Exploring the Potential of ChatGPT for Signal Processing in Hardware Technology
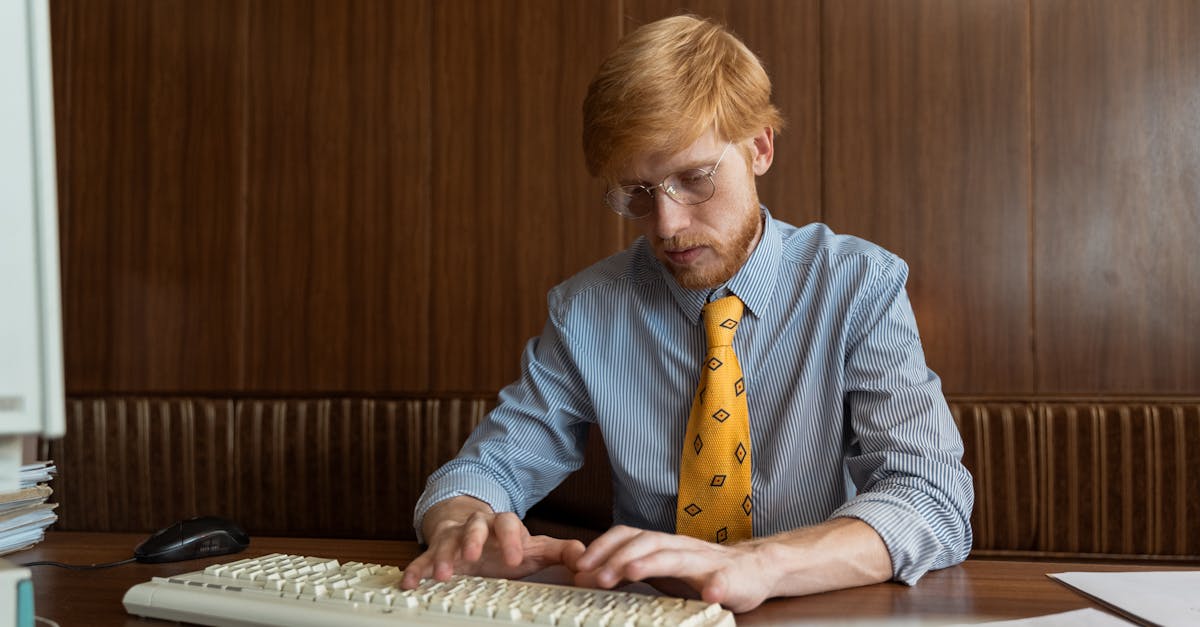
The advancement of technology has led to significant developments in the field of hardware, particularly in the area of signal processing. Signal processing applications, such as audio and video processing, noise reduction, data compression, and filtering, have become vital in various industries, including telecommunications, entertainment, and medical fields. One of the recent breakthroughs in this domain is the integration of ChatGPT-4, an AI-powered model, which can aid in the development of algorithms and techniques for signal processing applications.
Understanding ChatGPT-4
ChatGPT-4 is a state-of-the-art language model built on the GPT (Generative Pre-trained Transformer) architecture. It has been specifically designed to provide knowledgeable and coherent responses to various prompts, making it highly versatile in solving complex problems. With its advanced hardware technology, ChatGPT-4 offers unparalleled capabilities to process and analyze signals efficiently, paving the way for improved signal processing techniques.
Application in Signal Processing
The integration of ChatGPT-4 in signal processing applications opens up a plethora of possibilities. Let's explore some key areas where this technology can be utilized:
Audio and Video Processing
ChatGPT-4 can assist in the development of robust algorithms for audio and video processing. With its ability to understand natural language and generate coherent responses, it can improve the accuracy and efficiency of speech recognition systems, audio synthesis, and video analysis. By harnessing this technology, researchers and engineers can develop innovative methods for enhancing audio quality, removing background noise, and enhancing visual recognition in videos.
Filtering and Noise Reduction
Noise reduction is a critical process in signal processing, as it helps in eliminating unwanted distortions and improving signal clarity. ChatGPT-4 can aid in the development of advanced filtering techniques, enabling the removal of noise and interference from signals. This can be particularly beneficial in applications such as telecommunications, where clear voice transmission is essential, or in medical imaging, where precise diagnostics depend on accurate signal processing.
Data Compression
Efficient data compression techniques are crucial in various industries to reduce storage requirements and enable faster data transmission. ChatGPT-4 can contribute to the development of novel compression algorithms that can effectively reduce the size of audio, video, and image files without significant loss in quality. This would not only enhance data storage capabilities but also improve network efficiency, making data transfer faster and more scalable.
Advantages and Potential Impact
The integration of ChatGPT-4 in signal processing applications offers several advantages and can have a significant impact:
Enhanced Efficiency
By utilizing ChatGPT-4, researchers and engineers can save time and effort in developing algorithms for signal processing applications. Its ability to generate human-like responses and understand natural language allows for faster prototyping and testing, resulting in accelerated advancements in signal processing techniques.
Improved Accuracy
ChatGPT-4's advanced hardware technology enables more accurate signal processing, leading to enhanced results in areas such as noise reduction and data compression. Its sophisticated algorithms can analyze signals with precision and make intelligent decisions, minimizing errors and improving overall performance.
Broad Applications
The versatility of ChatGPT-4 makes it applicable in various signal processing domains. Whether it's telecommunications, multimedia, or medical fields, this technology can be utilized across industries to address specific challenges and develop innovative solutions.
Future Developments
As hardware technology continues to advance, the potential for integrating more powerful AI models like ChatGPT-4 further grows. This can lead to breakthroughs in signal processing techniques, enabling us to create even more efficient algorithms for advanced applications. The future holds immense possibilities for harnessing the power of hardware technology to push the boundaries of signal processing.
Conclusion
ChatGPT-4, with its hardware technology, is a game-changer in the field of signal processing. Its integration opens up exciting opportunities for developing advanced algorithms and techniques in applications such as audio and video processing, noise reduction, filtering, and data compression. As this technology continues to evolve, we can anticipate remarkable advancements in signal processing, revolutionizing various industries and improving the way we process and analyze signals.
Comments:
Thank you all for reading my article on ChatGPT for signal processing in hardware technology. I hope you found it insightful. I'll be happy to answer any questions or discuss any comments you may have!
Great article, James! I think ChatGPT has incredible potential in the field of signal processing. It could revolutionize hardware technology applications. Do you think there are any specific challenges in implementing ChatGPT for real-time signal processing?
I agree with Michael. The potential is immense! James, I'd like to know your thoughts on the computational requirements for implementing ChatGPT in hardware devices. Is there a risk of increased energy consumption?
Michael and David, thank you for your kind words! Indeed, implementation challenges exist in terms of computational requirements. Using ChatGPT in real-time signal processing would require powerful hardware, but progress is being made in optimizing such models for efficiency. While energy consumption might increase, the potential benefits it offers make it a promising direction worth exploring.
James, your article sheds light on an interesting application of ChatGPT. However, I wonder if the model's ability to generate text-based responses could limit its effectiveness for signal processing, where numerical analysis is often needed. What do you think?
Good point, Emily. While ChatGPT's text-based responses might seem less suitable for numerical analysis, it doesn't mean it cannot be used in conjunction with other algorithms or as a support system. For complex signal processing tasks, a hybrid approach might be more effective.
James, I really enjoyed your article. I believe natural language processing can make hardware technology more user-friendly and intuitive. Do you think ChatGPT could eventually be integrated into consumer products like smartphones or home automation systems?
Hi Lisa, thank you for your feedback! Absolutely, integrating ChatGPT into consumer products is an exciting possibility. It could enhance the user experience and make interacting with technology more intuitive. However, there might be challenges in balancing computational requirements and affordability for mass-market deployment.
James, I think utilizing ChatGPT for signal processing in hardware technology raises concerns about data privacy and security. How can we ensure that sensitive information is not exposed through the interaction with the model?
Excellent point, Jonathan. Maintaining data privacy and security is crucial when leveraging ChatGPT for such applications. Techniques like secure data transmission, encryption, and proper access controls can help mitigate these risks. Ethical considerations and robust safeguards need to be in place before widespread adoption.
I'm curious about the training process for ChatGPT in the context of signal processing. James, could you explain how the model is trained to recognize and respond to different types of signals?
Hi Amy, training ChatGPT for signal processing involves a multi-step process. Initially, the model is trained on a large dataset containing various signal types and corresponding responses. It learns patterns and relationships through supervised learning. Then, fine-tuning is done on specific tasks to increase its domain expertise. The combination of a diverse dataset and task-specific fine-tuning empowers the model to understand and respond to different signals.
James, I appreciated your mention of potential limitations in using ChatGPT for signal processing. Do you think developing explainability techniques for the model's decision-making process would be beneficial? It could be crucial, especially when dealing with critical hardware systems.
Absolutely, Oliver. Explainability is a significant concern when deploying AI models in critical systems. Developing techniques to understand the decision-making process of ChatGPT would not only ensure transparency but also enable better error detection and analysis. Explainability should be a priority to build trust in the model's outcomes, especially in high-stakes scenarios.
James, your article highlights the potential of ChatGPT in signal processing, but could there be any ethical implications in relying heavily on AI for hardware technology? For example, if an AI-based system makes a wrong decision that causes harm.
Sophia, you raise a critical concern. Ethical implications are an important aspect of adopting AI in hardware technology. Proper validation, rigorous testing, and fallback mechanisms are vital to prevent harmful decisions. A balance must be struck between the advantages of AI and the need to ensure safety, fairness, and accountability in hardware technology applications.
James, your article showcases the potential of ChatGPT in hardware technology. However, have there been any real-world implementations of ChatGPT for signal processing so far? It would be interesting to know about any practical examples.
Thanks for your question, Ryan. While ChatGPT is still relatively new, there have been some exploratory implementations for signal processing applications in research and experimental settings. However, large-scale real-world implementations may still be limited. Continued research and development are needed to fully unleash its potential.
James, your article got me thinking about the broader implications of ChatGPT in hardware technology. How do you envision its impact on job roles in the industry? Could it potentially replace certain roles or create new ones?
Great question, Ethan. The impact of ChatGPT on job roles in the industry is an important topic. While it might automate certain repetitive tasks, it could also create new roles focused on supervising and training AI models, data curation, or ensuring ethical deployment. The collaboration between humans and AI is likely to evolve rather than complete replacement. Adaptability and upskilling will be key for professionals in the industry.
James, I appreciate your article on ChatGPT for signal processing. Are there any existing alternatives to ChatGPT for signal analysis in hardware technology that you would recommend exploring?
Hi Sophie, thank you for your feedback! While ChatGPT shows promise, there are other signal processing techniques like Fast Fourier Transform (FFT), wavelet transforms, or adaptive filters that have been widely used and have their advantages in specific cases. The choice of technique depends on the requirements and constraints of the hardware technology application.
James, your article introduces an interesting application of ChatGPT. I'm curious if the model can handle real-time processing of signals with high sampling rates? Does it have any limitations in this regard?
Melissa, great question! ChatGPT's ability to handle real-time processing with high sampling rates might be limited due to computational requirements and response generation times. However, with advances in hardware and optimization techniques, it's possible to improve its performance. Balancing efficiency and accuracy in real-time processing is an ongoing area of research.
James, I enjoyed reading your article on ChatGPT for signal processing. Considering the rapid advancement of AI, do you think ChatGPT will become an industry standard for hardware technology in the future?
Thank you, Daniel! While it's difficult to predict the future, ChatGPT certainly has the potential to become an industry standard for certain applications in hardware technology. However, it's important to remember that technology landscapes are diverse, and different applications might require different approaches. The industry will continue to evolve, and AI models like ChatGPT will play a significant role in driving innovation.
James, I found your article on ChatGPT for signal processing intriguing. Do you have any recommendations for further reading or resources on this topic?
Certainly, Grace! If you're looking for further reading, I can recommend a few resources. 'Deep Learning for Signal Processing' by Li Deng and Dong Yu provides a comprehensive overview. 'Signal Processing for Machine Learning' by Michael J. Matarazzo is another excellent resource. These books delve into the intersection of signal processing and AI, laying the groundwork for exploring ChatGPT's potential in hardware technology.
James, your article opened my eyes to the potential of ChatGPT in signal processing. Are there any ongoing research efforts or challenges in this domain that you find particularly interesting?
Thanks, Aaron! Ongoing research efforts focus on optimizing ChatGPT's computational requirements, improving real-time processing capabilities, and enhancing domain expertise in signal processing. Overcoming these challenges requires interdisciplinary collaboration and innovative solutions. It's an exciting and evolving field with plenty of opportunities for further exploration.
James, I appreciate your article on ChatGPT for signal processing. Could you shed some light on the potential applications of this approach in fields outside of hardware technology?
Certainly, Liam! ChatGPT's applications extend beyond hardware technology. In fields like customer service, virtual assistants, or even content creation, ChatGPT can facilitate interactive and engaging experiences. It can be leveraged as a decision support system, an educational tool, or even for creative writing. The versatility of ChatGPT makes its potential applications vast and diverse.
James, your article brings up an interesting point about incorporating ChatGPT into hardware technology. Are there any notable limitations or drawbacks we should be aware of when considering its implementation?
Hi Hannah, great question! While ChatGPT shows promise, it's worth noting a few limitations. It may generate plausible but incorrect responses, and it heavily relies on the quality and diversity of training data. Concerns about data privacy, model explainability, and potential biases also need attention. It's crucial to address these limitations to ensure responsible and reliable use of ChatGPT in hardware technology.
James, your article highlights the potential of ChatGPT in hardware technology. How do you foresee its integration with existing signal processing algorithms? Can they work together effectively?
Alex, great question! Integration of ChatGPT with existing signal processing algorithms can be advantageous. While ChatGPT can provide contextual understanding and generate responses, traditional algorithms like FFT or wavelet transforms can handle numerical analysis and specific signal processing tasks more efficiently. Combining the two can create a powerful hybrid approach that leverages the strengths of both.
James, your article has me thinking about the potential impact of ChatGPT in the hardware technology industry. Do you foresee any regulatory challenges in the widespread adoption of this technology?
Noah, you raise an important point. The widespread adoption of ChatGPT in hardware technology may indeed face regulatory challenges. Issues related to data privacy, security, accountability, and fairness need to be addressed through appropriate regulations and frameworks. Ethical considerations and responsible deployment should be at the forefront. Collaboration between industry, researchers, policymakers, and society as a whole will be crucial in navigating these challenges.
James, your article on ChatGPT for signal processing was informative. Do you think there are any potential risks or ethical concerns in relying heavily on AI models like ChatGPT for critical hardware systems?
Emma, you bring up an important topic. Relying heavily on AI models like ChatGPT for critical hardware systems does carry inherent risks. The potential for incorrect responses, biases, or unforeseen failure modes requires careful validation, testing, and appropriate fail-safe mechanisms. Mitigating these risks and ensuring the responsible and ethical use of AI in critical systems should be a priority.
James, I found your exploration of ChatGPT for signal processing intriguing. Are there any specific areas within hardware technology that you find particularly suitable for the application of ChatGPT?
Thanks, Nathan! ChatGPT has potential applications in various areas of hardware technology. For example, interactive user interfaces, troubleshooting aids, or intelligent control systems could benefit from its natural language processing capabilities. It can also support data analysis, anomaly detection, or predictive maintenance tasks. The suitability of ChatGPT depends on the specific requirements and objectives of the application.
James, your article on ChatGPT for signal processing left me wondering about the computational requirements. Are there any advancements or techniques that can help address the potential energy consumption challenges?
Olivia, great question. Researchers are actively working on optimizing models like ChatGPT to reduce computational requirements and energy consumption. Techniques such as model compression, quantization, and efficient hardware accelerators can significantly improve efficiency. By striking a balance between model size, accuracy, and computational resources, we can address the energy consumption challenges and drive more sustainable AI implementations.
James, your article opened up an intriguing application of ChatGPT. With regards to hardware technology, do you think there are any unique challenges in training ChatGPT for signal processing compared to other domains?
Excellent question, Grace! Training ChatGPT for signal processing does have some unique challenges compared to other domains. Signal processing often requires domain-specific knowledge, pre-processing techniques, or specialized datasets. Ensuring the model captures the intricacies of different signal types and responds appropriately requires careful curation of training data and domain-specific fine-tuning. The challenge lies in striking the right balance between generality and domain expertise for effective signal processing.
James, your article highlights the potential of ChatGPT in signal processing. Are there any additional limitations or considerations when implementing ChatGPT in hardware systems with resource constraints?
Oliver, when implementing ChatGPT in hardware systems with limited resources, there are additional considerations. Model size and resource requirements need to be carefully managed to ensure feasibility. Techniques like model distillation, knowledge distillation, or efficient model architectures can address these constraints while preserving performance. Adapting the model to resource-constrained environments is an active area of research to make ChatGPT more accessible.
James, your article on ChatGPT in hardware technology was thought-provoking. Can you elaborate on the potential benefits of using ChatGPT over rule-based approaches in signal processing?
Thank you, Adam! ChatGPT's benefits in signal processing lie in its ability to generalize and adapt. Unlike rule-based approaches, ChatGPT can learn from data, handle complex and diverse signals, and generate context-aware responses. It can also improve over time with additional training and fine-tuning. By leveraging the power of machine learning, ChatGPT offers greater flexibility and potential for optimization in signal processing tasks.