider, Laura. While ChatGPT has made significant advancements in language generation, it's crucial to validate and refine its responses for accurate statistical analysis.
Thank you for addressing my question, Virginia. It's reassuring to know that efforts are being made to ensure the accuracy and reliability of ChatGPT in analytical contexts.
I see great potential in the collaboration between AI models like ChatGPT and human experts in the field of technological statistics. The synergistic effect can lead to innovative solutions.
I agree, Robert. Combining human expertise with AI technologies like ChatGPT can amplify the capabilities and generate novel insights in technological statistics.
Indeed, Robert and Karen. The human-machine collaboration has immense potential in pushing the boundaries of what we can achieve in data analysis.
One potential challenge is the interpretability of ChatGPT's decision-making process. Understanding how it arrives at certain conclusions is crucial to build trust in its insights.
You raise a valid concern, Gavin. Working on techniques to enhance the interpretability of AI models like ChatGPT is essential for their effective utilization in technological statistics.
I completely agree with you, Martin. Trust, transparency, and explainability should be at the forefront when harnessing AI technologies like ChatGPT for data analysis.
As technological statistics involve sensitive or confidential data, how can we ensure the privacy and security of such information when using AI models like ChatGPT?
Excellent question, Alice. Privacy and security should be paramount concerns. It's important to implement robust safeguards and comply with relevant data protection regulations.
Thank you for addressing my concern, Virginia. Ensuring the responsible handling of data is essential in the era of AI-driven analytics.
Thank you, Virginia. It's been fascinating to explore the potential and challenges associated with using ChatGPT in the realm of technological statistics.
I'm impressed by the potential applications of ChatGPT in technological statistics. It opens up new avenues for more impactful research and data-driven decision making.
Absolutely, Sophia! The continuous development and application of AI models like ChatGPT can reshape how we approach and gain insights from technological statistics.
Considering the impact of ChatGPT on technological statistics, do you think it will replace traditional statistical models and approaches in the future?
An intriguing question, Emily. While ChatGPT and other AI models offer new possibilities, I believe they will complement traditional statistical models rather than replace them.
I see your point, Virginia. Leveraging the strengths of both AI models and traditional statistical approaches can lead to more comprehensive analyses.
Indeed, Virginia. This discussion has deepened my understanding of the topic and highlighted the importance of responsible AI implementation in technological statistics.
This article highlights the evolving role of AI in technological statistics. It's exciting to witness the impact of ChatGPT in this field and its potential for innovation.
I couldn't agree more, John. AI models like ChatGPT are instrumental in pushing the boundaries of technological statistics and guiding future advancements.
While the benefits of AI in technological statistics are evident, we should be cautious not to over-rely on automated approaches and undervalue human expertise.
You're absolutely right, Samuel. AI models should be seen as tools to enhance human decision making and not replace the valuable domain knowledge of experts.
Precisely, Amy. Maintaining a balanced approach between AI and human expertise is essential for successful applications in technological statistics.
Thank you all for your insightful comments and engagement in this discussion. The impact of ChatGPT on technological statistics is a topic of great significance, and your perspectives enrich the discourse.
Thank you, Virginia, for bringing up this thought-provoking topic and fostering such a collaborative conversation. It has been an enlightening experience.
Thank you, Virginia. This topic has sparked my curiosity, and I look forward to further exploring the potential applications of ChatGPT in technological statistics.